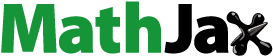
Abstract
Robotisation is increasing in warehouse operations, but human employment continues to be relevant. Traditionally manual activities, such as order picking, are being re-designed into collaborative human–robot tasks. This trend exemplifies the transition towards a human-centric Industry 5.0, focusing on synergy instead of seeking human replacement. However, human workers are increasingly hard to recruit and retain. We contribute to the underrepresented literature on human factors within the domain of operations and production management research and investigate the deployment of robotic technologies alongside human workers in a sustainable way. With a unique real-effort experiment, we investigate how the manipulation of picker’s experienced levels of autonomy affects their job satisfaction and core self-evaluations, two key behavioural outcomes that determine employee turnover intentions. We establish that the introduction of human–robot collaboration positively affects job satisfaction for the contrasting collaboration dynamics of (i) gaining control (the human leading the robot) and (ii) ceding control (the human following the robot). This positive effect is larger when the human is following the robot. We additionally find that following the robot positively affects pickers’ self-esteem and that self-efficacy related to human–robot interaction benefits from the introduction of collaborative robotics, regardless of the setup dynamics.
1. Introduction
In warehouses, the robots are coming, but the humans are here to stay. By deploying humans to complement robot labour or by using robots to supplement human labour, humans still play a key role in warehouse operations (Azadeh, De Koster, and Roy Citation2019; Ben-Ari and Mondada Citation2018; Fragapane et al. Citation2021). The size of the warehouse robotics automation industry has been growing by 12% on average per year from 2014 through 2019 (The Logistics iQ Citation2020). Adding more automation in a warehouse might not always be feasible. Important warehousing tasks such as order picking cannot be easily standardised or automated, due to the common plethora of products, each with different handling, stacking, and processing requirements (Azadeh, De Koster, and Roy Citation2019). Tasks like order picking typically require the flexibility and ability for customisation that human labourers provide, necessitating them to be involved in at least a part of the task. In those situations where human labour is still needed in increasingly robotised warehouses, humans are deployed to complement robot labour (Financial Times Citation2021). Such purposely designed robots, or ‘cobots’ are intended to ‘combine the repetitive performance of robots with the individual skills and ability of people’ (International Organization for Standardization Citation2016). As such, many warehouses are becoming part-manual, part-robot, thus forming systems of collaboration.
Consequently, while robots rush the warehouses in greater numbers, warehousing job vacancies for human workers are rising just as fast, with an example of 13.6% growth on average year-after-year in the US (The Wall Street Journal Citation2021). This growth was spurred by the increased emphasis on e-commerce during the Covid-19 pandemic. According to a salary data report ranking career trends across 15,000 + job titles posted on LinkedIn, warehouse workers on the frontlines of e-commerce became the most in-demand employees in 2020 (Seaman Citation2021). Many warehouses that struggled to meet surging online demand while facing a tight labour market, turned to robots that can work alongside humans and complement their performance (The Wall Street Journal Citation2021). In those situations, robots are used to supplement human labour and augment it by alleviating time-consuming and repetitive tasks such as transporting finished orders to a depot.
However, the way the work is divided between robots and humans in such collaborative systems may have an impact on dehumanisation and intensification of work which may plummet job satisfaction and ultimately incite employee dropout rates in semi-robotised warehouses (Financial Times Citation2021; The Guardian Citation2020). Such increased dropout rates in semi-robotised warehouses may intensify the existing problem of high warehouse worker turnover. Specifically, the demand for human workers in warehouses dwarves five-fold the growth in low-skilled warehousing jobs. This indicates a high degree of turnover. In the US, it has concurrently grown at a non-sustainable rate of 73% year-over-year (Seaman Citation2021). In one extreme case, Amazon has been accused of ‘burning through’ workers at turnover rates of even 150% per year, effectively replacing its entire warehouse workforce every eight months (New York Times Citation2021). Companies that do not actively maintain satisfied employees might simply find no more employees to work in their new collaborative robotics setups.
The potential pitfalls emerging from the novel context posed by human–robot collaboration are recognised by researchers and governments alike. Recent calls for research argue for the crucial importance of understanding the yet unknown implications of the human factor in production systems that deploy humans alongside robotic technologies (Donohue, Özer, and Zheng Citation2020; Olsen and Tomlin Citation2020). Even though high-level frameworks might be available for assessing how changes of work characteristics affect the job satisfaction of employees in an increasingly robotised environment, collaborative robotic systems are a novice and largely unexplored research opportunity (Winkelhaus, Grosse, and Glock Citation2022). Also on a policy level, this issue is recognised. In its vision for Industry 5.0, the European Commission has also called for a human-centric approach to technology-driven progress. Such an approach is necessary to establish resilient future prosperity by promoting considerations that include autonomy, human dignity, job satisfaction, and mental well-being of workers collaborating with robots (Breque, De Nul, and Petrides Citation2021; European Commission Citation2021).
This study investigates the immediate effects of imposing a human–robot collaboration (order picker with robots), as a proxy to understand the longer-term effects of such collaboration on the picker’s key behavioural outcomes that are related to employee turnover. We consider job satisfaction as a critical factor that is known to relate to employee turnover (Griffeth, Hom, and Gaertner Citation2000), and core self-evaluations as constructs further associated with job satisfaction (Judge et al. Citation1998, Citation2000, Citation2005; Judge and Bono Citation2001) and hence employee turnover. We, therefore, investigate the following questions: (1) How does the collaboration with robots affect the order picker’s job satisfaction? (2) How does the introduction of collaboration with robots affect the order picker’s core self-evaluations (in terms of self-esteem and self-efficacy)? (3) How does the introduction of collaborative robotics affect a picker’s self-efficacy in human–robot interaction? We contribute to the literature of operations and production management research in the following ways. Firstly, we propose job satisfaction and core self-evaluations as metrics for assessing the long-term success of human and robot collaborative systems. Secondly, we establish that the introduction of robots affects the picker’s job satisfaction, his or her core self-evaluations (self-esteem, self-efficacy), and the new context-specific self-evaluation trait of self-efficacy in human–robot interaction. Thirdly, we investigate these effects under different human–robot collaborative order picking systems, namely, with the human leading and the human following the robot. The results of this study will enable companies that introduce collaborative robotic solutions in warehouses to identify the desirable system design characteristics that relate to lower labour turnover, and hence sustainable long-term success.
2. Collaborative picking systems and related literature
2.1. Picker to parts human–robot collaborative order picking
Human–robot collaborative order picking systems are being implemented across warehouses as an upgrade of existing systems. In picker-to-parts order picking systems, human workers travel with a pick cart to retrieve items from different warehouse locations, to drop them off at a depot station. We may consider these manual setups as the status quo, or the ‘base’ system in which collaborative robotics are introduced. As such, robots can be used to replace the lifeless pick cart by an intelligent agent. This leads to higher utilisation of the human workers: workers can continuously focus on the more ‘complex’ and less standardised tasks of identifying and picking items to fulfil specific customer orders, while the robots take over more ‘simple’ and tedious tasks, e.g. travelling to the depot (Azadeh, De Koster, and Roy Citation2019; Gutelius and Theodore Citation2019).
