Abstract
Digital transformation is a major organisational challenge for manufacturing firms due to the extremely low success rate of such transformations to date. Capability Maturity theory suggests that firms need to develop digital transformation capability incrementally by focusing on a ‘vital few’ improvement priorities for advancing progress. The practitioner literature lacks empirical studies that validate extant capability maturity models (CMM) for digital transformation despite their importance. Moreover, there is a lack of assessment methods, and those that exist do not specify improvement points explicitly, nor prioritise them. Our research aims to address this gap through a systematic, quantitative analysis of digital capability by understanding the deployment of IT-enabled resources. Based on a sample of 302 manufacturing firms, results indicate that the digital transformation stages are punctuated by various resource-capability combinations. Results highlight that strategy- and organisation-related IT-enabled resources are the key drivers of digital transformation. We also observe that as a firm’s digital capability grows at each maturity stage, successively greater IT-enabled resources are required to support this in a stepwise function. To succeed, firms should be incentivised and supported to think beyond technology and develop five specific digital capabilities simultaneously. We also indicate the limitations that underlie our empirical work.
Introduction
Digital transformation is the modern-day battle to survive the existential threat of the Fourth Industrial Revolution (Saldanha Citation2019). Scholars have acknowledged that a growing number of businesses are undertaking digital transformation by leveraging contemporary information technologies (e.g. Berman Citation2012; Gerbert, Gauger, and Steinhäuser Citation2015; Müller, Buliga, and Voigt Citation2018; Rožanec et al. Citation2022), because it allows the integration and extension of manufacturing processes at both intra- and inter-organisational levels. With the help of these technologies, the manufacturing process becomes able to process diverse incoming orders with individual machine structures (Dolgui and Ivanov Citation2021) and is capable of changing the operation processing or setup sequences according to optimal capacity utilisation (Ivanov, Dolgui, and Sokolov Citation2019).
Nevertheless, buying state-of-the-art technology is not enough to get ahead of the competition, rather, firms create a competitive advantage by assembling complex systems of information technology-enabled resources (IT) that work together to create organisational capabilities (Bharadwaj Citation2000). Such IT-enabled resources are formed by blending IT assets with organisational resources. Most importantly, these IT-enabled resources are not bought directly from a vendor; instead, they are built and cultivated over time (Nevo and Wade Citation2010). In the process of becoming digital, firms transform their manufacturing into collaborative systems involving physical, software, and human agents resulting in the fusion of technical and business processes (Kusiak Citation2017).
Bibby and Dehe (Citation2018) emphasised that firms that are actively seeking to transform must begin with understanding the current maturity phase of the firm, determine their areas of weakness and strength, and prioritise improvements for progression. Most manufacturing firms, however, do not have the understanding or capability to assess their progress in digital transformation (Erol, Schumacher, and Sihn Citation2016; Schwab Citation2018; Lucato et al. Citation2019), or they are lacking the know-how of transforming their existing business into digital (Sony and Naik Citation2019; Saldanha Citation2019). They often experience difficulties in coordinating and managing the scope of business-to-technology transformation (Gökalp and Martinez Citation2021). On average, less than 20% of big companies are succeeding in their digital transformation efforts, let alone SMEs (Issa et al. Citation2018).
It is not surprising that digital transformation and Industry 4.0 have attracted growing scholarly attention (Fischer et al. Citation2020; Müller et al. Citation2018); however, most of the studies are either conceptual or technology-oriented (Liao et al. Citation2017; Xu, Xu, and Li Citation2018; Machado, Winroth, and Ribeiro da Silva Citation2020). The role of socio-cultural elements is usually underestimated (Tortorella et al. Citation2020), especially regarding providing empirical evidence about the effective implementation of digital transformation in manufacturing firms (Frank, Dalenogare, and Ayala Citation2019). Although some light has been shed on specific aspects, the full picture of what implementation encompasses remains fragmented (Nayernia, Bahemia, and Papagiannidis Citation2021). In addition, quantitative analysis is absent (Liao et al. Citation2017). Future research, therefore, needs to validate how organisations build capabilities to succeed in digital transformation (Warner and Wäger Citation2019).
Moreover, Issa et al. (Citation2018) argued that organisations need support in their endeavours to embrace digital transformation, especially concerning where to focus their efforts and resources, as these are assumed to be critical for succeeding in the transformation. Recent studies suggest that maturity process models could help organisations to learn about the consistent practices needed to succeed in their digital transformation journey (Ganzarain and Errasti Citation2016; Mittal et al. Citation2018; Moeuf et al. Citation2020; Gökalp and Martinez Citation2021). Comparing the best practices and the current state of a firm, it becomes possible to make relevant and informed decisions to set targets and allocate resources properly to pursue the digital transformation on a further stage (Lucato et al. Citation2019).
Taking this into consideration, we aim to: (i) develop a measurement scale that underpins a digital capability maturity model (DCMM) that can depict stages in the digital transformation process of B2B firms; and, (ii) identify the resource configurations that describe each digital capability maturity stage. Addressing these critical gaps in the literature, enables us to provide a valuable roadmap to understand the resource drivers in the digital capability maturity process. To achieve these aims, we surveyed 302 managers in manufacturing firms, using a digital capability index in five dimensions, and, as a result, four different maturity stages emerged from our dataset. Second, the intention was to extend the technology view of digital transformation with the study of IT-enabled resources that B2B organisations need for successfully becoming digital as suggested by Gökalp et al. (Citation2017) and Machado et al. (Citation2020). To meet this objective, we developed and tested the development of IT-enabled resources with a scale of 30 items in 6 dimensions. The third goal was to guide practitioners to properly invest in IT-enabled resources (Chirumalla et al. Citation2018; Guidici and Reinmoeller Citation2012). To assist with that, our research draws attention to the need for a paradigm shift that requires an investment in technology and non-technology related resources and capabilities.
Unlike prior work, we addressed this gap with robust, quantitative research. While the qualitative, often case study based maturity models can be revealing, they do not provide generalisable insights. Their validation calls for statistical analysis of primary data. Consequently, the present research is not a snapshot nor an assessment of where B2B firms are in terms of digital transformation. On the contrary, it extends our knowledge by presenting the operational validation of digital capability maturity and a resource scale for IT-enabled resources, improving our understanding of how firms succeed in their transformations, what are the critical learning points in terms of resource investments, and whether digital capabilities develop on similar scales. The results guide practitioners and researchers as well. Since implementation is the focus of the research, the theoretical foundation of the paper is built on the capability maturity concept. We developed and empirically validated a quantified assessment tool for digital capability maturity and IT-enabled Resource Scale (ITRS).
In summary, there is a need to study digital maturity through systematic, quantitative research and contribute to the understanding of what managers can do to enhance the probability of successful digital transformation and avoid the pitfalls of the change. Even though the results may raise new questions, the measurement for capability maturity during digital transformation is a critical contribution that sets an important baseline for predictive models that can build on these insights.
The first section of the article reviews the literature on digital transformation, IT-enabled resources and digital competencies, as well as digital maturity models, in particular, to identify the research gap in the empirical validation of how the composition of resources changes when firms acquire new digital capabilities. This is followed by a description of the methodology and research setting. Finally, results are presented and discussed, including the underlying theoretical, managerial, and policy implications as well as the limitations of the research.
Literature review
Digital transformation
Digital transformation is often understood as the process of implementing digital technologies. For example, Chen et al. (Citation2020) argue that it is made possible by Cyber-Physical Space-based production systems which include IoT, cloud computing, and big data. Bibby and Dehe (Citation2018) argued that there are other key technological components that are consistently linked to operationalise Industry 4.0, in particular additive manufacturing, cloud, manufacturing execution system, e-value chains, as well as autonomous robots. With the advancement of technological innovations, the list could be further enriched with virtual, augmented reality or machine learning. Researchers also pointed out that digital transformation is about becoming digital (Saldanha Citation2019). The elements are not stand-alone but interconnected leading to the emergency of a complex system (Chae and Olson Citation2021), including the transformation of business processes (Moeuf et al. Citation2018; Xu et al. Citation2018) which increases the complexity of the entire manufacturing system (Jiang et al. Citation2021). Manufacturers tend to initiate transformation either to gain a first-mover advantage or maintain their industry position, but sometimes as a response to external pressure, for example, to gain legitimacy (Zhou et al. Citation2022).
Various experts argue that one of the most common impediments to moving forward on the road to digital transformation is that most organisations are treating digital transformation as a typical IT project (Issa et al. Citation2018; Rogers Citation2016). In the past, information systems were used mainly to provide back-office support (El Sawy et al. Citation2010). Today, information technology is embedded throughout the organisation (Yeow et al. Citation2018) and hence, all the organisational functions undergo a transformative change (Rajnai and Kocsis Citation2018; Sony and Naik Citation2019). Digital transformation is therefore inherently multifunctional (Bharadwaj et al. Citation2013) and requires the complex consideration of organisational, managerial, and operational circumstances (Moeuf et al. Citation2018; Mittal et al. Citation2019; Nayernia et al. Citation2021) Brettel et al. (Citation2014) concluded that successful implementation requires the reorganisation of human actions, organisational processes and routines. For example, the renewal of the production capability (also called ‘manufacturing capability’) requires a new combination of tools, materials, methods and people engaged in producing a new or significantly improved production method (Romero et al. Citation2017).
The process is potentially chaotic as it requires changes in people, machines, and business processes simultaneously (Davenport and Westerman Citation2018). A paradigm shift is required, which is challenging. First, many organisations cannot assess their digital maturity stage or operationalise the transformation process (Colli et al. Citation2018). Second, members of the top management team often commit the mistake of identifying dozens of competing needs and then failing to act on any of them because they are overwhelmed by the size and complexity of the need (Paulk Citation2009). They may not have the understanding of where to focus, what should be the priority, and consequently, where to invest the resources to progress (Bibby and Dehe Citation2018). Besides, organisations may lack the capacity to mobilise the required organisational resources (Kouropalatis et al. Citation2018). Third, firms often tend to be locked in the trajectory of their legacy systems, work silos, and organisational politics (Weill and Woerner Citation2015). Finally, cultural barriers and confidentiality concerns can be also critical barriers (Gebhardt et al. Citation2021).
