Abstract
Additive manufacturing (AM) introduces a set of technology-specific problems, such as the proper orientation of parts or the placement of several heterogeneous parts in the same build cycle, which are not addressed by traditional approaches to production planning and scheduling. Although these new production subproblems have been implicitly addressed by several works according to generic nesting and scheduling concepts, a literature review revealed that there is no uniformity in identifying and, thus, solving all these subproblems. For this reason, and as a result of an in-depth analysis of the existent literature on AM production planning and an analogy with classic cutting and packing typologies, the present paper offers a framework to formalise the production planning problem in AM at the operational level. This framework can be used as a reference to focus on and address these AM-related problems for efficient production planning. It is designed at the subproblem level and centres on production order processing in AM. A coding strategy is specifically developed for the framework, which is applied to a review of relevant works that propose models for the production planning of AM systems. Finally, the review results are discussed and possible extensions of the framework are proposed.
1. Introduction
Additive manufacturing (AM) is a production technique that allows physical objects to be made from geometric data by joining materials layer upon layer as opposed to subtractive and formative manufacturing (Astm Citation2015). Although AM was initially exploited to create prototype parts (Chergui, Hadj-Hamou, and Vignat Citation2018), it is currently applied for end parts production (Ngo et al. Citation2018; Hassani Citation2020). As it continues its journey towards maturity as a production technique, the AM focus lies in achieving the mass production of customised products (Oh et al. Citation2020a; Bogers, Hadar, and Bilberg Citation2016; Y. J. Kim, Byung, and Kim Citation2022). However, the high purchasing cost of industrial AM machines leads companies to outsource their production to specialised AM services (Kucukkoc Citation2019; Yılmaz Citation2020). This results in AM service providers having to plan the production of a very heterogeneous set of parts requested by distributed customers. At the same time, AM is currently expanding thanks to the evolution of cloud manufacturing platforms that integrate different AM machines from several distributed manufacturers (De Antón et al. Citation2020; Cui et al. Citation2022). The result is a very complex environment in which a set of distributed customers demand the production of varied parts to be manufactured by a set of distributed AM machines (González-Varona et al. Citation2020; Gopsill and Hicks Citation2018).
AM manufacturers face the challenge of determining the production plan for complex and heterogeneous environments. They usually lack automated systems that help to design efficient production plans (Ransikarbum, Pitakaso, and Kim Citation2020). Academics have recently paid more attention to complex production contexts, such as multifactory production planning (Lohmer and Lasch Citation2021) or production planning and process modelling in the context of Industry 4.0 (Luo, Thevenin, and Dolgui Citation2022; Vještica et al. Citation2022); also, decision support systems (DSS) are emerging in operational production planning (Christ, Dauzère-Pérès, and Lepelletier Citation2022; Dauzère-Pérès and Nonås Citation2022). However, the research conducted in this work is precisely motivated by the absence of DSS that specialise in the AM production planning problem at the operational level. The objective is to, thus, develop a framework that serves as the base tool to design DSS for AM. As AM has barely penetrated industry, mainly theoretical studies have been conducted to date to solve the planning and/or scheduling of specific manufacturing contexts. The framework proposed in this paper, which is based on the analysis of these studies, will provide a state-of-the-art perspective about how an efficient DSS for AM should be designed.
Current AM growth and its challenging production environments have drawn the research community's interest. In the last few years, contributions to topics about production planning and scheduling in AM have exponentially grown (Che et al. Citation2021; Fera et al. Citation2020). These works started to address the production planning of simple AM contexts, such as the production of a single part on a single AM machine (Yang et al. Citation2003; Thrimurthulu, Pandey, and Venkata Reddy Citation2004). As AM has evolved towards the manufacturing of larger production volumes, the simultaneous manufacturing of many parts in a single machine has been paid attention (Gogate and Pande Citation2008; Canellidis, Giannatsis, and Dedoussis Citation2013; Y. Zhang, Gupta, and Bernard Citation2016). More recent works have begun to analyse the current more complex context in which numerous parts need to be grouped and assigned to a set of AM machines to be manufactured (Dvorak, Micali, and Mathieug Citation2018; Kapadia et al. Citation2021; Che et al. Citation2021). Throughout this evolution from simple to complex production contexts, new subproblems have been progressively incorporated to consider all operational production planning aspects in AM systems.
Despite research into production planning in AM growing, a literature review has evidenced lack of uniformity in the models and formulations proposed to address this problem. It is not unusual to find formulations that address the same production planning problems but call it differently. Moreover, formulations for the same context can be designed according to different optimisation criteria and, hence, their underlying subproblems differ. As uniformity is lacking new proposals focus only on partial problems of complete operational production planning in AM. Another consequence of this unguided research is the absence of benchmarks to compare different solutions to the same production planning problems in AM. Contrarily to research into traditional shop configurations, the new works that address planning problems in AM often develop ad hoc datasets to test their proposals, and do not directly compare their performance to previous works.
This paper presents a framework to formalise the subproblems encompassed in the operational production planning of AM systems. This framework, which is presented in line with Stamer, Zimmermann, and Sandkuhl (Citation2016), aims to provide a formal structure that underlies AM operational production planning for the purpose of: (i) formally identifying the subproblems addressed by the literature in the design of additive manufacturing models; (ii) assessing which of these subproblems have been further studied in-depth; (iii) establishing a reference to formally place the design of new models that address production planning in AM at the operational level. To this end, uniform coding is proposed to enable the classification of AM production planning models according to the proposed framework.
The rest of this paper is organised as follows. The related literature is reviewed in Section 2. Section 3 details the framework, where an intuitive description of the AM production process is provided by defining planning stages and their corresponding subproblems. The codification strategy is presented in Section 4 and is applied to a review of the relevant models for AM production planning. Lastly, Section 5 ends with the reached conclusions and proposals to further extend this work.
2. Literature review
The two main production planning problems in AM at the operational level are nesting parts into builds and scheduling builds on machines (Dvorak, Micali, and Mathieug Citation2018; Kucukkoc, Li, and Li Citation2021). Nonetheless, the scope and objective of each problem are not consistently defined in the literature. Both nesting and scheduling problems can be divided into several subproblems that must be addressed together to achieve overall optimisation. A proper scheme for addressing operational production planning in AM should consider the nesting and scheduling subproblems to analyse their features and to show the relations among them. The main schemes for the nesting and scheduling problems in the Operations Management (OM) area are reviewed to study their underlying subproblems. The appearance of these two terms in the AM field is also discussed.
The use of the term ‘nesting’ in the AM planning and scheduling literature derives from its definition in the operations research field. Dyckhoff (Citation1990) defined the nesting problem as a cutting and packing (C&P) problem with the following characteristics: two-dimensional packing of irregularly shaped items. This definition is also supported by Wäscher, Haußner, and Schumann (Citation2007), who present an improved typology for C&P problems based on the work of Dyckhoff (Citation1990). In line with these two previous works, Bennell and Oliveira (Citation2008) extend the nesting definition by considering the possibility of irregular items to be rotated during the packing process. Thus according to classic C&P typologies, nesting describes the problem in which a set of two-dimensional irregular items has to be laid out on a rectangular large object. Ikonen et al. (Citation1997) were the first to notice that the previous definition of the nesting problem matched the characteristics of the problem of placing parts in an AM machine's workspace. However, it was not until several years later when Canellidis, Giannatsis, and Dedoussis (Citation2013) introduced the nesting parts concept to solve the platform layout optimisation problem for the simultaneous fabrication of many parts on an AM machine. This is the sense in which nesting has been used in AM production planning ever since.
The term ‘scheduling’ as regards production planning in AM began to spread halfway through the 2010 decade (Fera et al. Citation2020). The two main differences in relation to the classic machine scheduling problem were: several heterogeneous parts in AM were grouped in the same build; AM machines had various speed, size and cost characteristics (Kucukkoc Citation2019). Specifically, the problem defined as scheduling in AM involved allocating the objects requested by customers to one of the many available 3D printers to increase printers’ throughput. Although a few works have introduced some scheduling problem aspects into AM before (see (Freens et al. Citation2016; Kucukkoc, Li, and Zhang Citation2016)), it was with the work by Li, Kucukkoc, and Zhang (Citation2017) that the scheduling concept was formally established in AM to describe the process of allocating production orders to AM machines.
