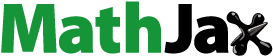
Abstract
Cognitive assistance systems help people with learning disabilities to increase their skills and consequently their employment opportunities in the regular labour market. Research on advanced work instructions has encouraged training disabled workers in cognitively demanding production tasks, especially manual assembly. However, studies lack evidence on the effect of repetition or work cycle alongside the form of instruction and type of disability. This paper addresses this gap and reports on an experiment conducted at a sheltered workplace. Four forms of instruction (paper-based, animations, projection, adaptive projection) were tested to assist operators with three types of disability (illiterate, psychosocial, cognitive) with a manual assembly task. The results show that projection enhances the first assembly cycle. Challenging operators by filtering the content of the instruction with increased experience leads to greater independence and a better understanding of their tasks. However, adaptive instructions can form a barrier for those operators who are most dependent on mentor support. The form of instruction should thus be considered carefully for each operator as their adaptation to changes and cognitive assistance systems varies. The results are discussed in light of the Industry 5.0 human-centric and socially sustainable production agenda with managerial and research implications and future research priorities.
1. Introduction
The European manufacturing industry has a crucial role in both economic and societal prosperity as it provides 15% of GDP while employing about 35 million people (Statista Citation2020; Ivanov Citation2023). The sustainability gap resulting from the demographic transition puts pressure on increasing employment rates and recruiting from a diverse population. However, manufacturing tasks, such as manual assembly, change often and are complex and, thus, require continuous learning. Learning in labour-intensive manufacturing is associated with gaining experience through increasing cumulative production or repetitions (Peltokorpi and Niemi Citation2019; Glock et al. Citation2019). Thanks to modern technologies, workers are provided with digital assistance systems to support learning (Hoedt et al. Citation2017; Mark, Rauch, and Matt Citation2022). The recently-released European ‘Industry 5.0’ aims at more human-centric, socially sustainable, and resilient production (Bai, Zhou, and Sarkis Citation2023), where the idea is to choose technologies based on an ethical rationale to support human values and needs (Longo, Padovano, and Umbrello Citation2020). Such a transition focuses on the interconnection between human intelligence and cognitive computing to assist with a human’s physical, sensorial, and cognitive capabilities. For example, a cognitive assistance system (CAS) (Mark, Rauch, and Matt Citation2022) can use artificial intelligence (AI) and augmented reality (AR) as enabling technologies. In the Industry 5.0 concept, these technologies are in the category of individualised human-machine interaction (Longo, Padovano, and Umbrello Citation2020).
CASs have mainly been tested with regular industrial workers (Mark et al. Citation2019; Schein and Rauschnabel Citation2022) but should be adapted to diverse groups of users (Mark, Rauch, and Matt Citation2022) with special needs, e.g. older employees, immigrants, and those with learning disabilities (LDs). LDs refer to ‘a heterogeneous group of disorders manifested by significant difficulties in the acquisition and use of listening, speaking, reading, writing, reasoning, or mathematical abilities’ (Seok, Meyen, and DaCosta Citation2010). People with disabilities are often outside the labour market; for example, in the EU area, only 51% of them are employed (European Commission Citation2021). In the United States, only 33% of working-age people with disabilities are employed, and two-thirds of the unemployed ones are willing to work but cannot find a job (Lindsay et al. Citation2018).
The design of CAS in terms of work instructions comprises two important aspects, which are the form of the information (the carrier or the means of storing information) and the content (the actual information) that is being conveyed to the operator (Fässberg, Fasth, and Stahre Citation2012). Our review of assembly experiments on the instruction and disability effect in Section 2.2 shows that the form of the information has dominated the content. Those studies often compare paper-based to various digital instruction forms, such as screen- (or monitor-) based and projection (which uses AR), and, less frequently, the content presented within a single form. Schein and Rauschnabel (Citation2022) point out that studies typically highlight the positive impacts of AR, with little attention being paid to the human aspect and potential barriers of AR as a workplace technology. In this line of research, one of the latest advances is the adaptation of instructions to individual needs, providing content that is relevant to the current skill level of a worker (Petzoldt et al. Citation2020).
There has been a growing interest in the potential benefits of CAS at assembly tasks for subjects with various, mainly cognitive, disabilities (Korn, Schmidt, and Hörz Citation2013; Funk, Mayer, and Schmidt Citation2015; Bosch et al. Citation2020; Vanneste et al. Citation2020). These studies report on the average performance, but very rarely on the improvement of performance, or learning, over repetitions, which is constantly needed in today’s industry. In addition, Mark et al. (Citation2019) recently showed that the current literature lacks attention to (1) the practical implementation of assistance systems in the industry for people with disabilities, and (2) the allocation of assistance systems to different types and levels of disabilities. Despite the acknowledged importance of CAS in supporting the inclusion of people with LDs in production, a question remains to be answered: How does the CAS, in more practical terms, the form of instruction, affect the assembly learning curve for people with specific disabilities?
This paper contributes to closing this gap by experimentally studying the effect of the form of instruction (paper-based, animations, projection, adaptive projection) and the type of disability (illiterate, psychosocial, cognitive) on industrial assembly performance. The effect on assembly cycle time (CT) is tested during three phases of the learning curve: the first cycle, when learning ceases (plateaus), and in the retention (plateau) phase. The ultimate goal of this study is to find out the potential of projection, specifically its adaptive version, in supporting the inclusion of people with various LDs in assembly production.
The paper is organised as follows. Section 2 provides the related literature and hypotheses. Section 3 describes the methodology used to conduct the experiment. Section 4 presents the results and Section 5 the discussion, with Industry 5.0 managerial and research implications, limitations, and recommendations for further research. Finally, Section 6 concludes the research.
2. Related literature and hypotheses
2.1. Cognitive load and learning
When a human performs an activity, a memory trace is formed. It aids a person in recalling techniques and procedures performed previously. Repetitions (work cycles) reinforce learning and improve performance as recall from memory becomes faster and dexterity increases. Cognitive load theory (CLT) (Sweller Citation1988) is concerned with how cognitive resources are distributed during learning and problem-solving. CLT distinguishes three types of loads: extraneous, intrinsic, and germane. An extraneous, or irrelevant, load interferes with the learning task at hand (Clark, Nguyen, and Sweller Citation2011). This could, for instance, be a conversation in the background while an operator is focusing on an assembly task or just work instructions that feature too much text. An intrinsic load is caused by the natural complexity of the task or the concept and information being presented (Sweller Citation2011). The intrinsic load is proportional to the interactivity of elements in the learning material. An assembly drawing that is composed of many interconnected elements is, for example, intrinsically difficult to understand (Peltokorpi and Niemi Citation2019; Peltokorpi and Jaber Citation2022). Unlike an extraneous load, an intrinsic load cannot be eliminated (as material needs to be learned); however, it can be minimised by providing the information in a structured and comprehensive way. Extraneous and intrinsic loads are additive and determine the total cognitive load. The germane load relates to schema acquisition (chunking), which is further directed to the interacting elements associated with the intrinsic cognitive load (Sweller Citation2011). For example, a schema could be a mental structure of a subassembly that needs to be processed in working memory, without the need for processing all the interacting elements of that subassembly. The learning capacity freed by reducing the extraneous load should be used to promote the germane load, to the limits of the capacity of working memory, which enhances learning. Avoiding the extraneous, managing the intrinsic, and promoting the germane load depends on the characteristics of the subject (e.g. level of experience, disability), the complexity of the task, work conditions, instructional methods, and their mutual relations (Kirschner Citation2002).
