Abstract
Visibility across supply chains has been a key concern for organisations for many years, but the tools and information systems to achieve real-time visibility have not been available until recently. In response to uncertainty and complexity, advanced information and communication technologies have been explored for supply chain visibility (SCV). However, managerial perspectives are largely absent from the current literature. In response, this paper systematically documents managerial factors influencing SCV and information that should be collected and shared among supply chain partners for better visibility. A multi-stage Delphi analysis was conducted with 26 supply chain experts from various globally recognised enterprises with manufacturing units located worldwide. The results provide details on prioritised managerial perspectives and experiences within (1) factors affecting SCV (drivers, enablers, challenges and contingencies), (2) SCV content (supplier, internal and customer information) and (3) implications of SCV (capabilities and performance effects). One observation was that forecasting is not deemed as important due to severe disruptions in supply chains. Real-time visibility for better predictability emerged as the top priority. This study is among the few that empirically explores factors influencing supply chain visibility and generates new insights into why barriers can be difficult to overcome in complex supply chain settings.
1. Introduction
In any crisis: decision, speed and accuracy are of the utmost importance for coping with changing realities and can be the difference between success and failure (Handfield and Linton Citation2017). The pandemic has shown that visibility is required to enable critical decision-making accuracy (Finkenstadt and Handfield Citation2021; Modgil, Singh and Hannibal Citation2021). In a study on conflict material disclosures, Swift et al. (Citation2019) found that firms can attain operational and market benefits by improving visibility in their supply chains. If supply chain visibility (SCV) is limited, the supply chain actors must act on incomplete, inaccurate and outdated information that can potentially lead to forecast errors, bullwhip effects, inventories of the wrong size and supply chain disruptions. Such unexpected events can lead to delays in deliveries and reduced materials, productivity and revenue for manufacturing companies across the globe (Yu and Goh Citation2014).
SCV refers to ‘the extent to which actors within a supply chain have access to or share information which they consider as key or useful to their operations and which they consider will be of mutual benefit’ (Barratt and Oke Citation2007). Organisations find it challenging to prepare the internal logistics and to quickly respond to unexpected events, due to low inter- and intra-site visibility (Srinivasan and Swink Citation2018). Collecting and sharing real-time data related to the order process, inventory, delivery and potential supply chain disruptions is not a well-adopted practice in production units (Bregman, Peng and Chin Citation2015). Additionally, because of low SCV, focal firms find it difficult to monitor all the actors involved in an efficient way. Thus, the visibility of the extended supply chain requires visibility of internal as well as external operations.
Hence, visibility is important for efficient decision-making, but difficult to achieve in practice, making SCV a key concern for organisations. Much of the extant literature on SCV focuses on technological advances, and digital technologies such as artificial intelligence (Modgil, Singh and Hannibal Citation2021), blockchain (Wang, Chen and Zghari-Sales Citation2021), cloud-based systems (Giannakis, Spanaki and Dubey Citation2019) and RFID (van Hoek Citation2019) have been proposed as important for achieving enhanced visibility through out the supply chain. Though previous studies have contributed to the understanding of SCV on a general level, the managerial perspectives are largely absent in the available literature (Somapa, Cools and Dullaert Citation2018; Calatayud, Mangan and Christopher Citation2019). To better understand the practitioners’ view on SCV, a multi-stage study was designed to address the following research questions (RQs):
RQ1: Which information is crucial to be made visible in a supply chain?
RQ2: What factors affect the implementation of SCV?
RQ3: What are the implications of SCV?
We employed an extensive Delphi study with 26 industry professionals from a variety of manufacturing industries as panel experts to seek their perspectives about SCV. The study contributes to the understanding of SCV by providing broad managerial perspectives. Based on the outcome of the Delphi study, we present a model that links factors affecting SCV, SCV content and implications of SCV. The model captures multiple aspects of SCV that can guide supply chain managers in planning, implementing and improving SCV in manufacturing supply chains. For researchers, it provides an overview of the practical aspects of managing SCV, highlighting the complexity of SCV.
This paper is structured as follows: First, we review the related literature. Second, we describe the Delphi methodology and the approach used in this research. We then present the results of the Delphi study, followed by the discussion section, where we present the SCV model that is based on the inputs from the Delphi study. Finally, in the conclusion section, we answer the research questions, present managerial and research implications, discuss limitations and suggestions for further research.
2. Related literature
Although the area of SCV is relatively new, three latest systematic literature reviews are available: Somapa, Cools and Dullaert (Citation2018), Calatayud, Mangan and Christopher (Citation2019) and Kalaiarasan et al. (Citation2022). Somapa et al. (Citation2018) include articles up to 2016 that focus on the characteristics and effectiveness of SCV. They found that SCV can be grouped into three main characteristics: automational, informational and transformational. Automational characteristics refer to the ability to automatically collect information and transfer it to business partners. Informational characteristics are based on the quality of information in terms of accuracy, timeliness and completeness. Transformational characteristics refer to the use of the information exchanged to enhance the operational and strategic activities of companies. They concluded that most previous studies have focused on either automational and/or informational characteristics, only a handful incorporating SCV effectiveness into their transformational characteristics.
Calatayud et al. (Citation2019) include articles up to 2018. They propose a supply chain model referred to as the ‘self-thinking supply chain’. This was predominantly enabled by Internet of things (IoT) and ‘artificial intelligence’ (AI) technologies, which make supply chains autonomous and increase predictive capacities. Based on their results, they further claim that IoT and AI are mostly associated with handling future supply chain challenges, supporting businesses in attaining higher levels of visibility.
Kalaiarasan et al. (Citation2022) include articles up to 2020 and propose a holistic framework for SCV driven by the insights from the content analysis of 47 empirical research papers. The framework covered a multitude of factors that either affect or were affected by SCV. In addition, they proposed an agenda for future research directions.
All these reviews call for more research on the managerial and organisational perspectives of SCV. Based on their literature review, Somapa et al. (Citation2018) claimed that challenges to establishing SCV are more related to organisational factors than technological factors. In line with this, Calatayud et al. (Citation2019) encouraged research investigating managerial perspectives on how self-thinking supply chains enabled by SCV might affect aspects of supply chains, such as power, balance and policies. Kalaiarasan et al. (Citation2022) proposed multiple-case or Delphi studies, involving a variety of firms, focusing on understanding which type of data are crucial to be managed for SCV.
2.1. Data elements of SCV
Supply chain visibility (SCV) requires management efforts to collect information about operations upstream, downstream and internally (within business processes) throughout the supply chains (Sodhi and Tang Citation2019). The extant literature includes a variety of data elements that are considered essential to make visible in the supply chain. These data elements can be divided into two broad areas: internal visibility and external visibility. Internal visibility refers to the collecting, recording and sharing of data within a business’ processes. It includes all the means, procedures and elements needed to record and share data along the internal supply chain. Internal tracking takes place when products or raw materials arrive, are stored in inventory and are processed internally in potentially various stages; the end product is then delivered to the customer or an inventory position in the distribution system. It involves an intra-site, real-time visualisation system for material management with tracking and tracing functionality. External visibility, on the other hand, refers to the products or goods outside of a business entity in the upstream and downstream supply chain; it concerns dynamic status information and the prediction of supply network status among supply chain partners and across different geographical areas. For external SCV to be effective, it relies on the existence and appropriate design of internal visibility in each entity (Somapa, Cools and Dullaert Citation2018).
