ABSTRACT
In the automotive industry, a great challenge of production scheduling is to sequence cars on assembly lines. Among a wide variety of scheduling approaches, academics and manufacturers pay close attention to two specific models: Mixed-Model Sequencing (MMS) and Car Sequencing (CS). Whereas MMS explicitly considers the assembly line balance, CS operates with sequencing rules to find the best car sequence fulfilling the assembly plant requirements, like minimising work overload for assembly workers. Meanwhile, automakers including Renault Group are increasingly willing to consider other requirements, like end-to-end supply chain matters, in production planning and scheduling. In this context, this study compares MMS- and CS-feasible solution spaces to determine which workload-oriented sequencing model would be the most appropriate to later integrate new optimisation. We introduce two exact methods based on Dynamic Programming to assess the gap between both models. Numerical experiments are carried out on real-life manufacturing features from a Renault Group assembly plant. They show that MMS generates more feasible sequences than CS regardless of the sequencing rule calculation method. Only the sequencing rules used by real-life production schedulers result in a higher number of distinct feasible sequences for CS, highlighting that the plant might select a sequence with work overload situations.
Acknowledgments
We would like to thank all anonymous reviewers for their consistent suggestions which helped us significantly improve this study.
Data availability statement
The authors confirm that the data supporting the findings of this study are available within the article.
Disclosure statement
No potential conflict of interest was reported by the author(s).
Additional information
Notes on contributors
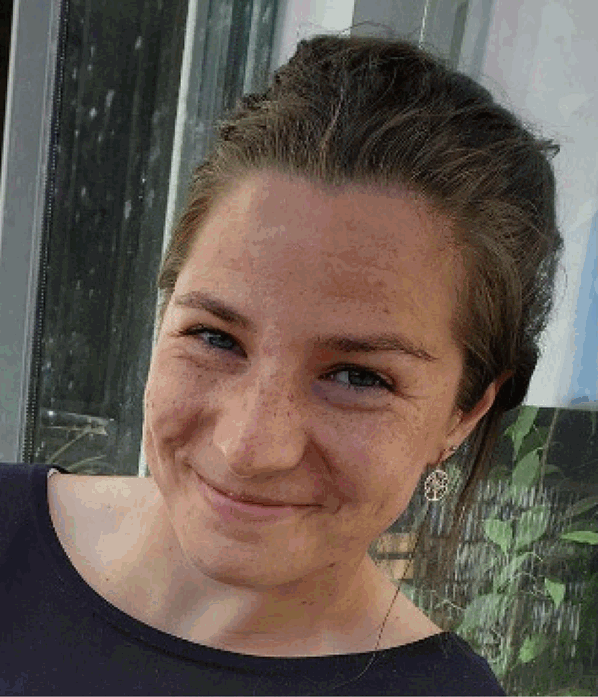
Adèle Louis
Adèle Louis is an Industrial Engineering graduate from Grenoble Institute of Technology. Her research interests are in modelling and optimisation of production and service systems. She has been working in the Production Control Process Engineering at Renault Group Supply Chain while undergoing her Ph.D. studies at University Grenoble Alpes, France. She graduated in 2022.
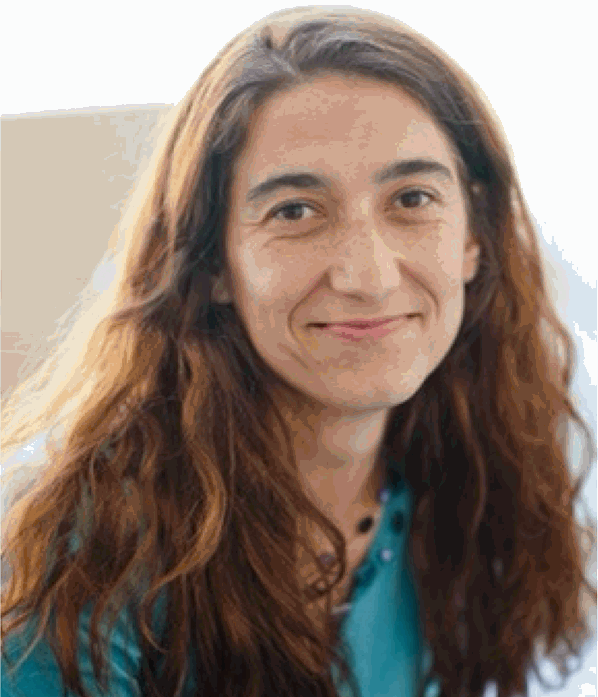
Gülgün Alpan
Gülgün Alpan is Professor of Industrial Engineering at Grenoble Institute of Technology, University Grenoble Alpes, France. Her research interests are in modelling, analysis and optimisation of production and service systems. Currently, she works on hybrid methods combining artificial intelligence with optimisation and simulation techniques, and she holds an Industry 4.0 Chair within the Multidisciplinary Institute on Artificial Intelligence in Grenoble. Gülgün Alpan is graduated from Rutgers University, New Jersey, USA, with M.Sc. and Ph.D. degrees in Industrial and Systems Engineering, in 1994 and 1997, respectively.