To optimise the performance of the system, also the interaction between humans and robots is important. Within such systems, robots can be designed to have either a leading or a supportive role. Pickers then undertake the complementary supportive or leading role, respectively (Azadeh, De Koster, and Roy Citation2019). Humans can lead the robots (i.e. the robots follow the order picker, e.g. Effidence Citation2016; ToyotaMHEurope Citation2011), they can support the robot (by following and being guided by the robot, e.g. 6 River Systems Citation2019), or work in a hybrid setup in which these roles alternate (e.g. Locus Robotics Autonomous Robots Citation2020).
The different literature streams use similar terms to classify these human–robot interaction dynamics. The stream of literature on human–robot interaction taxonomises these relationships according to the autonomy levels of the agents: the extent to which the robot’s actions are determined by the human, and the extent to which the human’s actions are determined by the robotic partner. Robots are therefore characterised as ‘active’ and ‘non-active’ (or ‘passive’), depending on the degree of autonomy that the robot exhibits (Bruemmer et al. Citation2005; Yanco and Drury Citation2004).
Consequently, the dynamics between the collaborating partners result in introducing a leader-follower relationship between humans and robots. As such, each partner’s behaviour can be classified as inactive (resting), active (leading), supportive (following), and adaptive (alternating) along a continuum from inactivity to full autonomy (L. Wang et al. Citation2020; X. Wang et al. Citation2017). However, these relationships have behavioural implications, which have only scarcely been investigated. Individual differences and human factors impacting order picking behaviour have been found in manual (e.g. De Vries, De Koster, and Stam Citation2016a; Grosse, Glock, and Neumann Citation2017), as well as in technology-assisted settings (e.g. De Vries, De Koster, and Stam Citation2016b). The literature on introducing human–robot collaborative settings in order picking is mostly limited to simulated settings (e.g. Azadeh, Roy, and De Koster Citation2020; Inam et al. Citation2018; Petković et al. Citation2019; Z. Wang et al. Citation2022) and subject to non-empirically validated assumptions. Nonetheless, in their study, Pasparakis, De Vries, and De Koster (Citation2021) investigated the effects of leader-follower human–robot relationships but focused only on performance metrics (productivity, accuracy) of different collaborative settings, and not on the effect that the collaboration introduction has on the behavioural outcome metrics of the subjects. In order to minimise employee turnover, the desire of human team members to work with their robotic partners in the long term should be maximised. We, therefore, further investigate the effect of introducing human–robot collaboration on the picker’s job satisfaction and core self-evaluation.
2.2. Job satisfaction
The classical perspective of job satisfaction in Human Resource Management is offered by Locke in his two influential papers on this subject. In these publications, Locke suggested that job satisfaction is a ‘pleasurable emotional state’, resulting from appraisal related to the achievement or facilitation of one’s job values (Locke Citation1969) or appraisal of one’s job or job experiences (Locke Citation1976). The consensus on the definition of job satisfaction was later outlined by Cranny, Smith, and Stone (Citation1992), with a definition of job satisfaction as ‘an affective (that is, emotional) reaction to one’s job, resulting from the incumbent’s comparison of actual outcomes with those that are desired (expected, deserved, and so on.)’ (1). However, even though the relationship between job satisfaction and job performance is arguably the ‘Holy Grail’ of Human Resource Management research, the copious amount of literature on the topic has failed to converge to a similar clear consensus (Weiss and Cropanzano Citation1996). Nonetheless, overall job satisfaction appears to be the best predictor in employee turnover, as summarised in a comprehensive meta-analysis of turnover antecedents by Griffeth, Hom, and Gaertner (Citation2000). As such, job satisfaction is a relevant success metric in the retention of warehouse employees.
Furthermore, as recent studies suggest, in order to assess the effect that the introduction of more automation and robotisation in intralogistics jobs has on worker job satisfaction, one needs to consider the specific system design characteristics (Winkelhaus, Grosse, and Glock Citation2022). Nevertheless, the traditional perspective that job satisfaction is an affective reaction to one’s job still serves as a guidance for investigating which changes in occupational characteristics might have an impact on the employee’s job satisfaction as a response. Specifically, in the focal area of autonomy, Spector (Citation1986) found in a meta-analysis across 88 studies that high levels of perceived control and autonomy are associated with high levels of job satisfaction. In the area of human–robot collaboration, the introduction of an autonomous robotic partner introduces control dynamics, as each partner has some level of autonomy. We can denote the level of perceived control in manual order picking as ‘base’ level, since the picker in a manual system is not dealing with control dynamics in relation with a lifeless cart. In the case of human leading the robot, the element that is controlled (intelligent machine) has some level of autonomy, over which the picker exerts power. Therefore, the picker’s level of control is increased when leading the robot. Similarly, by accepting the guidance of the robot when following, the picker gives up his control to the autonomous machine. Therefore, in general, the worker gains control when leading the robot and cedes control when following the robot. We hypothesise that:
H1a: Collaborative order picking with the Human leading the robot results in higher Job Satisfaction, compared to manual order picking.
H1b: Collaborative order picking with the Human following the robot results in lower Job Satisfaction, compared to manual order picking.
2.3. Core self-evaluations
The concept of core self-evaluations was introduced by Judge et al. (Citation1998), as the ‘fundamental assessments that people make about their worthiness, competence, and capabilities’ (257). This latent construct was originally comprised (among others) of individual difference constructs such as self-esteem and self-efficacy and has been related to several positive job-related outcomes such as job performance (Kacmar et al. Citation2009), motivation (Erez and Judge Citation2001), and coping processes (Kammeyer-Mueller, Judge, and Scott Citation2009). Most commonly, core self-evaluations have been positively linked to job satisfaction (Judge et al. Citation1998, Citation2000, Citation2005; Judge and Bono Citation2001). As such, positive effects on core self-evaluations are likely to result in higher job satisfaction, which may ultimately lead to lower turnover intentions (Griffeth, Hom, and Gaertner Citation2000), as outlined earlier.
Furthermore, the occupational experience of autonomy has been demonstrated to strongly influence core self-evaluation. This claim is largely supported for both the constructs self-esteem and self-efficacy. The early literature on work autonomy and self-esteem (as comprehensively summarised by Schwalbe Citation1985) suggests that autonomy at work is systematically positively related to self-esteem. Finally, work autonomy has been shown to impact self-esteem (viewed as psychological resource) as a result of an affective response (Xanthopoulou et al. Citation2009, Citation2012). Assuming that the level of perceived control is ‘base’ in manual order picking, then self-esteem should increase when workers are gaining control and lead the robots and decrease when workers cede control and follow the robots. Therefore, we hypothesise that:
H2a: Collaborative order picking with the Human leading the robot results in higher picker Self-Esteem, compared to manual order picking.