Nesting and scheduling are two problems that have been traditionally addressed separately in OM (Oh et al. Citation2020a). Each problem has its own optimisation objective; while nesting pursues maximum machine capacity utilisation to reduce costs, scheduling attempts to find the optimal processing sequence to reduce the makespan. The ultimate goal of both problems is to increase AM systems’ efficiency (J. Zhang, Yao, and Li Citation2020). The approaches found in the AM literature initially addressed nesting and scheduling as two separate problems. They focused on solving each problem independently by designing a two-step sequential process: the nesting of parts in builds was firstly solved; then those builds were scheduled on AM machines (Chergui, Hadj-Hamou, and Vignat Citation2018; Dvorak, Micali, and Mathieug Citation2018). Notwithstanding, recent works have started to develop integral nesting and scheduling formulations in their models, as in (J. Zhang, Yao, and Li Citation2020; Aloui and Hadj-Hamou Citation2021; Tevhide Altekin and Bukchin Citation2021; Kapadia et al. Citation2021; Makanda et al. Citation2022). Their formulations of the AM planning process already consider nesting and scheduling together for overall optimisation purposes. However, different authors design their models by considering the characteristics of their concrete AM contexts, which means that they do not all include the same underlying subproblems.
Despite the diversity of contexts and formulations regarding production planning in AM, only a few reviews and taxonomies have studied this problem in-depth. Y. Zhang, Gupta, and Bernard (Citation2016) reviewed works addressing the nesting problem in AM and proposed several criteria to classify them. Araújo et al. (Citation2019) proposed a taxonomy to classify nesting models in AM. Later three machine configurations were analysed to schedule AM machines in (Kucukkoc Citation2019). Recently, Cadiou, Demoly, and Gomes (Citation2022) presented a framework for multi-part scheduling using single-part jobs which, thus, excluded nesting. Although these works have developed schemes that focus on either the nesting or the scheduling problem, they fall short of covering the whole operational production planning problem in AM.
The only taxonomy and review that have thoroughly combined nesting and scheduling problems in AM were presented by Oh et al. (Citation2020a). This work proposed an interesting taxonomy for classifying models that focused mainly on nesting, mostly on scheduling, or presented a comprehensive approach to both nesting and scheduling. However, their classification was restricted to the higher level and did not delve into the specific subproblems encompassed in nesting and scheduling. At a higher level, there was the risk of using the same name for different problems. This occurs in the AM literature when describing the nesting problem; while some works define nesting as a bin-packing problem (e.g. (Li et al. Citation2019b; Aloui and Hadj-Hamou Citation2021; Kapadia et al. Citation2021)), others consider it to be a strip-packing problem (Alicastro et al. Citation2021; Y. Zhang, Gupta, and Bernard Citation2016), and some others regard it as a set-covering problem (Dvorak, Micali, and Mathieug Citation2018). Although the differences are minor, these are distinct problems that are all named in the same way (i.e. nesting). On scheduling in AM, some works only address the allocation of builds to several AM machines (e.g. (Li, Kucukkoc, and Zhang Citation2017; Ransikarbum, Pitakaso, and Kim Citation2020; Yılmaz Citation2020)), while others also develop strategies to find the best sequence for these builds (e.g. (Arık and Arık Citation2020; Dvorak, Micali, and Mathieug Citation2018; Ying et al. Citation2022)), and some others even find their proper starting times (Dvorak, Micali, and Mathieug Citation2018; Li, Zhang, and Kucukkoc Citation2019a; Y. J. Kim and Kim Citation2022). Regardless of these underlying differences, all the problems are described as AM machine scheduling. This conflict of using nesting and scheduling terms to describe problems with different nuances is overcome at the subproblem level, where the problem under study can be clearly identified.
As no framework that comprehensively analyses the production planning problem in AM from a subproblem perspective was found, traditional OM schemes were reviewed to study nesting and scheduling problems. Classic C&P problems investigate how to assign and place a set of small items in a set of large objects (Dyckhoff Citation1990). As a C&P problem, nesting can be decomposed into and analysed as traditional C&P schemes. One of the most important schemes is that of Wäscher, Haußner, and Schumann (Citation2007), who distinguished five subproblems that C&P problems must solve simultaneously to achieve a global optimum. These five subproblems are: (1) the selection problem as regards small items; (2) the selection problem for large objects; (3) the grouping problem regarding selected small items; (4) the allocation problem for assigning the subsets of small items to large objects; (5) the layout problem about the arrangement of small items in each selected large object. These five subproblems provide a comprehensive understanding of the kind of C&P problem that is faced. After adaptation to the AM context, this scheme can serve as a basis for defining the subproblems included in the AM nesting problem. However, scheduling is a very generic term that is recurrently used in the production planning literature. In the beginning, it was used to describe only the allocation of tasks to resources over time (i.e. only timing of tasks) insofar as the sequencing of tasks was regarded as a different problem. Later in the OM field, scheduling started to be conceived as a much broader problem: it encompassed the determination of the number of tasks to be manufactured, the assignment of tasks to resources, and both the sequencing and timing of tasks in each resource (Christos T. Maravelias Citation2012). These four subproblems allow the problem of scheduling several tasks to several resources to be comprehensively addressed and can be easily adapted for the AM context.
The framework proposed in this paper is based on the disaggregation of the two main problems in AM production planning at the operational level (i.e. nesting and scheduling) into subproblems. It is inspired by the schemes of Wäscher, Haußner, and Schumann (Citation2007) and Christos T. Maravelias (Citation2012), which are two reference contributions from the OM field that have been adapted for the AM environment. The framework includes the definition of all the subproblems faced in planning the production process of a set of part orders on a set of AM machines. Besides providing their definitions, four stages are defined through the planning process to organise subproblems. A coding strategy is developed to classify the models found in the AM production planning literature according to the framework. To the best of our knowledge, this is the first framework that aims to study AM operational production planning at a subproblem level.
3. Framework for production planning in AM
This section presents the framework. The adaptations of the works by Wäscher, Haußner, and Schumann (Citation2007) and Christos T. Maravelias (Citation2012) to the AM context are explained in Section 3.1. The four planning stages into which subproblems are arranged are specified and subproblems are defined in Section 3.2.
3.1. Framework design
As the previous taxonomies and reviews of AM models define the nesting problem in AM and the scheduling problem in AM with different nuances, both problems are herein disaggregated to eliminate any possible ambiguity. The framework proposed in this paper is based on subdividing nesting and scheduling problems into subproblems by focusing on the processing steps followed by part orders during the production process. So this scheme is restricted to the manufacturing stage and introduces a scheduling-oriented perspective that delves more deeply into the processing sequence of part orders.
Order processing in an AM environment starts by requesting part orders for several customers and ends by delivering the produced parts to the corresponding customers (see Figure ). As a trend to omit logistics issues in the design of models for production planning was found (Demir, Eyers, and Huang Citation2021), delivery tasks were excluded from the scheme herein developed. Accepting or rejecting the orders requested by customers is another issue that goes beyond the manufacturing scope. In this work, it was assumed that all the parts considered for planning had been previously accepted and can be manufactured by at least one AM machine; that is, customer orders may have been rejected before. Therefore, exclusive manufacturing tasks start with a previously accepted set of part orders and continue until parts are produced (see Figure ).
Figure 1. Part order processing in AM.
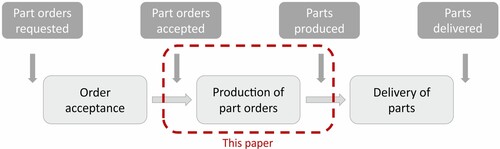
According to the hierarchical frameworks often used to classify production planning and control systems (see Oluyisola, Sgarbossa, and Strandhagen Citation2020; Luo, Thevenin, and Dolgui Citation2022), which consider different levels and planning horizons, the present work centres on the operational level (i.e. short-term horizon). The planning problem addressed, thus, starts once part orders have been confirmed either through customer demands or by the master production schedule, and focuses on day-by-day production scheduling (Chandra, Grabis, and Tumanyan Citation2007; Pinedo Citation2012).
The framework design is governed by two main premises: it should comprehensively suffice to show the complexity of the AM operational production planning problem; it should be modular and adaptable to different AM manufacturing environments. Accordingly, the framework is designed in a sequential fashion to better show the interrelations among subproblems. However, this is not a strictly sequential process and the subproblem order can be easily adapted to a specific production context.
