Abstract
An efficient multivariate single control chart to simultaneously monitor ‘location or mean’ and ‘scale or variabilities’, based on individual observations of multiple quality characteristics (MQC), is a continuous research endeavour. Compared to rational subgroups, time-ordered individual sample observations of MQC are common in manufacturing. However, few researchers proposed parametric and nonparametric multivariate control charts to monitor individual observations in this context. The proposed parametric approach has limited applicability due to the restrictive distribution assumption of MQC. In addition, most of the suggested parametric or nonparametric approaches recommend multiple charts to monitor process ‘location’ and ‘scale’ parameters simultaneously. Multiple charts can increase the control complexities and overall false-alarm-rate . MQC can also have significant nonlinear correlation structures and follow unknown or nonnormal distributions in real-life scenarios. Single parametric or nonparametric multivariate charts for individual observations can exhibit poor performance in such situations. In this study, an adaptive Mahalanobis depth and k-nearest neighbour (k-NN) rule-based r-AMD chart is proposed and verified to address the lacuna mentioned above. Monte-Carlo simulation studies are used to evaluate the performance of the r-AMD chart. Four real-life manufacturing scenarios are also considered to validate the suitability and superiority of r-AMD over the existing ‘r-MD’ and other competing charts.
Acknowledgement
The authors would like to thank the anonymous learned reviewers and Area Editor for many insightful comments and suggestions, which helped improve the manuscript's quality.
Disclosure statement
No potential conflict of interest was reported by the author(s).
Data availability statement
All case data sets used are published and available in journals (Hajlaoui Citation2010; Hajlaoui Citation2011; Kim et al. Citation2016; Turkoz et al. Citation2019).
Additional information
Notes on contributors
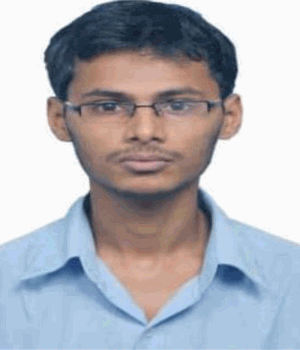
Arijit Maji
Arijit Maji completed his Bachelor's in Power Engineering from National Power Training Institute, Durgapur, India, and his Masters degree in Quality, Reliability & Operations Research from Indian Statistical Institute, Kolkata, India. He is a full-time Institute scholar at Shailesh J. Mehta School of Management, Indian Institute of Technology Bombay, and his primary area of research interest is quality engineering and management.
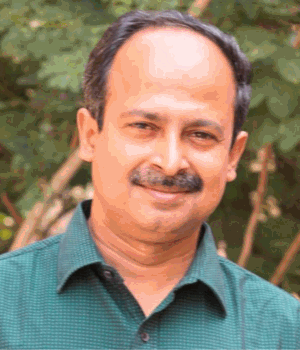
Indrajit Mukherjee
Indrajit Mukherjee is working as a Professor at Shailesh J. Mehta School of Management, IIT Bombay. He did his PhD from the Department of Industrial Engineering and Management, Indian Institute of Technology, Kharagpur. He completed his Masters in Quality, Reliability & Operations Research from Indian Statistical Institute, Kolkata, India. He has authored and co-authored several research papers published in reputed journals. His research contributions are reported in the International Journal of Production Research, European Journal of Operations Research, Journal of Manufacturing Systems, Quality and Reliability Management, Quality Engineering, and Computers & Industrial Engineering. His area of research interest is quality engineering and management.