It is not surprising then that the implementation of large-scale transformations has low rates of success across organisations (Weidong and Gwanhoo Citation2014; Loonam et al. Citation2018). The underlying reasons vary, as we have highlighted above, but a significant amount of evidence suggests that organisations need support in the implementation of digital transformation. Scholars have urged organisations to develop their ‘roadmap’ of digital transformation (Issa et al. Citation2018), which is based on a systematic audit and benchmark of the company (Amaral et al. Citation2019).
IT-enabled resources and digital capabilities
Organisational resources can be viewed as bundles of tangible and intangible assets, including a firm’s management skills, organisational processes and routines, and the knowledge it controls (Barney et al. Citation2001). Firms create a competitive advantage by assembling resources that work together to create organisational capabilities (Bharadwaj Citation2000). Capabilities are information-based, firm-specific processes for combining, deploying, and converting resources into final products and services (Amit et al. Citation1993). Thus, capabilities are the ‘glue’ that brings organisational resources together and enables their use to gain a competitive advantage (Grant Citation1991).
Resources and capabilities are often synergistic in nature and can be more valuable when combined (Paulraj Citation2011). For example, when firms blend IT assets – which are commodity-like technology-based products, such as a piece of commercially-available software – with organisational resources, then they create IT-enabled resources (Nevo and Wade Citation2010). Digital capabilities are the firm’s ability to deploy and convert IT-enabled resources. Digital capability consequently elevates the performance implications of information and communication technology (ICT) assets beyond efficiency and productivity metrics to those that drive competitive advantage (Piccarozzi et al. Citation2018).
An IT-enabled resource is not merely the sum of its components, rather it is a system, where the interactions between the components of this system give rise to new higher-order capabilities – that is, capabilities that neither component possessed by itself (Bharadwaj Citation2000). For example, a firm’s digital capabilities may emerge from the cross-functional integration of its marketing, IT, and operational capabilities. From a hierarchical perspective, lower-order capabilities are combined, and then higher-order capabilities emerge, which requires extensive learning (Grewal and Slotegraaf Citation2007). Both, the resources and the capabilities allow the firm to earn rent continuously (Winter Citation2003). Boothby et al. (Citation2010) found evidence that manufacturing enterprises that invest in IT-enabled resources and develop digital capabilities are more prosperous than those that do not. Consequently, Kraaijenbrink, Spender, and Groen (Citation2010) urged that future theorising could benefit from the study of the managerial processes of deploying resources and capabilities.
Scholars warned that this emergent capability is not realised until enabling conditions are met (Seddon Citation2014). Chen (Citation2012) argues that IT-enabled resources had significant synergistic effects on organisational capabilities when integrated properly and that the emergent digital capability had a stronger impact on operations, research and development, and marketing capabilities than their individual effects.
IT-enabled resources are often the force for changing the operational and management practices of firms (Sandkuhl et al. Citation2019; Warning and Weber Citation2017). Strategic investments in IT-enabled resources may also be critical to the firm’s customer orientation (Nakata and Zhu Citation2010; Souza das Neves et al. Citation2015). Moreover, IT-enabled resources help to improve most business functions (Devaraj and Kohli Citation2003). Jung et al. (Citation2017) proposed that there were discrete stages of performance when firms aimed to develop new smart capabilities in manufacturing systems.
Former research on capabilities has found that capabilities can be developed jointly, in a cumulative fashion. They support the sand cone view, which regards capabilities as complementary rather than mutually exclusive alternatives requiring trade-offs (Narasimhan and Schoenherr Citation2013). The firm can make improvements on multiple dimensions simultaneously by making changes to its existing resources (Schmenner and Swink Citation1998; Schroeder et al. Citation2011). The sand cone metaphor implies that the resources are the foundation, with different layers symbolising different capabilities. The wider the base, the taller the sand cone can be built. However, the analogy of the sand cone also suggests that a substantially greater amount of effort is needed to reach the next stage (Narasimhan and Schoenherr Citation2013). Although successive stages require increasingly higher levels of process integration and coordination, the mastery of the prior stages facilitates learning and the acquisition of new capabilities required for reaching the next stage (Bortolotti et al. Citation2015; Rosenzweig and Roth Citation2004).
According to Boon-Itt and Wong (Citation2016), cumulative capabilities are even more important for manufacturers from less developed countries, because of the synergetic effect among capabilities and their associations with productivity improvements, both of which are stronger for such manufacturers (Schoenherr et al. Citation2012).
Digital maturity
The basic idea behind capability maturity is that higher stages of maturity indicate improved capabilities in managing the specific domain/process (Rapaccini et al. Citation2013). The organisational performance corresponds to its maturity level (Issa et al. Citation2018), the more mature a capability gets, the closer the organisation is to the desired end-stage (Mettler Citation2011). Consequently, the process of becoming mature implies a potential for growth in capability and indicates the consistency of building an infrastructure and corporate culture that supports the development of explicitly defined, managed, measured, and controlled processes, that are mature.
The principal idea of the maturity models is that they describe the typical behaviour exhibited by a firm at each ‘maturity’ stage, which provides the opportunity to codify what might be regarded as good practice (and bad practice) (Fraser et al. Citation2002). Moreover, maturity models help an individual or an entity to reach a more sophisticated maturity stage following a step-by-step continuous improvement process (Mittal et al. Citation2018). They present a systematic approach to auditing and benchmarking an organisation, and ultimately to designating a path for improvement (Amaral et al. Citation2019). The capability maturity modelling (CMM) has been initially used to evaluate the degree of technology maturity. Since then, CMM has also been widely adopted as a general maturity model for business process assessment (Wang and Chen Citation2018).
Recently, maturity models have been developed with a particular focus on the conditions and implications of digital transformation, where the organisation’s current state of digital maturity is understood as a stage in carrying out the complete transformation (Ifenthaler and Egloffstein Citation2020). The total of the stages is considered to be the completion of full transformation and as such being digitally ready for taking advantage of Industry 4.0. The assessment of the current position of a firm provides valuable information on the preparedness of the conditions, resources and capabilities needed for advancement to the next stage (Benedict Citation2017). The underlying assumption of the maturity framework is that only the ‘vital few’ issues at each maturity stage need to be tackled first. Each maturity stage defines clear improvement priorities, with guidance for selecting those few improvement activities which are the foundations for moving into the next stage. Failure to start at the ‘right’ point or fully complete a stage can ultimately prevent the company from advancing to the next stage (Machado et al. Citation2020).
In general, maturity models are portrayed as a matrix, which consists of major components such as (a) a maturity stage (typically three to six levels), (b) a descriptor for each maturity stage (e.g. initial, managed, etc.), (c) a generic description of each stage, (d) a description of the elements or activities that might be performed to reach a successive maturity stage (Ifenthaler and Egloffstein Citation2020). The improvements at each stage provide the foundation on which to build improvements undertaken at the next stage (Paulk et al. Citation1991). For successful implementation of digital transformation, it is necessary to design an evolutionary path for the firm, a roadmap, along which the complex system of digital transformation capability is built up gradually (Issa et al. Citation2018). The difference between a digitally immature and a fully mature organisation is that the former starts going digital, while in the latter not only the infrastructure but also the organisation’s capabilities are mature (Paulk et al. Citation1991).
The capability maturity models differ from one another typically in terms of their dimensions. The dimensions are specific areas within which the company must demonstrate progression. In contrast, the steps are the number of stages that the company needs to go through until it becomes fully mature. The dimensions of CM models also vary immensely – from 2 to 9 – because some models are more specific, focusing on production technology, while other models include socio-economic dimensions, such as people, strategy, and the organisation among others (von Leipzig et al. Citation2017; Amaral et al. Citation2019). In their analysis of 30 CM models on digital transformation, Hizam-Hanafiah et al. (Citation2020) identified 158 dimensions out of which the following 6 were the most common: Technology, People, Strategy, Leadership, Process and Innovation. Technology accounted for 44% of the total dimensions. This is not surprising as today the application of data, computer skills, and information and communication technologies are primarily considered a core capability of successful B2B firms and market leaders (Ritter and Pedersen Citation2019).
Digital technology adoption is a well-researched area. For example, Mittal et al. (Citation2019) analysed 15 maturity models which were all focusing on technologies adopted at various stages of maturity. An important commonality of the maturity models is that the starting point is the consideration of new technologies and the willingness to adopt these technologies. However, the firm and its employees must be ready to use this technology. The indiscriminate application of technology may not necessarily induce progress in technology adoption (Gautam et al. Citation2005).
Schumacher et al. (Citation2016) proposed a maturity model which included a total of 62 maturity items grouped into nine dimensions: strategy, leadership, customers, products, operations, culture, people, governance, and technology. They also provided item descriptions and argued that the inclusion of diverse organisational aspects results in a more comprehensive model.
Reviewing the literature, the following gaps are evident. First, these CM models have emerged from extensive literature reviews or case studies (Frank et al. Citation2019). Very few empirical studies on digital transformation typically use the case method approach with small sample sizes, with a few exceptions (e.g. Bloching et al. Citation2015; Remane et al. Citation2017; Kane et al. Citation2017). Based on an extensive review of maturity models, van Hillegersberg (Citation2019) concluded that the literature lacks empirical studies that validate these models. Second, the maturity models lack an assessment method, and even if an assessment method is available, many models do not specify improvement points explicitly, nor prioritise them (Proença and Borbinha Citation2016). Without specific improvement points, these maturity models are less actionable (Tarhan et al. Citation2016). Third, they also neglect the role of socio-technical factors in the transformation (Tortorella et al. Citation2020). Consequently, the assessments of the digital maturity of firms on a large sample have not yet been validated.
We, therefore, believe that our study fills a critical gap by the validation of the DCMM on a large sample. We aim to assist practitioners in their understanding of how IT-enabled resources are deployed and what is the sequence of developing digital capabilities. The research focuses on the inputs used – particularly the resources – to enable us to evaluate and identify the development status of new digital capabilities during the firm’s digital transformation process. To fill this gap, we relied on previous work that provided a comprehensive and critical review of digital maturity models (i.e. Jokela et al. Citation2006; Mittal et al. Citation2018; Hizam-Hanafiah et al. Citation2020).