H2b: Collaborative order picking with Human following the robot results in lower picker Self-Esteem, compared to manual order picking.
H3a: Collaborative order picking with Human leading the robot results in higher picker Self-Efficacy, compared to manual order picking.
H3b: Collaborative order picking with Human following the robot results in lower picker Self-Efficacy, compared to manual order picking.
2.3.1. Self-efficacy in human–robot interaction
Not included in the original constructs of core self-evaluations, but increasingly important in a fast-changing world, is a construct for individual self-assessment as related to technology. Self-efficacy perceptions with regard to specific technologies are a crucial factor dictating the acceptance and the adoption of (new) technologies, and therefore affecting labour turnover. Recent examples of this context-specific self-evaluation, include self-efficacy perceptions in computers (Compeau, Higgins, and Huff Citation1999; Compeau and Higgins Citation1995), the Internet (Hsu and Chiu Citation2004; Torkzadeh and van Dyke Citation2001), and finally smartphones (Chen, Chen, and Yen Citation2011). Self-Efficacy in Human–Robot Interaction, as an extension of the self-efficacy construct is a scarcely investigated topic, with the exception of Pütten and Bock (Citation2018), who developed and validated a methodology for the evaluation of individuals’ beliefs regarding their abilities to interact with robotic technologies. The construct and corresponding measurement scale was deployed in their follow-up research (Rosenthal-Von Der Pütten, Bock, and Brockmann Citation2017), to successfully provide evidence that the simple process of interacting with a robot can increase the perceived self-efficacy in human–robot interaction, as well as the evaluation of the robot interaction, regardless of design specifications and availability for customisation. By definition, self-efficacy can be positively influenced by enactive attainment (Bandura Citation1986). We hypothesise that the introduction of collaborative robotics will have a positive effect on the picker’s self-efficacy in human–robot interaction, regardless of the specific system settings (and thus levels of autonomy).
H4a: The introduction of collaborative order picking with the Human leading the robot results in higher picker Self-Efficacy in Human–Robot interaction.
H4b: The introduction of collaborative order picking with the Human following the robot results in higher picker Self-Efficacy in Human–Robot interaction.
3. Methodology
3.1. Participants and research setting
We designed and executed a real-effort controlled experiment with 60 participants, to test our hypotheses and investigate the effects of introducing human–robot collaborative order picking on the picker’s job satisfaction and core self-evaluations as proxies for employee turnover. We used a within-subjects design to better control for individual extraneous variables and hence detect differences in effects across treatments. All participants were Dutch students of a vocational shipping and transport college in the Netherlands, being trained to work in logistics operations. The decision to use this sample was inspired by previous studies that demonstrated that these individuals are more appropriate subjects for physical order picking experiments than the commonly employed sample of university students (De Vries, De Koster, and Stam Citation2016b). The participants were randomly selected from the pool of interested senior students (Age, M = 18.0 years, SD = 1.3 years), and the sample ratio of female to male recruited students (10:50) was consistent with the school’s student population. The participants were motivated to participate and to perform with a combination of rewards: (i) a training certificate of experience in human–robot collaborative order picking, (ii) a flat monetary reward for participation, and (iii) an additional monetary bonus to be awarded to the top three performers among the participants. The rewards system was developed to realistically replicate common warehouse worker reward schemes, in which employees gain experience, a fixed salary, and potential bonuses according to their performance.
The experiment took place in a dedicated warehouse lab, specifically erected for the purposes of this research to resemble a typical shelf warehouse used for storing small items in small quantities. The experimental warehouse consisted of 25 professional metal racks (dimensions 1200 × 500 × 2100 mm, w × d × h), each of which divided into four vertical levels and three horizontal levels and arrayed along two and a half aisles (Figure ). This amounted to 300 picking locations, each uniquely codified with the aisle-section-level-position identity in natural progression. At each pick location, a unique household product was stored, ranging from 0.1 to 5 l in volume, with a weight between 10 and 2000 g, and with enough stock to allow for uninterrupted picking during the order picking tasks.
3.2. Manipulation and experimental procedure
The participants were subjected to the following manipulation: (a) first become familiar with manual order picking and perform a manual order picking task using a pick trolley (Phase 1) and (b) subsequently get introduced to human–robot collaborative order picking and perform the tasks in collaboration with autonomous mobile robots (Phase 2). In order to maintain a within-subjects design, both setups of human–robot collaborative order picking were performed by all participants and at random sequence (potential spill-over effects were investigated in the analysis). For each task, the participants received video training in Dutch, followed by live demonstration and a practice round of fulfilling four example orders. After completing each training round, the participants were deployed to perform a 20-minute run of order picking with their performance closely monitored in terms of speed and accuracy (outcome metric: correct pick lines per 20 min). This present experiment was conducted as part of a broader set of experiments conducted in the warehouse lab. The dynamics of the objectively measured outcomes were investigated in previous research and do not overlap with the manipulation, nor affect any subjective/perceptual outcomes of the current study. We do not reuse any data, and all the monitored psychometric data were collected solely for use in the present study. Finally, after each task, the participants were requested to fill in questionnaires that captured their reaction to their emotional state and perception over the performed task. Videos and questionnaires were administered in Dutch, the native language of all participants. The participant’s experimental journey is depicted and summarised in Figure , and the setups across the two phases of the manipulation are depicted in Figure . The total set of experiments took about two and a half hours per participant, with additional thirty minutes set up costs for the experimenters.
Figure 3. The participant's experimental journey.
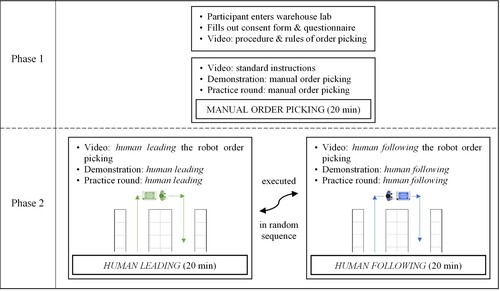
Figure 4. Left: Manual order picking with cart (Phase 1). Middle and Right: human–robot collaborative order picking (Phase 2; human leading and robot following, human following and robot leading).
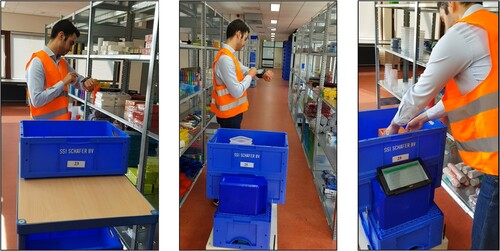
3.3. Order picking tasks
The order picking tasks that the participants were requested to perform, consisted of four steps: (1) Identify the next requested product (order line) on the available order list as presented to them on a screen. (2) Visit the product location in the warehouse. (3) Pick the requested number of units and put them in the order crate. (4) Confirm the completed order line with an appropriate tap on the screen, and proceed with the next order line. When all available order lines were picked and hence the order at hand was completed, the participants began picking the next order which was directly given to them. The orders were inexhaustible and consisted of 5–10 order lines (5: 40%, 6: 25%, 7: 15%, 8: 10%, 9: 5%, 10: 5%). The orders were generated to randomly request an item from several of the 300 pick locations, with the constraint of requesting at least one order per aisle in sequence. As such, the aisles were visited for orders with odd numbers in A▸B▸C sequence (Figure ) and for orders with even numbers in C▸B▸A sequence. This constraint was introduced to force participants to use a pre-defined S-shaped route traversing the entire warehouse to fulfil each order, and hence standardise the requirements for walking. Three such order sets were generated and randomly assigned to each picking round, so that each participant picked each order set once.