2.1.1. Learning curve
Production and operations management (POM) research uses learning curves (LC) to measure human performance when a psychomotor task is performed repetitively (Glock et al. Citation2019). The simple power-form LC of Wright (Citation1936) is a standard on which further modifications are built. Wright’s learning curve, henceforth WLC, is of the form:
(1)
(1) where
and
are the execution times for the
th and the first cycle, respectively, and b = −log(∅)/log(2), 0 ≤ b ≤ 1, is the learning exponent, where ∅ = % is the learning rate divided by 100. When fitting a trend line to raw data, the plot usually shows a considerable scatter around the trend line. The scatter in the data is caused by many factors, and their mutual relationships, which are interconnected with the cognitive load. Jaber et al. (Citation2021) recently adjusted WLC (Eq. 1) for cognitive interference by assuming that an operator cannot retain all the information from cycles, but some information is lost over time. Their interference-adjusted WLC, henceforth IAWLC, is of the form:
(2)
(2) where
is the cumulative residual experience (after interference),
is a fitting parameter,
is a decay exponent, 0 ≤
≤ 1, and
is a fixed time step in the calculation procedure. By increasing the decay exponent (indicating greater interference), the estimated curvature and level at which learning plateaus increase. When interference is not captured (
= 0), the model behaves like WLC. IAWLC was found to outperform other LCs, as it captures characteristics that are related to assembly processes better, i.e. it predicts poor performance during the first cycle (which is closely related to cognitive interference), followed by rapid learning in subsequent ones. Adjustment for cognitive interference improved the curve fit to assembly learning data from subjects who have cognitive disabilities (Jaber et al. Citation2021). The recent modification of IAWLC with a composite of cognitive and motor LCs (Peltokorpi and Jaber Citation2022) fitted various learning profiles, including ‘early strugglers’ and ‘early adopters’, well. Note that learning may cease for many reasons. In psychomotor tasks, this happens because cognitive task elements dominate over motor elements during earlier cycles, while motor elements (which are subject to a minimum or standard time) dominate over cognitive elements during later cycles. As workers gain experience, variation in performance typically decreases.
2.2. Learning with disabilities, using instructions
Forms of instruction should consider well-defined principles for handling various types of cognitive load (Annex A). These principles hold even more weight for those with LDs. Disabled learners may suffer from working memory deficits related to a phonological loop that is closely linked to the complex cognition required during many industrial tasks (Swanson and Siegel Citation2001). In general, tasks that place high demands on cognitive processing are difficult for those with LDs, as they have trouble with attentional processes (Mark, Rauch, and Matt Citation2022), especially while facing distraction. Problems with metacognitive regulation (i.e. how we control cognition) result in an individual inability to actively engage in learning the task at hand (Seok, Meyen, and DaCosta Citation2010).
Mark et al. (Citation2019) found that cognitively disabled persons may benefit from software systems, projection-based support, and AR in general. The design principles that aim at managing the intrinsic load (pre-training, segmenting, and allowing learners to control the pacing) are particularly beneficial for them (Seok, Meyen, and DaCosta Citation2010). The promotion of meaningful learning (memorising and deep understanding of instructional material) can be induced by multimedia learning, which integrates text and pictures (Mayer Citation2011). Pictorial instructions especially are helpful for those with impaired communication skills. Mark, Rauch, and Matt (Citation2022) recently proposed a methodology for the selection of an assistance system based on parameters that take into account the workplace, the type of disability, and the particular work task. The methodology was tested for mentally disabled workers who assembled components of a door lock at a sheltered workplace. The mental limitation was reflected in a lack of concentration, spatial imagination, and creativity. In this case, projection-based CAS best matched the conditions.
Most importantly, the form of the instruction should be driven by the learner as those with LDs also interact with CAS in different ways than regular persons do (Seok, Meyen, and DaCosta Citation2010). For example, extensive sound and visual projection elements can be disturbing for users who are sensitive to sensorial stimuli (Grund et al. Citation2020). On the other hand, additional mentor efforts may be needed during technology-assisted training (Bosch et al. Citation2020).
2.2.1. Case studies
Table gives an overview of thirteen case studies on the effect of the form of instruction and disability on assembly performance. For each contribution, the number of participants (N) and the type of their disability, the use case (whether industrial or not), the principle of pre-training, and the ranking of instruction forms (or disability types) per dependent variable (mainly CT and error rate) are presented. Among the different forms of instruction, the most elementary one is paper-based and that served as a baseline. Unless otherwise mentioned, the instructions consisted of the same content, i.e. text and images, presented step by step. Screen-based instructions are very similar to paper-based ones but are shown on a monitor. This often helps the operator as there is no need to search for the right instruction (page) after each step. By pushing a button, the next step is supported with the right instructions. Projection is a common way of showing AR instructions that uses camera-based technology to display crucial information in the operator’s field of view. Projection is the only form among AR technologies that does not require a see-through display, such as smart glasses, tablets, etc.
Table 1. Overview of empirical studies on the effect of the form of instruction and disability on assembly performance (n/a = significance not assessed, * = significant difference, otherwise non-significant difference).
Furniss et al. (Citation1999) experimented with whether two mentally disabled subjects could assemble a valve faster and with fewer errors by using a screen-based handheld device. A severely disabled subject performed better on both measures when audio prompts in the device were enabled. A less disabled subject was guided by paper- and screen-based instructions. The latter condition resulted in faster performance, while the paper instructions produced slightly fewer errors.
In the study of Lancioni et al. (Citation2013), two totally blind and profoundly intellectually disabled subjects assembled five-component trolley wheels. The baseline condition included, when necessary, only verbal assistance by an experimenter. An intervention with technology provided prompts, spatial guidance, and positive feedback in a way that enabled a faster task completion rate and the prevention of errors. Lancioni et al. (Citation2014) conducted another study with three multi-disabled subjects (each deaf and visually and intellectually impaired) who assembled five-component water pipes. Human-assisted conditions were compared with an intervention using light cues and a stimulation device. With the help of technology, the subjects were able to perform the assembly consistently and independently. In the old conditions, pipes were assembled correctly at a very slow rate while continuous human assistance was needed.
Korn, Schmidt, and Hörz (Citation2013) compared screen-based instructions and in situ projection (without feedback on errors) for cognitively and/or physically disabled subjects whose instructions were normally paper-based. The subjects assembled eight Lego cars, each consisting of nine bricks. The use of projection, on average, reduced CT by 8%, while the absolute increase in the error rate was 6.1%. In general, projection was seen as a way to reduce cognitive load. However, a more detailed analysis showed that 40% of those receiving instructions through projection performed worse than usual, and in particular there was a lack of quality. The authors concluded that the use of in situ projection should be restricted to those whom it suits well. For the ones it did not suit, they provided a potential explanation: they are slow learners and need more time to get adjusted to the new technology.
In the study of Korn, Funk, and Schmidt (Citation2015) mild cognitively (and partly physically) disabled subjects assembled metal shears in a process consisting of five steps. When there was a change from screen-based instructions to projection (with gamification), they were, on average, 6.4% faster but with a 6.0% absolute increase in their error rate. As the projection conditions in Korn, Schmidt, and Hörz (Citation2013), and Korn, Funk, and Schmidt (Citation2015) did not involve quality feedback (real-time error detection), a speed-accuracy trade-off was likely to occur. Korn et al. (Citation2016) studied the forms of instruction and assembly, like Korn, Funk, and Schmidt (Citation2015), but with five mentally disabled subjects during an entire workday. Each subject was assigned to one of the disability categories based on his/her performance index (PI), indicating a percentage capacity that the subject can perform a task against a regular worker. The most capable (PI = 35%) subject was significantly faster with projection and the least capable (PI = 5%) with screen-based instructions.
Similarly, Funk et al. (Citation2015) studied the assembly of a Lego product with cognitively disabled workers assigned to screen-based instructions or in situ projection (with feedback on errors). They found that, by using projection, the workers could assemble more complex products up to three times faster and with up to 50% fewer errors. In another study, Funk, Mayer, and Schmidt (Citation2015) compared pictorial, video, and contour visualisations for projected instructions to assemble a clamp consisting of five parts. 62 mentally disabled subjects participated in the experiment. The group using contour visualisation was the fastest and made the fewest errors, pictorial visualisation was the second fastest, and the group with video visualisations made the second fewest errors.