In summary, maturity models are valuable instruments for managers because they allow the assessment of the current situation of an organisation as well as the identification of reasonable improvement measures (Becker et al. Citation2009), they indicate how possible deviations toward expected performance can be overcome (De Bruin et al. Citation2005; Ifenthaler and Egloffstein Citation2020), as well as support the management to focus on the right resources and capabilities in a given transformation stage (Rajnai and Kocsis Citation2018). Understanding these various approaches to advancing through a series of stages in the course of digital transformation is a crucial task in the development of a maturity assessment model and is especially important when testing the model for its validity (Mettler Citation2011).
In summary, digital transformation is a must for companies aiming to stay competitive, which means that they need transform their resource base into IT-enabled resources and in the meantime develop digital capabilities. This challenge, however, cannot be answered overnight, it must be done wisely and step-by-step. Consequently, the present research aims to extend our current knowledge by codifying what might be regarded as good practice in carrying out the complete transformation. To do so, the relative comparisons of digital capabilities and the composition of resources across the stages of getting digitally matured is required.
Methodology
This section presents the research methodology of the comparative study of Industry 4.0 aware business-to-business (B2B) companies across four countries. It is organised as the introduction of the empirical context, the research design, data collection, and sample description.
Empirical context
Digital transformation is shifting the ability to add value to the production process, which poses a constant threat to the automotive and logistics industries in particular (Bloching et al. Citation2015). Germany was among the top three countries in the global innovation ranking in 2018 (Schwab Citation2018), while global corporations such as BMW, Daimler, Volkswagen, Bosch, and Siemens are assumed to be the driving forces. Western European societies such as Germany and France have shifted their industrial activities to locations in Central and Eastern Europe (CEE) (Faust et al. Citation2004) into countries that have a shared history and similar economic structure.
Formerly, the delocalisation of production phases of European firms to the CEE countries was the engine of economic growth in the region (De Benedictis and Tajoli Citation2003). Backé et al. (Citation2019) argue that today CEE countries face a somewhat limited scope for continued economic expansion because their current growth model is mostly based on assembly. Moreover, their production structure is tilted toward the automotive industry, and given the fundamental transformation, this sector is bound to go through, the only way these countries could stay at the forefront is if they move closer to the technological frontier by investing in digital transformation (Backé et al. Citation2019). Other studies also brought evidence that CEE countries, due to their close integration with Western European multinationals, can have a reverse impact on the overall progression (Nguyen and Rondeau Citation2019; Simonazzi et al. Citation2013) if they fail to move upward on the global value chain.
Not only are the structures of these economies similar, but they face comparable levels of business dynamism and innovation capability, resulting in a similar level of competitiveness (Schwab Citation2018). For example, empirically analysing the economic growth determinants in ten CEE countries, absolute convergence was found among them (Prochniak Citation2011).
Consequently, the authors argue that B2B firms from the CEE countries, due to their strong interdependencies with other European economies, can have a massive impact on Europe’s long-term competitiveness. The organisational capability of B2B firms to successfully implement digital transformation is, therefore, of contemporary interest to policymakers, economic decision-makers, and business analysts. From the CEE countries, data were gathered from B2B firms in Slovakia, Hungary, Romania, and Serbia and can, therefore, be considered as a single group or region.
Research design
Following the suggestion of (Shah and Ward Citation2007), the methodology is designed around a multi-step approach. This approach is frequently used in operation management for explorative studies that aim to identify homogenous groups of cases when there is no assumption made in advance about the likely relationships within the data. For example, the k-means clustering algorithm has been widely used to represent diversity and reduce redundancy within the data source. Hence, it is a powerful data mining tool, which is frequently used for taxonomy analysis (i.e. Jain et al. Citation1999).
Morgan and Katsikeas (Citation1997) and later Koufteros et al. (Citation1998), both suggested starting to develop measures with item generation based on reviewing theory and former studies as well as structured interviews with practitioners. The initial items must be refined and pretested with the help of industry and academic experts, before proceeding with the large-scale study. A similar method was undertaken in the explorative study by Kane et al. (Citation2017). In line with the suggested methodology, we first identified the initial list of items based on the literature review and presented it to two groups of executives in October and November 2018. The initial items were mainly corresponding with the model of Schumacher et al. (Citation2016). During the focus group discussions, with the involvement of 20 top managers, the dimensions, as well as the items, were discussed extensively. The dimensional structure corresponding to DCMM was refined into five dimensions: strategy, operations, technology, organisation and culture, and customers. They were measured on individual indices by evaluating the current status of digital capabilities in the company ranging from (0) incapable to (100) fully capable. Descriptive statistics of the indices are in Annex 1.
We also developed the ITRS to explore resource deployment. The number of items was expanded to 30 in the following functional areas: technology, strategy, organisation and culture, customers and marketing, production, logistics and procurement. We then operationalised the items using a five-point scale to measure the use of IT-enabled resources ranging from (1) strongly disagree to (5) strongly agree. Quantitative methods for constructing maturity models can be used for identifying configurations (maturity stages, clusters) (Lahrmann and Marx Citation2010) based on data from a Likert-like questionnaire where the respondent scores the relative performance of the organisation on a scale from 1 to n based on the statement of ‘good practice’ (Fraser et al. Citation2002). Descriptive statistics of the items are in Annex 2. Experts translated all the questions into local languages.
The population of the sample is the Industry 4.0 aware B2B companies. The initial list of B2B firms was obtained from local associations that assist with the transformation of the national manufacturing industry. For example, in Hungary, members of the Industry 4.0 National Technology Platform Association were contacted. Altogether, at the time of the research, there were less than 200 companies in each participating country (Slovakia, Hungary, Romania, and Serbia) that were Industry 4.0 aware.
Executives’ self-assessment reports are commonly used in social sciences, especially in marketing and strategic management research (Grinstein and Goldman Citation2006; Warner and Wäger Citation2019). Former research has also suggested that when respondents are familiar with digital transformation and play a key role (e.g. top managers), their opinions could be fairly representative (Szabo et al. Citation2020; Tortorella et al. Citation2020). Amaral et al. (Citation2019) reinforced this view claiming that for an SME to succeed, top management needs to be leading the momentum. Finally, we believe that members of the top management team, regardless of their functional background, are familiar with their organisation’s strategy and as such, they play a critical role in the implementation of digital transformation, therefore, their opinion is representative.
The large-scale data collection
The large-scale data collection took place in April and May 2019 in all four countries via face-to-face interviews and a structured questionnaire. Since our study is exploratory, we considered as the population of interest those B2B firms that are already seeing the potential as well as the necessity of digital transformation and, more than likely, were already at various stages of its implementation.
Consequently, we used a non-random approach for data collection, which is common in survey-based studies when respondents must be familiar with the studied phenomenon so that their opinion will be representative. Our selection criteria were that participants should be members of the top management team in financially and legally autonomous B2B enterprises. Data were collected from a single respondent, in particular, one top manager per B2B firm. Executives who were involved in the refinement of the instrument were purposely left out of the large-scale study.
Because the interviews were face-to-face, they lasted, on average, about 45–60 min. The interviews started with general questions about the enablers and barriers of digital transformation and context specifics. During the collection of the responses, interviewees were allowed to discuss each question with the interviewer if more clarification was required, and then the interviewer recorded their answers. Of course, the insights gained during the interviews enriched our understanding. Altogether, we collected 302 full responses: 78 from Hungary, 29 from Romania, 118 from Serbia, and 77 from Slovakia. These numbers are high, considering that the population of Industry 4.0 aware B2B firms was less than 200 firms in each country at the time of the study. All identified Industry 4.0 aware B2B firms were contacted. We surveyed all those that were open to our research (app. 40% of the population). In addition, the sample purposely comprised homogenous companies to reduce the variability of the data (Burmeister and Aitken Citation2012).
Our approach differs from previous research because most previous studies have used a case study approach for data collection. Our technique not only ensured the legitimacy of the information but also increased response rates and generated a statistically significant amount of data, which makes our results more generalisable. In Romania, we managed to contact businesses only in the Timisoara region, which is considered a limitation of our research.
We believe that the entire population of companies that recognised either the potential or the threat of digital transformation was relatively small at the time of the research, hence the sample of 302 firms are considered to be representative. According to the central limit theorem, the sample size of 30 is considered sufficient for most distributions. In our case, in Romania, the sample size was 29, but for all other countries, more than twice as many were recorded and the total samples are ten times higher than recommended. Finally, the high statistical significance reported in Tables indicates robust and valid results.
Table 1. Identification of digital capability maturity stages.
Table 2. Principal components analysis of IT-enabled resources.
Table 3. IT-enabled resources scale statistics.
Table 4. Resource-based differences in digital capability maturity stages.
Respondents
The characteristics associated with each respondent were examined. A majority were from medium-sized organisations (36%), with 25% from large organisations, 29% from small organisations, and 10% from micro-businesses. The categorisation of company size was based on the OECD classification, with micro-businesses having less than ten employees, small companies from 10 to 49 employees, medium-sized companies from 50 to 249 employees, and large companies with more than 250 employees.
In total, 76% of the respondents were men, and 24% were women. The respondents were from six main functional backgrounds: 31% were CEOs or founders, 25% sales and marketing managers, 20% were production or manufacturing directors, 10% were finance directors, 9% were product development managers, and 5% were logistics managers. The high number of CEOs and founders in the sample is not surprising, because previous research has shown that decisions in small and medium-sized firms are usually made by the senior executive (Barringer and Bluedorn Citation1999). In large organisations, however, the top management team is actively involved in screening the environment and working together to anticipate strategic changes (Yadav et al. Citation2007). Previous studies have also confirmed that digital decision-making is usually the responsibility of the executive team because the digital transformation agenda has significant strategic importance. Unless it is driven from the top of the enterprise, it will not have the required momentum to drive business change (Péladeau and Acker Citation2019).
This, therefore, confirmed the integrity of the data, because participants responded on issues within their domain of management responsibility, indicating the limited potential effect of key informant bias, which is in line with previous research (Morgan and Katsikeas Citation1997).
Analysis and results
This section presents the analysis of the data in line with the research interests: identification of DCM stages followed by the scale construction of IT-enabled resources in order to answer the research question of how the composition of resources change when firms acquire digital capabilities. Since there is little empirical research on digital transformation, we followed the basic principle that the items used to measure a single construct should converge with each other, but diverge from items that measure other constructs (Nunally Citation1970).