To be consistent with previous taxonomies, and to adjust to the standard terminology for AM in ISO/ASTM 52900:2015(E) (Astm Citation2015), the terms Part, Build and AM Machine are used as defined in (Oh et al. Citation2020a; Araújo et al. Citation2019). Part stands for a physical instantiation of the 3D model of an object, which is to be formed by joining material in layers; Build represents a group of parts simultaneously produced by an AM machine in a single build cycle; AM Machine refers to a machine used to complete a build that includes parts in a build cycle. It should be stressed that a build is a representation of the AM machine that allows a batch of parts to be created for simultaneous manufacturing. The parameters of build (e.g. dimensions or speed) are then obtained from the corresponding AM machine.
To disaggregate the nesting and scheduling problems in AM into subproblems, this work is based on the schemes of Wäscher, Haußner, and Schumann (Citation2007) and Christos T. Maravelias (Citation2012), as shown in Section 2. These schemes are now adapted to incorporate AM-specific features into them.
The definitions for the generic subproblems of C&P problems of Wäscher, Haußner, and Schumann (Citation2007) are adapted to the AM context by substituting the terms ‘small items’ and ‘large objects’ for the terms ‘part orders’ and either ‘builds’ or ‘AM machines’ respectively. Therefore, according to this scheme, the five subproblems can be rewritten as:
(n1) selection problem regarding part orders
(n2) selection problem regarding AM machines
(n3) grouping problem regarding the selected part orders
(n4) allocation problem regarding the assignment of subsets of part orders to builds
(n5) layout problem regarding the arrangement of part orders on each selected build in relation to the geometric condition
According to Wäscher, Haußner, and Schumann (Citation2007), some types of C&P problems do not necessarily include all five subproblems. To adapt these definitions to the nesting problem in AM, the subproblem of selecting part orders (i.e. n1) must be excluded. As the framework is restricted to manufacturing aspects, accepting or rejecting the part orders requested by customers is an issue that goes beyond the production scope. At the same time, a note must be made about the terms used to adapt these definitions to AM. The term ‘AM machines’ is used to replace ‘large objects’ in the original selection problem (n2) of Wäscher, Haußner, and Schumann (Citation2007) to decide which machines to use. As AM allows many parts to be manufactured on the same build platform, the term ‘large objects’ is instead replaced with the term ‘builds’ in the allocation (n4) and layout (n5) problems to group the parts to be manufactured in the same build cycle of the selected machine.
The scheme proposed by Christos T. Maravelias (Citation2012) to disaggregate the scheduling of several tasks into several machines considers four subproblems. The definitions of these subproblems are adapted by replacing the words ‘task’ and ‘machine’ with ‘build’ and ‘AM machine’, respectively. The four subproblems can, therefore, be rewritten as:
(s1) determining the number of builds to be manufactured
(s2) allocating builds to AM machines
(s3) sequencing the builds in each AM machine
(s4) timing the builds in each AM machine
According to these schemes, nesting in AM can be divided into four subproblems (n2–n5), whereas scheduling in AM can be broken down into four subproblems (s1–s4). It must be noted that the subproblem of determining the number of builds (s1) from AM scheduling is already solved in the subproblem of assigning the subsets of part orders to the builds (n4) from AM nesting. Therefore, subproblem s1 is omitted, which leaves only the three remaining subproblems (s2–s4) considered within the framework. This evidences that nesting and scheduling cannot be separated in AM because they are interrelated through the planning process, as claimed in (Oh et al. Citation2020a; Kucukkoc, Li, and Li Citation2021) and demonstrated in (Kucukkoc Citation2021). It is shown that scheduling decisions are influenced by previous nesting choices. Decisions, such as machine allocation or build sequencing and timing according to scheduling optimisation criteria (e.g. minimum makespan or tardiness), are affected by the grouping of parts and their allocation to builds. Groups of parts with similar heights can favour both nesting tasks, such as part-to-build allocation and part placement, and scheduling objectives, such as minimum tardiness. Therefore the AM planning problem must be handled from a holistic viewpoint (Kucukkoc, Li, and Li Citation2021).
To fully introduce AM characteristics, the framework is further refined by adding the matching subproblem (M). This subproblem helps to coordinate the selection of part orders and the selection of AM machines. The need to address matching is justified by the wide variability of the production context in AM, where several heterogeneous parts must be manufactured by several heterogeneous machines. Thus the matching subproblem allows proper AM machines to be selected for manufacturing part orders.
The remaining eight subproblems, namely four for nesting in AM (n2–n5), three for scheduling in AM (s2–s4) and one the matching (M), comprise the framework for the AM production planning at the operational level presented in this work (see Figure ): Grouping; Matching; Machine selection; Build allocation; Placement; Machine allocation; Sequencing; Timing. These subproblems define the sequence followed by part orders from the time they are accepted for production to the time they are manufactured by AM machines. A sequential approach for the whole production process is given in Section 3.2, where subproblems are reordered to follow the production sequence.
Figure 2. Production order processing of parts in an AM environment.
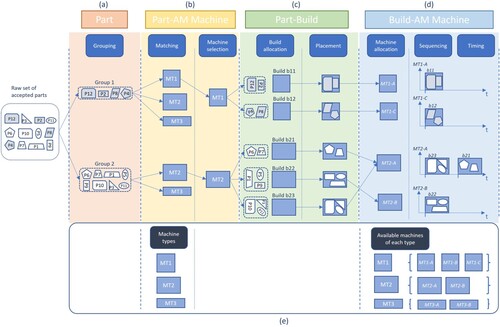
3.2. Subproblems in production order processing in AM
The framework describes the process followed by a set of part orders that must be manufactured by a set of AM machines. This process is known as production order processing. It starts once the part orders requested by customers have been accepted for their manufacturing and finishes when all the parts have been manufactured. The production order processing for AM is represented in Figure . The process is described for an AM context in which a set of machines with different characteristics (i.e. dimensions, speed, accuracy) has to manufacture a set of part orders with different requirements (i.e. dimensions, material, surface quality). The machines that share the same features are considered to belong to the same type.
Throughout production order processing, four planning stages are identified according to the planning question addressed in each one. These four stages are Part (Figure a), Part-AM Machine (Figure b), Part-Build (Figure c) and Build-AM Machine (Figure d). The questions for each stage are respectively:
how to regroup parts to improve their processing in AM
how to select the proper AM machine type for each part or group of parts
how to allocate and place many parts in multiple builds
how to assign and sequence builds in available AM machines.
These stages progressively appear according to the production planning sequence. The eight subproblems are arranged into these four planning stages. Each subproblem belongs to the stage whose question it addresses.
The subproblems that compose the framework are presented in sequence to follow the production order processing steps. Each process step corresponds to one subproblem. The sequence can be followed in Figure , starting from the raw set of parts accepted for their manufacturing. After its definition, each subproblem name is highlighted in the text by writing its name in brackets and italics.
Part. Firstly, the parts from the raw list may be grouped according to their own features (e.g. similar height, similar due date, same material, etc.) (Grouping). In AM, scheduling parts with similar characteristics in the same builds helps to obtain an efficient production plan (Oh et al., Citation2020a; J. Zhang, Yao, and Li Citation2020). As Grouping is the only subproblem that belongs to the Part planning stage, this stage is already completed (see Figure a).
Part-AM Machine. Next each group of parts is matched to all the AM machine types whose features make them capable of manufacturing that group (Matching). It must be noted that matching can also be done for each individual part order with minor changes, but here the matching for whole groups is considered. From the list of the feasible AM machine types for every group, one type is selected for each one (Machine type selection). The specific criteria for this selection are chosen by the production planner, who would typically consider production efficiency – and quality-related parameters. Planners can resort to supporting models for machine selection like that by Calabrese et al. (Citation2022), which proposes a model to select proper machines based on production quality features. The Part-AM Machine stage is now run (see Figure b).
Part-Build. At this point, the AM machine type on which each group of parts is to be manufactured is already selected. Consequently, the dimensions of the build volume of this machine type are known. Thus the parts in one group can now be allocated to different builds regarding the build volume dimensions (Build allocation). In each build, the placement (i.e. orientation and location) of parts is decided to obtain a platform layout (Placement). Once parts have been assigned to builds and their placements have been determined, the Part-Build stage is completed (see Figure c).
Build-AM Machine. Now the case of one AM machine type is taken. Once all the platform layouts corresponding to the builds of the considered type have been determined, each build must be allocated to one AM machine of this type. The availability of the machines from the considered type is checked and each build is assigned to one machine (Machine allocation). This is repeated for every AM machine type so that all the builds are assigned to only one machine. On the contrary, one machine can be assigned several builds. Lastly, the sequence of builds on each AM machine (Sequencing) and the start time of each build (Timing) have to be determined. Now the Build-AM Machine stage is complete (see Figure d) and the final production plan is obtained.