2.2. Factors affecting SCV
Previous studies have provided factors to consider when developing and implementing SCV. In essence, these can be divided into two main categories: managerial- and technical/technological-related factors. One factor that demands a managerial perspective is inter-organisational collaboration. Previous studies have accentuated that inter-organisational collaboration is key to increasing SCV (Srinivasan and Swink Citation2018; Swift, Guide and Muthulingam Citation2019). Brun, Karaosman and Barresi (Citation2020) indicated that SCV can be achieved through increased collaboration amongst supply chain partners. With regards to inter-organisational collaboration, trust is another major factor affecting the establishment and further improvement of SCV (Dubey et al. Citation2020). Brun, Karaosman and Barresi (Citation2020) claim that there is a direct correlation between the level of trust and the established SCV. Studies have also pointed out that information sharing is a factor leading to increased levels of SCV. For example, Dubey et al. (Citation2019, Citation2020) found that investing in and gathering resources to share information can enhance SCV. Furthermore, Barratt and Oke (Citation2007) indicated that the quality of information is important for SCV. It has also been stated that work cultures encouraging information sharing function as a prerequisite to attaining SCV (Doetzer Citation2020).
Technical and technological factors have been mentioned in both older and more recently published studies. Munir et al. (Citation2020) noted that SCV can be enhanced by external integration collecting reliable and accurate information. Collaborative systems are also presented as technological factors in aiding real-time visibility (Barratt and Oke Citation2007; Barratt and Barratt Citation2011). For example, Giannakis et al. (Citation2019) highlighted that cloud-based collaborative systems are key to enabling end-to-end SCV. Technological infrastructure supporting connectivity is another factor considered to be a prerequisite for achieving SCV (Dubey et al. Citation2019, Citation2020). The compatibility of IT infrastructure amongst supply chain partners is crucial to ensuring visibility through out the supply chain (Kyu Kim, Yul Ryoo and Dug Jung Citation2011). In the past, several studies have identified RFID as a key technology for attaining SCV. Specifically, RFID is key to attaining visibility as goods move along the supply chain (Caridi, Perego and Tumino Citation2013; Pero and Rossi Citation2014). Yu and Goh (Citation2014) mentioned that higher investments in RFID are likely to lead to higher levels of SCV. Van Hoek (Citation2019) stated that RFID can coexist with blockchain technology to attain increased levels of SCV. The application of blockchain can improve visibility in areas such as order management (Agrawal et al. Citation2022) and product life cycle (Liu et al. Citation2020). Higher levels of SCV can also be attained by combining blockchain with additive manufacturing (Kurpjuweit et al. Citation2021). Based on their studies, Wang, Chen and Zghari-Sales (Citation2021) and Wang et al. (Citation2019) mentioned that blockchain contributes to higher levels of SCV for tracking and tracing.
2.3. The benefits of SCV
The benefits of SCV can be envisioned in two steps: a direct effect in terms of the analytics capability of the firm and an indirect effect on operational performance (Srinivasan and Swink Citation2018). The benefits reported in other studies fall into either of these two categories. For example, agility (Williams et al. Citation2013), risk management (Sarker et al. Citation2016), enhanced decision-making (Handfield and Linton Citation2017) and planning capabilities (Caridi et al. Citation2014; Ghasemy Yaghin and Goh Citation2021) are all examples of analytics capabilities.
The expected improvements in performance through increased SCV can improve cost management. For instance, SCV can aid in the identification and reduction of costs, leading to an overall improvement in supply chain performance (Lee, Kim and Kim Citation2014; Srinivasan and Swink Citation2018). In addition, SCV can enhance social (Brun, Karaosman and Barresi Citation2020) and environmental sustainability (Wang et al. Citation2019). Both Brun et al. (Citation2020) and Wang et al. (Citation2019) argued that SCV is essential for enhancing combined total sustainability. Furthermore, SCV has a positive effect on customer service (Williams et al. Citation2013; Caridi et al. Citation2014) and improves the ability to respond to customer requirements (Swift, Guide and Muthulingam Citation2019). Ultimately, a higher level of profitability can be gained through increased levels of SCV (Lee, Kim and Kim Citation2014; Swift, Guide and Muthulingam Citation2019).
2.4. Synthesis of the literature
There is a growing number of published papers on SCV, indicating its importance for extended supply chains. Table summarises the data elements, factors and benefits of SCV that are identified in the literature and discussed in sections 2.1–2.3. However, the extant literature for instance, Somapa, Cools and Dullaert (Citation2018), Calatayud, Mangan and Christopher (Citation2019) and Kalaiarasan et al. (Citation2022), predominantly focused on the technological aspects of SCV and did not thoroughly explore managerial perspectives on how to deal with SCV, such as identifying or prioritising factors or understanding the benefits and requirements of implementation. Still, some studies have acknowledged that it is up to the managers to understand the merits of SCV and make decisions about its implementation. However, we still lack a thorough study on the managerial aspects of SCV, which this study addresses.
Table 1. Summary of data elements, factors and benefits of SCV identified in the literature.
3. Delphi methodology
This study followed a Delphi method to address the research questions. Developed by Dalkey and Helmer (Citation1963) at the Rand Corporation, Delphi is a well-established methodology used to collect expert-based empirical data to attain consensus on multifaceted and multidisciplinary questions. In this systematic approach, a group of experts—with extensive experience in the subject under study—interact anonymously in multiple rounds. The rounds continue until a saturation point or consensus is reached among the experts. Responses from each Delphi round are used as input to the next round in the form of the aggregate response. During each round, the experts can reflect on and change their responses. This helps in avoiding adverse effects related to interpersonal biases, dominating personalities, defensive attitudes and unproductive disagreements. Delphi research also creates opportunities to gain valuable insights from practising managers/industry experts and is suitable for exploratory theory building. In the first round, the experts traditionally answer open-ended questions that stimulate extensive, descriptive, independent and associative responses enablingl the generation of new concepts. In consecutive rounds, either the open-ended approach can be repeated or (more often) the experts rank or rate previously derived constructs in several dimensions. As a consensus-based Delphi approach is deemed suitable for analysing the evolution and potential implications of new phenomena, this study follows the method of examining and understanding factors that influence SCV, especially after the pandemic. In the past, Delphi-based approaches have been adopted in various research studies for needs assessments, policy development and forecasting (Ogden et al. Citation2005; Melnyk et al. Citation2009). In supply chain management, applications of the Delphi approach are found in studies investigating factors that influence decision making (see, e.g. Agrawal and Pal Citation2019; Kembro, Näslund and Olhager Citation2017; Hassan et al. Citation2015). Therefore, the Delphi approach is appropriate for exploring the factors that influence SCV and crucial information sets made visible in a supply chain. Other methods—for instance, surveys—tend to use a very limited set of items to describe SCV factors. In addition, some literature discusses factors that affect SCV, while other works discuss the effects of having SCV in place. To the best of our knowledge, there are no studies that focus on the managerial perspective on SCV, which forms the motivation for this research and for using a Delphi study to capture the perceptions of industrial supply chain experts. This study follows a four-round process, as shown in Table and discussed in the following section.
Table 2. The Delphi methodology followed in the study.
