Abstract
The Joint Assembly Line Balancing and Feeding Problem (JALBFP) assigns a line feeding mode to each component (Assembly Line Feeding Problem) and each task to a workplace of a station (Assembly Line Balancing Problem). Current literature offers numerous optimisation models that solve these problems sequentially. However, only few optimisation models, provide a joint solution. To solve the JALBFP for a multi-manned assembly line, we propose a Mixed Integer Linear Programming (MILP) model and a heuristic that relies on the Adaptive Large Neighborhood Search (ALNS) framework by considering multiple workplaces per station and three different feeding policies: line stocking, travelling kitting and sequencing. The objective function minimises the cost of the whole assembly system which considers supermarket, transportation, assembly operations, and investment costs. Although the JALBFP requires higher computation times, it leads to a higher total cost reduction compared to the sequential approach. Through a numerical study, we validate the heuristic approach and find that the average deviation to the MILP model is around 1%. We also compare the solution of the JALBFP with that of the sequential approach and find an average total cost reduction of 10.1% and a maximum total cost reduction of 43.8%.
Acknowledgments
We would like to thank the editor and three anonymous reviewers for the careful reading of our manuscript and for the suggestions that helped us improve the manuscript.
Data availability statement
Due to the nature of the research in Section 5.3, participants of this study did not agree for their data to be shared publicly, so supporting data is not available.
The authors confirm that the data that support the findings of Section 5.4 are available within the article and its supplementary materials.
Disclosure statement
No potential conflict of interest was reported by the author(s).
Additional information
Notes on contributors
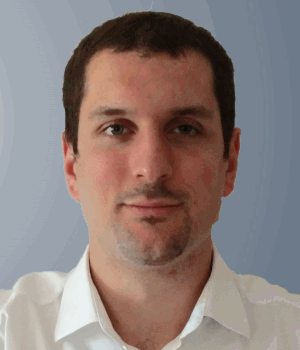
Francesco Zangaro
Francesco Zangaro is a Ph.D. candidate at the Ph.D. School of Mechatronics and Product Innovation Engineering of the University of Padua (UniPD) and at the Chair of Logistics and Supply Chain Management of the Technical University of Munich (TUM). His research interests are in part feeding optimisation and in-house logistics improvements.
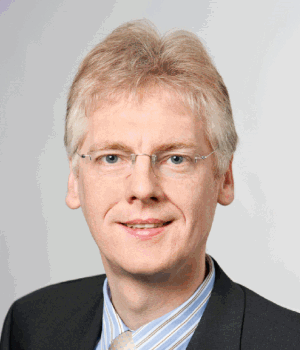
Stefan Minner
Stefan Minner is a Full Professor for Logistics and Supply Chain Management at the School of Management, Technical University of Munich (TUM). Currently, Stefan Minner is the Editor-in-Chief of the International Journal of Production Economics. His research interests are in business analytics, global supply chain design, transportation optimisation and inventory management.
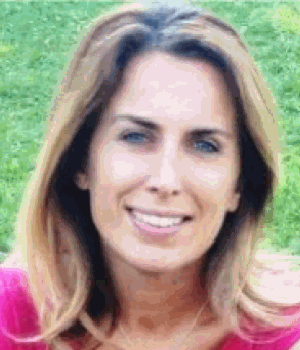
Daria Battini
Daria Battini is a full professor of Industrial Facilities Design and Industrial Logistics at the University of Padua (Italy) and she is the Coordinator of the Doctoral Course in Mechatronics and Product Innovation Engineering. She investigates supply chain design and management, industrial system optimisation, manufacturing system management for sustainability. She is the author and co-author of more than 100 publications in relevant international journals, about industrial logistics, material handling, materials management, supply chain, assembly system design.