Abstract
Throughput analysis plays an important role in the operations and management of automotive manufacturing. Predicting how the system throughput changes over time helps the plant managers to make timely operational decisions to meet the daily production requirement. In today’s automotive production systems, the availability of sensing data reflecting process variables and machine status across the plant floor raises new opportunities for improving the throughput prediction accuracy. However, challenges exist in extracting the most important features from the high-dimensional data and capturing the complicated time-vary interdependency among different assets in the system. To overcome such challenges, in this paper we propose a hierarchical Recurrent Neural Network (RNN)-based framework that is composed of clustering, dimension reduction and feature selection, regression, and prediction pruning/adjustment. In addition to predicting end-of-line throughput, our framework then identifies the associations for low throughput to facilitate downtime prevention and maintenance decision-making. The proposed framework is applied to an automotive production system, and its effectiveness is demonstrated by comparison with conventional methods.
Disclosure statement
No potential conflict of interest was reported by the author(s).
Data availability statement
Due to commercial restrictions, participants of this study did not agree for their data to be shared publicly, so supporting data is not available.
Additional information
Funding
Notes on contributors

Mengfei Chen
Mengfei Chen received the B.S. degree in aerospace engineering from Nanjing University of Aeronautics and Astronautics, Nanjing, China, in 2019. She is currently working toward the Ph.D. degree in industrial and systems engineering at Rutgers University, Piscataway, NJ, U.S.A. Her research interests include data-driven optimisation and physics-informed machine learning with applications to predictive quality in manufacturing systems and supply chain network design.
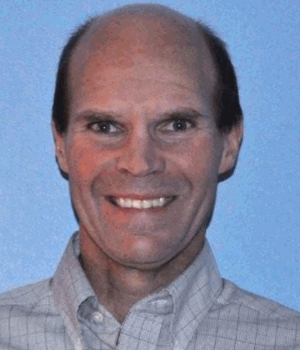
Richard Furness
Richard Furness received the Ph.D. degree in mechanical engineering from the University of Michigan, Ann Arbor, MI, U.S.A. in 1992. He is a retired global manager at Ford Motor Company. He is a highly experienced engineer and people leader with an extensive record of driving engineering and technology development, and strategic business and manufacturing process innovations to improve cost, throughput, quality, and efficiency.
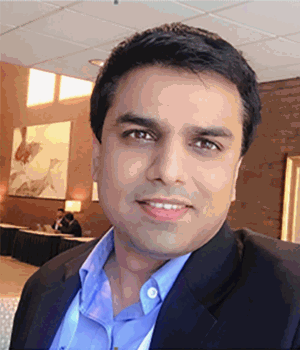
Rajesh Gupta
Rajesh Gupta has overall 15+ years of research andindustry experience in various domain including manufacturing, automotive, digital marketing, and pharmaceutical industry. He has earned his PhD degree in Mathematical Statistics from Lucknow University in 2008. From last couple of years, he is actively involved in Automotive Manufacturing and Transportation research work. He has 10+ publications in reputed journals.
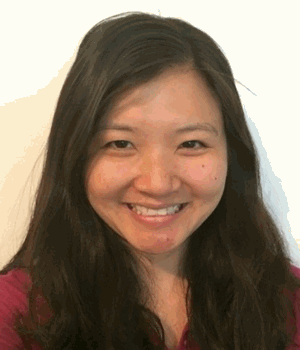
Saumuy Puchala
Saumuy Puchala works in Maintenance Analytics at the Global Data Insight and Analytics organization at Ford Motor Company. She has a BSE, MSE and PhD in Industrial Engineering from the University of Michigan-Ann Arbor. Her previous roles at Ford included lean manufacturing, throughput simulations and manufacturing technology development. In her current role, she leads a team that develops artificial intelligence and machine learning algorithms that are deployed in applications that enable inter-connected, smarter IIoT maintenance systems.
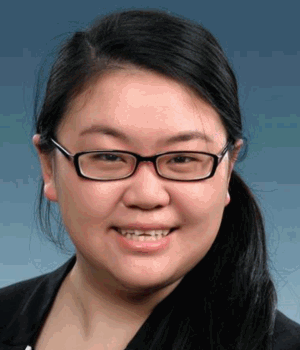
Weihong (Grace) Guo
Weihong (Grace) Guo received the B.S. degree in industrial engineering from Tsinghua University, Beijing, China, in 2010, and the M.S. and Ph.D. degrees in industrial and operations engineering from the University of Michigan, Ann Arbor, MI, U.S.A., in 2012 and 2015, respectively. She is currently an Associate Professor with the Department of Industrial and Systems Engineering, Rutgers University New Brunswick, NJ, U.S.A. Her research focuses on manufacturing data analytics, process monitoring, anomaly detection, quality evaluation, and system informatics. Dr. Guo is a member of the Institute of Industrial and Systems Engineers (IISE), the Institute for Operations Research and the Management Sciences (INFORMS), the American Society of Mechanical Engineers (ASME), the IEEE Robotics and Automation Society, and Tau Beta Pi.