A simulation-based case study of the above-described process is provided in Figure to show how the framework would work in practice. The case study solves the production order processing of 12 parts in up to seven Selective Laser Melting (SLM) machines. Three SLM machine types are considered (i.e. MT1, MT2, MT3): MT1 and MT2 are adapted from the SLM machines used in (Kucukkoc Citation2019) and (Kamarudin et al. Citation2017) respectively, while MT3 is a commercial industrial machine from the SLM® company. The numbers of machines per type are: three for MT1, two for MT2 and two for MT3 (see the right side of Figure e). Part and machine specifications are detailed in Table and Table , respectively (see the Appendix). Next the procedure to solve the production order processing of the parts is detailed following the subproblem sequence of Figure . All the relevant tables to the case study are included in the Appendix.
Grouping: two groups are created according to a similarity of heights criterion (see Table ). In the raw set of parts (the left side of Figure ) and in Figure (a), parts are coloured differently depending on their height. Manufacturing parts with a similar height in SLM significantly enhances process efficiency.
Matching: each group is matched to the machine types whose dimensions are larger than the dimensions of all the parts in the group. Machine type 1 (MT1) cannot be matched to group 2 because the heights of the parts in that group are larger than the maximum height of this machine type (see Table ). However, group 1 can be matched to either MT1, MT2 or MT3 because their parts are shorter and are compatible to the specifications of any of these machine types (see Table ). The groups of created parts are displayed in Table and in Figure (b).
Machine type selection: an efficiency-related criterion is used to assign one machine type to each group of parts: the faster feasible machine type is selected (i.e. that with a higher build rate, see Table ). Thus MT1 is selected for group 1 and MT2 is selected for group 2 (see Table and Figure b).
Build allocation and Placement: once machine types have been assigned to groups of parts, the allocation of parts to builds and the placement of parts in a build can be determined. In this case study, these two problems are solved by computing an adaptation of the nesting algorithm proposed in De Antón et al. (Citation2020). The heuristic takes the dimensions of both parts and machines as input data and returns build layouts. The parts in group 1 are distributed in two builds (i.e. builds b11 and b12), whereas three builds (i.e. b21, b22 and b23) are created for the parts in group 2 (see Table and Figure c). The layouts of the seven builds are also obtained (see Figure ).
Machine allocation: machine availability is checked at the time of assigning builds to machines (i.e. t = 0). In this case study as machine MT1-B is assumed unavailable at the planning time, it is not considered (see Table ). Moreover, in this case study, the first criterion to select from among the available machines is workload balance, while the second criterion is the historical usage of machines. Therefore, builds are firstly assigned to empty machines to balance the amount of work among them. If there are no empty machines, the current build is assigned to the machine with the fewest accumulated working hours. In the present case, two machines of type 1 (i.e. MT1-A and MT1-C) and two machines of type 2 (i.e. MT2-A and MT2-B) are available. The allocation of builds to machines is shown in Figure (d) and Table .
Sequencing: the sequence in which builds are to be processed in each machine is obtained by attempting to minimise the tardiness of part orders. As in AM, the parts in a build cannot be removed until the build has been completed. The due date of a build is determined by the earliest due date of the parts in that build. Hence for each machine, builds are sorted in ascending order of due dates. As machines MT1-A, MT1-C and MT2-B only have one assigned build (i.e. b11, b12 and b22, respectively), sequencing is unnecessary. However, the sequence of builds b21 and b23 for machine MT2-A must be determined according to the above criterion (see Table and Figure d).
Timing: this case study considers that the builds in each machine are triggered as soon as possible to minimise tardiness (see Figure d).
Having provided this sequential description of production order processing in AM, the main subproblem characteristics are summarised in Table . For each subproblem, a brief explanation, an abstraction of its objective and its expected output are presented in columns 2, 3 and 4, respectively. The correspondence between the names and the original coding for subproblems (i.e. n2–n5; s2–s4) are found in the first column of this table.
Table 1. Main features of the subproblems in AM production planning.
Table 2. Review of AM production planning models in line with the framework.
This framework provides a comprehensive overview of the planning process for AM production. While designing an AM-specific DSS tool, a decision maker should select those subproblems adapted to its production context and decide where to place the optimisation focus. Global optimisation would probably entail the simultaneous formulation of several subproblems.
4. Review in line with the framework
A review of the relevant works that address the production planning of AM systems was conducted to show the usefulness of the framework. The models presented in these works were classified in line with the framework according to a proposed coding. Two more planning-related variables were used to analyse these models. Section 4.1 presents the research methodology followed to select the relevant papers for the review. The coding strategy and the additional criteria for model classification purposes are explained in Section 4.2. Lastly, the results obtained from the analysis of the models are discussed in Section 4.3.
4.1. Research methodology
This review intends to prove the usefulness of the proposed framework for analysing the underlying structure of models for AM production planning. The nomenclature proposed by Oh et al. (Citation2020a) is used to select the relevant models for the review. Their nomenclature classifies models according to whether they: focus on nesting (NfAM – Nesting for AM); centre on scheduling (SfAM – Scheduling for AM); present a comprehensive approach to both nesting and scheduling (NSfAM – Nesting and Scheduling for AM). As in the review by Oh et al. (Citation2020a), this research work is limited to those papers that present an applied model or the formulation of the operational production planning problem in AM. Theoretical and review papers are not considered.
The works selected for the review must belong to the NSfAM and SfAM types. Pure nesting problems (i.e. NfAM) are not included because they are restricted to packing issues and do not look at scheduling aspects in-depth. As a comprehensive review of the nesting and scheduling works in AM has been conducted in Oh et al. (Citation2020a), most of those papers categorised in their work as NSfAM and SfAM are included in the review. Only the SfAM models that do not address the allocation of parts to builds are left aside because they provide generic formulations that do not reflect the actual complex AM production problem. Thus 23 papers from the review by Oh et al. (Citation2020a) are included. To update the review with more recent works, a search that combines the expressions ‘additive manufactur*’, ‘3D print*’, ‘production planning’, ‘production scheduling’, ‘nesting’, ‘scheduling’ and ‘packing’ with AND, OR logical operators (see Figure ) was performed.
Figure 3. Keywords for the literature review.
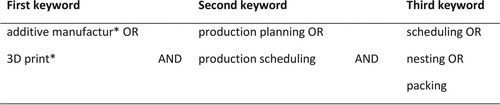
The search was conducted in September 2021 and repeated in September 2022 to cover the most recent contributions. The included scientific databases were Scopus (all fields) and Web of Science (all fields). Removing duplicates and the papers already included in the review by Oh et al. (Citation2020a), and excluding non-matching works (i.e. theoretical and NfAM models), gave 15 new papers. Thirty-eight papers were selected for the review. As the framework can be applied to any AM production planning environment, the works that presented different models for different machine configurations were independently analysed. One such case is Kucukkoc (Citation2019), who proposed three different formulations for the three different machine configurations herein considered (see Section 4.2). Consequently, 40 models were analysed.
4.2. Coding for the framework
A coding strategy was developed to classify AM production planning models or formulations in line with the framework. Coding is based on the eight subproblems that compose the framework and on the four planning stages in which they are organised. Before defining the rules for the coding strategy, one point is made about the order of subproblems. In Section 3.2, subproblems are presented following the expected sequence of production order processing. Nonetheless, as the formulations of different authors may present distinct approaches, not all the models are required to adjust to this sequence.
In an attempt to maintain the abstraction requirement low for readers, the initials of the subproblem names are used for coding. The initials to be used for all the subproblems are shown in brackets next to their name in column 1 of Table . The arrangement into four planning stages is considered, and is represented by separating the subproblems included in the same stage by a vertical bar ‘|’. The two rules that define the coding strategy are as follows:
If none of the subproblems in the same stage is addressed, a hyphen ‘-’ is used to indicate that the full stage is not considered
If some of the subproblems in the same stage are addressed, the initials of only the addressed subproblems appear
The classification of the works found in the research is provided in Table . By way of example, the coding of a model that includes all the subproblems in the framework is: ‘Gr | M,Se | Ba,Pl | Ma,Sq,Ti’. A model that does not address the Part-AM Machine stage and does not include the Timing subproblem in the Build-AM Machine stage looks like: ‘Gr | - | Ba,Pl | Ma,Sq’. This simple coding strategy helps to summarise a large amount of information with a low abstraction level and employs only a few symbols. This coding strategy is used in Table to illustrate the application of the proposed framework to the literature about production planning in AM.