The manual order picking task was performed using a pick trolley that carried two order crates. The pickers sequentially fulfilled two orders at a time, and, therefore, traversed the entire warehouse and returned to the depot station (A▸B▸C▸B▸A) where they were given empty crates to continue their order picking task. As such, no additional time was spent in unnecessary travelling. The order lists were presented to the picker via a small touch screen that was fitted on the subject’s non-dominant arm. For the human–robot collaborative tasks two autonomous guided vehicles were deployed. One was assigned to carry the odd numbered orders. The other to carry the even numbered orders, so that the pickers were always finding an available robot waiting for them at the end of the route where they finished their previous order (Figure ). For the human leading the robot setup, the same screen was given to the picker, but now the robot followed the picker while carrying the order crate. For the human following the robot setup, a larger screen was fitted on the robot (to account for the greater viewing distance) and the subjects were instructed to follow the robot as it guides them to the next picking location. In both collaborative setups, when each order was finished, the robot would go to the depot station and the picker would continue working uninterrupted with the other robot. The collaboration was (i) framed as having leader-follower dynamics and (ii) physically enforced by having the human lead or follow the robot, respectively. Nonetheless, the setups were more similar than the participants were led to believe, as the robots always performed the same pre-programmed movements defined by the experimenters. With the robot characteristics, movements, and speed identical for each pre-defined order sets, all observed subjective outcomes are a result of variation in human perception and reaction. This ‘Wizard of Oz’ illusionary effect was not disclosed to the participants, nor was there any indication that it was ever discovered.
3.4. Dependent variables
For operationalising the dependent/outcome variables we used the original versions of the questionnaires, and minimally adapted them to our setting as outlined below. We measured Job Satisfaction using a five-question questionnaire adapted from the job diagnostic survey – Job satisfaction (Hackman and Oldham Citation1974, 5-point Likert). The questions were adjusted to measure the picker’s affective response ‘related to the task they just completed’. We measured Self-Esteem with the original 10-question Rosenberg Self-Esteem scale (Rosenberg Citation1965, 4-point Likert), and Self-Efficacy with the original ten-question General Self-Efficacy Scale (GSE) (Schwarzer and Jerusalem Citation1995, 4-point Likert). Finally, we measured Self-Efficacy in HRI through the 18-question Human–Robot Interaction Scale (Pütten and Bock Citation2018, 6-point Likert scale).
3.4. Independent variables
To explain part of the variance in our models, we use several control variables that are expected to be important predictors of the dependent variables. Firstly, we consider the number of correct order lines picked during each round as the level of Competence of the pickers (correct picks per 20 min). Only the direct effect of competence was included and reported in the final models, as interaction terms between competence and setups did not improve the fit of the models. In addition, we initially controlled for the sequence of the collaborative setups to account for any spill over effects of learning of fatigue but excluded this from the reported models as they did not yield statistically significant model improvements (West, Welch, and Galecki Citation2014). We also control for the different order sets that were used in each pick round, to capture differences due to variety in the pick lists. Moreover, we control for the Perceived Usefulness (PUsf) and Perceived Ease of Use (PEoU) of the collaborative setups, two constructs we borrowed from the literature of Acceptance and Use of Technology (Venkatesh, Thong, and Xu Citation2016). To measure these constructs, we used the eight-question, 5-point Likert adapted versions by Venkatesh, Thong, and Xu (Citation2016), of the scales introduced by Davis (Citation1989). PUsf and PEoU have been linked to user acceptance of technological solutions, and we, therefore, used them to capture the user intentions to work with the robots regardless of the system design. These two variables were measured for both scenarios of the human leading the robot (PUsf, M = 3.68, SD = 0.96; PEoU, M = 3.68, SD = 0.76), and the human following the robot (PUsf, M = 3.84, SD = 0.91; PEoU, M = 3.81, SD = 0.65). As such, we initially confirm that the two robotic systems were perceived comparably equally by the user (no significant difference between the two), and we subsequently investigate their average effect as an element to be considered in the design goals for collaborative robotics systems. Lastly, we captured the age, sex, education level, and order picking experience of the participants. Order picking experience measured in months (M = 2.12, SD = 5.40) was the only variable that was included in the final reported models, due to limited variance across subjects on age and education level, and the non-significant effect found for sex.
4. Results
4.1. Descriptive statistics
First, we report the descriptive statistics that were calculated to provide the overall magnitude of the monitored outcome variables. Table shows means, standard deviations and timing of measurements. The measurements were conducted before and after the order picking task, following the methodology and using the scales described in Sections 3.4 and 3.5.
Table 1. Descriptive statistics (mean, standard deviation) and measurement timing of outcome variables.
4.2. Main results
To control for the effect of individual pickers over the experiments, we use the package ‘lme4’ (Bates et al. Citation2015) in R version 4.0.3 (R Core Team Citation2020) to fit linear mixed-effects models to our collected data. These models are used to predict job satisfaction [M1] and the extended core self-evaluation [M2-4] traits in the transition from manual order picking to human–robot collaborative order picking. The models use manual order picking as the base scenario. Consequently, the results of the two setups of human leading the robot and human following the robot are relative to manual order picking (in line with the hypotheses). All 60 participants completed all 3 rounds and were assigned at random to one of two sequences: Manual ▸ Human leading & Robot leading, or Manual ▸ Robot leading & Human leading. As such, the data were nested in two dimensions, per participant and per sequence. To model this internal structure, we introduced a random intercept to account for the effects of random subject due to the nesting of the data at picker level. The resulting models to be estimated follow Equation (1):
(1)
(1) where
is the corresponding outcome variable in the models [M1-4],
is the setup {Manual, Human leading, Human Following},
are the individuals,
is the number of predictors,
is the random error per individual, and
is the independent error across all observations.