3.1. Expert selection
We followed the general guidelines for Delphi studies (Melnyk et al. Citation2009; Kembro, Näslund and Olhager Citation2017) to set up an appropriate panel to ensure the validity of the results. The experts should have extensive experience and knowledge related to the specific issue under study. We invited senior supply chain executives from large and medium-sized industrial firms in Sweden with global supply chains. These experts were primarily drawn from, or recommended by, industry contacts and research partners. All invited executives had at least 10 years of experience in global SCM. Overall, 34 SCM practitioners were invited, and 26 agreed to participate in the Delphi expert panel. They represented a variety of industries: aerospace, automotive, fast-moving consumer goods, food and beverage, forest and garden equipment, furniture, heat transfer, heavy industrial goods, optoelectronics, packaging, steel and telecommunication. An overview of our 26 panel experts is provided in Appendix A. This number is in the recommended range for Delphi studies, as less than 20 risks individual biases while more than 30 sometimes generates few additional insights and limits the exploration of insights that emerge (Ogden et al. Citation2005; Agrawal and Pal Citation2019).
3.2. First round: exploring the issues related to SCV
The first round consists of open-ended questions: (i) ‘Please describe in your own words what supply chain visibility means to you, your company and your supply chains. Please provide examples’, (ii) ‘Why is supply chain visibility important to your company and your supply chains?’, (iii) ‘What factors affect the visibility of your supply chains and how?’ and (iv) ‘Is there anything else that you think is important in the context of supply chain visibility that you would like to add?’ The first three questions refer to three fundamental perspectives on SCV and are linked to the three research questions addressed in this study. The questions were easy to comprehend, thus avoiding ambiguous responses. No supporting information or framework concerning SCV (e.g. from the available literature) were provided to the panellists in an attempt to avoid guiding the respondents in a particular direction.
For the first, second and third rounds, experts were given three weeks, in each round, to respond, directly by email or through the attached Microsoft Word documents. Reminders were sent every week, and the researchers’ details were provided in case of any queries. The responses were analysed individually by the four researchers (one PhD student, one postdoctoral researcher and two professors) to identify potential themes and categories. Coding was compared, and in most cases, there was full agreement among the researchers. Differences in interpretations were thoroughly re-examined and resolved in consensus.
3.3. Second round: reaching an understanding
Based on the responses from the first round, an exhaustive list of factors and crucial SCV information were compiled in a Word document. Information was categorised under three headings: information concerning ‘market and product’, ‘internal’ operational data and ‘supply’ data. Factors were categorised into those affecting SCV, that is, ‘enabler’, ‘drivers’, ‘barriers/challenges’ and ‘contingencies’, and those concerning the implications of SCV, that is, ‘capabilities’ and ‘performance’. Experts were asked to indicate the factors and pieces of information that they considered ‘important’ for SCV and those that they considered ‘not important’. Provision for commentary input was provided with each factor and piece of information. The responses were analysed for each factor and piece of information by counting the number of experts that indicated ‘important’, ‘not important’ or ‘no response’. Based on the results, an understanding was reached concerning the important factors and pieces of information (those with a high number of ‘important’ counts), while the factors and information with a high number of ‘not important’ counts were eliminated from further consideration (see Appendix B).
3.4. Third round: quantifying the impact of different factors
In the third round, experts were asked to rate the factors and information on a Likert scale of one to seven. For data/information, enablers and drivers, we asked the experts to rate how important each factor is for SCV (1 = not important and 7 = very important). For capabilities and performance effects, we asked the experts to rate how each factor is likely to be affected by an implementation or improvement of SCV (1 = not at all affected and 7 = extremely positively affected). Similarly, for barriers/challenges, we asked them to rate how difficult (or impossible) it is to overcome and solve a factor in implementing SCV (1 = not even a challenge and 7 = barrier). Finally, for contingencies, we asked how important SCV is in these situations (1 = not important and 7 = very important) and how feasible (1 = infeasible and 7 = very infeasible) it is to achieve a successful implementation of SCV in these situations. The responses were analysed for each factor and piece of information by calculating the mean and standard deviation. Based on the results, factors and information were ranked from highest to lowest. Again, factors with very low ratings were dropped (see Appendix B).
3.5. Fourth round: focus group seminars
As a fourth round, two focus group seminars were organised to facilitate maximum participation. During the webinar, the results from the third round were presented. Special emphasis was given to factors with high standard deviation to understand the possible causes of dispersed responses. This was followed by a discussion on supply chain visibility to allow for knowledge- and experience-sharing among experts from various leading manufacturing companies. The input from the seminars was transcribed and used as supporting arguments while discussing the results.
4. Results
In this section, we present our findings from the Delphi study. The structure draws on the collective findings from the four rounds in the Delphi study and is broadly divided into managerial perspectives and experiences within three areas, as illustrated in Figure .
Figure 1. Input-output model of supply chain visibility (SCV).

We first present the content of supply chain visibility, that is, the set of data for which visibility is important along the supply chain, as perceived by the Delphi experts. We then present the factors that affect SCV and, finally, review the implications of SCV. In each section, we discuss the factors that the expert group identified in this study (from stages 1 and 2) and the quantitative results that provide the relative impact of the various factors (from stage 3).
4.1. Data for which visibility is important
After examining the results of the first Delphi round, data elements that are crucial for SCV could be divided into three categories: customer-related, internal and supplier-related. To avoid complexities, we included only the most important elements. These elements were further filtered as per the results of the second round, and only the most important ones were carried forward to the third round. Table summarises the crucial data elements in the three categories obtained in the third Delphi round. The elements appear in descending order of importance within the category, based on the mean rating obtained from the experts. The element with the highest mean is perceived as the most crucial data element for SCV.
Table 3. Data elements that are important for supply chain visibility.
These data elements are crucial, and actors should make efforts to capture them internally and share them externally among actors through a common database to attain the desired positive impacts of SCV. This was supported by experts, with one of the experts mentioning the following: ‘Visibility is about having the same information as your partners in the SC at the same time. It is also about having good tools to visualise forecasts, plans and orders. A good BI [business intelligence] tool with the same information is key to analysing the current status and the future. Common SC master data is important.’ It can be observed from Table that information related to deliveries, deviations (customer-related), inventory levels, capacity, lead time (internal), purchasing plans and supplier capabilities (supplier-related) got high ratings and was considered very crucial for better SCV. As per an expert, ‘Part of visibility in supply chains is about making information about capacity, lead time, order volume and inventory development visible by creating better processes for demand planning, capacity planning, phasing in and phasing out, etc. Another dimension of visibility in supply chains is about making information available and visible internally within the company and to other stakeholders in the supply chain, e.g. suppliers, carriers, etc.’
Regarding customer- and supplier-related data, one of the experts mentioned the following: ‘[For] visibility in supply chains, you should have access to data on customers’ needs, suppliers’ location, capacity and lead time, logistics suppliers’ flows, capacity, lead time and all mapped risks available in real time visually so you can act, plan, customise and wing up in real time’. Another expert added: ‘Visibility means that it is possible to see the status of key figures in the supply chain in a clear, correct and easily accessible way—preferably in the form of interactive ‘dash-boards’ and reports—and that in the event of deviations or changes it is easy to ‘drill down’ in current areas to make an analysis and identify corrective actions. For example: [the] scheduled production start of a production order. This has consequences for the entire supply chain, including on purchased material which is to be postponed and also on the delivery of the order. This must be discovered so that information and corrective action can be taken.’