Kosch et al. (Citation2018) compared three different error-feedback modalities, visual (projected light), auditory (error sound), and haptic (vibrating gloves), for cognitively impaired subjects who assembled a Lego product using paper instructions. Each feedback modality was used once per subject. Visual feedback outperformed auditory and haptic feedback in terms of both productivity and quality.
Bosch et al. (Citation2020) reported two case studies where novices were trained by a human or, in addition, projection guidance. In both studies, the subjects had one or more unspecified disabilities. In the first study, a part of a solar panel was assembled. At the beginning of the training session, those supported only by a human were 45% faster. In the end, the use of projection guidance, where the amount of information was reduced every 10 min, was 22% faster. It is noteworthy that additional human support was needed with the use of projection. After training, in the retention phase, the projection support was removed and no significant difference in CT was found between the subject groups. For both groups, human support decreased by 90% from that at the beginning of the training. The second study investigated the amount of training needed to reach a predetermined CT in a light assembly context. In the old situation, with only human support, it took one to eight hours. With projection guidance, three cycles were needed for, on average, a 30% reduction in CT, and two-thirds of the subjects needed less human support.
Vanneste et al. (Citation2020) studied three tasks, assemblies of a light fixture and wheel and quality control of an aluminium part, with subjects with varying levels of cognitive disability and experience. Each subject first performed one of the three assembly tasks four times, and then the two remaining tasks once each. The capabilities of the subjects and the tasks and the sequence in which they were performed were counterbalanced between three forms of instruction, oral, paper-based, and projection. The results show that projection slightly increases the mean CT in comparison to paper and oral instructions. The advantage of better productivity with projection in the first cycle disappeared during the subsequent cycles, probably as a result of the operators being only distracted by the projection while needing less support with increasing experience. Projection reduces the mentor’s effort during training and allows the operator to access more complex assembly activities.
In only one case study in Table (#11, [Bosch et al. Citation2020]) projection support was steadily reduced as the subjects gained more experience. Hence, the next section reviews the literature on adaptive instructions, which are also used in our experimental study.
2.2.2. Adaptive instructions
The effect of individual adaptivity in AR instructions has, to our knowledge, not yet been studied with disabled subjects (Heinz et al. Citation2021). Petzoldt et al. (Citation2020) studied a CAS comprising four functionalities: (1) instruction system; (2) quality and completeness control; (3) progress recognition; (4) individualisation of support and worker qualification. They stated that the assistance system should adapt to the needs of an individual worker, provide only relevant information, and decide the appropriate detailing of instructions. This would happen by measuring appropriate indicators to calculate a corresponding qualification score. As for performance indicators, they propose the average throughput time for a given number of assembled products and its variation. This would also apply to individual process steps and searching time. Furthermore, they suggest this approach to facilitate the training of new employees.
Kelleher and Hnin (Citation2019) conducted experiments to explore and provide insights into the effect of the average number of attempts, steps, and restarts on the degree of struggling (the rated cognitive load) when completing puzzles. They also developed a model with the intention of predicting the cognitive load on a future code puzzle problem. For future work, they recommend developing adaptive learning systems that shape the learning experience in new ways, such as predicting the cognitive load from learning curves.
Tsutsumi et al. (Citation2020) used multiple physical human properties and a self-evaluated assembly skill level to estimate the experience level of a worker before the start of production. Along the process, the experience level is updated after a certain number of assembly iterations. They state that changing the instructions should be well-considered since it could cause the workers some confusion. Heinz et al. (Citation2021) presented a concept and implemented an assembly line with interactive in situ projections to detect changes in the performance levels based on the analysis of the processing times of workers with and without disabilities. For further studies, they suggest investigating the ways in which ‘workers with disabilities can benefit from performance-based adaptations’.
2.3. Hypotheses
The LC effect received very little attention in the case studies (Table ), despite its importance in the POM field and manual assembly (Glock et al. Citation2019; Peltokorpi and Jaber Citation2022). In this study, the effects of the form of instruction and type of disability on assembly CT are tested during three phases of the LC: the first cycle, when learning ceases (plateaus), and in the retention (plateau) phase. The plateau CT, or threshold, is defined later in Eq. (5) in Section 4.1.2. We assume, like Jaber et al. (Citation2021), that an operator cannot retain all the information from cycles, but some information is lost over time. We fit their LC, in Eq. (2), to individual learning data, to estimate the level of cognitive interference and retention.
The following six research questions (RQ) and hypotheses (H) are used. Hypotheses H1-H3 are for the effect of instruction form and are derived from the case studies. Instead, H4-H6 are derived from the experience of the present sheltered workplace, as the effect of disability type has not been studied before.
RQ1: How does the form of instruction affect the first assembly CT for disabled workers?
H1: More advanced instructions have a positive impact on the first assembly CT. The mean of the first assembly CTs is the shortest with projected instructions, followed by animations and paper instructions.
RQ2: How does the form of instruction affect CT when learning plateaus?
H2: More advanced instructions have a positive impact on CT when learning plateaus. After a percentage improvement, the mean of the CTs at which learning plateaus is the shortest for adaptive projection, followed by projection, animations, and paper instructions.
RQ3: How does the form of instruction affect retention in the plateau phase?
H3: In the plateau phase, instructions are no longer needed, and workers perform equally. Retention in the plateau phase in terms of the percentage of CTs below the threshold and the level of cognitive interference is the same with each form of instruction.
RQ4: How does the type of disability affect the first assembly CT?
H4: Illiteracy slows down the comprehension of new learning material. The mean of the first assembly CTs is longer for illiterate subjects than for psychosocially and cognitively disabled ones.
RQ5: How does the type of disability affect CT when learning plateaus?
H5: Cognitive disability has a negative impact on CT when learning plateaus. After a percentage improvement, the mean of the CTs at which learning plateaus is the longest for cognitively disabled subjects, followed by psychosocially disabled and illiterate subjects.
RQ6: How does the type of disability affect retention in the plateau phase?
H6: Retention in the plateau phase is the worst (the percentage of CTs below the threshold is the lowest and the level of cognitive interference is highest) for cognitively disabled subjects, followed by psychosocially disabled and illiterate subjects.
3. Methodology
3.1. Case product and participants
To investigate the hypotheses, industrial experiments were conducted at Senzer, a sheltered workplace located in the Netherlands, where people with various disabilities are given the opportunity to be employed. The case product was the adjuster of a Dorel’s Maxi Cosi car seat. This seat adjuster consists of three subassemblies and a total of 17 parts to be assembled.
A total of 24 subjects (both male and female) participated in the experiment and were divided into three groups (with eight subjects in each) based on their disability. Illiterate (ILLIT) cannot read, psychosocially (PSYSOC) disabled subjects are either sensitive to stress or more dependent on mentor support, and cognitive (COG) disabled subjects have intellectual issues or are just slow learners.
3.2. Instructions
Four forms of instruction were studied: paper-based (PAPER), animations (ANIM), projection (PROJ), and adaptive projection (ADAPT). The elements used for each form of instruction are presented in Figure , inside the corresponding box. In general, the instructions follow the design principles and guidelines presented in Annex A. Each form of instruction shows the required tools and parts and the progression after each of the 49 micro steps to complete the three subassemblies of a seat adjuster.
Figure 1. Forms of instruction studied with the elements inside a box.
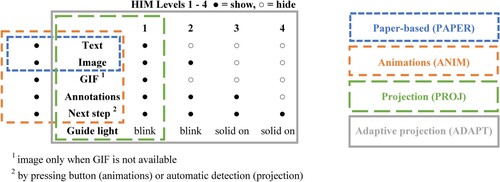
Paper-based instructions, with text and images, form the baseline for more advanced instructions. Animations present text and images on a screen extended with GIFs (where possible) and annotations, such as arrows and wrong/right symbols. The operator controls the transition to the next step with a button. The projection extends the animations with a blinking guiding light, and all the instructional elements are projected on the tabletop using the HIMFootnote1 (Human Interface Mate) spatial AR technology. The HIM is a commercially available device, developed by Arkite,Footnote2 that tracks and registers the progress of manual assembly operators via a vision and infrared sensor system. The HIM uses AI technology to automatically detect when a step is finished and shows the next step. Thus, the HIM fits perfectly into the Industry 5.0 concept.