Eight columns are used to indicate which subproblems appear in each model. These columns are named by employing subproblems’ initials. Besides, the column Scheme summarises the approach of models to the production planning problem in AM with the help of the proposed coding. Two complementary variables are used to help to understand models’ structure. The first is shown in the Machine configuration column. It allows the AM context to be analysed in relation to the AM machine set configuration. The second evaluates the strategy followed to solve the planning of AM machines and is indicated in the Planning approach column. These two additional variables are explained in the following paragraphs. The last column shows the AM process for which each model is developed. Three different AM processes are identified in the analysed works: vat photopolymerisation (VP), material extrusion (ME) and powder bed fusion (PBF). These are the most studied AM processes because they are more broadly adopted in industry.
Three different machine configurations can be considered in the AM scheduling problem according to Kucukkoc (Citation2019); single machine (s), parallel identical machines (Pm), and parallel non-identical machines (Rm). Parameter s describes the production planning problem for a single machine, while parameters Pm and Rm describe the production planning problem for several machines with the same features, and for several machines with different features, respectively. These three configurations are used to classify models according to their AM contexts; that is, models are s, Pm or Rm according to the amount and nature of the AM machines considered in each case. If one work develops different models for distinct machine configurations, each model is considered independently for its classification (e.g. (Kucukkoc Citation2019)).
While the parameter for machine configuration is useful to know the production context in which planning has to be determined, it does not provide information about the approach followed to do that planning. To overcome this issue, a parameter is included in column 3 of Table to indicate the specific planning approach presented for the model. One model can describe a context with many non-identical machines, but their planning is solved by scheduling the production of each machine one by one. This individual strategy only pursues the efficient utilisation of machines and, thus, overlooks scheduling-related measures, such as load balancing or makespan minimisation. On the contrary, a comprehensive production plan can be determined for all the machines at the same time. This holistic strategy is oriented more to time-related optimisation (i.e. makespan minimisation or on-time delivery). Abbreviation Ind in the table is used to indicate that an individual strategy is followed in the model, and abbreviation Hol denotes that a holistic strategy is applied.
4.3. Discussion
The classification presented in Table allows to analyse the scope, the approach and the structure of the relevant models for the production planning and scheduling of AM systems. This will help to identify similar models which, in turn, will facilitate making comparisons among proposals. The 40 reviewed models are studied according to the framework to analyse their subproblem structures and their particularities regarding each planning stage. The most repeated structures are highlighted. The influence of machine configuration and the planning approach on models’ design are discussed.
Regarding the Part planning stage, which focuses on how to regroup parts to improve their processing in AM, the Grouping subproblem is only introduced into seven models. Parts are grouped according to common parameters, such as product type (Zipfel, Neufeld, and Buscher Citation2021; Dvorak, Micali, and Mathieug Citation2018), quality requirements (Zipfel, Neufeld, and Buscher Citation2021), similar height (Wang et al. Citation2019), near location (Tafakkori, Tavakkoli-Moghaddam, and Siadat Citation2022), the same material (Y. J. Kim and Kim Citation2022; He et al. Citation2022) or setup time (H. J. Kim Citation2018).
In the Part-AM Machine planning stage, the addressed problem is how to select the proper AM machine type for each part or group of parts. Accordingly, the Matching subproblem is included in more than half the models (19), while the Machine type selection subproblem appears in 10 models. The latter subproblem is conditioned to include the former. Hence the Machine type selection subproblem always comes together with the Matching one. As expected, both subproblems only arise in the models with the Rm machine configuration. For configurations Pm and s, all the machines are assumed to be feasible for manufacturing any part.
As in the Part-Build planning stage, the analysis focuses on how to allocate and place many parts in many builds. Not surprisingly, the Batch allocation subproblem is included in all the models. This makes sense because this subproblem is the core of AM operational production planning for being common to both nesting and scheduling, which are the two main problems in AM. The specific location of parts in builds (i.e. the Placement subproblem) is addressed in 21 models. These models can also develop orientation strategies (Oh, Zhou, and Behdad Citation2018, Citation2020b; Tafakkori, Tavakkoli-Moghaddam, and Siadat Citation2022; Makanda et al. Citation2022) and allow the rotation of parts throughout the packing process (Che et al. Citation2021; Kapadia et al. Citation2019; Dvorak, Micali, and Mathieug Citation2018; J. Zhang, Yao, and Li Citation2020; Gopsill and Hicks Citation2018; Arık and Arık Citation2020).
Lastly in the Build-AM Machine planning stage, a decision must be made about how to assign and sequence builds on available AM machines. The assignment of builds to AM machines based on machine availability (i.e. the Machine allocation subproblem) is addressed in 19 of the models, which include the models that present either Rm or Pm machine configurations. As expected, none of these models’ planning approach is of the Ind type, but they are all of the Hol type instead. The Sequencing and Timing subproblems appear in most models as only seven models do not include either. Although the common scheduling rules are first-in-first-out (FIFO) for build sequencing and as-soon-as-possible (ASAP) for build timing, some works have developed their own strategies for these subproblems (Li, Zhang, and Kucukkoc Citation2019a; Li et al. Citation2019b; Dvorak, Micali, and Mathieug Citation2018; Kapadia et al. Citation2019; Alicastro et al. Citation2021; Arık and Arık Citation2020; Ying et al. Citation2022; Y. J. Kim and Kim Citation2022).
Regarding machine configuration and planning strategy, it is apparent that the most repeated model structure presents a context with several non-identical machines (i.e. Rm), which are planned through a holistic approach (i.e. Hol). This shows that most models attempt to reproduce complex heterogeneous AM environments and propose comprehensive production system optimisation. Besides, it must be noted that the only model that incorporates all eight subproblems is that of Dvorak, Micali, and Mathieug (Citation2018), and it is of the Rm and Hol types.
The fact that only four of the 40 analysed models introduce logistics aspects (Dvorak, Micali, and Mathieug Citation2018; Yılmaz Citation2020; Ransikarbum, Pitakaso, and Kim Citation2020; Tafakkori, Tavakkoli-Moghaddam, and Siadat Citation2022), and only four models from the review incorporate order acceptance into the production planning problem (Li, Zhang, and Kucukkoc Citation2019a; Li et al. Citation2019b; Kapadia et al. Citation2021; Tafakkori, Tavakkoli-Moghaddam, and Siadat Citation2022), supports the previous assumption that current planning models in AM mainly focus on production aspects.
5. Conclusions and further research
In this paper, a framework to formalise the structure of the production planning problem in AM at the subproblem level is developed for the first time. Lack of uniformity among the existent formulations for this problem motivated the design of this tool to support developing DSS for production planning in AM systems. The framework, inspired in the works by Wäscher, Haußner, and Schumann (Citation2007) and Christos T. Maravelias (Citation2012), is based on disaggregating the subproblems of the two main problems in AM: nesting and scheduling. These subproblems are arranged in four stages according to the planning aspects that they deal with. This framework provides a comprehensive description of the AM production planning problem to identify the most challenging subproblems and the relations among them. It offers a tool for AM planners to design efficient DSS and a coding for AM researchers to reference their planning models.
A review of relevant models for AM production planning allowed the usefulness of the framework to be proved. Forty models are classified employing a coding strategy developed specifically for the framework. Two additional criteria are introduced to analyse the relation between the structure of AM planning models: the production context to which they are applied and the planning strategy employed for its design. The second criterion is firstly proposed in this work. This review also confirms the validity of the framework to be applied to different formulations for the AM production planning problem, which may be designed for distinct AM environments. It also allows the planning schemes that are repeated the most to be seen.
The results from the review also support the assumption that most of the models for production planning in AM developed to date do not incorporate order acceptance and transportation issues. As more comprehensive models are developed for planning AM systems, order acceptance and delivery of parts to customers are two possible extensions to design a more general framework that is not limited to production aspects. Likewise, the need for post-processing operations for most AM processes is another possibility to further extend theframework.
Another research line could be to extend the review herein presented. This would entail using the framework to analyse the relation between models’ structure and other relevant criteria, such as their overall optimisation objective or the AM technology in use. This would allow the underlying structure of the most promising models for each AM technology to be identified and discussed.
Acknowledgements
We are grateful to the 15th International Conference on Industrial Engineering and Industrial Management and XXV Congress of Organization Engineering, where the authors presented a preliminary draft of the framework developed in this manuscript (De Antón et al. Citation2021). We thank the anonymous reviewers for their careful reading of our manuscript and their many insightful comments and suggestions. The usual disclaimer applies.
Disclosure statement
No potential conflict of interest was reported by the author(s).
