Abstract
The primary objective of this study was to develop a novel data-driven machine learning-based multiobjective robust optimisation strategy to improve the overall quality of multivariate manufacturing processes. The new strategy was conceptualised considering a manufacturing environment with unreplicated non-normal data observations and limited opportunity for off-line sequential design of experiments. At a macro level, the new strategy adopts suitable artificial intelligence-based process models and a fine-tuned non-dominated sorting genetic algorithm-II (NSGA-II) to derive robust efficient process setting conditions. These robust solutions are iteratively derived considering process model predictive uncertainties, process setting sensitivities, and variance-covariance structure of uncontrollable multivariate non-normal inputs (or covariates). These solutions are also ranked based on multicriteria decision-making (MCDM) techniques to facilitate implementation. In this study, the quality of the best-ranked solutions was compared (w.r.t. closeness to specified multiple targets and predicted multivariate output variabilities) with those of the solutions obtained from parametric and commercial software-based approaches using three different real-life manufacturing cases.
Data availability statement
Due to commercial and legal restrictions, organisations of the case studies did not agree for their data to be shared publicly, so supporting data are not available.
Disclosure statement
No potential conflict of interest was reported by the author(s).
Additional information
Notes on contributors
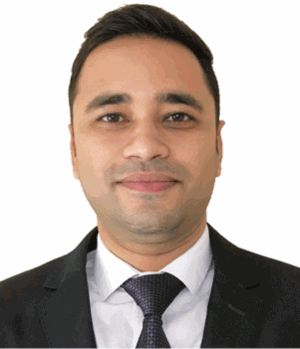
Abhinav Kumar Sharma
Abhinav Kumar Sharma is working as an Assistant Professor at the School of Business Management, Narsee Monjee Institute of Management Studies, Mumbai, India. He did his Ph.D. from Shailesh J. Mehta School of Management, Indian Institute of Technology Bombay, India. He did his Master’s degree in Mechanical Systems Design from the Indian Institute of Information Technology, Design and Manufacturing, Kancheepuram, Tamil Nadu, India. His research works have appeared in international journals and conference proceedings. His primary area of research interest is quality control and quality management.
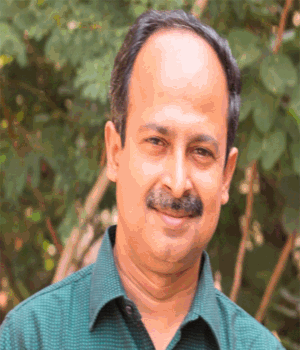
Indrajit Mukherjee
Indrajit Mukherjee is working as a Professor in Shailesh J. Mehta School of Management, IIT Bombay. He did his Ph.D. from the Industrial Engineering and Management Department, Indian Institute of Technology, Kharagpur. He did his Master’s degree in Quality, Reliability & Operations Research from Indian Statistical Institute, Kolkata, India. He has authored and co-authored several research papers published in refereed journals. His research contributions are published in the European Journal of Operational Research, Journal of Manufacturing Systems, Quality and Reliability Management, Quality Engineering, Computers & Industrial Engineering. His primary research interest is quality control, quality management, and sourcing in the supply chain.
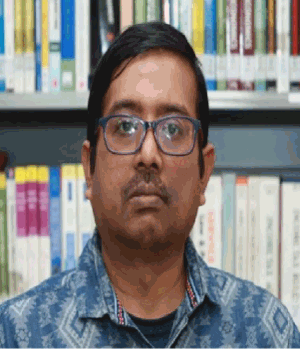
Sasadhar Bera
Sasadhar Bera is working as an Associate Professor at the Indian Institute of Management Ranchi, Jharkhand, India. He completed his Ph.D. from Shailesh J. Mehta School of Management, Indian Institute of Technology Bombay, India. He did his Bachelor’s degree in Mechanical Engineering from the National Institute of Technology (NIT) Durgapur, West Bengal, India, and a Master’s degree in Quality, Reliability, and Operations research from Indian Statistical Institute Kolkata, India. His research works appeared in various international journals like the European Journal of Operational Research, Quality Engineering, and the International Journal of Advanced Manufacturing Technology. His research interests include quality control, quality management, and multivariate data analytics.