Table presents the results of the final linear mixed-effects models for the four calculated models fitted using the restricted maximum likelihood ratio parameter estimation criterion (Wu et al. Citation2017). Variables were sequentially inserted in the models, assessing the improvement of model fit using ANOVA tests (West, Welch, and Galecki Citation2014). Only the final models were reported in the manuscript due to length limitations, but relationships were found to be analogous at each step. Other variables and interactions omitted in preliminary stages are mentioned in Section 3.5. Model M1 shows that collaborative order picking with the human leading the robot results in higher job satisfaction compared to manual order picking, supporting hypothesis H1a. Additionally, the model provides evidence that collaborative order picking with the human following the robot results in higher job satisfaction compared to manual order picking, contrary to our hypothesis H1b. Post hoc analysis additionally suggests that the positive effect of collaborative order picking on job satisfaction (compared to manual order picking) is larger in the case of human following the robot compared to human leading the robot. We further find that both competence and perceived usefulness of the collaborative setups positively affect job satisfaction. Model M2 provides no support for hypothesis H2a that collaborative order picking with the human leading the robot results in higher job satisfaction compared to manual order picking. It does provide evidence, however, that collaborative order picking with human following the robot results in higher picker self-esteem compared to manual order picking, in contrast with our hypothesis H2b. Additionally, it is evident that a large part of self-esteem is stable in the transition from manual to collaborative order picking, as indicated by the large effect of the model’s intercept. Model M3 provides no supporting evidence for either hypothesis H3a or H3b, suggesting that picker’s self-efficacy is not directly affected by the introduction of collaborative order picking. As with self-esteem, the largest part of self-efficacy appears to not be sensitive in the transition, as indicated by the large effect of the intercept. Perceived ease of use positively affects self-efficacy. Model M4 provides evidence to support both hypotheses H4a and H4b, asserting that the introduction of collaborative order picking results in higher picker self-efficacy in HRI, in both setups of the human leading the robot and the human following the robot (post hoc: with similar effect). Additionally, the model provides evidence that perceived ease of use positively affects self-efficacy in HRI.
Table 2. Linear mixed-effects models predicting Job Satisfaction, Self-Esteem, Self-Efficacy, Self-Efficacy in Human–Robot interaction.
The hypothesis testing results are summarised in Figure .
5. Conclusion and discussion
This study contributes to the existing literature of operations and production management research by establishing the effects of the introduction of collaborative human–robot order picking on picker job satisfaction and core self-evaluation traits. We argue that the introduction of a robotic partner leads to changes in the picker’s experienced levels of occupational autonomy due to the imposed control dynamics, which subsequently affect these key behavioural outcomes that are critical determinants of employee turnover intentions (Griffeth, Hom, and Gaertner Citation2000).
5.1. Implications for theory
We find that the introduction of collaborative robotics increases the job satisfaction of pickers in both collaborative setup dynamics of the human leading the robot and human following the robot (M1). We observed a greater positive effect when the humans are guided by the robots (M1; post-hoc). As pickers physically experience more control over the robotic partner, we find that they are indeed more satisfied with the task at hand (H1a supported by M1), which is in line with previous studies that connect autonomy and control with job satisfaction (Spector Citation1986). However, we find additional evidence suggesting that even when the pickers cede control to the robot, their job satisfaction levels are positively affected (M1 contradicting H1b). The theoretical considerations ensuing from this finding indicate that there might be additional benefits from ceding control to the robots, which outweigh the expected dissatisfaction associated with ceding autonomy to the machine. An example of such a confounding effect might be an increase in occupational satisfaction due to the reduced complexity of the order picking task. Indeed, by their guidance within the warehouse, as well as by their mere physical indication of the correct pick location, the robots aid the pickers to confidently identify pick locations and therefore reduce the mental load as well as the potential to commit mistakes. Another example would be that the robots externally introduce a pace for the pickers at which they can comfortably keep a steady rhythm in their task. Finally, it is possible that these considerations are especially true for blue-collar workers who may disproportionately appreciate more clear and less complex tasks, as in the case of following a robot compared to leading a robot or doing the task manually.
Furthermore, we establish that the traditional core self-evaluation traits of self-esteem and self-efficacy are largely insensitive to the introduction of human–robot collaborative order picking tasks (as shown by the large effect of the constant term in M2 & M3 which is independent of the manipulation). Nonetheless, they are still affected by the introduction of human–robot collaborative order picking. Indeed, we find evidence, which suggests that self-esteem is positively affected by the introduction of a collaborative setup where the human is following the robot (M2 contradicting H2b). We attribute this finding to the potentially increased feeling of self-worth by the picker, as a result of supporting the robots. In this case, the pickers may feel that their involvement is critical for the success of such the system, and realise their importance and relevance for the long-term success of similar warehouse operations.
Other findings (H4a and H4b supported by M4) provide evidence that even the short introduction and training of human–robot collaborative order picking to workers may have an immediate impact on their self-efficacy in human–robot interaction. This niche sub-concept of self-efficacy in relation with robot interaction, which we utilised as an extension of the original Self Core Evaluations, may be an important consideration for a future human-centric industry with a high prevalence of robotic solutions (Rosenthal-Von Der Pütten, Bock, and Brockmann Citation2017).
5.2. Implications for management practice
The immediate managerial implication emanating from these theoretical implications and understanding, is that the introduction of human–robot collaborative systems may be overall beneficial for worker job satisfaction and core self-evaluations, and, as a consequence, for worker retention and long-term success of the systems (Griffeth, Hom, and Gaertner Citation2000). Job satisfaction benefits from the collaboration with robots, regardless of the setup details and the loss or gain of autonomy and control (M1). Larger benefits for job satisfaction may come as a result of introducing systems where the robots lead the human workers (M1 post hoc). Worker self-esteem may also benefit from this setup (M2). Self-efficacy in human–robot interaction benefits equally from any of the setups that were considered (M4).
The effects of the constructs under the Acceptance and Use of Technology models (Venkatesh, Thong, and Xu Citation2016) might also be of interest from a practical perspective. Findings on the perceived usefulness of the human–robot collaborative systems suggest that it is an important contributor to the overall job satisfaction of the pickers (M1), with the picker’s satisfaction being linked to the extent to which they perceive the robot to be adding value in the collaborative task. This means that human workers may experience lower job satisfaction when forced to work with robots that they do not perceive as useful for the task at hand. It might therefore be considered that managers need to make the added value of the robotic setup salient for the human workers during the collaborative part of their job, and not only rely on the part where the robots behave independently from the humans (e.g. transporting crates). Final findings on the perceived ease of use suggest that pickers collaborating with robots receive a boost in self-efficacy, both as a core self-evaluation trait (M3) as well as in relation to human–robot interaction (M4).
5.3. Strengths, limitations, and avenues for future research
First, our study draws strength from the large-scale real-effort experiment that was executed in a dedicated warehouse lab, specifically erected for the study. This methodology was chosen to combine the realistic replication of warehouse operations, with the controlled environment of the lab. This allowed us to use a within subjects design, as well as deploy fully comparable setups across the different order picking tasks. As such, our results control for individual worker differences, order list differences, and robot movement specifications. Therefore, we believe that the results of our study might be generalised to the broader population of warehouse employees, working at typical warehouses used for storing small items in small quantities in Western Europe that choose to introduce collaborative robotics solutions. Second, our sample of vocation college students was chosen to be representative of the population of warehouse employees, and the reward system was designed to reflect business practice standards (experience, standard compensation, bonuses). This allowed us to draw conclusions from our results with academic rigour and yet readily transferable to practice.