Adaptive projection uses an algorithm to determine the operator’s experience level, with 1 indicating a beginner (equals ‘projection’) and 4 the most experienced level (as in Moghaddam et al. (Citation2021)). More precisely, the experience level is determined for 17 step groups, each consisting of an average of three sequential micro steps, for example: (1) grab the axle pusher, (2) push the axle against a stop, and (3) put the axle pusher back. Figure depicts an example of the adaptation of an operator’s experience level as a function of assembly cycles for this step group. To determine the current experience level, the algorithm considers the CTs of the last three step groups and makes a comparison with the step group CT of an outstanding operator. In this example, the operator reaches an experience level of 3. When the experience level increases, the number of instructional elements shown (within the step group) decreases, and vice versa. Level 2 (column 2 in Figure ) hides text and GIFs, level 3 images, and level 4 annotations. At levels 3 and 4, the guiding light is set to ‘solid on’ as the operators are more familiar with the locations and a blinking light would only distract them.
3.3. Experimental procedure
The study used a between-groups (types of disability, forms of instruction) repeated measures (numbers of assembly cycle) design with random assignment of subjects to the instruction forms. The experiments took four weeks, with one instructional group per week. Each day, two subjects executed the assembly of a seat adjuster as many times as possible during a two-hour session. The subjects had no prior experience with this product or the HIM unit, and they were not allowed to conduct pre-training. A short introduction to the assembly task was given by a mentor, along with a brief explanation of the form of instruction. The mentor helped with any substantial difficulties to ensure the progression of the operator. This happened at the initiative of either the operator or the mentor. The first runs were video-recorded as the subjects were most probably struggling with the tasks and might need substantial help from the mentor. This made it possible for the researcher in the later analysis to count the times each subject was helped. At the end of the experimental session, the mentor could write a general comment about how the subject performed. Note that mentor help and comments are not considered primary indicators but provide only additional insights into the performance. After cycles 1, 2, 4, and 8, the subjects had a break of one minute.
4. Results
For hypotheses H1-H6, the effects of the form of instruction and type of disability on initial and plateau CT and retention in the plateau phase were analysed. The analysis was conducted using the IBM SPSS Statistics v.28 software. The mean CTs at instruction form level and disability type level in each cycle are calculated as follows:
(3)
(3)
(4)
(4) where
and
are the total numbers of subjects with the form of instruction
and type of disability
in cycle
. In most cases, 15 cycles were considered. Some subjects did not reach this number of cycles, which has been taken into account in the results and analysis.
4.1. Instruction effect
Figure presents the mean CT for each form of instruction and cycle (Eq. 3).
Figure 3. Effect of form of instruction on mean and SD (2σ) of CT in each cycle.
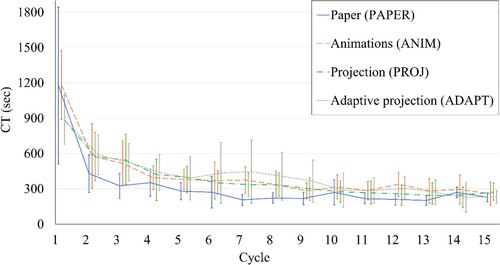
4.1.1. First cycle time
The mean of the first assembly CTs is the shortest with projection-based (PROJ and ADAPT) instructions. Since the first cycle is not subjected to adaptivity, these groups were combined (with 885.8 ± 207.9 sec). ANIM (1182.5 ± 292.7) and PAPER (1178.4 ± 665.8) performed worse in this regard. According to an independent samples T-test, with ANIM, it took significantly longer in the first cycle than with projected instructions (p < 0.012; size effect Cohen’s d = 1.249). Between PAPER and projected instructions, the difference is non-significant (p < 0.086; d = 0.714). Thus, H1 is partially validated. In the first cycle, the mentor helped significantly more often those using projections (7.75 ± 3.39 times) than PAPER (3.83 ± 4.07; p < 0.023; d = −1.083) and ANIM (4.33 ± 3.14; p < 0.028; d = −1.031).
4.1.2. Plateau cycle time
After a rapid initial learning phase, LC levels out i.e. enters a plateau. Entering the plateau, or steady-state, phase needs sufficient training (cycles). Therefore, the plateau analyses in this study exclude the six operators who finished less than 14 products, i.e. one operator with COG and one with PSYSOC disability in the ANIM group, and two COG disabled in both the PROJ and ADAPT groups. For the remaining 18 operators, we assume that entering the plateau phase occurs when a total improvement of 85% (difference between the first and the best CT) is reached for the first time. Beyond that, CTs are assumed to fall within the (upper) limit of the remaining 15% of improvement. This threshold was set based on the collected data. 77% of the CTs after the defined plateau entrance are within the expected interval. Thus, for each subject, the plateau CT is calculated as follows:
(5)
(5) where
represents the max. CT for an operator to enter the plateau phase,
is the first, and
is the shortest (best) CT of an operator. The analysis revealed that, on average, the subjects entered the plateau phase at cycle 6.1 ± 3.2, with
= 305.8 ± 86.3 sec. ADAPT group has the lowest plateau CT (263.5 ± 38.8 sec, entered at cycle 6.5 ± 2.4), followed by PROJ (303.6 ± 80.8, at 6.0 ± 3.4), PAPER (311.8 ± 113.7, at 5.8 ± 2.5), and ANIM (341.3 ± 92.0, at 6.3 ± 5.4); however, the difference between ADAPT and ANIM is non-significant (p < 0.085, d = 1.103), as in other pairwise comparisons. Thus, H2 is rejected.
4.1.3. Retention
We use two measures to assess retention in the plateau phase. The first one is the percentage of CTs below the plateau CT in Eq. (5). The second one is the memory decay exponent, indicating the level of interference, and obtained from fitting the interference-adjusted model (IAWLC) by Jaber et al. (Citation2021), Eq. (2), to each subject’s learning data. These two measures have a strong negative correlation (−0.62) over the entire population, i.e. more CTs below the threshold indicates less interference. The percentage of CTs below the threshold is significantly lower with ADAPT (57.5 ± 22.5%) compared to ANIM (84.8 ± 15.2%; p < 0.046; d = 1.422) and PROJ (85.3 ± 10.5%; p < 0.033; d = 1.582), and non-significant compared to PAPER instructions (73.3 ± 31.3%). On the other hand, ANIM has the highest decay exponent ( = 0.317 ± 0.078), followed by PAPER (
= 0.298 ± 0.280), ADAPT (
= 0.253 ± 0.050), and PROJ group (
= 0.147 ± 0.112). The difference between ANIM and PROJ groups is significant (p < 0.024; d = 1.759). Thus, H3 is rejected.
4.2. Disability effect
Figure presents the mean CT for each type of disability and cycle (Eq. 4).
Figure 4. Effect of type of disability on mean and SD (2σ) of CT in each cycle.
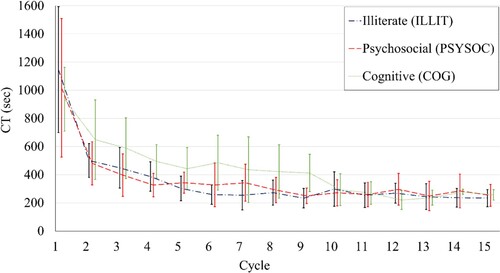
4.2.1. First cycle time
The mean of the first assembly CTs is the shortest for COG (936.5 ± 227.0), followed by PSYSOC (1016.9 ± 492.6) and ILLIT group (1146.0 ± 447.7). This order is in line with H4; however, each pairwise difference is non-significant. In the first cycle, the mentor helped PSYSOC group (3.75 ± 2.605) significantly less often than ILLIT (6.75 ± 2.765; p < 0.021; d = 1.117) and COG (7.25 ± 5.092; p < 0.053; d = −0.865) groups.