Digital capability maturity stages
First, the DCMM was analysed by k-means cluster exploratory data analysis. The analysis produced four separate groups with distinct, non-overlapping boundaries. To validate the stability of the clustering, we chose the Euclidean distance measure of similarity, as suggested by Morgan and Katsikeas (Citation1997).
The scoring value for the measurement indices of digital capability maturity ranged from 0 to 100, where 100 indicated complete maturity. Descriptive statistics of the indices are in Annex 1. The assignment to a maturity stage was made based on the given score calculated for the four maturity stages (Azhari et al. Citation2014). Four maturity stages were identified. These stages were named Novice (new to), Beginner (learning), Competent (practising), and Expert (transformed). The dimension, in a particular technology, operations, organisation, culture and customers were all evolving significantly at each maturity stage. We found that there is a massive leap from Novice to Beginner in all competencies except for organisation and culture.
The Hungarian subsample contains more mature and less immature firms than the Slovakian subsample (mean difference (MD) = .044, p < .05), while there are no other significant regional, firm size or sectoral differences. The respondents’ functional background has no impact on the results.
IT-enabled resource scale
We analysed the ITRS with 30 items that respondents scored on a Likert scale from 1 to 5. Upon close examination, it can be suggested that our measures of strategy and organisation resources depict an element of ‘qualification’ which might suggest that these are more capabilities (outcomes) than resources (inputs).Footnote1 We suggest that these are not capabilities per se but rather they are the strategic and organisational imperatives that provide the resource repertoire for developing coherence in digital strategy and digital coordination across the organisation. In this sense, we make no inference as to the capability value or capability rarity for example. Furthermore, there is no measurement equivalence in terms of competitor referents or comparison with other firms and so consistent with the working definition of resources that we adopt. We note especially that these items capture these repeated routines are the qualified resources that enable capabilities to be orchestrated with other resources to create products and services (Amit et al. Citation1993). It remains though that resources and capabilities are often synergistic in nature and can be more valuable when combined (Paulraj Citation2011). Descriptive statistics of the items are in Annex 2.
Following former research (cf. Morgan and Katsikeas Citation1997), the data were reduced to meaningful dimensions and were used as factor scales in the principal component analysis to explore the latent dimensionality of the ITRS construct. The purpose was to determine the smallest number of underlying dimensions that accounted for the maximum proportion of the total variance in the data.
The Kaiser–Meyor–Olkin (KMO) coefficient, a measure of sampling adequacy, was 0.918, and the chi-square value of Bartlett’s test of sphericity was 5119.808 with 435 degrees of freedom (p < .001) concluding that the using factor analysis on the data set was appropriate. The KMO value suggested that the degree of common variance was middling and the values for Bartlett’s test suggest that the sample inter-correlation matrix did not come from a population in which the inter-correlation matrix was an identity matrix. Consequently, the variables in the population correlation matrix are uncorrelated. The analysis extracted six factors based upon an eigenvalue criterion of one or more in combination with the scree test. This solution explained 64.63% of the total variance and was characterised by strong individual loadings on each factor.
As a result, we obtained six factors that were labelled as Technology, Strategy & Organisation, Marketing, Production, Logistics, and Procurement. shows the principal components analysis of firms’ usage of IT-enabled resources. The principal components analysis reveals that the correlations between the principal components and the original variables are greater than 0.5, which suggests that the resources vary together.
Slovakian companies use more digital resources in the production process than Hungarian and Serbian companies (Mean Difference of the factor scores (MDf) = .85 & 0.73, p < .01), and focus more on technology than Romanian companies (MDf = .45, p < .05). Moreover, Hungarian companies have more focus on strategy and organisation than Romanian companies (MDf = .64, p < .05). Besides, there are no significant regional, firm size, or sectoral differences. The respondents’ functional background has no impact on the results.
Scale construction of IT-enabled resources
Indices were constructed for each factor by calculating the composite scale mean of the items with a loading of 0.50 or higher (Gerbing and Anderson Citation1988). For the assessment of scale reliability, in the form of variation attributable to random error, Cronbach’s alpha coefficient was calculated. All the scales satisfied the required threshold (Nunnally Citation1975) of acceptable reliability with an alpha value of 0.70 or higher. Item-total correlation analyses gauged the validation of each scale. All correlation coefficients were positive and high, in the anticipated direction and statistically significant where p < .001 (), suggesting that no scale item needed to be deleted. concludes that all the resources tend to increase together.
shows a consistent pattern of digital capability development. The progression starts with the development of technology-related capabilities, but strategic, production, marketing, and procurement capabilities equally develop. As the firm progresses along the maturity stages, they tend to further develop those capabilities. Post hoc comparisons using one-way analysis of variance, in conjunction with Scheffe’s multiple comparisons, were used to examine the significance of differences amongst the maturity stages. Scheffe’s comparison highlights the sequence and stages of progression in each dimension.
Discussion and conclusions
The academic contribution and novelty of this paper are the development of a measurement scale for DCMM and its empirical investigation of a large sample of B2B firms. The results help in particular manufacturing firms to build capabilities for successful digital transformation.
The analysis of the results showed that there are four stages in progressing from novice to digitally mature firm. The results confirm the sand cone model since all 5 dimensions of capabilities are built up gradually. Consequently, digital capabilities are complementary rather than mutually exclusive to one another, and successful firms can progress on multiple dimensions simultaneously by making changes to the existing capabilities. The capability development should be balanced to become more mature.
The results also reveal that the formation of a strategy is critical for launching the transformation process. Novice firms start by exploring how to benefit from digital transformation, therefore the management starts prioritising and planning the digital initiatives. At the same time, the technology, operations, and organisational culture must begin to change simultaneously. Surprisingly, customer-related capabilities are lower at this stage. In the beginner phase, the capabilities related to technology’s domain come forward, while the development of new organisational cultural capabilities is lagging. An underlying explanation could be that when implementation begins, it is critical to master first the use of technology. Besides, cultural transformation requires a change in the values, attitudes, and assumptions of the organisational members, which takes time. The next stage is competence; this is where a great progression in terms of organisational capabilities happens. Possibly, because this is where most of the organisational and cultural changes are implemented. It may require internal reorganisation, as well as the training and development of employees. Competent firms further develop their technology-related capabilities, while the other four dimensions of capabilities are progressing to a relatively same level. In the expert stage, all five capabilities are equally progressing, and they reach maturity together. That is when the firm becomes digitally fully mature.
The second contribution of the results is the identification of resource configurations that describe a digital capability maturity stage. To answer the question of how IT-enabled resource configurations evolve during the development of digital maturity, our findings highlight a distinct pattern among Novice, Beginner, Competent, and Expert firms in terms of their resource use. The Novice and Expert stages of maturity are very different in their resource-use patterns. A detailed description of the stages is shown in , based on the methodological considerations made by Ifenthaler and Egloffstein (Citation2020), Issa et al. (Citation2018) and Proença and Borbinha (Citation2016).
Table 5. Digital Capability Maturity Model summary.
Highly-mature groups of firms use significantly more IT-enabled resources than less mature firms. Novice and Beginner firms use digital technologies moderately, and Competent and Expert firms use them significantly more. This difference implies that there is a definite shift between the second and third maturity stages. A possible explanation is that it takes time to absorb new information, interpret its meaning, build capabilities, and then mobilise the organisation to act.
The results show that firms usually start using digital resources in the field of technology, then move into strategy and organisation, production, marketing, and procurement, with logistics lagging. We, therefore, suggest that ample evidence has been found that digital capabilities are complementary rather than mutually exclusive of one another, and successful digital transformation requires firms to develop these capabilities simultaneously.
In addition to the scientific contributions, this work also has significant practical implications which are summarised as follows:
Implications for practitioners
Our findings have several implications for practitioners and managers. In recent years, it has become increasingly clear that we need a better understanding of the management of digital transformation. The digital maturity model presented in provides a framework that can be used by managers to prepare for digital transformation, benchmark their own progress, and understand what is needed to reach a successive maturity stage or how to prioritise their efforts. It is based on an empirically tested assessment tool, which gives suggestions on how to move forward to the successive maturity stage, and how to prioritise work. We suggest that managers may wish to consider the following actions:
The results have the potential to be used for benchmarking, especially for identifying a firm’s capability gaps. Understanding in which functional area the firm is efficient or deficient in digital capabilities is critical for progression in maturity, as this is the first step to move to the next stage.
Digital capabilities are complementary rather than mutually exclusive of one another. Digital transformation requires firms to develop these capabilities simultaneously, while capability development should be balanced.
The failure to develop these capabilities and deploy these resources in the right order and right amount (e.g. by under- or over-developing a capability in a given stage or under- or over-using an IT-enabled resource) will lead to a variety of predictable organisational problems and transformation ‘pains’.
Firms that have been successful in managing digital transformation (Experts) understood the sequence, the right combination of IT-enabled resources and the interconnectedness of these five digital capabilities.
Implications for policymakers
Our findings have several implications for policymakers, too. B2B firms, especially manufacturing firms in the CEE region, are worth studying because their digital transformation capabilities and practices affect the growth and stability of the whole of Europe. Studying these firms is crucial, but focusing solely on a few limits the development of knowledge. The results also confirm Furr and Shipilov’s (Citation2019) findings, that digital transformation is indeed as much about organisational change as technology. To succeed, firms should be incentivised and supported to think beyond investment into technology and think in terms of developing capabilities in all five dimensions.
Summary, limitations, and directions for future research directions
The main scientific contributions of the article are as follows: First, until now, there has been no widely accepted model to assess either digital capability maturity or scales to measure IT-enabled resources. The present research aims to fill this gap. First, it provides a measurement for capability maturity during digital transformation by addressing the critical resources and capabilities of the firm. Based on a sample of 302 B2B firms, the study reveals that there are distinct patterns of differential resource deployment underlying each capability stage, and at each stage, managers need to shift focus to ensure progress. Progress toward digital maturity requires careful consideration of the socio-technical factors of the firms. Besides technology, strategy- and organisation-related IT-enabled resources are the key drivers of digital transformation. Other functional areas, including production, marketing, procurement and logistics, are followers. Finally, the results confirm that digital capability improves at each maturity stage and that the most mature firms use significantly more IT-enabled resources.