Data availability statement
Data are available upon request from the authors.
Additional information
Funding
Notes on contributors
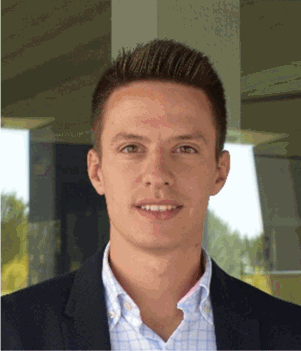
Juan De Antón
Juan De Antón is a Ph.D researcher in Industrial Engineering in the Department of Business Organisation and Market Research, University of Valladolid (UVa). He earned his B.Sc. and M.Sc. from the same UVa in Industrial Engineering in 2018 and in Project Management in 2019, respectively. His research interests in the INSISOC Group include additive manufacturing, operations management and combinatorial auctions.
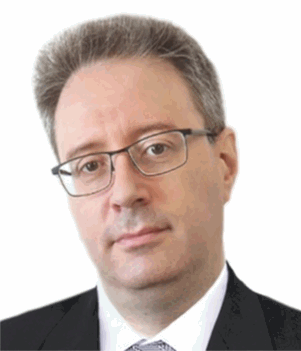
Félix Villafáñez
Félix Villafáñez is an Associate Professor in the Department of Business Organisation and Market Research, University of Valladolid (UVa). He holds a B.Sc. in Industrial Engineering since 2003 from the UVa and obtained his Ph.D. in 2014 on project scheduling optimisation, also at the UVa. His research interests in the INSISOC Group include agent-based modelling, combinatorial auctions, project management and project scheduling.
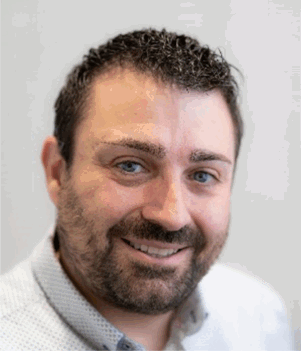
David Poza
David Poza is an Associate Professor in the Department of Business Organisation and Market Research, University of Valladolid (UVa). He received a B.Sc. in Telecommunications Engineering in 2007 from the UVa and obtained his Ph.D. in 2012 on applications of agent-based simulation to the emergence and diffusion of socio-economic norms also from the UVa. His research interests in the INSISOC Group include additive manufacturing, network theory and combinatorial auctions.
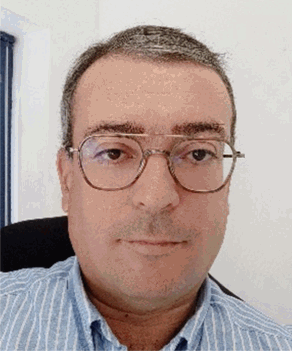
Adolfo López-Paredes
Adolfo López-Paredes is a Professor in the Department of Economics and Business Management at the University of Málaga (UMa). He holds a B.Sc. in Industrial Engineering from the University of Oviedo, and obtained his Ph.D. on applications of agent-based simulation to economic analysis in 2000 from the UVa. His research interests in the INSISOC Group include agent-based modelling, project management, managerial decision support systems and organisational resilience.
References
- Alicastro, Mirko, Daniele Ferone, Paola Festa, Serena Fugaro, and Tommaso Pastore. 2021. “A Reinforcement Learning Iterated Local Search for Makespan Minimization in Additive Manufacturing Machine Scheduling Problems.” Computers & Operations Research 131 (January 2020): 105272. doi:10.1016/j.cor.2021.105272.
- Aloui, Aymen, and Khaled Hadj-Hamou. 2021. “A Heuristic Approach for a Scheduling Problem in Additive Manufacturing Under Technological Constraints.” Computers and Industrial Engineering 154 (March 2020): 107115. doi:10.1016/j.cie.2021.107115.
- Antón, Juan De, David J Poza, Adolfo López-Paredes, and Félix Villafáñez. 2021. “A Framework for Production Planning in Additive Manufacturing.” In XXV Congreso de Ingeniería de Organización, 159. https://pressbooks.pub/cioxxv/chapter/a-framework-for-production-planning-in-additive-manufacturing-81/.
- Antón, J. De, J. Senovilla, J.M. González, and F. Acebes. 2020. “Production Planning in 3D Printing Factories.” International Journal of Production Management and Engineering 8 (2): 75. doi:10.4995/ijpme.2020.12944.
- Araújo, Luiz J.P., Ender Özcan, Jason A.D. Atkin, and Martin Baumers. 2019. “Analysis of Irregular Three-Dimensional Packing Problems in Additive Manufacturing: A New Taxonomy and Dataset.” International Journal of Production Research 57 (18): 5920–5934. doi:10.1080/00207543.2018.1534016.
- Arık, Oğuzhan Ahmet, and O. A. Arık. 2020. “Additive Manufacturing Scheduling Problem Considering Assembly Operations of Parts.” Operational Research, doi:10.1007/s12351-021-00649-y.
- Astm, Iso. 2015. “ASTM52900-15 Standard Terminology for Additive Manufacturing—General Principles—Terminology.” ASTM International, West Conshohocken, PA 3 (4): 5.
- Bennell, Julia A., and Jose F. Oliveira. 2008. “The Geometry of Nesting Problems: A Tutorial.” European Journal of Operational Research 184 (2): 397–415. doi:10.1016/j.ejor.2006.11.038.
- Bogers, Marcel, Ronen Hadar, and Arne Bilberg. 2016. “Additive Manufacturing for Consumer-Centric Business Models: Implications for Supply Chains in Consumer Goods Manufacturing.” Technological Forecasting and Social Change 102 (January): 225–239. doi:10.1016/J.TECHFORE.2015.07.024.
- Cadiou, Thibaut, Frédéric Demoly, and Samuel Gomes. 2022. “A Multi-Part Production Planning Framework for Additive Manufacturing of Unrelated Parallel Fused Filament Fabrication 3D Printers.” Designs 6 (1): 11. doi:10.3390/DESIGNS6010011.
- Calabrese, Maurizio, Teresa Primo, Antonio del Prete, and Giuseppe Filitti. 2022. “Nesting Algorithm for Optimization Part Placement in Additive Manufacturing.” International Journal of Advanced Manufacturing Technology 119 (7–8): 4613–4634. doi:10.1007/S00170-021-08130-Y/FIGURES/21.
- Canellidis, Vassilios, John Giannatsis, and Vassilis Dedoussis. 2013. “Efficient Parts Nesting Schemes for Improving Stereolithography Utilization.” CAD Computer Aided Design 45 (5): 875–886. doi:10.1016/j.cad.2012.12.002.
- Chandra, Charu, Janis Grabis, and Armen Tumanyan. 2007. “Problem Taxonomy: A Step Towards Effective Information Sharing in Supply Chain Management.” International Journal of Production Research 45 (11): 2507–2544. doi:10.1080/00207540601020486.
- Che, Yuxin, Kanxin Hu, Zhenzhen Zhang, and Andrew Lim. 2021. “Machine Scheduling with Orientation Selection and Two-Dimensional Packing for Additive Manufacturing.” Computers and Operations Research 130: 105245. doi:10.1016/j.cor.2021.105245.
- Chergui, Akram, Khaled Hadj-Hamou, and Frédéric Vignat. 2018. “Production Scheduling and Nesting in Additive Manufacturing.” Computers and Industrial Engineering 126 (May): 292–301. doi:10.1016/j.cie.2018.09.048.
- Christ, Quentin, Stéphane Dauzère-Pérès, and Guillaume Lepelletier. 2022. “A Three-Step Approach for Decision Support in Operational Production Planning of Complex Manufacturing Systems.” September, 1–26. doi:10.1080/00207543.2022.2118387.
- Cui, Jin, Lei Ren, Jingeng Mai, Pai Zheng, and Lin Zhang. 2022. “3D Printing in the Context of Cloud Manufacturing.” Robotics and Computer-Integrated Manufacturing 74 (October): 102256. doi:10.1016/j.rcim.2021.102256.
- Dauzère-Pérès, Stéphane, and Sigrid Lise Nonås. 2022. “An Improved Decision Support Model for Scheduling Production in an Engineer-to-Order Manufacturer.” 4OR May: 1–54. doi:10.1007/s10288-022-00508-2.
- Demir, Emrah, Daniel Eyers, and Yuan Huang. 2021. “Competing Through the Last Mile: Strategic 3D Printing in a City Logistics Context.” Computers and Operations Research 131: 105248. doi:10.1016/j.cor.2021.105248.
