Abstract
This work extends the energy-efficient job shop scheduling problem with transport resources by considering speed adjustable resources of two types, namely: the machines where the jobs are processed on and the vehicles that transport the jobs around the shop-floor. Therefore, the problem being considered involves determining, simultaneously, the processing speed of each production operation, the sequence of the production operations for each machine, the allocation of the transport tasks to vehicles, the travelling speed of each task for the empty and for the loaded legs, and the sequence of the transport tasks for each vehicle. Among the possible solutions, we are interested in those providing trade-offs between makespan and total energy consumption (Pareto solutions). To that end, we develop and solve a bi-objective mixed-integer linear programming model. In addition, due to problem complexity we also propose a multi-objective biased random key genetic algorithm that simultaneously evolves several populations. The computational experiments performed have show it to be effective and efficient, even in the presence of larger problem instances. Finally, we provide extensive time and energy trade-off analysis (Pareto front) to infer the advantages of considering speed adjustable machines and speed adjustable vehicles and provide general insights for the managers dealing with such a complex problem.
Data availability statement
The data that support the findings of this study are openly available at https://fastmanufacturingproject.wordpress.com/problem-instances.
Disclosure statement
No potential conflict of interest was reported by the author(s).
Notes
1 Some authors consider that the jobs are initially located at the machines where their first operation is processed on.
2 In the presence of a non-sequence dependent set-up time, we simply add the set-up time to the operation processing time.
3 All data pertaining to the problem instances proposed in Yin et al. (Citation2017) and in Salido et al. (Citation2016) as well as to the adapted instances can be downloaded from https://fastmanufacturingproject.wordpress.com/problem-instances.
Additional information
Funding
Notes on contributors
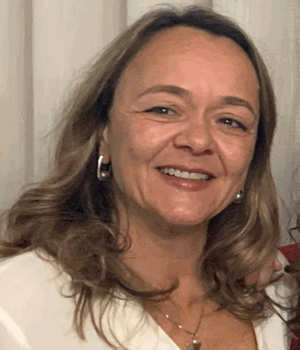
Dalila B.M.M. Fontes
Dalila B. M. M. Fontes an Associate Professor in Operations and Logistics, at the Faculdade de Economia (FEP), Universidade do Porto and a researcher and board member of LIAAD, Laboratório de Inteligência Artificial e de Apoio à Decisão, a unit of INESC TEC. She holds a Ph.D. from the Imperial College of Science Technology and Medicine and a Master from The London School of Economics and Political Sciences. Dalila research focus is on developing a comprehensive understanding of complex problems in operations management, supported by novel and sound models and solution methods. She is an associate editor of the Journal of Combinatorial Optimization and the Operations Research Forum and a member of the several editorial boards. Her research interests are on the areas of Operations and Supply Chain Management where she has been exploring the optimisation of global systems accounting for economic and environmental concerns. The solution methods developed include linear programming, dynamic programming, genetic algorithms, particle swarm optimisation, simulated annealing, among others She has published several papers and one book in these areas and has been coordinating several scientific projects.
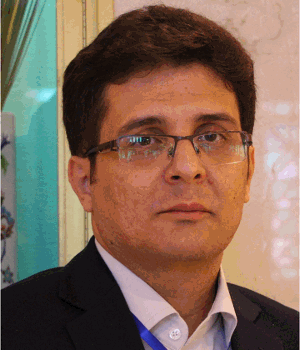
Seyed Mahdi Homayouni
S. Mahdi Homayouni is a Researcher at the Centre for Enterprise Systems Engineering (CESE), INESC TEC, Porto, Portugal, and an invited Assistant Professor in Operations Management at the Faculdade de Economia (FEP), Universidade do Porto. He has been an Assistant Professor at the Department of Industrial Engineering, Islamic Azad University, Lenjan Branch, Isfahan, Iran from Feb 2011 to January 2017. He holds a Ph.D. and a Master's in industrial and Systems Engineering, from Universiti Putra Malaysia and a Bachelor of Engineering in Industrial Production Engineering from Azad University of Najafabad, Iran. Mahdi's research focus is on developing exact and heuristic solutions approaches for the supply chain and operations management problems, particularly, operations planning and scheduling problems in advanced manufacturing systems and seaport container terminals, promoting sustainability objectives.
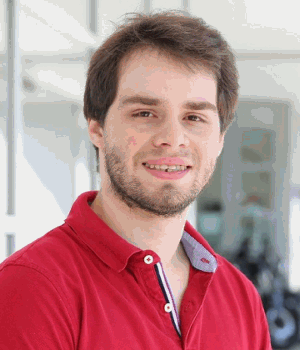
João Chaves Fernandes
João Fernandes is a researcher at LIAAD and Ph.D. student at FEUP, currently attending the Doctoral Program in Engineering and Industrial Management. His research interests are Operations Research, Metaheuristics, Predictive Analytics and Machine Learning. He is currently researching Operations Scheduling Problems, tackling Energy-Efficiency in Job Shop Scheduling Problems. João has a MsC in Industrial Engineering and Management (FEUP). He also has two years of professional experience in Data Science, having previously worked at Glintt and NOS Comunicações.