4.2.2. Plateau cycle time
COG and PSYSOC groups have equal plateau CT, but the first-mentioned reached it 1.7 cycles later (293.2 ± 114.8 sec, entered at cycle 7.7 ± 2.1; and 295.4 ± 95.6, at 6.0 ± 3.4, respectively). ILLIT group has a slightly longer CT than the other two but entered the plateau earlier (319.5 ± 78.1, at 5.6 ± 3.5). The CTs (and the number of cycles needed) to enter the plateau phase were not significantly different between the types of disability, and thus H5 is rejected.
4.2.3. Retention
In the plateau phase, the percentage of CTs below the threshold is the lowest for COG (63.3 ± 28.7%), followed by PSYSOC (66.4 ± 23.5%) and ILLIT group (86.9 ± 18.8%). The percentage is significantly lower for PSYSOC (p < 0.042, d = 0.968) but non-significantly lower for COG (p < 0.069, d = 1.099), compared to ILLIT. COG disabled subjects experienced the greatest interference (γ = 0.426 ± 0.191), followed by PSYSOC (γ = 0.242 ± 0.209) and ILLIT (γ = 0.211 ± 0.112). Interference was significantly higher for COG than ILLIT (p < 0.021, d = −1.607). Based on these results, H6 can be validated.
5. Discussion
In this section, we discuss the results for each hypothesis, with the aim being to gain a better understanding of the factors behind them. We then provide managerial implications concerning the suitability of the forms of instruction for each type of disability. The discussion ends with research implications and the study limitations and future research priorities.
5.1. Hypotheses and results
H1: More advanced instructions have a positive impact on the first assembly CT. The mean of the first assembly CTs is the shortest with projected instructions, followed by animations and paper instructions.
Result: The mean of the first assembly CTs was shorter with projection than with paper instructions and significantly shorter than with animations.
H2: More advanced instructions have a positive impact on CT when learning plateaus. After a percentage (85%) improvement, the mean of the CTs at which learning plateaus is the shortest for adaptive projection, followed by projection, animations, and paper instructions.
Result: The differences between the forms of instruction were non-significant.
H3: In the plateau phase, instructions are no longer needed, and workers perform equally. Retention in the plateau phase in terms of the percentage of CTs below the threshold and the level of cognitive interference is the same with each form of instruction.
Result: The percentage of CTs below the threshold is significantly lower with adaptive projection compared to animations and projection. The level of cognitive interference is significantly higher with animations than with projection.
H4: Illiteracy slows down the comprehension of new learning material. The mean of the first assembly CTs is longer for illiterate subjects than for psychosocially and cognitively disabled ones.
Result: The differences between the types of disability were non-significant.
H5: Cognitive disability has a negative impact on CT when learning plateaus. After a percentage (85%) improvement, the mean of the CTs at which learning plateaus is the longest for cognitively disabled subjects, followed by psychosocially disabled and illiterate subjects.
Result: The differences between the types of disability were non-significant.
H6: Retention in the plateau phase is the worst (the percentage of CTs below the threshold is the lowest and the level of cognitive interference is the highest) for cognitively disabled subjects, followed by psychosocially disabled and illiterate subjects.
Result: The percentage of CTs below the threshold is significantly lower for psychosocially disabled compared to illiterate subjects. The level of cognitive interference was significantly higher for cognitively disabled than illiterate subjects.
5.2. Industry 5.0 managerial implications
In this section, we provide some managerial implications of the results, in line with the Industry 5.0 concept of individualised support. Some additions to the results are given to clarify our opinion on the results. Those are mainly based on observations the mentor made during the experiment that was conducted.
5.2.1. Illiterate
The first cycle time of the ILLIT group, divided over the four forms of instruction, was the highest. Over the cycles, they almost reached the assembly time of the subjects in the other groups. This means that they have the greatest potential in terms of productivity, but they face difficulties when a new procedure must be learned. For those who are illiterate, it is very important to tailor the instructions to their needs when they learn new assembly tasks, i.e. avoid text and make the instructions as intuitive as possible. The mentor’s remarks are: ‘can’t read instructions, so had a lot of trouble with it’ and ‘went better once the procedure was known’. For this group, text-based instructions led to much worse productivity in comparison with more advanced instructions. In this study, the ILLIT group was perhaps best supported with ANIM, which includes pictorial elements that are helpful for those with impaired communication skills (Mark, Rauch, and Matt Citation2022). By extending PROJ with ADAPT some improvement is observed, by a lower CT when plateauing, but at a cost of worse retention thereafter. However, the differences at the plateau level are not significantly high.
5.2.2. Psychosocial disability
It is hard to draw strong conclusions about the PSYSOC disabled group. First of all, there is a great difference in cognitive abilities among them. Furthermore, these subjects depend much more than the others on their immediate feeling. However, from the results it is clear that animations are to be avoided since people with PSYSOC issues are ‘easily disturbed’ (mentor’s remark) by moving images. Both for the first and the plateau CT, ANIM provided the least productive results and PROJ the best results. As with ILLIT group, PROJ extended with ADAPT slightly reduced the plateau CT but at a cost of worse retention. A less independent operator with PAPER instructions was exceptionally fast in the first cycle but her CTs scattered to a great extent thereafter. This suggests that some people with PSYSOC disability feel especially comfortable when they are assigned instructions they are familiar with. However, to increase their ability to maintain mental capability and working pace, they often need prompts (Furniss et al. Citation1999), such as projections. This was also reported by the mentor when observing a subject using the ADAPT system: ‘uncertain at first, but after a few iterations it went better’.
5.2.3. Cognitive disability
People who are COG disabled learn much more slowly than others. Although their first CT was the best (on average) over the different forms of instruction, they had the smallest margin of improvement. Just like the previous group, animations are not recommended for this group. The quickly-changing images are hard to capture and translate to reality. The best performance was achieved using PAPER instructions. Not only was the CT the shortest for the first cycle, but also the subjects were more independent. Because of their disability, they experience a higher intrinsic workload, which consequently should be taken into account when instructing them. Both subjects with PAPER kept assembling more than twice as many products as their peers with projection. Some of the remarks the mentor made with this group using projection-based instructions: ‘does not work alone’; ‘has a lot of trouble understanding the instructions’ and ‘continuously struggling’ with certain components, which is in line with Korn, Schmidt, and Hörz (Citation2013). The COG disabled persons worked very unpredictably, meaning that no clear learning effect is visible when plotting the data. Subsequently, they do not benefit from adaptivity (which is based on predictivity). The barrier to getting used to the HIM unit is also quite high for them. Because of their deficits in controlling cognition, the adaptive algorithm may also shift their experience level too soon, resulting in discomfort and a reduction of productivity.
5.2.4. General remarks
In order to support impaired assembly operators with their job, work instructions should be tailored to the individual’s needs. No form of instruction is the best; it all depends on the user (Mark, Rauch, and Matt Citation2022). The trend towards more advanced CAS for guiding operators has already proved its value in the regular market (Petzoldt et al. Citation2020); however, in the case of impaired people the use of such systems must be handled with care. During the different experimental runs, it became clear that often the intuitive and adaptive CAS forms a barrier for the people rather than a support. This is probably caused by the short introduction to the system the subjects received. Therefore, we strongly recommend providing enough time to introduce the new CAS to the operators, since this is crucial for the system to be effective.