The research has its inherent limitations. First, it is based on subjectivity and human perception of understanding digitalisation. We have tried to overcome this limitation by surveying and interviewing key informants from the top management team of Industry 4.0 aware companies. Many of the respondent firms achieved the (currently possible) highest stage of digital transformation and could serve as role models for others. Second, this caused another limitation, that the population was very limited at the time of the survey, i.e. less than 200 companies in each participating country (which has been rapidly growing since then). Because former research suggested that many firms need support in the implementation of digital transformation, hence the purposive sampling technique was used to collect insights from experts and explore the progression of digital capabilities across the stages of the maturity curve. Third, the data collection was limited to four CEE countries, and in the case of Romania, only to the Timisoara Region. The authors believe that the relevance and validity of obtained results could be improved by extending this research to a European level. Former research found that there are still significant differences between CEE countries in terms of uncertainty avoidance or future orientation (i.e. Catana et al. Citation2013). Consequently, future research is needed to investigate whether firms differ from one another in terms of business and societal cultural values as well as practices.
Finally, another limitation of the present study is the application of the non-probability sampling technique which does not allow researchers to control variability and bias. The sample is strongly biased towards the inclusion of those firms that are already actively engaged in digital transformation and thereby exhibit advanced knowledge of the requisite capabilities. Nevertheless, the use of a non-probability approach for data collection is common in survey-based studies because it allows for patterns to be delineated and much information can become available. Moreover, Goodman and Blum (Citation1996) found that non-random sampling may affect the means and the variance of some of the variables, but does not affect the relationships among the variables. Hence, Faugier and Sargeant (Citation1997) recommended the application of non-random sampling for researching members of special populations. In conclusion, the calculated boundaries of maturity levels may need a follow-up investigation given the preponderance of firms identified as both ‘competent’ and ‘expert’ most especially.
To summarise, our paper draws scholarly attention to the need to study digital maturity through systematic, quantitative research. Previous studies did not provide a proper, quantifiable assessment tool, and a generalisable framework. Their findings can only really be interpreted in the given context. In contrast, our model contributes to the understanding of what managers can do to enhance the probability of successful digital transformation and avoid the pitfalls of the change.
The results raise many questions which should be addressed by future research, such as whether B2C firms show similar progress, whether the results vary across regions, and how digital capabilities and IT-enabled resources enhance firm performance over time. Scholarly work is at the embryonic stage of understanding the effects of digitalisation and digital transformation, and there are many possible paths for future research.
Disclosure statement
No potential conflict of interest was reported by the author(s).
Data Availability Statement
The data that support the findings of this study are available on request from the corresponding author, Lilla Hortovanyi. The data are not publicly available due to containing information that could compromise the privacy of research participants.
Additional information
Funding
Notes on contributors

Lilla Hortovanyi
Lilla Hortovanyi* (PhD, Dr. Habil) holds a Senior Fellow Researcher position at Mathias Corvinus Collegium Alapitvany. She has 15+ years of leadership experience in the management of IT at multi-unit firms. She has been involved in a number of research projects on the social and economic impacts of technological progress. She is the author and co-author of several research papers and books as well as book chapters about strategy and entrepreneurship. Her main areas of expertise are strategic and change management, corporate entrepreneurship, organizational ambidexterity, digital transformation, and Industry 4.0.

Robert E. Morgan
Robert E. Morgan (Ph.D.) holds the Sir Julian Hodge Chair and is a Professor of Marketing and Strategy at Cardiff University. He is a Strategy Research Foundation Scholar and hold visiting professorships including VU Universiteit Amsterdam and Copenhagen Business School. His research focuses on firm capabilities, new product development and technology management from a strategy perspective. His work has appeared in the British Journal of Management, Strategic Management Journal, Journal of Management Studies, Journal of the Academy of Marketing Science, Journal of Service Research, Strategic Entrepreneurship Journal and Journal of Product Innovation Management, among others.
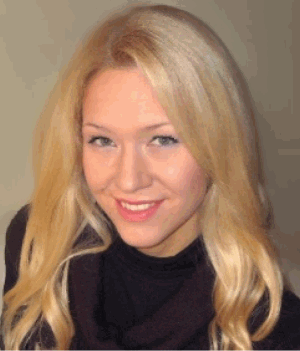
Iva Vuksanovic Herceg
Iva Vuksanovic Herceg (Ph.D.) is an Associate Professor at the Faculty of Economics, University of Belgrade. She teaches undergraduate courses Strategic Management and Enterprise risk management, graduate course Strategic Finance, and PhD course Economics of Strategy. She received her PhD degree from the Faculty of Economics, University of Belgrade. She managed both domestic as well as EU funded research projects. She is Visiting Scholar at the Faculty of Economics and Business University in Zagreb.
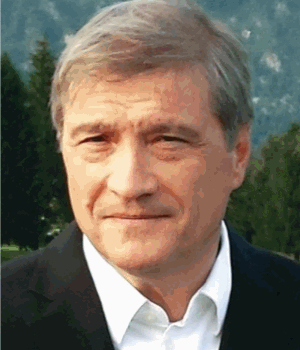
Dragan Djuricin
Dragan Djuricin (Ph.D.) is a fellow of the World Academy of Art and Science as well as a founder and board member of the Serbian Chapter of the Club of Rome. He is a retired professor of Strategic Management, Project Management, Enterprise Risk Management, Economics of Strategy, and Strategic Financial Management at the University of Belgrade, Faculty of Economics. He wrote dozens of books in the fields of strategic management, project management, systemic transition, and risk management.
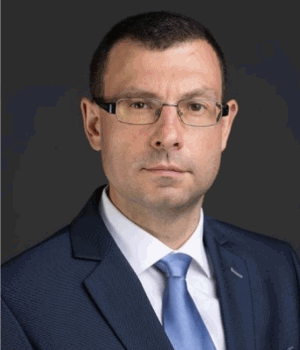
Robert Hanak
Robert Hanak (Ph.D.) is an Associate Professor in the Department of Information Management at the Faculty of Business Management, the University of Economics in Bratislava. His research interests include i) decision making: complex and complicated decision task, factors influencing decision outcome such as experience, expertise, intuition, rationality, cognitive profile, social factors; ii) information and communication technologies and data analysis.
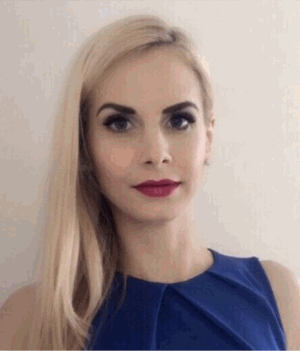
Dora Horvath
Dora Horvath is a doctoral candidate and assistant lecturer at the Corvinus University of Budapest, Department of Strategy and Management. Besides her academic role, she works in the banking industry. She published in highly ranked international journals such as MIT Sloan Management Review, Technological Forecasting & Social Change, Renewable and Sustainable Energy Reviews. Her main areas of expertise are strategic management, business model innovation, digital transformation, and Industry 4.0.
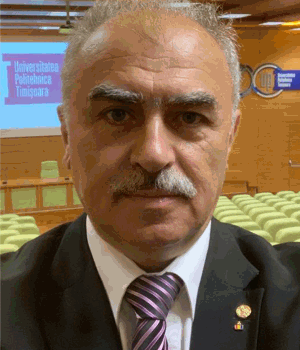
Marian L. Mocan
Marian L. Mocan (Ph.D.) is a university professor at the Polytechnic University of Timisoara. He teaches Strategic Management, Logistics and Supply Chain Management courses at the university. He is the coordinator of the Department of Entrepreneurship and European Projects of the university. He is a PhD supervisor in the field of Engineering and Management. Outside the university, he has had a business and management consulting firm since 2000. He was the chairman of the board of directors of Timisoara International Airport from 2014-2020.
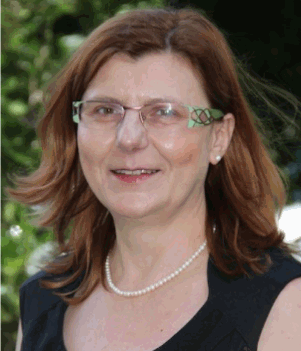
Anita Romanova
Anita Romanova (PhD) is an associate professor in the Department of Information Management. She is a member of the scientific committee of the Faculty of Business Management and of University of Economics in Bratislava. Her research interests include information technology, e-commerce, especially Enterprise Resource Planning (ERP) systems, IT governance, information systems management, IT management, and information systems effectiveness.
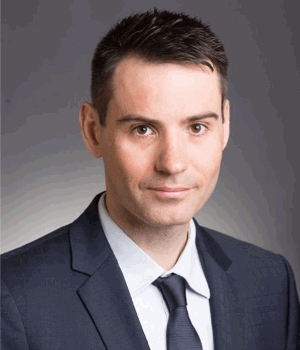
Roland Z. Szabo
Roland Z. Szabo (Ph.D., Dr. Habil) works in the fields of academia, business and consultancy. He is an Associate Professor at Széchenyi István University. He is currently helping B2B firms to succeed in digital transformation. He has received three international Best Paper Awards (2019, 2018, 2017), and published in highly ranked journals, such as Renewable & Sustainable Energy Reviews, Technological Forecasting & Social Change, Cities, Technology in Society and Sustainability. His main areas of expertise are strategic and change management, business model innovation, entrepreneurship, organizational ambidexterity, digital transformation, and Industry 4.0.
Notes
1 We thank an anonymous reviewer for indicating this.
References
- Amaral, A., D. Jorge, and P. Peças. 2019. “Small Medium Enterprises and Industry 4.0: Current Models’ Ineptitude and the Proposal of a Methodology to Successfully Implement Industry 4.0 in Small Medium Enterprises.” Procedia Manufacturing 41: 1103–1110. doi:10.1016/j.promfg.2019.10.039.
- Amit, R., P. J. H. J. Schoemaker, Raphael Amit, and P. J. H. J. Schoemaker. 1993. “Strategic Assets and Organizational Rent.” Strategic Management Journal 14 (1): 33–46. doi:10.1002/smj.4250140105.