A limitation of the study is that the outcome metrics of job satisfaction and core self-evaluations are investigated a for a short period of time. In practice, such metrics need to be monitored and assessed during longer term human–robot collaboration for understanding their development over time. Another limitation is that we have only considered the extreme cases of the human leading the robot and the human supporting the robot in our experimental setups. In reality, the roles may alternate in some hybrid solution or for different tasks (e.g. Locus Robotics Autonomous Robots Citation2020).
Future research may use our study as a starting point and further investigate the effects of introducing human–robot collaboration dynamics in order picking. Possible avenues include delving into identifying which individual characteristics relate to successful collaboration with robots (picker heterogeneity), which will further substantiate the notion of matching the right people with the right solution. Future field research may also replicate our findings in the field, as part of introductory pilot tests of collaborative robotic solutions in real operational warehouses. Studies may also consider the effects of different levels of autonomy that lie between the spectrum bounded by the setups we investigated. Hybrid setups where the leader-follower relationship roles alternate between humans and robots might also provide opportunities to investigate the mitigation of downsides that freedom of choice might provide (e.g. Locus Robotics Autonomous Robots Citation2020).
The social responsibility of safeguarding the human component in future operations in production and logistics, will be key for successfully building a sustainable, human-centric future industry 5.0 (Breque et al., Citation2021; European Commission Citation2021). We may aid the transition from the status quo, by improving employee retention, as a result of promoting and adopting business solutions that foster harmonious human-robot occupational coexistence.
Acknowledgements
The authors are grateful to Probotics AGV Systems, STC Group, Dexion, SSI Schäfer, and DHL who sponsored in kind.
Disclosure statement
No potential conflict of interest was reported by the author(s).
Data availability statement
The data that support the findings of this study are available (anonymized) from the corresponding author, upon reasonable request.
Additional information
Funding
Notes on contributors
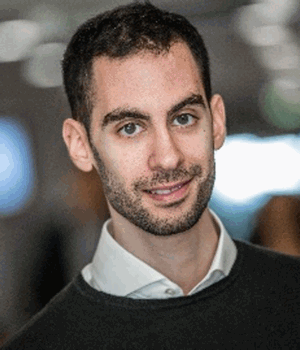
Alexandros Pasparakis
Alexandros Pasparakis is a PhD Candidate at the department of Technology and Operations Management, Rotterdam School of Management, Erasmus University. He received his B. Sc. & M. Sc. diploma in Civil Engineering, and Transportation Planning and Engineering from the National Technical University of Athens, Greece. His research aims at elevating the human condition by incorporating behavioural factors in the design and implementation of advanced technologies for operations, transportation, and the supply chain. His research findings have been presented at various international conferences, including INFORMS Annual meeting, Behavioral Operations Conference (BOC), and Manufacturing and Service Operations Management (MSOM). [email protected]
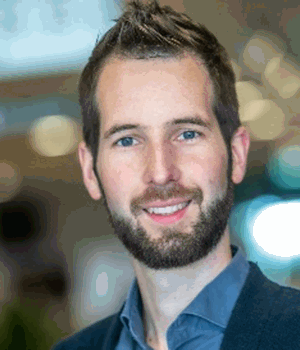
Jelle De Vries
Jelle de Vries is an associate professor of Operations Management at Rotterdam School of Management, Erasmus University. Before joining RSM, Jelle worked as Assistant Professor at VU University Amsterdam. He obtained his PhD (cum laude) and MPhil at RSM. In his research, Jelle focuses on the influence of behavioural characteristics and outcomes in operational settings. On this topic, he has published in well-respected academic outlets such as the Journal of Operations Management (JOM) and Production and Operations Management (POM). [email protected]
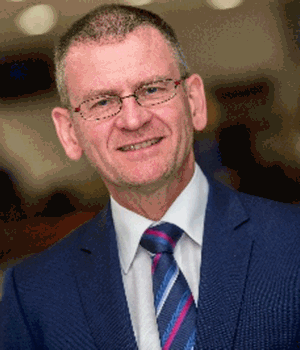
René De Koster
René de Koster is a professor of Logistics and Operations Management at Rotterdam School of Management, Erasmus University. He holds a PhD from Eindhoven University of Technology. He is the 2018 honorary Francqui Professor at Hasselt University. His research interests are warehousing, material handling, terminal operations, and behavioural operations. He is the founder of the Material Handling Forum and is author / editor of 8 books and over 250 papers in academic journals and books. He is associate editor of Transportation Science, Service Science, and Operations Research. [email protected]
References
- 6 River Systems. (2019). Meet Chuck. 6 River Systems. https://6river.com/meet-chuck/
- Azadeh, K., R. De Koster, and D. Roy. 2019. “Robotized and Automated Warehouse Systems: Review and Recent Developments.” Transportation Science 53 (4): 917–945. doi:10.1287/trsc.2018.0873.
- Azadeh, K., D. Roy, and M. B. M. De Koster. 2020. Dynamic Human-Robot Collaborative Picking Strategies. Available at SSRN 3585396.
- Bandura, A. 1986. “The Explanatory and Predictive Scope of Self-Efficacy Theory.” Journal of Social and Clinical Psychology 4 (3): 359–373. doi:10.1521/jscp.1986.4.3.359.
- Bates, D., M. Mächler, B. Bolker, and S. Walker. 2015. “Fitting Linear Mixed-Effects Models Using lme4.” Journal of Statistical Software 67 (1): 1–48. doi:10.18637/jss.v067.i01.
- Ben-Ari, M., and F. Mondada. 2018. “Elements of Robotics (Robots and Their Applications).” Elements of Robotics 1–20. doi:10.1007/978-3-319-62533-1.
- Bruemmer, D. J., D. A. Few, R. L. Boring, J. L. Marble, M. C. Walton, and C. W. Nielsen. 2005. “Shared Understanding for Collaborative Control.” IEEE Transactions on Systems, Man, and Cybernetics Part A:Systems and Humans 35 (4): 494–504. doi:10.1109/TSMCA.2005.850599
- Chen, K., J. V. Chen, and D. C. Yen. 2011. “Dimensions of Self-Efficacy in the Study of Smart Phone Acceptance.” Computer Standards and Interfaces 33 (4): 422–431. doi:10.1016/j.csi.2011.01.003.
- Compeau, D., and C. Higgins. 1995. “Computer Self-Efficacy: Development of a Measure and Initial Test.” MIS Quarterly: Management Information Systems 19 (2): 189–210. doi:10.2307/249688.
- Compeau, D., C. A. Higgins, and S. Huff. 1999. “Social Cognitive Theory and Individual Reactions to Computing Technology: A Longitudinal Study.” MIS Quarterly: Management Information Systems 23 (2): 145–158. doi:10.2307/249749.
- Cranny, C. J., P. C. Smith, and E. Stone. 1992. Job Satisfaction How People Feel About their Jobs.