4.2. Factors affecting SCV
The Delphi panel identified a broad set of factors affecting SCV, which the research team categorised into four themes: drivers, enablers, challenges and contingency effects. The themes are presented in separate sections.
4.2.1. Drivers of SCV
Drivers are factors that cause a specific phenomenon to happen, grow or develop. Experts listed several drivers for SCV. The less important ones were eliminated in the second Delphi round. Table lists all the important factors driving SCV in manufacturing SC. These are arranged in descending order of their importance based on the mean rating obtained from the experts.
Table 4. Drivers for supply chain visibility.
Among these, managing deviations in SC and improving SC control emerged as the most crucial drivers of SCV. Some of the experts even had detailed thoughts and comments in relation to the same. They mentioned, ‘To know and understand the full value chain [through SCV] gives you the possibility to make proactive initiatives instead of reactive. Supply chain risk management has become increasingly important, given the recent years’ development with trade barriers, pandemic natural disasters etc.’, and ‘Through [SCV], as early as possible, [we] identify deviations and problems to maintain a good decision-making process with relevant facts and consequences for the alternatives that exist. We work actively and proactively towards the most suitable solution where we actively choose the path and understand the consequences that it entails. For us, it is about capital efficiency, customer value and risk mitigation.’
One of the experts mentioned that better SC control is essential for the legal aspect that drives SCV: ‘Visibility is important for [our company], both downstream and upstream. We have worked hard with the life cycle aspect, and then good visibility is needed in the supply chain. Upstream, we want control of the supply chain, as we place strict demands on our tier 1 suppliers and we expect them to pass on the same requirements to tier 2, tier 3, etc. There are also legal aspects to this.’
It was interesting to observe that despite so many ongoing efforts and discussions around sustainability and overall sustainable industrial transformation, factors concerning sustainability still received relatively low ratings. The reasons, as perceived by the experts, were that (a) sustainability-related factors should be considered as performance effects rather than drivers, and (b) sustainability factors are related to processes rather than data-driven. As explained by one expert, ‘I believe that [sustainability-related factors] will be kind of covered anyway in the new processes [we implement], so they are kind of secondary [for us]. We will have them anyway because we are building things in this new circular process that will take care of the sustainability issues’.
4.2.2. Enablers for SCV
Enablers are factors that make it possible for a particular phenomenon to happen or be done. The experts listed several enablers that facilitate SCV implementation. Table lists all the crucial enablers for SCV. They are also arranged in descending order of their importance based on ratings from the experts.
Table 5. Enablers for supply chain visibility.
Data quality and information sharing are the top two enablers for SCV. As one of the experts highlighted, ‘When you get information, the resolution of the information and how accurate it is [plays a crucial role]. There should be a balance between these information attributes and the inherent variance that exists in the various activities and processes. For example, detailed information needs to be accurate to be meaningful; the information should at the same time come within the reaction time to generate maximum value.’
Regarding information sharing, one of the experts stated, ‘For us, it [SCV] mainly means to ‘connect the islands’ in our company. What I mean by that is that our different distribution centres and factories operate rather independently towards each other (more like a customer-supplier relationship). We are striving to automate and enhance the information sharing and ordering between the internal entities.’
Trust is another important enabler identified by one expert: ‘To be able to gain visibility in the supply chain, you need to gain trust from the suppliers, build a win-win relationship with your strategic partners. When you have trust, it’s easier to share information between buyers and suppliers’.
4.2.3. Challenges to SCV
Factors considered to be challenges require great effort to be completed successfully, whereas barriers simply block something (in this case, SCV) from happening. Table summarises all the factors that are considered challenges for implementing SCV. As indicated in the table, a score of ‘1’ signifies a factor that is not even considered a challenge, whereas a score of ‘7’ is considered a barrier. The third column indicates the number of experts who marked the factor as a barrier. Although most experts do not think that these factors are barriers, at least one expert perceives a factor to be a hindrance to SCV.
Table 6. Factors acting as challenges to supply chain visibility.
Many of the experts stated that the main barriers to SCV are a lack of standardisation, SC complexity, a lack of IT infrastructure and an unwillingness to share data. Lack of standardisation is the top challenge for implementing SCV systems. As the product moves along the stages of the supply chain, standardisation becomes a vital element for effective data sharing and technology integration. As mentioned by one expert, ‘If visibility means being able to “see” the supplier's supply chain, or my order's position against the plan in it, then that possibility is today greatly affected by existing IT systems that are rarely or never created for visualisation in this dimension’. Considering the many factors that act as challenges, creating successful SCV in practice seems like a most difficult task.
4.2.4. Contingency factors for SCV
Three contingencies were identified as important by the Delphi panel: customer order decoupling points (CODP)- Made-to-Stock (MTS) vs. Made-to-Order (MTO), supply chain complexity (low vs. high) and supply chain geographical dispersion (local vs. global). Supply chain complexity refers to the number of nodes, tiers, connections, etc., that need to be managed. The Delphi experts were asked to rate the importance (value) of having SCV in each situation as well as the feasibility of achieving SCV in each respective situation. Importance was rated along a seven-point scale, from ‘1’ = unimportant (no value) to ‘7’ = extremely important (extremely valuable), and feasibility was rated from ‘1’ = infeasible to ‘7’ = very feasible. The results, displayed in Table , show an interesting pattern. The two situations connected to a contingency factor (e.g. low versus high complexity) are rated inversely such that one is associated with a higher level of importance but a lower level of feasibility than the other. This holds true for all three contingency variables. This result is illustrated in Figure , where importance and feasibility are displayed as two dimensions.
Figure 2. Importance versus feasibility for contingency variables.
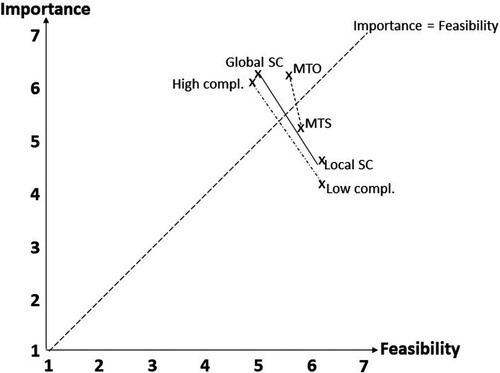
Table 7. Contingency variables for supply chain visibility.
4.3. Implications of SCV
Finally, we present the management perspectives on the implications of SCV. From the expert comments in the Delphi study, we concluded that the implications can be categorised into two sub-groups: capabilities and performance effects. The relationship between capabilities and performance effects is such that capabilities refer to the possibilities of utilising the data made visible through SCV effectively and efficiently, which in turn can lead to a better performance. Consequently, capabilities can be regarded as a direct effect, while performance as an indirect effect of SCV. Table presents the effect factors divided into the two sub-categories of capabilities and performance effects.
Table 8. Effects of supply chain visibility.
The key capabilities identified by the panel experts are concerned with forward-looking planning and control: predictive capability, decision-making support, supply chain control and planning capability. These four factors score between 6.10 and 5.65 on the 7-point scale. One expert emphasises the forward-looking aspect: ‘Supply chain visibility is an integral part of reaching a truly connected supply chain, which is one of our strategic movements in the coming years, as it will make us better equipped for addressing future challenges, identifying new opportunities and delivering even more value to our customers’. Just below the general planning and control capabilities on the ranking in Table , we find two specific areas—risk management and traceability—which have received increasing attention during the pandemic and will most likely continue to be two key areas in which SCV can be beneficial. This is emphasised by one expert: ‘[There is an] increasing importance of supply chain risk management, given the developments with trade barriers, the pandemic, natural disasters, etc. in recent years. It is important to know and understand the capacity availability in sub-tiers; one recent example is semi-conductors’.