In general, PAPER, along with ADAPT instructions, showed the best plateauing effect over the three disability groups. There might be two reasons for this. First, an operator who knows the task at hand can easily neglect PAPER instructions and is well served by the ADAPT system, where ANIM interfere with the task at hand. This is an example of an extraneous cognitive load, meaning that instructions should only be provided when it is necessary. Second, the use of PAPER is quite demanding, since the operator must search actively for the right information. This additional effort results in a higher CT at the beginning, but it also enforces retention, which, in the following iterations, helps to speed up the learning process. Challenging operators does not result in quick wins, but in the long run, it can be beneficial with respect to their productivity and independence.
Even though no serious improvement in productivity is observed using PROJ and ADAPT instructions, the mentors declared an improvement in the independence of the impaired workers. On top of that, the technology enables some of them to execute more complex tasks. This could have a great impact on the employment rate of people with a disability. To summarise the managerial implications, high-tech CAS has the potential to support the inclusion of people with various learning disabilities in industrial production. This directly contributes to the Industry 5.0 agenda on human-centric and socially sustainable production (Ivanov Citation2023), and the UN sustainable development goals of industry, innovation, and infrastructure (SDG 9), and reduced inequalities (SDG 10) (Bai, Zhou, and Sarkis Citation2023).
5.3. Industry 5.0 research implications
This study contributes to the Industry 5.0 literature (Mark et al. Citation2019) by practically implementing a CAS in the industry for people with various types of disabilities. The assembly learning data obtained from the experiment is valuable in the POM research, which develops and uses LCs to measure human performance in repetitive tasks (Glock et al. Citation2019). The data and the results have implications for developing LC theory where the form of instructions and type of disability are the explanatory factors. The results can further be used to develop the methodologies for the selection of CAS based on parameters that consider the type of disability and work task (Mark, Rauch, and Matt Citation2022). The findings of our study imply that high-tech CAS can have a positive impact on the employment of people with cognitive issues, in line with the review of (Sauer, Parks, and Heyn Citation2010).
5.4. Limitations and future research priorities
Despite its interesting findings, this paper has some methodological limitations that should be addressed in future research. First and foremost, some results are statistically non-significant and cannot be generalised. More studies with larger sample sizes would give more reliable evidence. For example, the plateau CT comparisons showed a very large effect size d = 1.103 and practical significance in favour of adaptive instructions over animations. However, to have enough statistical power (of 0.8) the group sample sizes should be increased from N = 4 to N = 11. In the field of psychology, a large effect size of d = 0.8 is assumed. This corresponds to a group sample size of N = 21, which, in our experimental setting, requires 84 participants (28 of each type of disability), which would be extremely time-consuming, if not impossible.
Another limitation is a rather short experimental time of 2 h for novices. The instruction effect should be tested over a longer training period to allow disabled workers to overcome the learning barriers of new technologies and enable gradual improvement. Adaptation to individual needs could be performed by alternating between traditional paper instructions and the most promising advanced form of instruction. In addition, instructions should be tailored to respond better to the behaviour and needs of a specific disability, such as a cognitive one.
Worker independence received rather little attention in this study despite it being an important performance indicator for disabled subjects. In further studies, mentor support (both quantity and quality) should be registered more systematically over repetitions, perhaps using automatic detection. The next limitation of our study is the lack of subjective evaluation of user experience and perceived workload. During the experiment, we learned that standard questionnaires did not work, hence, further research should develop specific methods to enable a more realistic assessment of disabled workers’ experience and perceived workload.
6. Conclusions
In this paper, we studied the effect of both the form of instruction (paper-based, animations, projection, adaptive projection) and worker disability (illiterate, psychosocial, cognitive) on the learning of a manual assembly task. The operators assembled the adjuster of a Dorel’s Maxi Cosi car seat. Times were registered and observations were made during the assembly runs. The learning effect, in terms of cycle time, was analysed in three phases: the first cycle, when entering a plateau level, and retention thereafter. Although each individual had different needs, some general conclusions can be drawn based on the results of this study:
More instructional elements and cognitive assistance (projection-based) enhance the first cycle.
Projected instructions are useful but make operators more dependent on the guidance system. If the operator’s profile allows it, challenging him/her by filtering the instruction content with increased experience leads to more independence and a better understanding of their tasks. People with psychosocial issues, on the other hand, do not feel comfortable when the level of assistance drops.
Highly intuitive and adaptive instructions do not seem appropriate for cognitively disabled operators with unpredictable performance and great dependence on mentor support.
The form of instruction should be considered individually and in an unprejudiced manner, as the differences between operators and adaptation to cognitive assistance systems vary a lot, including within the same disability group.
The experimental methods and findings contribute to the Industry 5.0 individualised human-machine interaction framework. The used cognitive assistance system with AI and spatial AR technologies enables automatic monitoring of work and projecting those instructional elements that each operator needs in each work step. Theory, experimental results, and experiences in a sheltered workplace provide insights into how to implement support for a range of disabled operators. Intuitive and adaptive instructions enable more people to become involved in complex tasks and have a better chance of being employed in the regular labour market. The advantages of cognitive assistance systems also hold true for regular workers, especially when they are constantly facing new products. The cost-efficiency they provide can be significant as companies can increase cross-training while releasing mentors for other tasks.
Acknowledgements
We thank Ton Janssen, Liese van Oort, and Rob Kerkhofs, at Senzer, and Bart Lamberigts, at Arkite NV, for providing resources and support during the project. We also thank the anonymous reviewers for their constructive comments to improve this paper.
Data availability statement
The data that supports the findings of this study is available from the corresponding author upon reasonable request.
Disclosure statement
No potential conflict of interest was reported by the author(s).
Additional information
Funding
Notes on contributors
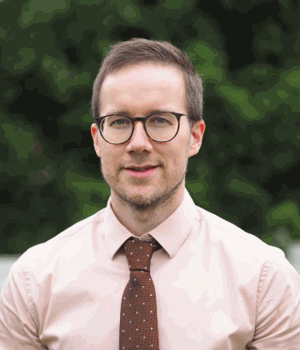
Jaakko Peltokorpi
Jaakko Peltokorpi is a Post-Doc Researcher at Aalto University, Department of Mechanical Engineering, in the Materials to Products (M2P) research group. He received a Ph.D. degree with the title ‘Worker Coordination, Collaboration and Learning in Make-to-order Assembly Production’ at Aalto University in 2018. Since then, he has been investigating and developing analytical learning curve models which also consider the cognitive load individuals encounter during assembly work. He is currently working in the ‘Green Factory – towards carbon neutral production (GREEF)’ project in the Sustainable Manufacturing Finland programme, funded by Business Finland.
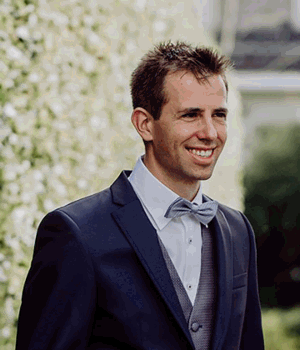
Steven Hoedt
Steven Hoedt is a Master of Science in automation engineering and a Ph.D. candidate at Ghent University. The focus of his Ph.D. studies in Industrial Engineering and Operations Research is mainly on the assessment of the learning and forgetting of manual assembly operators and how to tackle the forgetting effect via training or on-the-job support systems. In addition to his Ph.D. research, he coordinates various research projects that mainly focus on human-augmented technology in the manufacturing industry and is an active researcher within the FlandersMake@UGent-ISyE research group.
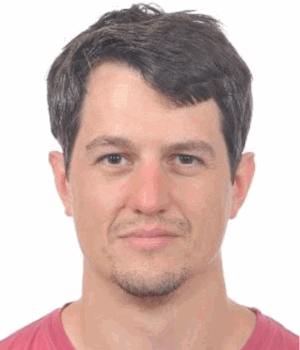
Thomas Colman
Thomas Colman is a Master of Science in electronics engineering and works at the data analytics team at Kion Mobile Automation. During and for this research project he has been developing an algorithm, commissioned by Arkite NV, that monitors the learning curve of a certain test person, determines a personal experience level, and adjusts the amount of information shown accordingly. By using his experience in practical research, he played a key role in setting up, conducting, and maintaining the physical field tests.
