Abstract
The dynamic characteristics (i.e. autocorrelation and cross-correlation) in modern industrial systems have raised great challenges to process fault detection. To cope with the dynamics and uncertainty of dynamic industrial processes, this paper proposes a new process control method, dynamic convolutional gated recurrent unit attention auto-encoder (DCGRUA-AE) for fault detection in dynamic processes. Firstly, DCGRUA-AE integrates a convolutional gated recurrent unit (CGRU) with a local convolution layer to learn both global and local features of dynamic process data in an unsupervised fashion. Secondly, a dual attention module is embedded in the deep network to preserve effective features. Finally, DeconvGRU combined with a dense layer is used as the encoder to reconstruct the original process data. Two statistics (i.e. T-square (T2) and squared prediction error (SPE)) based on DCGRUA-AE are used to set the control limits for fault detection. The feasibility and superiority of DCGRUA-AE-based fault detection method have been verified on four industrial processes. The experimental results indicate that the CGRU and dual attention mechanism can significantly improve the fault detection performance of DCGRUA-AE in dynamic processes. The hybrid of CGRU, attention mechanism and auto-encoder provides a new method for fault detection in dynamic industrial processes.
Acknowledgements
This research was supported by the National key research and development program (2022YFF0605700), the National Natural Science Foundation of China (92167107, 71771173), Fundamental Research Funds for the Central Universities (22120220575), and Shanghai Pujiang Program (2020PJD071).
Disclosure statement
No potential conflict of interest was reported by the author(s).
Data availability statement
The data that support the findings of this study are available from the corresponding author, upon reasonable request.
Additional information
Funding
Notes on contributors
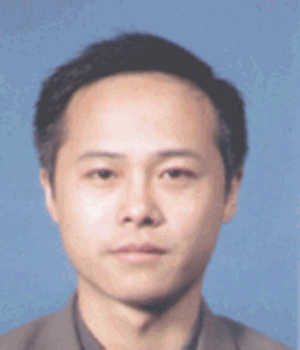
Jianbo Yu
Jianbo Yu received the B.Eng. degree from the Department of Industrial Engineering, Zhejiang University of Technology, Zhejiang, China, in 2002, the M.Eng. degree from the Department of Mechanical Automation Engineering, Shanghai University, Shanghai, China, in 2005, and the Ph.D. degree from the Department of Industrial Engineering, Shanghai Jiaotong University, Shanghai, China, 2009. From 2009-2013, he was an associate professor at Shanghai University, Shanghai, China. Since 2016, he worked as a professor at the School of Mechanical Engineering, Tongji University, Shanghai, China. His research interests focus on machine maintenance and machine learning. He is Associate Editor of IEEE Transactions on Instrumentation Measurement.
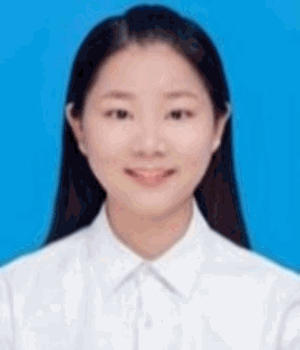
Shijin Li
Shijin Li received the B.Eng. degree from the school of Mining Engineering, China University of Mining and Technology, Jiangsu, China, in 2021. She is currently a Ph.D. degree candidate in the department of Industrial Engineering, Tongji University, Shanghai, China. Her current research interests include process fault diagnosis and machine learning.
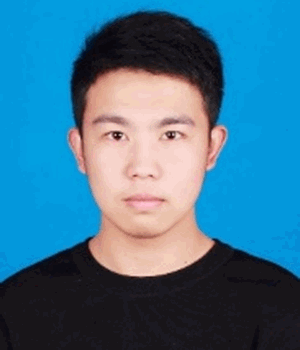
Xing Liu
Xing Liu, received his B.Eng. degree in 2018 from the Department of Industrial Engineering, Nanjing University of Finance & Economics. Now he is a master degree candidate in Tongji University. His main research interests include machine learning, fault detection and process monitoring.
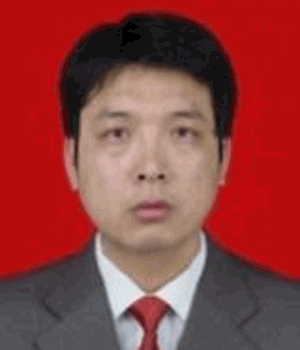
Yanfeng Gao
Yanfeng Gao currently is a Professor at, the School of Mechanical and Automotive Engineering, Shanghai University of Engineering Science. He obtained his Master’s and Ph.D. degrees from Xi’an Jiaotong University and Nanchang University in 2003 and 2009 respectively. The major areas of his teaching and research interests are in the fields of automated welding, numerical control technology, etc. He has published more than 80 papers in journals and international conferences.
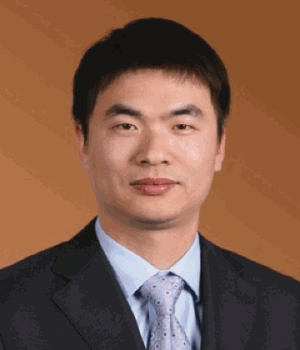
Shijin Wang
Shijin Wang, received the B.Eng. degree from the Department of Industrial Engineering, Zhejiang University of Technology, Zhejiang, China, in 2002; and the Ph.D. degree from the Department of Industrial Engineering and Management, Shanghai Jiaotong University, Shanghai, China, 2009. From 2009-Present, he worked as associate professor at the Department of Management Science & Engineering, School of Economics & Management, Tongji University, Shanghai, China. His current research interests include optimisation in combinatorial optimisation, production scheduling, artificial intelligence based algorithms, and supply chain optimisation.
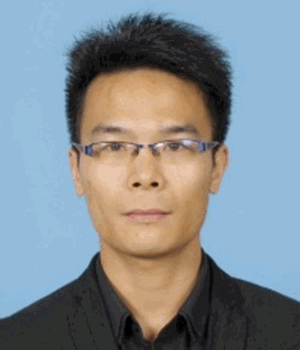
Changhui Liu
Changhui Liu received the B.Sc. degree in mechanical engineering from Hunan University, Changsha, China, in 2010, and the Ph.D. degree from Shanghai Jiao Tong University, Shanghai, China, in 2016. From 2016 to 2018, he was a post-doctor in the Shanghai Key Laboratory of Digital Manufacture for Thin-walled Structures, Institute of Automobile Engineering, School of Mechanical Engineering, Shanghai Jiao Tong University, Shanghai, China. From 2018 to 2019, he was a post-doctor with H.Milton Stewart School of Industrial and Systems Engineering, Georgia Institute of Technology, Atlanta, GA, U.S.A. He is currently an Assistant Professor at the School of Mechanical Engineering, Tongji University. His primary research interests are in data mining, machine learning, and quality control.