The highest-ranking factor of the performance effects was customer service, which scored 6.10. Many experts rated it a 7, and one expert highlighted that customer service is the overarching goal: ‘Everything collapses to customer service’. Of the three dimensions of sustainability, social sustainability (4.40) is ranked lower than economic sustainability (5.40) and environmental sustainability (5.20), which reflects the historical development of these sustainability goals. Nevertheless, one expert exemplifies the importance of the social aspect: ‘For sustainability and CSR reasons, knowing how and where your ingoing components and raw materials are produced is important’.
5. Discussion
Our Delphi study systematically explored the perspectives that managers perceive to be relevant to SCV in one way or another. Figure displays the outcome of our Delphi study in terms of a conceptual model. It includes the subcategories drivers, enablers, challenges, contingencies, content, capabilities and performance effects (from left to right in Figure ).
Figure 3. SCV model of factors to consider.
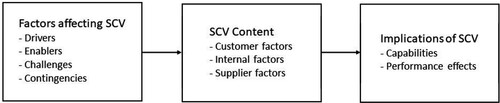
The model informs that there is a multitude of factors that affect SCV, and thereby the content and expected outcome of SCV. By combining the individual contributions from 26 panel experts, the SCV model captured the extant managerial perspectives on and priorities in SCV.
5.1 Factors affecting the SCV
The study showed that many factors affect SCV. The key drivers for SCV are managing deviations (Ghasemy Yaghin and Goh Citation2021), executing supply chain control and providing better customer service (Caridi et al. Citation2014). Moreover, in terms of enablers for SCV, data quality (Barratt and Oke Citation2007), information sharing (Dubey et al. Citation2020), top management support, trust (Brun, Karaosman and Barresi Citation2020), and inter-organisational collaboration (Swift, Guide and Muthulingam Citation2019) are considered the most important. It is worth observing that most of the soft factors such as top management support, trust and collaboration are perceived as key enablers for SCV by the managers. This is contrary to the findings from the available research literature (Kalaiarasan et al. Citation2022) that instead highlights hard factors such as technology as the key enabler. Nonetheless, there are certain major challenges, such as a lack of standardisation (Klueber and O’Keefe Citation2013) and supply chain complexities (Brun, Karaosman and Barresi Citation2020), that are perceived as barriers to implementing SCV. Due to the involvement of multiple partners, most located in distinct geographical locations and using a variety of information management tools, it becomes challenging to share information. Many organisations (e.g. Odette for the automotive industry) are working towards standardising information-sharing tools and protocols.
5.2 SCV content
The study led to an argument that complete SCV entails data elements related to customer, internal and supplier being visible among the supply chain actors. Customer-related information concerning deliveries and deviations was among the most crucial for SCV. The experts gave it a high rating with a low standard deviation in the mean response, depicting a strong agreement among the respondents. The results are quite evident in the pandemic, as up-to-date deliveries and deviation information helps supply chain managers plan operational activities to ensure smooth production and material flow within the supply chain. This supports the findings of Swift et al. (Citation2019). Concerning internal data elements, inventory level and capacity information are deemed crucial to ensure smooth production and on-time delivery. Finally, supplier-related information concerning purchasing plans and supplier capabilities is perceived as important, as it helps in strategic sourcing. An example of this is the recent semiconductor crisis, where insufficient visibility in supplier operations resulted in a major disruption in production at the buyers’ end. Nevertheless, information concerning ‘country of origin’ and ‘track and trace’ received comparatively low ratings and high standard deviations, which implies that this information was perceived as relatively less important by experts. On the other hand, due to high standard deviation, it is also notable that there is a high range of variation among the ratings. Upon further discussion with experts, we found that these data elements are crucial for some supply chains—for instance, textile (Agrawal and Pal Citation2019), pharmaceutical (Chiacchio et al. Citation2020) and food (Doukidis, Pramatari and Kelepouris Citation2007)—where traceability and knowledge of product origins is a major concern; meanwhile, other products, such as, for example, automotive and steel products, have well-established supply chains with close buyer-supplier relationships, and traceability is crucial for product recall (Dai et al. Citation2021).
5.3 Implications: capabilities and performance effects
The experts highlighted several capabilities and performance effects as implications of SCV. Predictive and planning capabilities (Ghasemy Yaghin and Goh Citation2021), along with decision-making support (De Giovanni Citation2020), were among the highest-ranked capabilities that the experts perceived to be gained by organisations due to SCV. Recently, these capabilities have been among the most crucial ones following the pandemic, Suez Canal incident and semiconductor crises. Companies are suffering considerably, and SCV has emerged as an important solution that can help organisations develop resilience by having better predictive capacity and control over supply chains. It was also interesting to observe that forecasting, which has been among the key capabilities gained by SCV, as per the literature, is not currently as important due to the severe disruptions in the supply chains. The experts believe that, even after effective forecasting and inventory control, production has been disrupted due to bad predictive capabilities. Real-time visibility for better predictability and decision support is salient. In terms of performance, customer service (Swift, Guide and Muthulingam Citation2019) and economic sustainability (Dubey et al. Citation2020) are ably guaranteed by SCV. With SCV, organisations can better understand customer demand and provide better services. Similarly, SCV contributes to effective resource utilisation and better productivity, thus resulting in better profitability and cost savings.
5.4 Interrelationships and contexts
We observe a connection between enablers and challenges, as well as between drivers and effects. Factors related to data appear both in enablers (data quality, common master data) and challenges (data from multiple sources). In fact, ‘standardisation’ was mentioned both as an enabler and challenge (the challenge was captured as ‘lack of standardisation’). Experts mentioned that standardisation is one of the key elements to facilitate SCV. However, much needs to be done with existing data capturing and sharing systems to allow interoperability. Thus, if an enabler is not in place, it becomes a challenge.
There is a strong relationship between drivers and effects. All drivers are also cited as expected effects of SCV—either as a capability or performance outcome. The top driver ‘managing deviations’ is related to the top capability ‘predictive capability’, while the other driver-effect combinations are identical, such as ‘supply chain control’, ‘decision making support’ and ‘risk management’ as drivers and capabilities, and ‘customer service’ along with all aspects of sustainability as drivers and performance outcomes. This is natural, since any attempt to initiate or improve SCV should include the perception that one or more capabilities and/or one or more performance measures should improve.
The results on contingencies (displayed in Table and Figure ) shows two types of contexts: one where SCV is more important than feasible (MTO, high complexity and global supply chains) and one where SCV is more feasible than important (MTS, low complexity and local supply chains). The first context is characterised by a higher level of complexity and uncertainty, which makes SCV important—at the same time, however, it may be difficult to implement it. The second context is related to lower levels of complexity and uncertainty, which may not require SCV due to the simple nature of the situation—even though SCV could be implemented. This finding suggests that there may be a tension between importance and feasibility, such that SCV is difficult to implement in important contexts, and that the feasible contexts do not require SCV due to contextual simplicity. It will be important to report on these three contingency variables (at least) when the context of an SCV implementation’s success is reported to improve our understanding of when and how SCV can contribute.