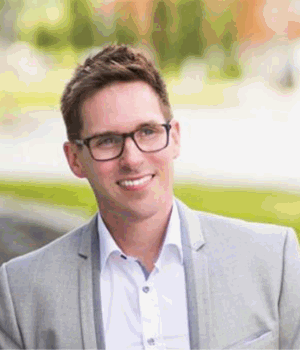
Kim Rutten
Kim Rutten has a Master’s degree in chemical engineering from the Technical University of Eindhoven in the Netherlands. After ten years of innovation engineering, he joined Arkite, a company specialising in Augmented Reality guidance systems for the manufacturing industry. Aside from proprietary 3D detection algorithms, he focused on mining operator performance data. He is currently working as a product manager for Machine Learning at Software AG. Leveraging machine learning capabilities for leading platforms for IIoT – Cumulocity IoT, for self-service analytics – TrendMiner, for streaming analytics – Apama and the Thin Edge revolution.
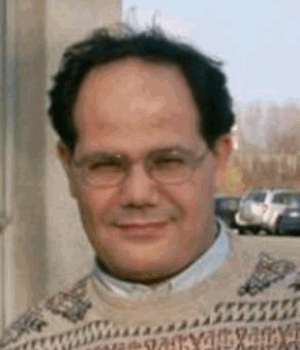
El-Houssaine Aghezzaf
El-Houssaine Aghezzaf is a professor of industrial systems engineering and operations research at the Faculty of Engineering and Architecture of Ghent University. He holds a Master of Science degree and a Ph.D. in applied mathematics and operation. He currently heads the department of Industrial Systems Engineering and Product Design and has an active role in Flanders Make, a strategic research centre in Flanders. His main research interests are in integrated optimisation and simulation approaches to the design, planning, and control problems arising in manufacturing systems and in logistical and utility networks.
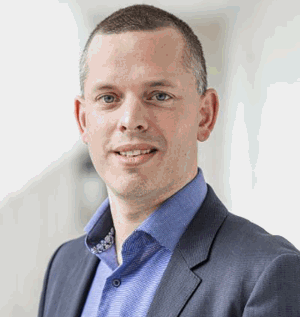
Johannes Cottyn
Johannes Cottyn is an assistant professor in industrial automation at Ghent University in the department of Industrial Systems Engineering (ISyE) and Product Design. He leads the FlandersMake@UGent-ISyE research group within the Flexible Assembly of Flanders Make research cluster. His initial research interests lie in the combination of industrial control and software systems (e.g. MES) and manufacturing excellence best practices (e.g. Lean). His current research activities are focused on human-augmented and digital-twin-enabled manufacturing.
Notes
1 HIM installed at Senzer (sheltered workplace in NL). Retrieved 21 February 2023, from https://www.youtube.com/watch?v=YF9Fbvejp-s.
2 Arkite Platform. Retrieved 21 February 2023, from https://www.arkite.com/platform/.
References
- Bai, Chunguang, Hua Zhou, and Joseph Sarkis. 2023. “Evaluating Industry 4.0 Technology and Sustainable Development Goals – A Social Perspective.” International Journal of Production Research, 1–21. doi:10.1080/00207543.2022.2164375.
- Bosch, Tim, Gu van Rhijn, Frank Krause, Reinier Könemann, Ellen S. Wilschut, and Michiel de Looze. 2020. “Spatial Augmented Reality.” In Proceedings of the 13th ACM International Conference on Pervasive Technologies Related to Assistive Environments, 1–7. New York: ACM. doi:10.1145/3389189.3397975.
- Clark, Ruth C, Frank Nguyen, and John Sweller. 2011. Efficiency in Learning: Evidence-Based Guidelines to Manage Cognitive Load. Hoboken, NJ: John Wiley & Sons.
- European Commission. 2021. Union of Equality: Strategy for the Rights of Persons with Disabilities 2021-2030 - Employment, Social Affairs & Inclusion - European Commission. European Union. doi:10.2767/31633.
- Fässberg, Tommy, Åsa Fasth, and Johan Stahre. 2012. “A Classification of Carrier and Content of Information.” Proceedings of the 4th CIRP Conference on Assembly Technologies and Systems, 1–4. http://cirp.me.engin.umich.edu/CIRP_CATS_2012_Proceedings_small.pdf.
- Funk, Markus, Andreas Bächler, Liane Bächler, Oliver Korn, Christoph Krieger, Thomas Heidenreich, and Albrecht Schmidt. 2015. “Comparing Projected In-Situ Feedback at the Manual Assembly Workplace with Impaired Workers.” In Proceedings of the 8th ACM International Conference on Pervasive Technologies Related to Assistive Environments, 1–8. New York: ACM. doi:10.1145/2769493.2769496.
- Funk, Markus, Sven Mayer, and Albrecht Schmidt. 2015. “Using In-Situ Projection to Support Cognitively Impaired Workers at the Workplace.” In Proceedings of the 17th International ACM SIGACCESS Conference on Computers & Accessibility - ASSETS ‘15, 185–192. New York: ACM. doi:10.1145/2700648.2809853.
- Furniss, Fred, Alison Ward, Giulio Lancioni, Nelson Rocha, Bernardo Cunha, Philip Seedhouse, Pedro Morato, and Neil Waddell. 1999. “A Palmtop-Based Job Aid for Workers with Severe Intellectual Disabilities.” Technology and Disability 10 (1): 53–67. doi:10.3233/TAD-1999-10106.
- Glock, Christoph H., Eric H. Grosse, Mohamad Y. Jaber, and Timothy L. Smunt. 2019. “Applications of Learning Curves in Production and Operations Management: A Systematic Literature Review.” Computers & Industrial Engineering 131: 422–441. doi:10.1016/j.cie.2018.10.030.
- Grund, Jonas, Moritz Umfahrer, Lea Buchweitz, James Gay, Arthur Theil, and Oliver Korn. 2020. “A Gamified and Adaptive Learning System for Neurodivergent Workers in Electronic Assembling Tasks.” In Mensch und Computer 2020 (MuC’20), 491–494. New York: ACM. doi:10.1145/3404983.3410420.
- Heinz, Mario, Sebastian Büttner, Sascha Jenderny, and Carsten Röcker. 2021. “Dynamic Task Allocation Based on Individual Abilities - Experiences from Developing and Operating an Inclusive Assembly Line for Workers with and without Disabilities.” Proceedings of the ACM on Human-Computer Interaction 5: 1–19. doi:10.1145/3461728.
- Hoedt, Steven, Arno Claeys, Hendrik van Landeghem, and Johannes Cottyn. 2017. “The Evaluation of an Elementary Virtual Training System for Manual Assembly.” International Journal of Production Research 55 (24): 7496–7508. doi:10.1080/00207543.2017.1374572.
- Ivanov, Dmitry. 2023. “The Industry 5.0 Framework: Viability-Based Integration of the Resilience, Sustainability, and Human-Centricity Perspectives.” International Journal of Production Research 61: 1683–1695. doi:10.1080/00207543.2022.2118892.
- Jaber, Mohamad, Y. Jaakko Peltokorpi, Christoph H. Glock, Eric H. Grosse, and Martin Pusic. 2021. “Adjustment for Cognitive Interference Enhances the Predictability of the Power Learning Curve.” International Journal of Production Economics 234: 108045. doi:10.1016/j.ijpe.2021.108045.
- Kelleher, Caitlin, and Wint Hnin. 2019. “Predicting Cognitive Load in Future Code Puzzles.” In Proceedings of the 2019 CHI Conference on Human Factors in Computing Systems, 1–12. New York: ACM. doi:10.1145/3290605.3300487.
- Kirschner, Paul A. 2002. “Cognitive Load Theory: Implications of Cognitive Load Theory on the Design of Learning.” Learning and Instruction 12 (1): 1–10. doi:10.1016/S0959-4752(01)00014-7.