- Davis, F. D. 1989. “Perceived Usefulness, Perceived Ease of use, and User Acceptance of Information Technology.” MIS Quarterly: Management Information Systems 13 (3): 319–339. doi:10.2307/249008.
- De Vries, J., R. De Koster, and D. Stam. 2016a. “Aligning Order Picking Methods, Incentive Systems, and Regulatory Focus to Increase Performance.” Production and Operations Management 25 (8): 1363–1376. doi:10.1111/poms.12547.
- De Vries, J., R. De Koster, and D. Stam. 2016b. “Exploring the Role of Picker Personality in Predicting Picking Performance with Pick by Voice, Pick to Light and RF-Terminal Picking.” International Journal of Production Research 54 (8): 2260–2274. doi:10.1080/00207543.2015.1064184.
- Donohue, K., Ö Özer, and Y. Zheng. 2020. “Behavioral Operations: Past, Present, and Future.” Manufacturing and Service Operations Management 22 (1): 191–202. doi:10.1287/msom.2019.0828.
- Effidence. 2016. EffiBOT, (AGV/AMR). Effidence. https://www.effidence.com/effibot-agv-amr-3/.
- Erez, A., and T. A. Judge. 2001. “Relationship of Core Self-Evaluations to Goal Setting, Motivation, and Performance.” Journal of Applied Psychology 86 (6): 1270–1279. doi:10.1037/0021-9010.86.6.1270.
- European Commission. 2021. Industry 5.0. Research and innovation European Union. https://ec.europa.eu/info/research-and-innovation/research-area/industrial-research-and-innovation/industry-50_en.
- European Commission, Directorate-General for Research and Innovation, M. Breque, L. De Nul, and A. Petridis. 2021. Industry 5.0 - Towards a Sustainable, Human-Centric and Resilient European Industry. Publications Office. https://data.europa.eu/doi/10.2777/308407.
- Financial Times. 2021. Why I was wrong to be optimistic about robots. Financial Times. https://www.ft.com/content/087fce16-3924-4348-8390-235b435c53b2.
- Fragapane, G., R. De Koster, F. Sgarbossa, and J. O. Strandhagen. 2021. “Planning and Control of Autonomous Mobile Robots for Intralogistics: Literature Review and Research Agenda.” European Journal of Operational Research 294 (2): 405–426. doi:10.1016/j.ejor.2021.01.019.
- Griffeth, R. W., P. W. Hom, and S. Gaertner. 2000. “A Meta-Analysis of Antecedents and Correlates of Employee Turnover: Update, Moderator Tests, and Research Implications for the Next Millennium.” Journal of Management 26 (3): 463–488. doi:10.1177/014920630002600305.
- Grosse, E. H., C. H. Glock, and W. P. Neumann. 2017. “Human Factors in Order Picking: A Content Analysis of the Literature.” International Journal of Production Research 55 (5): 1260–1276. doi:10.1080/00207543.2016.1186296.
- The Guardian. 2020. ‘I'm not a robot’: Amazon workers condemn unsafe, grueling conditions at warehouse. The Guardian. https://www.theguardian.com/technology/2020/feb/05/amazon-workers-protest-unsafe-grueling-conditions-warehouse.
- Gutelius, B., and N. Theodore. 2019. The Future of Warehouse Work: Technological Change in the U.S. Logistics Industry, (October), 1–76. http://laborcenter.berkeley.edu/future-of-warehouse-work/.
- Hackman, J. R., and G. R. Oldham. 1974. The Job Diagnostic Survey: An Instrument for the Diagnosis of Jobs and the Evaluation of Job Redesign Projects. Report No. 4. Yale University, Department of Administration Science, New Haven, CT, (1).
- Hsu, M. H., and C. M. Chiu. 2004. “Internet Self-Efficacy and Electronic Service Acceptance.” Decision Support Systems 38 (3): 369–381. doi:10.1016/j.dss.2003.08.001.
- Inam, R., K. Raizer, A. Hata, R. Souza, E. Forsman, E. Cao, and S. Wang. 2018. “Risk Assessment for Human-Robot Collaboration in an Automated Warehouse Scenario.” IEEE international conference on emerging technologies and factory automation, ETFA, 2018-septe, 743–751. doi:10.1109/ETFA.2018.8502466
- International Organization for Standardization. 2016. ISO/TS 15066:2016: Robots and Robotic Devices - Collaborative Robots. ISO.
- Judge, T. A., and J. E. Bono. 2001. “Relationship of Core Self-Evaluations Traits - Self-Esteem, Generalized Self-Efficacy, Locus of Control, and Emotional Stability - With job Satisfaction and job Performance: A Meta-Analysis.” Journal of Applied Psychology 86 (1): 80–92. doi:10.1037//0021-9010.86.1.80.
- Judge, T. A., J. E. Bono, and E. A. Locke. 2000. “Personality and job Satisfaction: The Mediating Role of job Characteristics.” Journal of Applied Psychology 85 (2): 237. doi:10.1037/0021-9010.85.2.237.
- Judge, T. A., A. Erez, J. E. Bono, and E. A. Locke. 2005. “Core Self-Evaluations and job and Life Satisfaction: The Role of Self-Concordance and Goal Attainment.” Journal of Applied Psychology 90 (2): 257–268. doi:10.1037/0021-9010.90.2.257.
- Judge, T. A., E. A. Locke, C. C. Durham, and A. N. Kluger. 1998. “Dispositional Effects on job and Life Satisfaction: The Role of Core Evaluations.” Journal of Applied Psychology 83 (1): 17–34. doi:10.1037/0021-9010.83.1.17.
- Kacmar, K. M., B. J. Collins, K. J. Harris, and T. A. Judge. 2009. “Core Self-Evaluations and Job Performance: The Role of the Perceived Work Environment.” Journal of Applied Psychology 94 (6): 1572–1580. doi:10.1037/a0017498.
- Kammeyer-Mueller, J. D., T. A. Judge, and B. A. Scott. 2009. “The Role of Core Self-Evaluations in the Coping Process.” Journal of Applied Psychology 94 (1): 177–195. doi:10.1037/a0013214.
- Locke, E. A. 1969. “What is job Satisfaction?” Organizational Behavior and Human Performance 4 (4): 309–336. doi:10.1016/0030-5073(69)90013-0.
- Locke, E. A.. 1976. “The Nature and Causes of job Satisfaction.” In Handbook of Industrial and Organizational Psychology. Vol. 1, 1297–1343. American Psychological Association.
- Locus Robotics Autonomous Robots. 2020. Locus Robotics - How it works [Video]. Locus Robotics. https://locusrobotics.com/video/how-it-works/.
- The Logistics iQ. 2020. Warehouse Automation Market. The Logistics iQ. https://www.thelogisticsiq.com/research/warehouse-automation-market/.
- New York Times. 2021. Inside Amazon's Employment Machine. New York Times. https://www.nytimes.com/interactive/2021/06/15/us/amazon-workers.html.