6. Conclusions
6.1 Answering the research questions
We addressed three research questions. RQ1 aimed to explore crucial information that should be made visible in a supply chain. We found multiple data elements and categorised them into three groups: customer-related, internal and supplier-related data, and listed them in their order of importance as perceived by the experts. The top priorities were data on deliveries and deviations (customer-related), inventory levels, capacity, and lead time (internal) and purchasing plans and supplier capabilities (supplier-related).
RQ2 targeted the factors that affect the implementation of SCV. These were categorised as drivers, enablers, challenges and contingencies. These were further refined and prioritised to identify the most important ones and to examine potential relationships among them. Managing deviation (driver), data quality (enabler) and lack of standardisation (challenge) were the top priorities. The view on contingencies suggests that there is a tension between importance and feasibility; SCV is less feasible in important contexts, but more feasible in less important contexts.
Finally, RQ3 aimed to explore and examine the implications of SCV. We answered the question by exploring various effects of SCV. Predictive capability and customer service (performance) effects were among those with high rating and effects perceived as important by the experts.
6.2. Managerial implications
This study can function as a reference point for managers. In particular, the SCV model provides useful insights to supply chains and logistics managers by providing managerial perspectives on SCV gathered from various industries, such as automotive, aerospace and telecommunication. First, the factors categorised as enablers (eight factors), drivers (eight), challenges (seven) and contingencies (three) can be used by managers to aid the discussion, planning and implementation of SCV. These different categories help managers to gain a holistic view of the factors or intricacies relating to SCV. It is also important for managers to understand that all factors identified do not necessarily have to be relevant in a particular practical situation. Nevertheless, the rating of factors and commentary inputs from experts would facilitate developing a customised SCV implementation strategy for their company. Managers should also recognise and understand that tensions between importance and feasibility can affect the willingness to attain higher levels of SCV. Secondly, the SCV model captured 16 prioritised data elements that should be displayed in a potential SCV system. It further communicates to managers that to fully benefit from SCV, a multitude of factors that are customer-, internal- and supplier-related have to be made visible. This information supports managers in decision making and facilitates proactive actions by providing insights regarding SC deviation to visualise for manufacturing supply chains. Third, the SCV model presents the possible implications of SCV in terms of both capabilities and performance effects that might not previously have been identified by the firm. This enables managers to determine how SCV can be used to improve both the operational and strategic performance of their supply chains and how vital SCV is for well-informed decision making to achieve the desired outcomes in terms of performance and capabilities.
6.3. Research implications
This research provides a state-of-the-art view of the practical issues of SCV. Most recent publications focus on technical and technological factors. Our study adds to the extant literature on SCV by providing empirical evidence on managerial perspectives. Furthermore, it identified new factors to consider for the planning, implementation and management of SCV. In particular, the factors can be used in survey research as a baseline for developing items and combining items into constructs. Together with previous studies, the results illuminate how SCV can support decision-making in practice. In the midst of the pandemic and digitalisation era, the identified implications of SCV also serve as guidance points for attaining both supply chain sustainability and resilience. Moreover, reflecting on a possible theoretical contribution, it was observed that SCV capabilities can deliver a competitive advantage especially in dynamic conditions. This Delphi study was conducted in the second and third quarters of 2021, that is, during the pandemic, which most likely has emphasised the dynamic nature of the surrounding environment in which the firms operate. Most of the managers prioritised SCV capabilities and performance effects like predictive capability, decision-making support, customer service and flexibility indicating SCV as key contributors to the dynamic capabilities for a gaining competitive advantage. Rich and real-time data from various sources along the supply chain can help organisations sense the deviations and reconfigure the resources to meet customer demands.
6.4. Limitations
A limitation of this research is that, although all experts have extensive experience with global supply chains, they are all based in Northern Europe. Since they represent many different industries with global presences, it is unlikely that another expert panel would arrive at a substantially different set of factors. However, as the area of SCV matures, other Delphi panels may identify additional factors that are important—for example, more contingency variables that affect SCV’s perceived importance and feasibility.
6.5. Future research
The results call for future research on SCV. We propose four directions. First, survey research that can explore other geographical areas to understand the relative importance of the factors that we identified in our study is warranted. Cultural aspects, the level of innovation, digitalisation, industrial priorities, data sharing regulations and governmental policies may differ and can impact factors of SCV. Second, most previous studies have been limited to the first-tier supplier or customer perspective. However, our results support the view that the full potential of SCV is related to the end-to-end supply chain. Hence, there is a need to further explore and understand the factors influencing SCV beyond first-tier suppliers. We therefore encourage more research on extended supply chains involving multi-tier suppliers. Third, more empirical studies on SCV, such as case studies, action research and best practices are warranted. This will support a deeper understanding of the factors and their interrelationships, for example, identifying more contingency factors. Fourth, a better understanding of the development and implementation of SCV systems in practice is needed. A systems approach can be useful for gaining more knowledge regarding the practical aspects of SCV systems. Thus, many interesting research avenues can be pursued.
Disclosure statement
No potential conflict of interest was reported by the author(s).
Data availability statement
The data that support the findings of this study are available from the corresponding author, [TKA], upon reasonable request.
Additional information
Funding
Notes on contributors
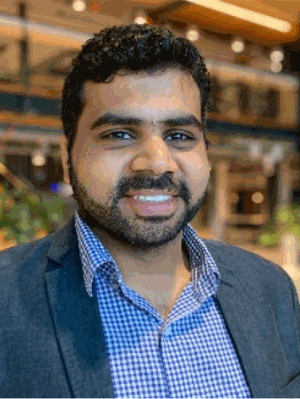
Tarun Kumar Agrawal
Tarun Kumar Agrawal is a Researcher in Production Logistics at KTH Royal Institute of Technology and Senior Lecturer in Logistics at Chalmers University of Technology. His research interest includes production logistic and supply chain information sharing, supply chain digitalisation, blockchain application in manufacturing industry and data driven decision-making. He graduated from ENSAIT, France with an MSc in Industrial Systems Engineering (2015) and conducted his PhD under Erasmus Mundus Fellowship by the European Commission. He holds Joint Doctorate (2019) in Production Automation from University of Lille, France, Engineering from Soochow University, China and Textile Management from University of Borås, Sweden.
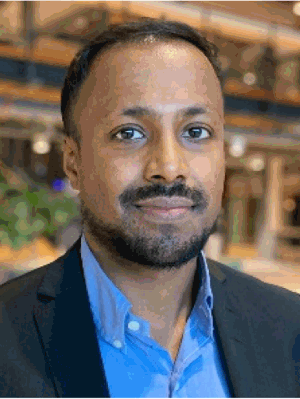
Ravi Kalaiarasan
Ravi Kalaiarasan, is an industrial PhD student at KTH Royal Institute of Technology and employed at Scania CV. His research focuses on data-driven supply chain visibility for informed decision making to improve supply chain performance. Prior to his doctoral studies, Ravi has been working within the automotive industry with work experiences from production, purchasing and IT. Ravi holds a M.Sc. in Production and Logistics from Mälardalens University.