- Azhari, P., N. Faraby, A. Rossmann, B. Steimel, and K. S. Wichmann. 2014. Digital transformation report 2014. Neuland Gmbh. https://www.wiwo.de/downloads/10773004/1/dta_report_neu.pdf.
- Backé, P., I. Korhonen, D. Ritzberger-Grünwald, and L. Solanko. 2019. “A Tribute to 30 Years of Transition in CESEE.” Focus on European Economic Integration Q3: 11–28.
- Barney, J., M. Wright, and D. J. Ketchen. 2001. “The Resource-Based View of the Firm: Ten Years After 1991.” Journal of Management 27 (6): 625–641. doi:10.1177/014920630102700601.
- Barringer, B. R., and A. C. Bluedorn. 1999. “The Relationship Between Corporate Entrepreneurship and Strategic Management.” Strategic Management Journal 20 (5): 421–444.
- Becker, J., R. Knackstedt, and J. Pöppelbuß. 2009. “Developing Maturity Models for IT Management: A Procedure Model and Its Application.” Business & Information Systems Engineering 1 (3): 213–222. doi:10.1007/s12599-009-0044-5.
- Benedict, G. F. 2017. “Nontraditional Manufacturing Processes.” In Nontraditional Manufacturing Processes. CRC Press. doi:10.1201/9780203745410
- Berman, S. J. 2012. “Digital Transformation: Opportunities to Create new Business Models.” Strategy & Leadership 40 (2): 16–24. doi:10.1108/10878571211209314.
- Bharadwaj, A. S. 2000. “A Resource-Based Perspective on Information Technology Capability and Firm Performance: An Empirical Investigation.” MIS Quarterly 24 (1): 169–196. doi:10.2307/3250983.
- Bharadwaj, A., O. A. El Sawy, P. A. Pavlou, and N. Venkatraman. 2013. “Digital Business Strategy: Toward a Next Generation of Insights.” MIS Quarterly, doi:10.25300/MISQ/2013/37:2.3.
- Bibby, L., and B. Dehe. 2018. “Defining and Assessing Industry 4.0 Maturity Levels – Case of the Defence Sector.” Production Planning & Control 29 (12): 1030–1043. doi:10.1080/09537287.2018.1503355.
- Bloching, B., P. Leutiger, T. Oltmanns, C. Rossbach, T. Schlick, G. Remane, P. Quick, and O. Shafranyuk. 2015. “The Digital Transformation of Industry.” In Roland Berger Strategy Consultants GmbH & BDI.
- Boon-Itt, S., and C. Y. Wong. 2016. “Empirical Investigation of Alternate Cumulative Capability Models: A Multi-Method Approach.” Production Planning & Control 27 (4): 299–311. doi:10.1080/09537287.2015.1124299.
- Boothby, D., A. Dufour, and J. Tang. 2010. “Technology Adoption, Training and Productivity Performance.” Research Policy 39 (5): 650–661. doi:10.1016/j.respol.2010.02.011.
- Bortolotti, T., P. Danese, B. B. Flynn, and P. Romano. 2015. “Leveraging Fitness and Lean Bundles to Build the Cumulative Performance Sand Cone Model.” International Journal of Production Economics 162: 227–241. doi:10.1016/j.ijpe.2014.09.014.
- Brettel, M., N. Friederichsen, M. Keller, and M. Rosenberg. 2014. “How Virtualization, Decentralization and Network Building Change the Manufacturing Landscape: An Industry 4.0 Perspective.” International Journal of Mechanical, Industrial Science and Engineering 8 (1): 37–44.
- Burmeister, E., and L. M. Aitken. 2012. “Sample Size: How Many Is Enough?” Australian Critical Care 25 (4): 271–274. doi:10.1016/j.aucc.2012.07.002.
- Catana, D., D. Pučko, and R. Krzykała-Schaefer. 2013. “How Future Managers View Societal Culture: A Comparison Across Seven CEE Countries.” Journal of East European Management Studies 18: 463–481. doi:10.5771/0949-6181-2013-4-463.
- Chae, B., and D. Olson. 2021. “Technologies and Applications of Industry 4.0: Insights from Network Analytics.” International Journal of Production Research, 1–23. doi:10.1080/00207543.2021.1931524.
- Chen, J.-L. 2012. “The Synergistic Effects of IT-Enabled Resources on Organizational Capabilities and Firm Performance.” Information & Management 49: 142–150. doi:10.1016/j.im.2012.01.005.
- Chen, G., P. Wang, B. Feng, Y. Li, and D. Liu. 2020. “The Framework Design of Smart Factory in Discrete Manufacturing Industry Based on Cyber-Physical System.” International Journal of Computer Integrated Manufacturing 33 (1): 79–101. doi:10.1080/0951192X.2019.1699254.
- Chirumalla, K., P. Oghazi, and V. Parida. 2018. “Social Media Engagement Strategy: Investigation of Marketing and R&D Interfaces in Manufacturing Industry.” Industrial Marketing Management 74 (5): 138–149. doi:10.1016/j.indmarman.2017.10.001.
- Colli, M., O. Madsen, U. Berger, C. Møller, B. V. Wæhrens, and M. Bockholt. 2018. “Contextualizing the Outcome of a Maturity Assessment for Industry 4.0.” IFAC-PapersOnLine 51 (11): 1347–1352. doi:10.1016/j.ifacol.2018.08.343.
- Davenport, T. H., and G. Westerman. 2018. “Why So Many High-Profile Digital Transformations Fail.” Harvard Business Review 9: 15.
- De Benedictis, L., and L. Tajoli. 2003. Economic integration, similarity and convergence in the EU and CEECs trade structures. (No. 148).
- De Bruin, T., M. Rosemann, R. Freeze, and U. Kaulkarni. 2005. “Understanding the Main Phases of Developing a Maturity Assessment Model.” In Australasian Conference on Information Systems (ACIS). Australasian Chapter of the Association for Information Systems, edited by D. Bunker, B. Campbell, and J. Underwood, 8–19.
- Devaraj, S., and R. Kohli. 2003. “Performance Impacts of Information Technology: Is Actual Usage the Missing Link?” Management Science 49 (3): 273–289. doi:10.1287/mnsc.49.3.273.12736.
- Dolgui, A., and D. Ivanov. 2021. “5G in Digital Supply Chain and Operations Management: Fostering Flexibility, end-to-end Connectivity and Real-Time Visibility Through Internet-of-Everything.” International Journal of Production Research 60 (2): 442–451. doi:10.1080/00207543.2021.2002969.
- El Sawy, O. A., A. Malhotra, Y. Park, and P. A. Pavlou. 2010. “Seeking the Configurations of Digital Ecodynamics: It Takes Three to Tango.” Information Systems Research. 21 (4): 835–848. doi:10.1287/isre.1100.0326.
- Erol, S., A. Schumacher, and W. Sihn. 2016. “Strategic Guidance Towards Industry 4.0: A Three-Stage Process Model.” International Conference on Competitive Manufacturing, 495–501.
- Faugier, J., and M. Sargeant. 1997. “Sampling Hard to Reach Populations.” Journal of Advanced Nursing 26 (4): 790–797. doi:10.1046/j.1365-2648.1997.00371.x.
- Faust, M., U. Voskamp, and V. Wittke. 2004. European Industrial Restructuring in a Global Economy: Fragmentation and Relocation of Value Chains. Soziologisches Forschungsinstitut, Sofi.
- Fischer, M., F. Imgrund, C. Janiesch, and A. Winkelmann. 2020. “Strategy Archetypes for Digital Transformation: Defining Meta Objectives Using Business Process Management.” Information & Management, 103262–103213. doi:10.1016/j.im.2019.103262.
- Frank, A. G., L. S. Dalenogare, and N. F. Ayala. 2019. “Industry 4.0 Technologies: Implementation Patterns in Manufacturing Companies.” International Journal of Production Economics 210 (September 2018): 15–26. doi:10.1016/j.ijpe.2019.01.004.
- Fraser, P., J. Moultrie, and M. Gregory. 2002. “The Use of Maturity Models/Grids as a Tool in Assessing Product Development Capability.” IEEE International Engineering Management Conference, 244–249. doi:10.1109/IEMC.2002.1038431.
- Furr, N., and A. Shipilov. 2019. “Digital Doesn’t Have to be Disruptive: The Best Results Can Come From Adaptation Rather than Reinvention.” Harvard Business Review 97 (4): 94–104.
- Ganzarain, J., and N. Errasti. 2016. “Three Stage Maturity Model in SME’s Toward Industry 4.0.” Journal of Industrial Engineering and Management 9 (5): 1119–1128. doi:10.3926/jiem.2073.
- Gautam, R., W. A. Muhanna, and J. B. Barney. 2005. “Information Technology and the Performance of the Customer Service Process: A Resource-Based Analysis.” MIS Quarterly 29 (4): 625–652. doi:10.2307/25148703.
- Gebhardt, M., M. Kopyto, H. Birkel, and E. Hartmann. 2021. “Industry 4.0 Technologies as Enablers of Collaboration in Circular Supply Chains: A Systematic Literature Review.” International Journal of Production Research, 1–29. doi:10.1080/00207543.2021.1999521.
- Gerbert, P., C. Gauger, & S. Steinhäuser (2015). The Double Game of the Digital Strategy (p. 6). BCG Perspectives.
- Gerbing, D. W., and J. C. Anderson. 1988. “An Updated Paradigm for Scale Development Incorporating Unidimensionality and its Assessment.” Journal of Marketing Research 25 (2): 186–192. doi:10.1177/002224378802500207.
- Goodman, J. S., and T. C. Blum. 1996. “Assessing the non-Random Sampling Effects of Subject Attrition in Longitudinal Research.” Journal of Management 22 (4): 627–652. doi:10.1177/014920639602200405.
- Gökalp, Ebru, and V. Martinez. 2021. “Robust Capacity Planning for Sterilisation Department of a Hospital.” International Journal of Production Research 59: 1–15. doi:10.1080/00207543.2021.2015807.
