Abstract
We study an integrated optimisation problem with blending, scheduling, and routing components for a melted material blending production system. The problem is formulated as a mixed-integer linear programming model that considers the blending machine environment, due dates, target amounts, required chemical compositions of the products, and ready times of the materials in containers. This model aimed to determine the container pairings, blending plants for container pairs, and schedules for blending operations while minimising the total end time of material usage, total penalty for violating component specifications, and employee workload. Further, we propose a three-stage approach that involves solving a relaxed problem and then resolving the problem with fixed variables. We developed a combinatorial Benders decomposition algorithm with a minimal infeasible subsystem identification algorithm for the blending scheduling problem. The experimental results indicate that the proposed method can find high-quality solutions within a reasonable amount of time.
Data availability statement
The data that support the findings of this study are available from the corresponding author, B.-I. Kim, upon reasonable request.
Disclosure statement
No potential conflict of interest was reported by the author(s).
Additional information
Funding
Notes on contributors
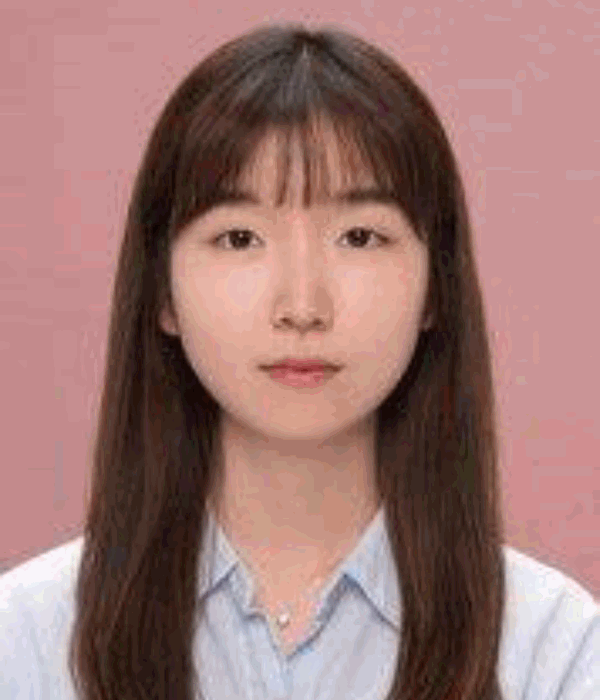
Myungeun Eom
Myungeun Eom is currently a Ph. D. candidate in Department of Industrial and Systems Engineering at Georgia Institute of Technology. She received her B.S. and M.S. degrees in Industrial and Management Engineering from POSTECH, Korea in 2018 and 2020, respectively. Her research interests include discrete optimisation, dynamic systems, and logistics.
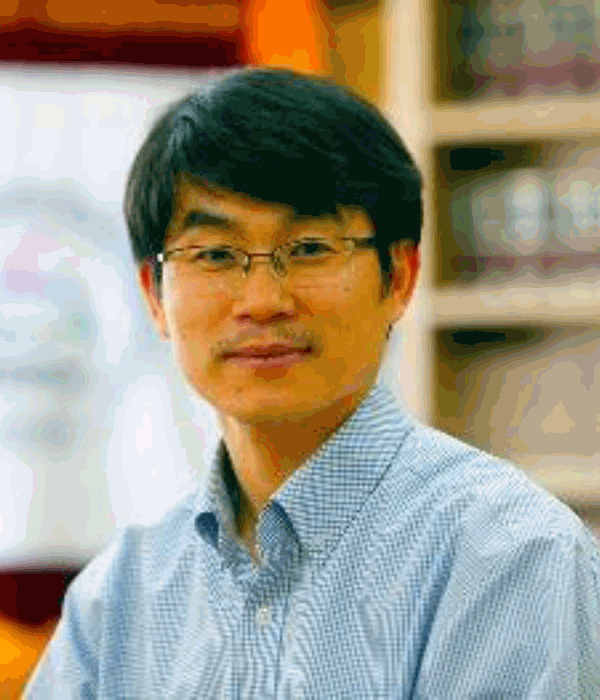
Byung-In Kim
Byung-In Kim is a Professor and the Head of the Department of Industrial and Management Engineering at POSTECH, Korea. He received his B.S. and M.S. degrees in Industrial Engineering from POSTECH, Korea, and Ph.D. degree in Decision Sciences and Engineering Systems from Rensselaer Polytechnic Institute, Troy, New York, in 1991, 1994, and 2002, respectively. He was an Assistant Professor of Industrial and Systems Engineering at the University of Memphis and the Director of Research and Development at Institute of Information Technology, Inc., The Woodlands, Texas. His research interests include vehicle routing problems, industrial optimisation problems, traffic signal optimisation, and logistics. He received the 2004 Franz Edelman Finalist Award from INFORMS, 2013 Hyun-Woo Management Science Award from the Korean Operations Research and Management Science Society, and 2018 POSCO Smart Innovation Award from POSCO. He is an associate member of the National Academy of Engineering of Korea, fellow of Asia Pacific Industrial Engineering and Management Society, and vice president of the Korean Institute of Industrial Engineers. He has more than 60 papers published in international journals such as International Journal of Production Research, European Journal of Operational Research, and IISE Transactions.