Abstract
Conceptual design is a pivotal stage of new product development. The function-behaviour-structure framework is adopted in this stage to help designers search design space and generate conceptual solutions iteratively. Computer-aided methods developed within this framework will yield significant insight into facilitating the cognitive activities of designers. In order to solve the mapping process from behaviours to structures which is a typical constraint satisfaction problem, a cerebellar operant conditioning-inspired constraint satisfaction approach is proposed in this paper. The design constraints-driven operant conditioning and its regulation mechanism by the cerebellum are analysed for the first time. Proposition logic is applied to transfer the constraint satisfaction problem into a propositional satisfiability problem while an undirected graph is utilised to model design space. Inspired by the modularised cerebellar structure, a modularised constraint satisfaction neural network is constructed to determine the satisfiability of design problems. Conceptual solutions can be generated by clustering the embedding of nodes in this network. The proposed approach imitates the design constraint-driven operant conditioning to narrow down design space without assigning specific values to design components. It reduces design iterations and avoids combinatorial explosions during conceptual design.
Disclosure statement
No potential conflict of interest was reported by the author(s).
Data availability statement
The data that support the findings of this study are openly available in Mendeley Data.
Additional information
Funding
Notes on contributors
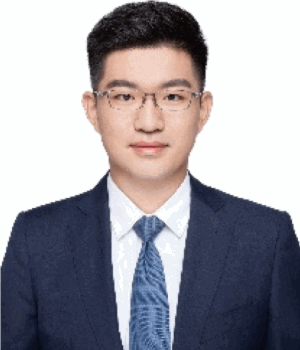
Mingdong Li
Mingdong Li received B.S degree in mechanical design, manufacturing and its automation from Tongji University, Shanghai, China, in 2018, and M.S degree in mechanical design and theory from Zhejiang University, Hangzhou, China in 2021. He is a Member of the State Key Laboratory of Fluid Power and Mechatronic Systems, Zhejiang University. His interests lie in the brain–machine interface, brain-inspired intelligence, and product innovative design.
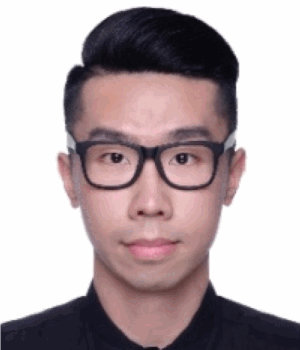
Shanhe Lou
Shanhe Lou received the B.S. degree in mechanical engineering and automation from Wuhan University of Technology, Wuhan, China, in 2015, and the Ph.D. degree in mechanical design and theory from Zhejiang University, Hangzhou, China, in 2021. He was a Member of the State Key Laboratory of Fluid Power and Mechatronic Systems, Zhejiang University. He is currently a Research Fellow with the Department of Mechanical and Aerospace Engineering, Nanyang Technological University, Singapore. His research interests include intelligent design and manufacturing, and brain-inspired intelligence.
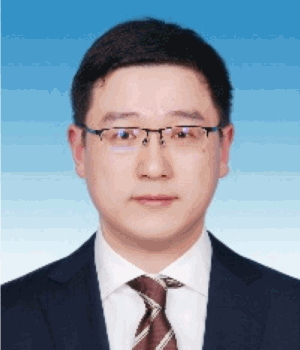
Yicong Gao
Yicong Gao received the B.S. degree in mechanical engineering from East China University of Science and Technology, Shanghai, China, in 2005, the Ph.D. degrees in mechanical engineering from Zhejiang University, Hangzhou, China, in 2011, respectively. He is currently an Associate Professor with the School of Mechanical Engineering of Zhejiang University, China and the member of State Key Laboratory of Fluid Power and Mechatronic Systems, Zhejiang University, China. His research focuses on mechanical product design theory, 4D printing, intelligent automation and advance manufacture technology. He has been the principal investigator of two projects supported by National Natural Science Foundation of China.
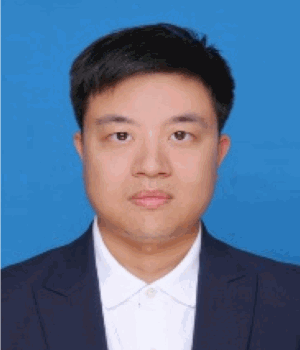
Hao Zheng
Hao Zheng received the B.S. degree in mechanical engineering from Southwest Jiaotong University, Chengdu, China, in 2010, and the Ph.D. degree in mechanical engineering from Zhejiang University, Hangzhou, China, in 2017. He is currently an Associate Research Fellow with the Hangzhou Innovation Institute of Beihang University, China. His research interests include data-driven design, decision-making and optimisation, multi-objective evolutionary algorithms, and human-machine integration.
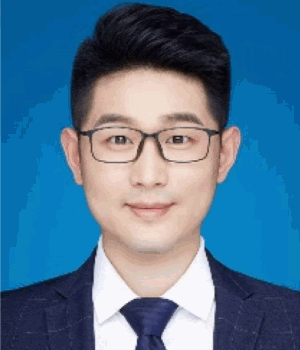
Bingtao Hu
Bingtao Hu received the B.S. and Ph.D. degrees in mechanical engineering from Zhejiang University, Hangzhou, Zhejiang, China, in 2014 and 2021, respectively. He is currently a Research Assistant with the Department of Mechanical Engineering and a Member of the State Key Laboratory of Fluid Power and Mechatronic Systems, Zhejiang University, China. His research interests include big data and design theory.
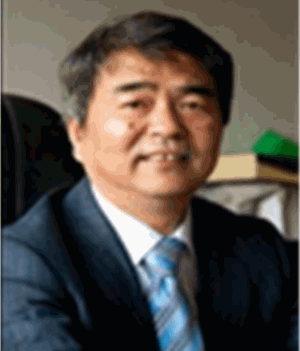
Jianrong Tan
Janrong Tan received the B.S. and M.S. degree in mechanical engineer and electronic engineering from The Open University of China, Beijing, China, in 1982, the M.S. degree in engineering from the Huazhong University of Science and Technology, Wuhan, China, in 1985, and the Ph.D. degree in mathematics from Zhejiang University, Hangzhou, China, in 1987. He is currently a specially appointed Professor in Zhejiang University, a Doctorial Supervisor and an Academician of the Chinese Academy of Science, the Dean of Mechanical Engineering, the Associate Supervisor of the CAD&CG State Key Laboratory, the Head of the Institute of Engineering & Computer Graphics, Zhejiang University, and the Chief Supervisor of Engineering Graphics State Fundamental Courses. His research focuses on mechanical designing and theory, and digitalised designing and manufacturing. He has published eight pieces of monograph or compile, 142 pieces of articles indexed by SCI/EI, with more than 1600 times citations. He gathered his 15 years of working experience in manufactory and his theory in science, and proposed the technique in the combination of batch and customisation which is used for multitudinous customisation, the status in engineering transition, the fuzzy status, the technology simulation of random status modelling and digitalised prototype integration, the combination of figures and geometry in multi-component correlation in intricate equipment, the analysis of multi-level disposing, and multi-parameter matching. He received the National Pride for four times, which includes second prize in National Technology Progress twice, the first prize in National Excellent Education Achievement. He has also received the first prize in Provincial Level Technology Progress for six times. He transforms his technology into the software and got 12 copyrights of computer software, which achieved a lot in manufacturing enterprises.