- Gökalp, E., U. Şener, and P. E. Eren. 2017. “Development of an Assessment Model for Industry 4.0: Industry 4.0-MM.” In Software Process Improvement and Capability Determination. SPICE 2017. Communications in Computer and Information Science, edited by A. Mas, A. Mesquida, R. O’Connor, T. Rout, and A. Dorling. Springer. doi:10.1007/978-3-319-67383-7_10
- Grant, R. M. 1991. “The Resource-Based Theory of Competitive Advantage: Implications for Strategy Formulation.” California Management Review 33 (3): 114–135. doi:10.2307/41166664.
- Grewal, R., and R. J. Slotegraaf. 2007. “Embeddedness of Organizational Capabilities.” Decision Sciences 38 (3): 451–488. doi:10.1111/j.1540-5915.2007.00166.x.
- Grinstein, A., and A. Goldman. 2006. “Characterizing the Technology Firm: An Exploratory Study.” Research Policy 35 (1): 121–143. doi:10.1016/j.respol.2005.09.003.
- Guidici, A., and P. Reinmoeller. 2012. “Dynamic Capabilities in the Dock: A Case of Reification?” Strategic Organization 10 (4): 436–449. doi:10.1177/1476127012457977.
- Hizam-Hanafiah, M., M. A. Soomro, and N. L. Abdullah. 2020. “Industry 4.0 Readiness Models: A Systematic Literature Review of Model Dimensions.” Information 11 (7): 364. doi:10.3390/info11070364.
- Ifenthaler, D., and M. Egloffstein. 2020. “Development and Implementation of a Maturity Model of Digital Transformation.” TechTrends 64: 302–309. doi:10.1007/s11528-019-00457-4.
- Issa, A., A. Hatiboglu, A. Bildstein, and T. Bauernhansl. 2018. Industrie 4.0 roadmap: Framework for digital transformation based on the concepts of capability maturity and alignment. 51st CIRP Conference on Manufacturing Systems, 973–978.
- Ivanov, D., A. Dolgui, and B. Sokolov. 2019. “The Impact of Digital Technology and Industry 4.0 on the Ripple Effect and Supply Chain Risk Analytics.” International Journal of Production Research 57 (3): 829–846. doi:10.1080/00207543.2018.1488086.
- Jain, A. K., M. N. Murty, and P. J. Flynn. 1999. “Data Clustering: A Review.” ACM Computing Surveys 31 (3): 264–323. doi:10.1145/331499.331504.
- Jiang, Z., S. Yuan, J. Ma, and Q. Wang. 2021. “An Online Community-Based Dynamic Customisation Model: The Trade-off Between Customer Satisfaction and Enterprise Profit.” International Journal of Production Research 59: 1–29. doi:10.1080/00207543.2019.1693649.
- Jokela, T., M. Siponen, N. Hirasawa, and J. Earthy. 2006. “A Survey of Usability Capability Maturity Models: Implications for Practice and Research.” Behaviour & Information Technology 25 (3): 263–282. doi:10.1080/01449290500168079.
- Jung, K., S. Choi, B. Kulvatunyou, H. Cho, and K. C. Morris. 2017. “A Reference Activity Model for Smart Factory Design and Improvement.” Production Planning & Control 28 (2): 108–122. doi:10.1080/09537287.2016.1237686.
- Kagermann, H., W. Wahlster, and J. Helbig. 2013. Recommendations for Implementing the Strategic Initiative Industrie 4.0: Securing the Future of German Manufacturing Industry. In Final Report of the Industrie 4.0 Working Group, Forschungsunion (Issue April). doi:10.13140/RG.2.1.1205.8966.
- Kane, G. C., D. Palmer, A. N. Phillips, D. Kiron, and N. Buckley. 2017. Achieving digital maturity. MIT Sloan Management Review, 31. http://sloanreview.mit.edu/digital2017.
- Koufteros, X. A., M. A. Vonderembse, and W. J. Doll. 1998. “Developing Measures of Time-Based Manufacturing.” Journal of Operations Management 16 (1): 21–41. doi:10.1016/S0272-6963(97)00027-2.
- Kouropalatis, Y., A. Giudici, and O. A. Acar. 2018. “Business Capabilities for Industrial Firms: A Bibliometric Analysis of Research Diffusion and Impact Within and Beyond Industrial Marketing Management.” Industrial Marketing Management 83: 8–20. doi:10.1016/j.indmarman.2018.11.012.
- Kraaijenbrink, J., J. C. Spender, and A. J. Groen. 2010. “The Resource-Based View: A Review and Assessment of Its Critiques.” Journal of Management 36 (1): 349–372.
- Kusiak, A. 2017. “Smart Manufacturing Must Embrace big Data.” Nature 544 (7648): 23–25. doi:10.1038/544023a.
- Lahrmann, G., and F. Marx. 2010. “Systematization of Maturity Model Extensions.” In Global Perspectives on Design Science Research, edited by R. Winter, J. L. Zhao, and S. Aier, 522–525. Springer.
- Liao, Y., F. Deschamps, E. De Freitas, R. Loures, L. Felipe, and P. Ramos. 2017. “Past, Present and Future of Industry 4.0 - a Systematic Literature Review and Research Agenda Proposal.” International Journal of Production Research 55 (12): 3609–3629. doi:10.1080/00207543.2017.1308576.
- Loonam, J., S. Eaves, V. Kumar, and G. Parry. 2018. “Towards Digital Transformation: Lessons Learned from Traditional Organizations.” Strategic Change 27 (2): 101–109. doi:10.1002/jsc.2185.
- Lucato, W. C., A. P. T. Pacchini, F. Facchini, and G. Mummolo. 2019. “Model to Evaluate the Industry 4.0 Readiness Degree in Industrial Companies.” IFAC-PapersOnLine 52 (13): 1808–1813. doi:10.1016/j.ifacol.2019.11.464.
- Machado, C. G., M. P. Winroth, and E. H. D. Ribeiro da Silva. 2020. “Sustainable Manufacturing in Industry 4.0: An Emerging Research Agenda.” International Journal of Production Research 58 (5): 1462–1484. doi:10.1080/00207543.2019.1652777.
- Mettler, T. 2011. “Maturity Assessment Models: A Design Science Research Approach.” International Journal of Society Systems Science 3 (1/2): 81–98. doi:10.1504/IJSSS.2011.038934.
- Mittal, S., M. A. Khan, J. K. Purohit, K. Menon, D. Romero, and T. Wuest. 2019. “Contour Parallel Tool Path Planning Based on Conformal Parameterisation Utilising Mapping Stretch Factors.” International Journal of Production Research 57: 1–15. doi:10.1080/00207543.2018.1456699.
- Mittal, S., M. A. Khan, D. Romero, T. Wuest, and S. Mittal. 2018. “A Critical Review of Smart Manufacturing & Industry 4.0 Maturity Models: Implications for Small and Medium-Sized Enterprises (SMEs).” Journal of Manufacturing Systems 49: 194–214. doi:10.1016/j.jmsy.2018.10.005.
- Moeuf, A., S. Lamouri, R. Pellerin, S. Tamayo-Giraldo, E. Tobon-Valencia, and R. Eburdy. 2020. “Identification of Critical Success Factors, Risks and Opportunities of Industry 4.0 in SMEs.” International Journal of Production Research 58 (5): 1384–1400. doi:10.1080/00207543.2019.1636323.
- Moeuf, A., R. Pellerin, S. Lamouri, S. Tamayo-Giraldo, and R. Barbaray. 2018. “The Industrial Management of SMEs in the era of Industry 4.0.” International Journal of Production Research 56 (3): 1118–1136. doi:10.1080/00207543.2017.1372647.
- Morgan, R. E., and C. S. Katsikeas. 1997. “Obstacles to Export Initiation and Expansion.” Omega 25 (6): 677–690. doi:10.1016/S0305-0483(97)00035-2.
- Müller, Julian Marius, O. Buliga, and K. I. Voigt. 2018a. “Fortune Favors the Prepared: How SMEs Approach Business Model Innovations in Industry 4.0.” Technological Forecasting and Social Change 132: 2–17. doi:10.1016/j.techfore.2017.12.019.
- Müller, J. M., B. Pommeranz, J. Weisser, and K. I. Voigt. 2018b. “Digital, Social Media, and Mobile Marketing in Industrial Buying: Still in Need of Customer Segmentation? Empirical Evidence from Poland and Germany.” Industrial Marketing Management 73: 70–83. doi:10.1016/j.indmarman.2018.01.033.
- Nakata, C., and Z. Zhu. 2010. “Information Technology and Customer Orientation: A Study of Direct, Mediated, and Interactive Linkages.” Journal of Marketing Management 22 (3–4): 319–354. doi:10.1362/026725706776861208.
- Narasimhan, R., and T. Schoenherr. 2013. “Revisiting the Progression of Competitive Capabilities: Results from a Repeated Cross-Sectional Investigation.” International Journal of Production Research 51 (22): 6631–6650. doi:10.1080/00207543.2013.825739.
- Nayernia, H., H. Bahemia, and S. Papagiannidis. 2021. “An Online Community-Based Dynamic Customisation Model: The Trade-off Between Customer Satisfaction and Enterprise Profit.” International Journal of Production Research 59: 1–29. doi:10.1080/00207543.2019.1693649.
- Nevo, S., and M. R. Wade. 2010. “The Formation and Value of IT-Enabled Resources: Antecedents and Consequences of Synergistic Relationships.” MIS Quarterly 34 (1): 163–183. doi:10.2307/20721419.
- Nguyen, H. S., and F. Rondeau. 2019. “The Transmission of Business Cycles: Lessons from the 2004 Enlargement of the EU and the Adoption of the Euro.” Economics of Transition and Institutional Change 27 (3): 729–743. doi:10.1111/ecot.12212.
- Nunally, J. C. 1970. Introduction to Psychological Measurement. McGraw-Hill.
- Nunnally, J. C. 1975. “Psychometric Theory— 25 Years Ago and Now.” Educational Researcher 4 (10): 7–21. doi:10.3102/0013189X004010007.
- Paulk, Mark C. 2009. “A History of the Capability Maturity Model for Software.” ASQ Software Quality Professional 12 (1): 5–19. www.asq.org.
- Paulk, M. C., B. Curtis, M. B. Chrissis, E. L. Averill, J. Bamberger, T. C. Kasse, M. D. Konrad, J. R. Perdue, C. V. Weber, and J. V. Withney. 1991. Capability Maturity Model for Software (CMU/SEI-91-TR-24). Carnegie Mellon University, Software Engineering Institute.