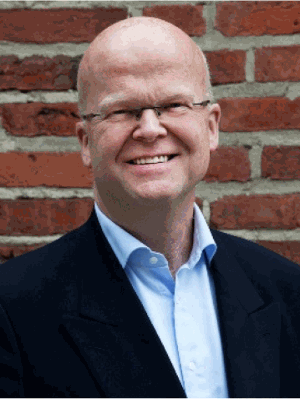
Jan Olhager
Jan Olhager is Professor in Supply Chain and Operations Strategy at Lund University. He received a M.Eng. in Industrial Engineering and Operations Research from University of California at Berkeley, and a Ph.D. in Production Economics from Linköping University. He is Honorary Fellow of EurOMA, European Operations Management Association. He is Associate Editor of Decision Sciences and IJOPM and serves on the editorial boards of IJPR and PPC. He has published more than 80 papers in international scientific journals and a couple of books. His research interests include international manufacturing networks, reshoring, operations strategy, supply chain integration, and operations planning and control.
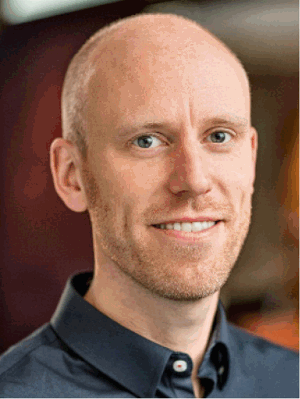
Magnus Wiktorsson
Magnus Wiktorsson is a Professor of Production Logistics at KTH Royal Institute of Technology. His research interest concerns how complex production logistic systems can be described and predicted. The application areas are within manufacturing industry and his research is based on digitisation within supply chains and the need for transformation into environmentally sustainable production. Magnus Wiktorsson has a professional background with experience from business, government and universities. He graduated from the KTH Royal Institute of Technology in Stockholm with an MSc in Systems Engineering (1995) and a PhD in Manufacturing Engineering (2000). He is also head of the department for Sustainable Production Development as well as the department for Production Engineering at KTH.
References
- Agrawal, Tarun Kumar, Jannis Angelis, Wajid Ali Khilji, Ravi Kalaiarasan, and Magnus Wiktorsson. 2022. “Demonstration of a Blockchain-Based Framework Using Smart Contracts for Supply Chain Collaboration.” International Journal of Production Research 0: 1–20. doi:10.1080/00207543.2022.2039413.
- Agrawal, Tarun Kumar, and Rudrajeet Pal. 2019. “Traceability in Textile and Clothing Supply Chains: Classifying Implementation Factors and Information Sets via Delphi Study.” Sustainability 11 (6): 1698. doi:10.3390/su11061698.
- Barratt, Mark, and Ruth Barratt. 2011. “Exploring Internal and External Supply Chain Linkages: Evidence from the Field.” Journal of Operations Management 29 (5): 514–528. doi:10.1016/j.jom.2010.11.006.
- Barratt, Mark, and Adegoke Oke. 2007. “Antecedents of Supply Chain Visibility in Retail Supply Chains: A Resource-Based Theory Perspective.” Journal of Operations Management 25 (6): 1217–1233. doi:10.1016/j.jom.2007.01.003.
- Bregman, Robert, David Xiaosong Peng, and Wynne Chin. 2015. “The Effect of Controversial Global Sourcing Practices on the Ethical Judgments and Intentions of U.S. Consumers.” Journal of Operations Management 36 (May): 229–243. doi:10.1016/j.jom.2015.01.001.
- Brun, Alessandro, Hakan Karaosman, and Teodosio Barresi. 2020. “Supply Chain Collaboration for Transparency.” Sustainability 12 (11): 4429. doi:10.3390/su12114429.
- Calatayud, Agustina, John Mangan, and Martin Christopher. 2019. “The Self-Thinking Supply Chain.” Supply Chain Management: An International Journal 24 (1): 22–38. doi:10.1108/SCM-03-2018-0136.
- Caridi, Maria, Antonella Moretto, Alessandro Perego, and Angela Tumino. 2014. “The Benefits of Supply Chain Visibility: A Value Assessment Model.” International Journal of Production Economics 151 (May): 1–19. doi:10.1016/j.ijpe.2013.12.025.
- Caridi, Maria, Alessandro Perego, and Angela Tumino. 2013. “Measuring Supply Chain Visibility in the Apparel Industry.” Benchmarking: An International Journal 20 (1): 25–44. doi:10.1108/14635771311299470.
- Chiacchio, Ferdinando, Lucio Compagno, Diego D’Urso, Luca Velardita, and Philipp Sandner. 2020. “A Decentralized Application for the Traceability Process in the Pharma Industry.” Procedia Manufacturing, International Conference on Industry 4.0 and Smart Manufacturing (ISM 2019), 42 (January): 362–369. doi:10.1016/j.promfg.2020.02.063.
- Dai, Bin, Yu Nu, Xia Xie, and Jianbin Li. 2021. “Interactions of Traceability and Reliability Optimization in a Competitive Supply Chain with Product Recall.” European Journal of Operational Research 290 (1): 116–131. doi:10.1016/j.ejor.2020.08.003.
- Dalkey, Norman, and Olaf Helmer. 1963. “An Experimental Application of the DELPHI Method to the Use of Experts.” Management Science 9 (3): 458–467. doi:10.1287/mnsc.9.3.458.
- De Giovanni, Pietro. 2020. “Blockchain and Smart Contracts in Supply Chain Management: A Game Theoretic Model.” International Journal of Production Economics 228 (October): 107855. doi:10.1016/j.ijpe.2020.107855.
- Doetzer, Mathias. 2020. “The Role of National Culture on Supply Chain Visibility: Lessons from Germany, Japan, and the USA.” International Journal of Production Economics 230 (December): 107829. doi:10.1016/j.ijpe.2020.107829.
- Doukidis, Georgios, Katerina Pramatari, and Thomas Kelepouris. 2007. “RFID-Enabled Traceability in the Food Supply Chain.” Industrial Management & Data Systems 107 (2): 183–200. doi:10.1108/02635570710723804.
- Dubey, Rameshwar, Angappa Gunasekaran, David J. Bryde, Yogesh K. Dwivedi, and Thanos Papadopoulos. 2020. “Blockchain Technology for Enhancing Swift-Trust, Collaboration and Resilience Within a Humanitarian Supply Chain Setting.” International Journal of Production Research 58 (11): 3381–3398. doi:10.1080/00207543.2020.1722860.
- Dubey, Rameshwar, Angappa Gunasekaran, Stephen J. Childe, Thanos Papadopoulos, Constantin Blome, and Zongwei Luo. 2019. “Antecedents of Resilient Supply Chains: An Empirical Study.” IEEE Transactions on Engineering Management 66 (1): 8–19. doi:10.1109/TEM.2017.2723042.
- Finkenstadt, Daniel Joseph, and Robert Handfield. 2021. “Blurry Vision: Supply Chain Visibility for Personal Protective Equipment During COVID-19.” Journal of Purchasing and Supply Management 27 (3): 100689. doi:10.1016/j.pursup.2021.100689.
- Ghasemy Yaghin, R., and M Goh. 2021. “Procurement, Production, and Price Planning Under Visibility and Risk: A Generalised Benders Decomposition Method.” International Journal of Production Research 59 (18): 5626–5646. doi:10.1080/00207543.2020.1788735.
- Giannakis, Mihalis, Konstantina Spanaki, and Rameshwar Dubey. 2019. “A Cloud-Based Supply Chain Management System: Effects on Supply Chain Responsiveness.” Journal of Enterprise Information Management 32 (4): 585–607. doi:10.1108/JEIM-05-2018-0106.