- Olsen, T. L., and B. Tomlin. 2020. “Industry 4.0: Opportunities and Challenges for Operations Management.” Manufacturing and Service Operations Management 22 (1): 113–122. doi:10.1287/msom.2019.0796.
- Parker, S. K., and S. Ohly. 2008. “Designing Motivating Work.” In Work Motivation: Past, Present and Future, edited by R. Kanfer, G. Chen, and R. Prtichard, 233–284. New York, NY: Routledge.
- Parker, S. K., H. M. Williams, and N. Turner. 2006. “Modeling the Antecedents of Proactive Behavior at Work.” Journal of Applied Psychology 91 (3): 636–652. doi:10.1037/0021-9010.91.3.636.
- Pasparakis, A., J. De Vries, and M. B. M. De Koster. 2021. In Control or under Control? Human-Robot Collaboration in Warehouse Order Picking. Human-Robot Collaboration in Warehouse Order Picking (March 31, 2021).
- Petković, T., D. Puljiz, I. Marković, and B. Hein. 2019. “Human Intention Estimation Based on Hidden Markov Model Motion Validation for Safe Flexible Robotized Warehouses.” Robotics and Computer-Integrated Manufacturing 57: 182–196. doi:10.1016/j.rcim.2018.11.004
- Pütten, A. R. D. E. R., and N. Bock. 2018. Development and Validation of the Self-Efficacy in Human-Robot-Interaction Scale (SE-HRI).” ACM Transactions on Human-Robot Interaction (THRI) 7 (3): 1–30. doi:10.1145/3139352.
- R Core Team. 2020. R: A Language and Environment for Statistical Computing. Vienna, Austria: R Foundation for Statistical Computing. https://www.R-project.org/.
- Rosenberg, M. 1965. “Rosenberg Self-Esteem Scale (RSE).” Acceptance and Commitment Therapy. Measures Package 61 (52): 18. doi:10.1037/t01038-000.
- Rosenthal-Von Der Pütten, A. M., N. Bock, and K. Brockmann. 2017. “Not Your Cup of Tea?: How Interacting with a Robot Can Increase Perceived Self-Efficacy in HRI and Evaluation.” ACM/IEEE international conference on human-robot interaction, part F1271, 483–492. doi:10.1145/2909824.3020251
- Schwalbe, M. L. 1985. “Autonomy in Work and Self-Esteem.” Sociological Quarterly 26 (4): 519–535. doi:10.1111/j.1533-8525.1985.tb00242.x.
- Schwarzer, R., M. Jerusalem. and others. 1995. “Generalized Self-Efficacy Scale.” Measures in Health Psychology: A User’s Portfolio. Causal and Control Beliefs 1 (1): 35–37.
- Seaman, A. 2021. LinkedIn Jobs on the Rise: 15 opportunities that are in demand and hiring now. LinkedIn. https://www.linkedin.com/pulse/linkedin-jobs-rise-15-opportunities-demand-hiring-now-andrew-seaman/.
- Spector, P. E. 1986. “Perceived Control by Employees: A Meta-Analysis of Studies Concerning Autonomy and Participation at Work.” Human Relations 39 (11): 1005–1016. doi:10.1177/001872678603901104.
- Torkzadeh, G., and T. P. van Dyke. 2001. “Development and Validation of an Internet Self-Efficacy Scale.” Behaviour & Information Technology 20 (4): 275–280. doi:10.1080/01449290110050293.
- ToyotaMHEurope. 2011. DSV Road levels their workflow and decreases stress thanks to 4 BT Autopilots. Toyota Forklifts. https://toyota-forklifts.eu/about-toyota/news-and-editorials/dsv-road-levels-their-workflow-and-decreases-stress-thanks-to-4-bt-autopilots/.
- Venkatesh, V., J. Y. L. Thong, and X. Xu. 2016. “Unified Theory of Acceptance and Use of Technology: A Synthesis and the Road Ahead.” Journal of the Association for Information Systems 17 (5): 328–376. doi:10.1080/1097198X.2010.10856507.
- The Wall Street Journal. 2021. Warehouses Look to Robots to Fill Labor Gaps, Speed Deliveries. The Wall Street Journal. https://www.wsj.com/articles/warehouses-look-to-robots-to-fill-labor-gaps-speed-deliveries-11621878163.
- Wang, X. V., Z. Kemény, J. Váncza, and L. Wang. 2017. “Human–Robot Collaborative Assembly in Cyber-Physical Production: Classification Framework and Implementation.” CIRP Annals - Manufacturing Technology 66 (1): 5–8. doi:10.1016/j.cirp.2017.04.101.
- Wang, L., S. Liu, H. Liu, and X. V. Wang. 2020. “Overview of Human-Robot Collaboration in Manufacturing.” Proceedings of 5th international conference on the industry 4.0 model for advanced manufacturing. Springer International Publishing. doi:10.1007/978-3-030-46212-3
- Wang, Z., J.-B. Sheu, C.-P. Teo, and G. Xue. 2022. “Robot Scheduling for Mobile-Rack Warehouses: Human-Robot Coordinated Order Picking System.” Production and Operations Management 31 (1): 98–116. doi:10.1111/poms.13406.
- Weiss, H. M., and R. Cropanzano. 1996. “Affective events theory.” Research in organizational behavior 18 (1): 1–74.
- West, B. T., K. B. Welch, and A. T. Galecki. 2014. Linear Mixed Models: A Practical Guide Using Statistical Software. New York: Chapman and Hall/CRC.
- Winkelhaus, S., E. H. Grosse, and C. H. Glock. 2022. “Job Satisfaction: An Explorative Study on Work Characteristics Changes of Employees in Intralogistics 4.0.” Journal of Business Logistics 43 (3): 343–367. doi:10.1111/jbl.12296.
- Wu, A. C., M. L. Gumpertz, D. D. Boos, C. Wu, M. L. Gumpertz, and D. D. Boos. 2017. Comparison of GEE, MINQUE, ML, and REML Estimating Equations for Normally Distributed Data Published by : Taylor & Francis, Ltd. On behalf of the American Statistical Association Stable. http://www.jstor.org/stable/2685999 Comparison of GEE, MI, 55(2), 125–130.
- Xanthopoulou, D., A. B. Bakker, E. Demerouti, and W. B. Schaufeli. 2009. “Work Engagement and Financial Returns: A Diary Study on the Role of job and Personal Resources.” Journal of Occupational and Organizational Psychology 82 (1): 183–200. doi:10.1348/096317908X285633.
- Xanthopoulou, D., A. B. Bakker, E. Demerouti, and W. B. Schaufeli. 2012. “A Diary Study on the Happy Worker: How job Resources Relate to Positive Emotions and Personal Resources.” European Journal of Work and Organizational Psychology 21 (4): 489–517. doi:10.1080/1359432X.2011.584386.
- Yanco, H. A., and J. Drury. 2004. “Classifying Human-Robot Interaction: An Updated Taxonomy.” 2004 IEEE international conference on systems, Man and cybernetics (IEEE Cat. No. 04CH37583) Vol. 3, pp. 2841–2846.