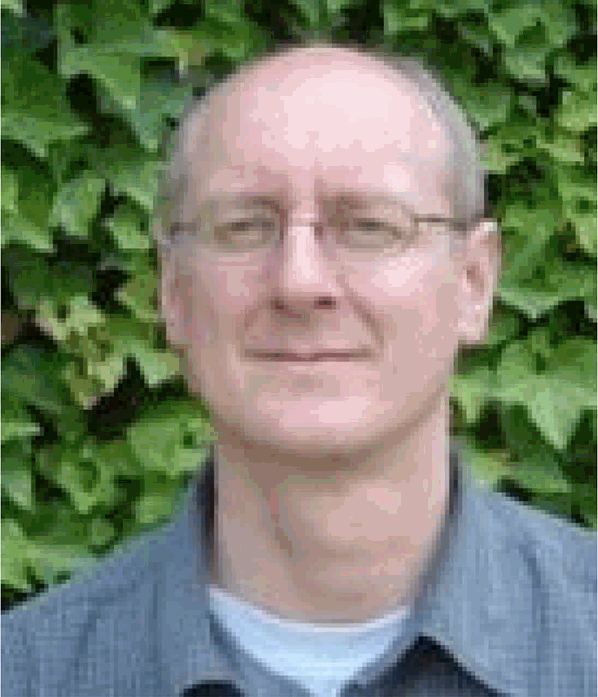
Bernard Penz
Bernard Penz is Professor of Industrial Engineering at Grenoble Institute of Technology, University Grenoble Alpes, France. His research interests are in modelling, analysis and optimisation of production and service systems. Currently, he works on scheduling, lot sizing and transportation problems. Bernard Penz is graduated from Université Joseph Fourier, Grenoble, France, with M.Sc. and Ph.D. degrees in Operations Research and Applied Mathematics, in 1990 and 1994, respectively.
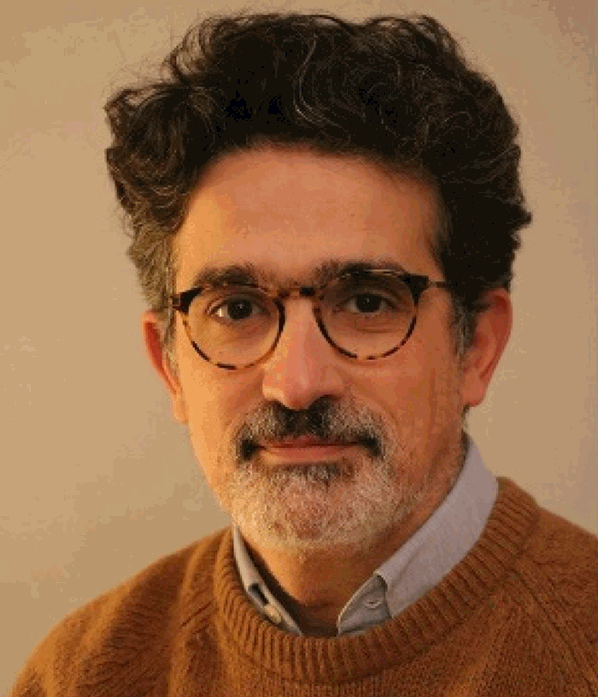
Alain Benichou
Alain Benichou is General Manager for Production Control Process Engineering at Renault Group Supply Chain, France. Production planning & scheduling has been the common thread of his career at Renault since 1995 (information systems, process, operations). Alain Benichou is graduated from Ecole Centrale de Lyon, France, in 1990.