- Handfield, Robert, and Tom Linton. 2017. The LIVING Supply Chain: The Evolving Imperative of Operating in Real Time. John Wiley & Sons.
- Hassan, Mayadah, Maged Ali, Emel Aktas, and Kholoud Alkayid. 2015. “Factors Affecting Selection Decision of Auto-Identification Technology in Warehouse Management: An International Delphi Study.” Production Planning & Control 26 (12): 1025–1049. doi:10.1080/09537287.2015.1011726.
- Kalaiarasan, Ravi, Jan Olhager, Tarun Kumar Agrawal, and Magnus Wiktorsson. 2022. “The ABCDE of Supply Chain Visibility: A Systematic Literature Review and Framework.” International Journal of Production Economics. doi:10.1016/j.ijpe.2022.108464.
- Kembro, Joakim, Dag Näslund, and Jan Olhager. 2017. “Information Sharing Across Multiple Supply Chain Tiers: A Delphi Study on Antecedents.” International Journal of Production Economics 193 (November): 77–86. doi:10.1016/j.ijpe.2017.06.032.
- Klueber, Roland, and Robert M. O’Keefe. 2013. “Defining and Assessing Requisite Supply Chain Visibility in Regulated Industries.” Journal of Enterprise Information Management 26 (3): 295–315. doi:10.1108/17410391311325243.
- Kurpjuweit, Stefan, Christoph G. Schmidt, Maximilian Klöckner, and Stephan M. Wagner. 2021. “Blockchain in Additive Manufacturing and Its Impact on Supply Chains.” Journal of Business Logistics 42 (1): 46–70. doi:10.1111/jbl.12231.
- Kyu Kim, Kyung, Sung Yul Ryoo, and Myung Dug Jung. 2011. “Inter-Organizational Information Systems Visibility in Buyer–Supplier Relationships: The Case of Telecommunication Equipment Component Manufacturing Industry.” Omega 39 (6): 667–676. doi:10.1016/j.omega.2011.01.008.
- Lee, Ho, Moon Sun Kim, and Kyung Kyu Kim. 2014. “Interorganizational Information Systems Visibility and Supply Chain Performance.” International Journal of Information Management 34 (2): 285–295. doi:10.1016/j.ijinfomgt.2013.10.003.
- Liu, X. L., W. M. Wang, Hanyang Guo, Ali Vatankhah Barenji, Zhi Li, and George Q. Huang. 2020. “Industrial Blockchain Based Framework for Product Lifecycle Management in Industry 4.0.” Robotics and Computer-Integrated Manufacturing 63 (June): 101897. doi:10.1016/j.rcim.2019.101897.
- Melnyk, Steven A., Rhonda R. Lummus, Robert J. Vokurka, Laird J. Burns, and Joe Sandor. 2009. “Mapping the Future of Supply Chain Management: A Delphi Study.” International Journal of Production Research 47 (16): 4629–4653. doi:10.1080/00207540802014700.
- Modgil, Sachin, Rohit Kumar Singh, and Claire Hannibal. 2021. “Artificial Intelligence for Supply Chain Resilience: Learning from Covid-19.” The International Journal of Logistics Management). doi:10.1108/IJLM-02-2021-0094.
- Munir, Manal, Muhammad Shakeel Sadiq Jajja, Kamran Ali Chatha, and Sami Farooq. 2020. “Supply Chain Risk Management and Operational Performance: The Enabling Role of Supply Chain Integration.” International Journal of Production Economics 227 (September): 107667. doi:10.1016/j.ijpe.2020.107667.
- Ogden, Jeffrey A., Kenneth J. Petersen, Joseph R. Carter, and Robert M. Monczka. 2005. “Supply Management Strategies for the Future: A Delphi Study.” The Journal of Supply Chain Management 41 (3): 29–48. doi:10.1111/j.1055-6001.2005.04103004.x.
- Pero, Margherita, and Tommaso Rossi. 2014. “RFID Technology for Increasing Visibility in ETO Supply Chains: A Case Study.” Production Planning & Control 25 (11): 892–901. doi:10.1080/09537287.2013.774257.
- Sarker, Sudipa, Mats Engwall, Paolo Trucco, and Andreas Feldmann. 2016. “Internal Visibility of External Supplier Risks and the Dynamics of Risk Management Silos.” IEEE Transactions on Engineering Management 63 (4): 451–461. doi:10.1109/TEM.2016.2596144.
- Sodhi, ManMohan S., and Christopher S. Tang. 2019. “Research Opportunities in Supply Chain Transparency.” Production and Operations Management 28 (12): 2946–2959. doi:10.1111/poms.13115.
- Somapa, Sirirat, Martine Cools, and Wout Dullaert. 2018. “Characterizing Supply Chain Visibility – a Literature Review.” The International Journal of Logistics Management 29 (1): 308–339. doi:10.1108/IJLM-06-2016-0150.
- Srinivasan, Ravi, and Morgan Swink. 2018. “An Investigation of Visibility and Flexibility as Complements to Supply Chain Analytics: An Organizational Information Processing Theory Perspective.” Production and Operations Management 27 (10): 1849–1867. doi:10.1111/poms.12746.
- Swift, Caroline, V. Daniel R. Guide, and Suresh Muthulingam. 2019. “Does Supply Chain Visibility Affect Operating Performance? Evidence from Conflict Minerals Disclosures.” Journal of Operations Management 65 (5): 406–429. doi:10.1002/joom.1021.
- van Hoek, Remko. 2019. “Exploring Blockchain Implementation in the Supply Chain.” International Journal of Operations & Production Management 39 (6/7/8): 829–859. doi:10.1108/IJOPM-01-2019-0022.
- Wang, Yingli, Catherine Huirong Chen, and Ahmed Zghari-Sales. 2021. “Designing a Blockchain Enabled Supply Chain.” International Journal of Production Research 59 (5): 1450–1475. doi:10.1080/00207543.2020.1824086.
- Wang, Yingli, Meita Singgih, Jingyao Wang, and Mihaela Rit. 2019. “Making Sense of Blockchain Technology: How Will it Transform Supply Chains?” International Journal of Production Economics, 211. doi:10.1016/j.ijpe.2019.02.002.
- Williams, Brent D., Joseph Roh, Travis Tokar, and Morgan Swink. 2013. “Leveraging Supply Chain Visibility for Responsiveness: The Moderating Role of Internal Integration.” Journal of Operations Management 31 (7): 543–554. doi:10.1016/j.jom.2013.09.003.
- Yu, Min-Chun, and Mark Goh. 2014. “A Multi-Objective Approach to Supply Chain Visibility and Risk.” European Journal of Operational Research 233 (1): 125–130. doi:10.1016/j.ejor.2013.08.037.
Appendix
APPENDIX A. Overview of the panel experts in our Delphi study
APPENDIX B. Factors that were excluded in round 2 and 3.
These factors that were excluded, since they were mentioned by very few panel experts (less than 4 persons out of 26). Moreover, majority of the excluded factors were mentioned by only one expert.
B1. Factors dropped in round 2.
B2. Factors dropped in round 3.
These factors were excluded, since they received very low importance scores, i.e. majority of the experts mentioned these as ‘not important’ or very few (less than 4) mentioned it as ‘important’