Abstract
Multi-variety and small-batch production mode enables manufacturing industries to expeditiously satisfy customers' personalised demands, where a large amount of identical jobs can be split into several sublots, and be processed by reconfigurable machines with multiple machining technics. However, such highly flexible manufacturing environments bring some intractable problems to the production scheduling. Mathematical programming and meta-heuristic methods become less efficient when a scheduling problem contains both discrete and continuous optimisation attributes. Therefore, matheuristic, which combines advantages of the two methodologies, is regarded as a promising solution. This paper investigates a flexible job shop scheduling problem with lot-streaming and machine reconfigurations (FJSP-LSMR) for the total weighted tardiness minimisation. First, a monolithic mixed integer linear programming (MILP) model is established for the FJSP-LSMR. Afterwards, a matheuristic method with a variable neighbourhood search component (MH-VNS) is developed to address the problem. The MH-VNS adopts the classical genetic algorithm (GA) as the framework, and introduces two MILP-based lot-streaming optimisation strategies, LSO1 and LSO2, to improve lot-sizing plans with varying degrees. Four groups of instances are extended from the well-known Fdata benchmark to evaluate the performance of proposed MILP model, LSO1 and LSO2 components, and MH-VNS. Numerical experimental results suggest that LSO1 and LSO2 are efficient in different scenarios, and the proposed MH-VNS can well balance the solution quality and computational costs for reasonably integrating the GA- and MILP-based local search strategies. In addition, a complicated FJSP-LSMR case is abstracted from a real-world shop floor for processing large-sized structural parts to further validate the MH-VNS.
Disclosure statement
No potential conflict of interest was reported by the author(s).
Data availability statement
The data that support the findings of this study are openly available in ‘FJSP-LSMR’ at https://github.com/Ram313131/FJSP-LSMR.
Additional information
Funding
Notes on contributors
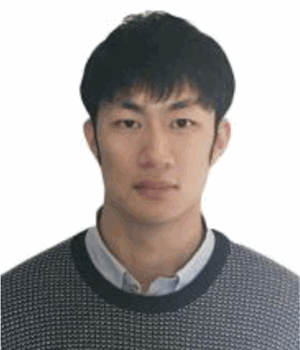
Jiaxin Fan
Jiaxin Fan received his B.Eng. degree from the school of automation, Huazhong University of Science and Technology, Wuhan, China, in 2017. He is currently pursuing the Ph.D. degree in industrial engineering at Huazhong University of Science and Technology. His current research interests include optimisation algorithms and production scheduling.
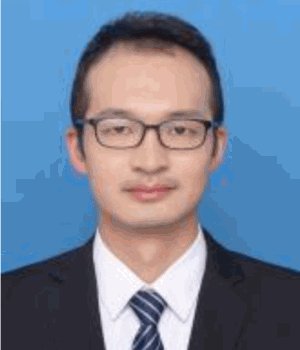
Chunjiang Zhang
Chunjiang Zhang received the B.Eng. and Ph.D. degrees in industrial engineering from Huazhong University of Science and Technology (HUST), Wuhan, China, in 2011 and 2016, respectively. From 2015 to 2016, he was a visiting student with the National University of Singapore, Singapore. From 2017 to 2018, he was a Research Fellow with the Nanyang Technological University, Singapore. From 2019 to 2021, he was a Postdoctoral Researcher with HUST. He is currently a lecturer with HUST. His current research interests include evolutionary algorithms, constrained optimisation, multiobjective optimisation, and their applications in production scheduling.
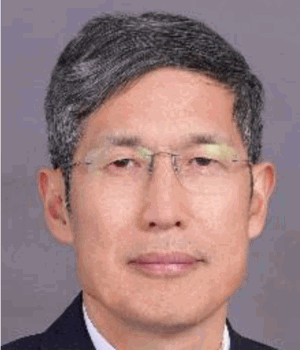
Weiming Shen
Weiming Shen received his Bachelor and Master's degrees from Northern (Beijing) Jiaotong University, China (in 1983 and 1986, respectively) and his Ph.D. degree from the University of Technology of Compiegne, France (in 1996). He is currently a Professor at Huazhong University of Science and Technology, China, and an Adjunct Professor at the University of Western Ontario, Canada. His research interest includes intelligent software agents, wireless sensor networks, IoT, Big Data, and their applications in industry. He is a Fellow of IEEE, a Fellow of Canadian Academy of Engineering, and a Fellow of the Engineering Institute of Canada.
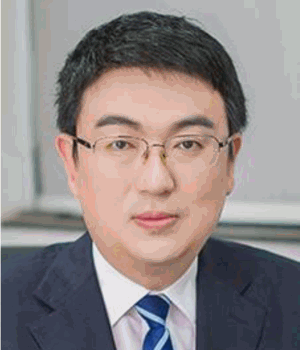
Liang Gao
Liang Gao is Professor of the Department of Industrial and Manufacturing Systems Engineering (IMSE), the Deputy Director of State Key Laboratory of Digital Manufacturing Equipment and Technology and Chairman of School of Mechanical Science and Engineering, Huazhong University of Science and Technology (HUST). He obtained his B.Sc. degree in mechatronic engineering from Xidian University, Xi'an, China, in 1996, and the Ph.D. degree in mechatronic engineering from HUST, Wuhan, China, in 2002. He was supported by the Program for New Century Excellent Talents in University in 2008 and National Science Fund for Distinguished Young Scholars of China in 2018. His research interests include intelligent optimisation algorithms, big data, deep learning with theirs application in Design & Manufacturing. He published more than 300 papers indexed by SCIE, authored 9 monographs. He currently serves as co-Editor-in-Chief of IET Collaborative Intelligent Manufacturing, Associate Editor of Swarm and Evolutionary Computation, Journal of Industrial and Production Engineering, etc.