- Paulraj, A. 2011. “Understanding the Relationships Between Internal Resources and Capabilities, Sustainable Supply Management and Organizational Sustainability.” Journal of Supply Chain Management 47 (1): 19–37. doi:10.1111/j.1745-493X.2010.03212.x.
- Péladeau, P., and O. Acker. 2019. Have we reached “peak” chief digital officer? Tech & Innovation, 1–7.
- Piccarozzi, M., B. Aquilani, and C. Gatti. 2018. “Industry 4.0 in Management Studies: A Systematic Literature Review.” Sustainability 10 (10): 3821. doi:10.3390/su10103821.
- Prochniak, M. 2011. “Determinants of Economic Growth in Central and Eastern Europe: The Global Crisis Perspective.” Post-Communist Economies 23 (4): 449–468. doi:10.1080/14631377.2011.622566.
- Proença, D., and J. Borbinha. 2016. “Maturity Models for Information Systems - A State of the Art.” Procedia Computer Science 100: 1042–1049. doi:10.1016/j.procs.2016.09.279.
- Rajnai, Z., and I. Kocsis. 2018. Assessing Industry 4.0 Readiness of Enterprises. SAMI 2018 - IEEE 16th World Symposium on Applied Machine Intelligence and Informatics, 2018-February, 225–230. doi:10.1109/SAMI.2018.8324844.
- Rapaccini, M., N. Saccani, G. Pezzotta, T. Burger, and W. Ganz. 2013. “Service Development in Product-Service Systems: A Maturity Model.” The Service Industries Journal 33: 300–319. doi:10.1080/02642069.2013.747513.
- Remane, G., A. Hanelt, F. Wiesböck, and L. M. Kolbe. 2017. Digital maturity in traditional industries: An exploratory analysis. Twenty-Fifth European Conference on Information Systems (ECIS).
- Ritter, T., and C. L. Pedersen. 2019. “Digitization Capability and the Digitalization of Business Models in Business-to-Business Firms: Past, Present, and Future.” Industrial Marketing Management 11), doi:10.1016/j.indmarman.2019.11.019.
- Rogers, B. 2016. Why 84% of companies fail at digital transformation. Forbes. https://www.forbes.com/sites/brucerogers/2016/01/07/why-84-of-companies-fail-at-digital-transformation/?sh=2b133c38397b.
- Romero, D., L. Larsson, AÖ Rönnbäck, and J. Stahre. 2017. “Strategizing for Production Innovation.” In Advances in Production Management Systems: The Path to Iitelligent, Collaborative and Sustainable Manufacturing, edited by H. Lödding, R. Riedel, K. Thoben, G. von Cieminski, and D. Kiritsis, 3–12. Springer. doi:10.1007/978-3-319-66923-6_1
- Rosenzweig, E. D., and A. V. Roth. 2004. “Towards a Theory of Competitive Progression: Evidence from High-Tech Manufacturing.” Production and Operations Management 13 (4): 354–368. doi:10.1111/j.1937-5956.2004.tb00223.x.
- Rožanec, J. M., J. Lu, J. Rupnik, M. Škrjanc, D. Mladenić, B. Fortuna, X. Zheng, and D. Kiritsis. 2022. “Actionable Cognitive Twins for Decision Making in Manufacturing.” International Journal of Production Research 60 (2): 452–478. doi:10.1080/00207543.2021.2002967.
- Saldanha, T. 2019. Why Digital Transformations Fail: The Surprising Disciplines of How to Take Off and Stay Ahead. Berrett-Koehler Publishers.
- Sandkuhl, K., N. Shilov, and A. Smirnov. 2019. “Facilitating Digital Transformation by Multi-Aspect Ontologies: Approach and Application Steps.” IFAC-PapersOnLine, 1609–1614. doi:10.1016/j.ifacol.2019.11.430.
- Schmenner, R. W., and M. L. Swink. 1998. “On Theory in Operations Management.” Journal of Operations Management 17 (1): 97–113. doi:10.1016/S0272-6963(98)00028-X.
- Schoenherr, T., D. Power, R. Narasimhan, and D. Samson. 2012. “Competitive Capabilities among Manufacturing Plants in Developing, Emerging, and Industrialized Countries: A Comparative Analysis.” Decision Sciences 43 (1): 37–72. doi:10.1111/j.1540-5915.2011.00341.x.
- Schroeder, R. G., R. Shah, and D. Xiaosong Peng. 2011. “The Cumulative Capability ‘Sand Cone’ Model Revisited: A new Perspective for Manufacturing Strategy.” International Journal of Production Research 49 (16): 4879–4901. doi:10.1080/00207543.2010.509116.
- Schumacher, A., S. Erol, and W. Sihn. 2016. “A Maturity Model for Assessing Industry 4.0 Readiness and Maturity of Manufacturing Enterprises.” Procedia CIRP 52: 161–166. doi:10.1016/j.procir.2016.07.040.
- Schwab, K. 2018. The gobal competitiveness report 2018. http://www3.weforum.org/docs/GCR2018/05FullReport/TheGlobalCompetitivenessReport2018.pdf.
- Seddon, P. B. 2014. “Implications for Strategic IS Research of the Resource-Based Theory of the Firm: A Reflection.” The Journal of Strategic Information Systems 23 (4): 257–269. doi:10.1016/j.jsis.2014.11.001.
- Shah, R., and P. T. Ward. 2007. “Defining and Developing Measures of Lean Production.” Journal of Operations Management 25 (4): 785–805. doi:10.1016/j.jom.2007.01.019.
- Simonazzi, A., A. Ginzburg, and G. Nocella. 2013. “Economic Relations Between Germany and Southern Europe.” Cambridge Journal of Economics 37 (3): 653–675. doi:10.1093/cje/bet010.
- Sony, M., and S. Naik. 2019. “Key Ingredients for Evaluating Industry 4.0 Readiness for Organizations: A Literature Review.” In Benchmarking. Emerald Group Publishing Ltd. doi:10.1108/BIJ-09-2018-0284
- Souza das Neves, B., J. Manoel, S. Marins, F. Augusto, and K. Akabane. 2015. “Deployment the MES (Manufacturing Execution System) Aiming to Improve Competitive Priorities of Manufacturing.” Independent Journal of Management & Production 6: 449–463. doi:10.14807/ijmp.v6i2.233.
- Szabo, R. Z., I. Vuksanović Herceg, R. Hanák, L. Hortovanyi, A. Romanová, M. Mocan, and D. Djuričin. 2020. “Industry 4.0 Implementation in B2B Companies: Cross-Country Empirical Evidence on Digital Transformation in the CEE Region.” Sustainability 12 (22): 9538. doi:10.3390/su12229538.
- Tarhan, A., O. Turetken, and H. A. Reijers. 2016. “Business Process Maturity Models: A Systematic Literature Review.” Information and Software Technology 75: 122–134. doi:10.1016/j.infsof.2016.01.010.
- Tortorella, G. L., A. Cawley Vergara, J. A. Mac Garza-Reyes, and R. Sawhney. 2020. “Organizational Learning Paths Based upon Industry 4.0 Adoption: An Empirical Study with Brazilian Manufacturers.” International Journal of Production Economics 219: 284–294. doi:10.1016/j.ijpe.2019.06.023.
- van Hillegersberg, J. 2019. “The Need for a Maturity Model for Maturity Modeling.” In The Art of Structuring, edited by K. Bergener, M. Räckers, and A. Stein, 145–151. Springer International Publishing. doi:10.1007/978-3-030-06234-7_14
- von Leipzig, T., M. Gamp, D. Manz, K. Schöttle, P. Ohlhausen, G. Oosthuizen, and K. von Leipzig. 2017. “Initialising Customer-Orientated Digital Transformation in Enterprises.” Procedia Manufacturing 8: 517–524. doi:10.1016/j.promfg.2017.02.066.
- Wang, T., and Y. Chen. 2018. “Capability Stretching in Product Innovation.” Journal of Management 44 (2): 784–810. doi:10.1177/0149206315594847.
- Warner, K. S. R., and M. Wäger. 2019. “Building Dynamic Capabilities for Digital Transformation: An Ongoing Process of Strategic Renewal.” Long Range Planning 52 (3): 326–349. doi:10.1016/j.lrp.2018.12.001.
- Warning, A., and E. Weber. 2017. Wirtschaft 4.0: Digitalisierungverändert die betriebliche Personalpolitik (12/2017). Institut für Arbeitsmarkt-und Berufsforschung (IAB). http://www.iab.de/stellenerhebung.
- Weidong, X., and L. Gwanhoo. 2014. “Complexity of Information Systems Development Projects: Conceptualization and Measurement Development.” Journal of Management Information Systems 22 (1): 45–83. doi:10.1080/07421222.2003.11045831.
- Weill, P., and S. L. Woerner. 2015. “Optimizing Your Digital Business Model.” IEEE Engineering Management Review 43 (3): 123. doi:10.1109/emr.2015.7059380.
- Winter, S. 2003. “Understanding Dynamic Capabilities.” Strategic Management Journal 24 (10): 991–995. doi:10.1002/smj.318.
- Xu, L. D., E. L. Xu, and L. Li. 2018. “Industry 4.0: State of the art and Future Trends.” International Journal of Production Research 56 (8): 2941–2962. doi:10.1080/00207543.2018.1444806.
- Yadav, M. S., J. C. Prabhu, and R. K. Chandy. 2007. “Managing the Future: Ceo Attention and Innovation Outcomes.” Journal of Marketing 71 (4): 84–101. doi:10.1509/jmkg.71.4.084.
- Yeow, A., C. Soh, and R. Hansen. 2018. “Aligning with new Digital Strategy: A Dynamic Capabilities Approach.” The Journal of Strategic Information Systems 27 (1): 43–58. doi:10.1016/j.jsis.2017.09.001.
- Zhou, L., Z. Jiang, N. Geng, Y. Niu, F. Cui, K. Liu, and N. Qi. 2022. “Production and Operations Management for Intelligent Manufacturing: A Systematic Literature Review.” International Journal of Production Research 60 (2): 808–846. doi:10.1080/00207543.2021.2017055.