- Dvorak, Filip, Maxwell Micali, and Mathias Mathieug. 2018. “Planning and Scheduling in Additive Manufacturing.” Inteligencia Artificial 21 (62): 40–52. doi:10.4114/intartif.vol21iss62pp40-52.
- Dyckhoff, Harald. 1990. “A Typology of Cutting and Packing Problems.” Journal of Heuristics 18 (4): 677–697. doi:10.1007/s10732-012-9203-9.
- Fera, M., F. Fruggiero, A. Lambiase, R. Macchiaroli, and V. Todisco. 2018. “A Modified Genetic Algorithm for Time and Cost Optimization of an Additive Manufacturing Single-Machine Scheduling.” International Journal of Industrial Engineering Computations 9 (4): 423–438. doi:10.5267/j.ijiec.2018.1.001.
- Fera, M., Roberto Macchiaroli, Fabio Fruggiero, and Alfredo Lambiase. 2020. “A Modified Tabu Search Algorithm for the Single-Machine Scheduling Problem Using Additive Manufacturing Technology.” International Journal of Industrial Engineering Computations 11 (3): 401–414. doi:10.5267/j.ijiec.2020.1.001.
- Freens, Johan P. N., Ivo J. B. F. Adan, Alexander Yu Pogromsky, and Hugo Ploegmakers. 2016. “Automating the Production planning of a 3D Printing Factory.” Proceedings - Winter Simulation Conference 2016-Febru: 2136–47. doi:10.1109/WSC.2015.7408327.
- Gogate, A. S., and S. S. Pande. 2008. “Intelligent Layout Planning for Rapid Prototyping.” International Journal of Production Research 46 (20): 5607–5631. doi:10.1080/00207540701277002.
- González-Varona, José M., David Poza, Fernando Acebes, Félix Villafáñez, Javier Pajares, and Adolfo López-Paredes. 2020. “New Business Models for Sustainable Spare Parts Logistics: A Case Study.” Sustainability (Switzerland) 12 (8): 3071. doi:10.3390/SU12083071.
- Gopsill, James A, and Ben J Hicks. 2018. “Investigating the Effect of Scale and Scheduling Strategies on the Productivity of 3D Managed Print Services.” Proceedings of the Institution of Mechanical Engineers, Part B: Journal of Engineering Manufacture 232 (10): 1753–1766. doi:10.1177/0954405417708217.
- Hassani, Vahid. 2020. “An Investigation of Additive Manufacturing Technologies for Development of End-Use Components: A Case Study.” International Journal of Pressure Vessels and Piping 187 (November): 104171. doi:10.1016/J.IJPVP.2020.104171.
- He, Jianjia, Jian Wu, Ye Zhang, Yaopeng Wang, and Hua He. 2022. “Large-Scale Customized Production Scheduling of Multiagent-Based Medical 3D Printing.” Computational Intelligence and Neuroscience 2022, 1–13. doi:10.1155/2022/6557137.
- Ikonen, Ilkka, William E. Biles, Anup Kumar, John C. Wissel and Rammohan K. Ragade. 1997. “A Genetic Algorithm for Packing Three-Dimensional Non-Convex Objects Having Cavities and Holes.” In Proceedings of the 7th International Conference on Genetic Algorithms, edited by Thomas Black, 591–598, July 19–23. East Lansing, MI: Morgan Kaufmann.
- Kamarudin, K., M. S. Wahab, A. A. Raus, Aqeel Ahmed, and S. Shamsudin. 2017. “Benchmarking of Dimensional Accuracy and Surface Roughness for AlSi10Mg Part by Selective Laser Melting (SLM).” AIP Conference Proceedings 1831 (April). doi:10.1063/1.4981188.
- Kapadia, Maaz Saleem, Binil Starly, Alec Thomas, Reha Uzsoy, and Donald Warsing. 2019. “Impact of Scheduling Policies on the Performance of an Additive Manufacturing Production System.” Procedia Manufacturing 39: 447–456. doi:10.1016/j.promfg.2020.01.388.
- Kapadia, Maaz Saleem, Reha Uzsoy, Binil Starly, and Donald P Warsing. 2021. “A Genetic Algorithm for Order Acceptance and Scheduling in Additive Manufacturing.” International Journal of Production Research October: 1–18. doi:10.1080/00207543.2021.1991023.
- Kim, Hyun Jung. 2018. “Bounds for Parallel Machine Scheduling with Predefined Parts of Jobs and Setup Time.” Annals of Operations Research 261 (1–2): 401–412. doi:10.1007/S10479-017-2615-Z/FIGURES/5.
- Kim, Yong Jae, and Byung Soo Kim. 2022. “Part-Grouping and Build-Scheduling with Sequence-Dependent Setup Time to Minimize the Makespan for Non-Identical Parallel Additive Manufacturing Machines.” The International Journal of Advanced Manufacturing Technology 119 (3–4): 2247–2258. doi:10.1007/S00170-021-08361-Z.
- Kucukkoc, Ibrahim. 2019. “MILP Models to Minimise Makespan in Additive Manufacturing Machine Scheduling Problems.” Computers and Operations Research 105: 58–67. doi:10.1016/j.cor.2019.01.006.
- Kucukkoc, Ibrahim. 2021. “Metal Additive Manufacturing: Nesting vs. Scheduling.” Optimization and Data Science: Trends and Applications. AIRO Springer Series 6: 169–180. doi:10.1007/978-3-030-86286-2_13.
- Kucukkoc, Ibrahim, Qiang Li, Naihui He, and David Zhang. 2018. “Scheduling of Multiple Additive Manufacturing and 3D Printing Machines to Minimise Maximum Lateness.” In Twentieth International Working Seminar on Production Economics, edited by Robert W. Grubbström, and Hans H. Hinterhuber, 237–247. Innsbruck, Austria: Elsevier.
- Kucukkoc, Ibrahim, Zixiang Li, and Qiang Li. 2021. “2D Nesting and Scheduling in Metal Additive Manufacturing.” Communications in Computer and Information Science 1407 CCIS: 97–112. doi:10.1007/978-3-030-76307-7_8.
- Kucukkoc, Ibrahim, Qiang Li, and David Z. Zhang. 2016. “Increasing the Utilisation of Additive Manufacturing and 3D Printing Machines Considering Order Delivery Times.” 19th International Working Seminar on Production Economics, no. July 2017: 195–201.
- Li, Qiang, Ibrahim Kucukkoc, and David Z. Zhang. 2017. “Production Planning in Additive Manufacturing and 3D Printing.” Computers and Operations Research 83: 1339–1351. doi:10.1016/j.cor.2017.01.013.
- Li, Qiang, David Zhang, and Ibrahim Kucukkoc. 2019a. “Order Acceptance and Scheduling in Direct Digital Manufacturing with Additive Manufacturing.” IFAC-PapersOnLine 52 (13): 1016–1021. doi:10.1016/j.ifacol.2019.11.328.
- Li, Qiang, David Zhang, Shilong Wang, and Ibrahim Kucukkoc. 2019b. “A Dynamic Order Acceptance and Scheduling Approach for Additive Manufacturing On-Demand Production.” The International Journal of Advanced Manufacturing Technology 105 (9): 3711–3729. doi:10.1007/s00170-019-03796-x.
- Lohmer, Jacob, and Rainer Lasch. 2021. “Production Planning and Scheduling in Multi-Factory Production Networks: A Systematic Literature Review.” International Journal of Production Research. Taylor and Francis Ltd. doi:10.1080/00207543.2020.1797207.
- Luo, Dan, Simon Thevenin, and Alexandre Dolgui. 2022. “A State-of-the-Art on Production Planning in Industry 4.0″.” International Journal of Production Research, 1–31. doi:10.1080/00207543.2022.2122622.
- Luzon, Yossi, and Eugene Khmelnitsky. 2019. “Job Sizing and Sequencing in Additive Manufacturing to Control Process Deterioration.” IISE TRANSACTIONS 51 (2): 181–191. doi:10.1080/24725854.2018.1460518.
- Makanda, Inno Lorren Désir, Maolin Yang, Haoliang Shi, Wei Guo, and Pingyu Jiang. 2022. “A Multi-Part Production Planning System for a Distributed Network of 3D Printers Under the Context of Social Manufacturing.” Machines 10 (8): 605. doi:10.3390/machines10080605.
- Maravelias, Christos T. 2012. “General Framework and Modeling Approach Classification for Chemical Production Scheduling.” AIChE Journal 59 (4): 215–228. doi:10.1002/aic.