- Korn, Oliver, Markus Funk, and Albrecht Schmidt. 2015. “Towards a Gamification of Industrial Production.” In Proceedings of the 7th ACM SIGCHI Symposium on Engineering Interactive Computing Systems, 84–93. New York: ACM. doi:10.1145/2774225.2774834.
- Korn, Oliver, Johannes Lang, Andreas Korge, Haris Causegic, and Albrecht Schmidt. 2016. “Gamification of a Workday: A Study on the Effects in Sheltered Employment.” In CHI '16 Extended Abstracts on Human Factors in Computing Systems, 3114–3121. San Jose, CA: ACM. doi:10.1145/2851581.2892283.
- Korn, Oliver, Albrecht Schmidt, and Thomas Hörz. 2013. “The Potentials of In-Situ-Projection for Augmented Workplaces in Production.” In CHI ‘13 Extended Abstracts on Human Factors in Computing Systems, 979–984. New York: ACM. doi:10.1145/2468356.2468531.
- Kosch, Thomas, Markus Funk, Albrecht Schmidt, and Lewis L. Chuang. 2018. “Identifying Cognitive Assistance with Mobile Electroencephalography.” Proceedings of the ACM on Human-Computer Interaction 2 (EICS): 1–20. doi:10.1145/3229093.
- Lancioni, Giulio E., Nirbhay N. Singh, Mark F. O’Reilly, Vanessa A. Green, Doretta Oliva, and Francesca Campodonico. 2013. “Two Men with Multiple Disabilities Carry out an Assembly Work Activity with the Support of a Technology System.” Developmental Neurorehabilitation 16 (5): 332–339. doi:10.3109/17518423.2012.736419.
- Lancioni, Giulio E., Nirbhay N. Singh, Mark F. O’Reilly, Jeff Sigafoos, Gloria Alberti, Viviana Perilli, Dominga Laporta, Francesca Campodonico, Doretta Oliva, and Jop Groeneweg. 2014. “People with Multiple Disabilities Learn to Engage in Occupation and Work Activities with the Support of Technology-Aided Programs.” Research in Developmental Disabilities 35 (6): 1264–1271. doi:10.1016/j.ridd.2014.03.026.
- Li, Dan, Sandra Mattsson, Omkar Salunkhe, Åsa Fast-Berglund, Anders Skoogh, and Jesper Broberg. 2018. “Effects of Information Content in Work Instructions for Operator Performance.” Procedia Manufacturing 25: 628–635. doi:10.1016/j.promfg.2018.06.092.
- Lindsay, Sally, Elaine Cagliostro, Mikhaela Albarico, Neda Mortaji, and Leora Karon. 2018. “A Systematic Review of the Benefits of Hiring People with Disabilities.” Journal of Occupational Rehabilitation 28 (4): 634–655. doi:10.1007/s10926-018-9756-z.
- Longo, Francesco, Antonio Padovano, and Steven Umbrello. 2020. “Value-Oriented and Ethical Technology Engineering in Industry 5.0: A Human-Centric Perspective for the Design of the Factory of the Future.” Applied Sciences 10 (12): 4182. doi:10.3390/app10124182.
- Mark, Benedikt G., Sarah Hofmayer, Erwin Rauch, and Dominik T. Matt. 2019. “Inclusion of Workers with Disabilities in Production 4.0: Legal Foundations in Europe and Potentials through Worker Assistance Systems.” Sustainability 11 (21): 5978. doi:10.3390/su11215978.
- Mark, Benedikt G., Erwin Rauch, and Dominik T. Matt. 2022. “Systematic Selection Methodology for Worker Assistance Systems in Manufacturing.” Computers & Industrial Engineering 166: 107982. doi:10.1016/j.cie.2022.107982.
- Mayer, Richard E. 2011. “Multimedia Learning and Games.” In Computer Games and Instruction, edited by Sigmund Tobias, and J. D. Fletcher, 281–305. Charlotte, NC: IAP Information Age Publishing.
- Moghaddam, Fatemeh Besharati, Angel J. Lopez, Stijn de Vuyst, and Sidharta Gautama. 2021. “Operator’s Experience-Level Classification Based on Micro-Assembly Steps for Smart Factories.” 2021 IEEE 8th International Conference on Industrial Engineering and Applications (ICIEA): 142–148. doi:10.1109/ICIEA52957.2021.9436710.
- Peltokorpi, Jaakko, and Mohamad Y. Jaber. 2022. “An Interference-Adjusted Power Learning Curve for Tasks with Cognitive and Motor Elements.” Applied Mathematical Modelling 101: 157–170. doi:10.1016/j.apm.2021.08.016.
- Peltokorpi, Jaakko, and Esko Niemi. 2019. “Effects of Group Size and Learning on Manual Assembly Performance: An Experimental Study.” International Journal of Production Research 57 (2): 452–469. doi:10.1080/00207543.2018.1444810.
- Petzoldt, Christoph, Dennis Keiser, Thies Beinke, and Michael Freitag. 2020. “Functionalities and Implementation of Future Informational Assistance Systems for Manual Assembly.” International Conference on Subject-Oriented Business Process Management 1278: 88–109. doi:10.1007/978-3-030-64351-5_7.
- Sauer, Angela L., Andra Parks, and Patricia C. Heyn. 2010. “Assistive Technology Effects on the Employment Outcomes for People with Cognitive Disabilities: A Systematic Review.” Disability and Rehabilitation: Assistive Technology 5 (6): 377–391. doi:10.3109/17483101003746360.
- Schein, Katrin, and Philipp Rauschnabel. 2022. “Augmented Reality in Manufacturing: Exploring Workers’ Perceptions of Barriers.” IEEE Transactions on Engineering Management, 1–14. doi:10.1109/TEM.2021.3093833.
- Seok, Soonhwa, Edward L. Meyen, and Boaventura DaCosta. 2010. Handbook of Research on Human Cognition and Assistive Technology: Design, Accessibility and Transdisciplinary Perspectives: Design, Accessibility and Transdisciplinary Perspectives. New York: IGI Global.
- Statista. 2020. “Number of Employees in the European Union (EU27) and United Kingdom as of 3rd Quarter 2020 (in 1,000s), by Sector.” https://www.statista.com/statistics/1195197/employment-by-sector-in-europe/.
- Swanson, H. Lee, and Linda Siegel. 2001. “Learning Disabilities as a Working Memory Deficit.” Issues in Education: Contributions from Educational Psychology 7 (1): 1–48.
- Sweller, John. 1988. “Cognitive Load during Problem Solving: Effects on Learning.” Cognitive Science 12 (2): 257–285. doi:10.1016/0364-0213(88)90023-7.
- Sweller, John. 2011. “Cognitive Load Theory.” In Psychology of Learning and Motivation, edited by Jose P. Mestre, and Brian H. Ross, Vol. 55, 37–76. Academic Press. doi:10.1016/B978-0-12-387691-1.00002-8.
- Tsutsumi, Daisuke, Dávid Gyulai, Emma Takács, Júlia Bergmann, Youichi Nonaka, and Kikuo Fujita. 2020. “Personalized Work Instruction System for Revitalizing Human-Machine Interaction.” Procedia CIRP 93: 1145–1150. doi:10.1016/j.procir.2020.04.062.
- Vanneste, Pieter, Yi Huang, Jung Yeon Park, Frederik Cornillie, Bart Decloedt, and Wim van den Noortgate. 2020. “Cognitive Support for Assembly Operations by Means of Augmented Reality: An Exploratory Study.” International Journal of Human-Computer Studies 143: 102480. doi:10.1016/j.ijhcs.2020.102480.
- Wright, Theodore P. 1936. “Factors Affecting the Cost of Airplanes.” Journal of the Aeronautical Sciences 3 (4): 122–128. doi:10.2514/8.155.
Annex A
Principles, their explanations, and related guidelines to handle various types of cognitive load (Clark, Nguyen, and Sweller Citation2011; Li et al. Citation2018).