- Ngo, Tuan D., Alireza Kashani, Gabriele Imbalzano, Kate T.Q. Nguyen, and David Hui. 2018. “Additive Manufacturing (3D Printing): A Review of Materials, Methods, Applications and Challenges.” Composites Part B: Engineering 143 (June): 172–196. doi:10.1016/J.COMPOSITESB.2018.02.012.
- Oh, Yosep, Paul Witherell, Yan Lu, and Timothy Sprock. 2020a. “Nesting and Scheduling Problems for Additive Manufacturing: A Taxonomy and Review.” Additive Manufacturing 36 (August): 101492. doi:10.1016/j.addma.2020.101492.
- Oh, Yosep, Chi Zhou, and Sara Behdad. 2018. “Production Planning for Mass Customization in Additive Manufacturing: Build Orientation Determination, 2D Packing and Scheduling.” Proceedings of the ASME Design Engineering Technical Conference 2A-2018 (August). doi:10.1115/DETC2018-85639.
- Oh, Yosep, Chi Zhou, and Sara Behdad. 2020b. “The Impact of Build Orientation Policies on the Completion Time in Two-Dimensional Irregular Packing for Additive Manufacturing.” International Journal of Production Research 58 (21): 6601–6615. doi:10.1080/00207543.2019.1683253.
- Oluyisola, Olumide Emmanuel, Fabio Sgarbossa, and Jan Ola Strandhagen. 2020. “Smart Production Planning and Control: Concept, Use-Cases and Sustainability Implications.” Sustainability 2020 12 (9): 3791. doi:10.3390/SU12093791.
- Pinedo, Michael. 2012. Scheduling - Theory, Algorithms, and Systems. 5th ed. Springer. https://link.springer.com/content/pdf/10.1007978-3-319-26580-3.pdf.
- Ransikarbum, Kasin, Sangho Ha, Jungmok Ma, and Namhun Kim. 2017. “Multi-Objective Optimization Analysis for Part-to-Printer Assignment in a Network of 3D Fused Deposition Modeling.” Journal of Manufacturing Systems 43: 35–46. doi:10.1016/j.jmsy.2017.02.012.
- Ransikarbum, Kasin, Rapeepan Pitakaso, and Namhun Kim. 2020. “A Decision-Support Model for Additive Manufacturing Scheduling Using an Integrative Analytic Hierarchy Process and Multi-Objective Optimization.” Applied Sciences (Switzerland) 10 (15), doi:10.3390/app10155159.
- Rohaninejad, Mohammad, Zdeněk Hanzálek, and Reza Tavakkoli-Moghaddam. 2021. “Scheduling of Parallel 3D-Printing Machines with Incompatible Job Families: A Matheuristic Algorithm.” IFIP Advances in Information and Communication Technology 630 IFIP: 51–61. doi:10.1007/978-3-030-85874-2_6.
- Stamer, Dirk, Ole Zimmermann, and Kurt Sandkuhl. 2016. “What Is a Framework?-A Systematic Literature Review in the Field of Information Systems.” LNBIP 261: 145–158. doi:10.1007/978-3-319-45321-7_11.
- Tafakkori, Keivan, Reza Tavakkoli-Moghaddam, and Ali Siadat. 2022. “Sustainable Negotiation-Based Nesting and Scheduling in Additive Manufacturing Systems: A Case Study and Multi-Objective Meta-Heuristic Algorithms.” Engineering Applications of Artificial Intelligence 112 (June): 104836. doi:10.1016/J.ENGAPPAI.2022.104836.
- Tavakkoli-Moghaddam, Reza, Shadi Shirazian, and Behdin Vahedi-Nouri. 2020. “A Bi-Objective Scheduling Model for Additive Manufacturing with Multiple Materials and Sequence-Dependent Setup Time.” In IFIP Advances in Information and Communication Technology. Vol. 592 IFIP. Springer International Publishing. doi:10.1007/978-3-030-57997-5_52.
- Tevhide Altekin, F., and Yossi Bukchin. 2021. “A Multi-Objective Optimization Approach for Exploring the Cost and Makespan Trade-off in Additive Manufacturing.” European Journal of Operational Research, doi:10.1016/j.ejor.2021.10.020.
- Thrimurthulu, K., Pulak M. Pandey, and N. Venkata Reddy. 2004. “Optimum Part Deposition Orientation in Fused Deposition Modeling.” International Journal of Machine Tools and Manufacture 44 (6): 585–594. doi:10.1016/J.IJMACHTOOLS.2003.12.004.
- Vještica, Marko, Vladimir Dimitrieski, Milan Mirko Pisarić, Slavica Kordić, Sonja Ristić, and Ivan Luković. 2022. “Production Processes Modelling Within Digital Product Manufacturing in the Context of Industry 4.0.” International Journal of Production Research, 1–20. doi:10.1080/00207543.2022.2125593.
- Wang, Yuanbin, Pai Zheng, Xun Xu, Huayong Yang, and Jun Zou. 2019. “Production Planning for Cloud-Based Additive Manufacturing—A Computer Vision-Based Approach.” Robotics and Computer-Integrated Manufacturing 58 (March): 145–157. doi:10.1016/j.rcim.2019.03.003.
- Wäscher, Gerhard, Heike Haußner, and Holger Schumann. 2007. “An Improved Typology of Cutting and Packing Problems.” European Journal of Operational Research 183 (3): 1109–1130. doi:10.1016/j.ejor.2005.12.047.
- Yang, Yong, Jerry Y.H. Fuh, Han Tong Loh, and Yoke San Wong. 2003. “Multi-Orientational Deposition to Minimize Support in the Layered Manufacturing Process.” Journal of Manufacturing Systems 22 (2): 116–129. doi:10.1016/S0278-6125(03)90009-4.
- Ying, Kuo-Ching, Fabio Fruggiero, Pourya Pourhejazy, and Bo-Yun Lee. 2022. “Adjusted Iterated Greedy for the Optimization of Additive Manufacturing Scheduling Problems.” Expert Systems with Applications 198 (July): 116908. doi:10.1016/J.ESWA.2022.116908.
- Yılmaz, Ömer Faruk. 2020. “Examining Additive Manufacturing in Supply Chain Context Through an Optimization Model.” Computers and Industrial Engineering 142 (January): 106335. doi:10.1016/j.cie.2020.106335.
- Zhang, Yicha, Ravi Kumar Gupta, and Alain Bernard. 2016. “Two-Dimensional Placement Optimization for Multi-Parts Production in Additive Manufacturing.” Robotics and Computer-Integrated Manufacturing 38: 102–117. doi:10.1016/j.rcim.2015.11.003.
- Zhang, Jianming, Xifan Yao, and Yun Li. 2020. “Improved Evolutionary Algorithm for Parallel Batch Processing Machine Scheduling in Additive Manufacturing.” International Journal of Production Research 58 (8): 2263–2282. doi:10.1080/00207543.2019.1617447.
- Zhao, Zhen, Lin Zhang, and Jin Cui. 2018. “A 3D Printing Task Packing Algorithm Based on Rectangle Packing in Cloud Manufacturing.” Lecture Notes in Electrical Engineering 460: 21–31. doi:10.1007/978-981-10-6499-9_3.
- Zipfel, Benedikt, Janis S. Neufeld, and Udo Buscher. 2021. “Customer Order Scheduling in an Additive Manufacturing Environment.” IFIP Advances in Information and Communication Technology 633 IFIP (September): 101–109. doi:10.1007/978-3-030-85910-7_11.
Appendix
The tables with data on the parts and machines used in the case study are included here. The part dataset has been taken from (Kucukkoc, Li, and Li Citation2021). The tables with the outputs and results from the simulated case study are displayed here.
Figure A1. Build layouts returned from the nesting heuristic.
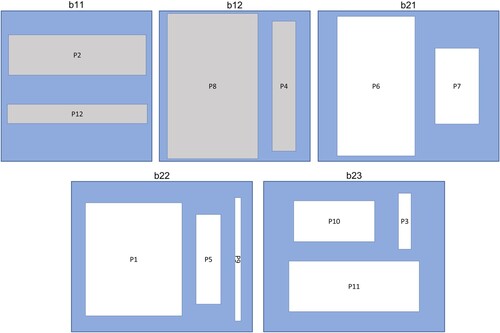
Table A1. Part dataset used for the case study.
Table A2. Machine type specifications.
Table A3. Groups of parts created.
Table A4. Allocation of parts to builds.
Table A5. Assignment of builds to machines.
Table A6. Sequence of builds in machines.