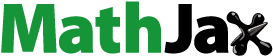
Abstract
Inventory planning in fashion markets is highly challenging, owing to uncertain demand; yet, in making inventory decisions, retailers may be able to capitalise on high substitutability between products. This research develops single-period inventory-management models describing a market with two substitutable products, under stockout-based substitution; i.e. when a customer’s preferred product is out-of-stock, s/he may choose to purchase the substitute. Two settings are considered: centralised (a single retailer who sells both products) and competitive (two retailers, each selling one product). For each setting, we derive closed-form analytical solutions for the inventory levels that maximise expected profit. The model is further enriched with sales data from an online apparel retailer offering substitutable products (a sneaker in different colours), and we analyse the sensitivity of the optimal inventory levels and profits to parameter values. Key findings include the following: (i) Under competitive conditions, both retailers always order positive inventory so as not to lose customers. However, in a single-retailer setting, there are situations in which the retailer orders inventory for only one product. (ii) The optimal inventory levels and corresponding profits are highly sensitive to consumers’ willingness to substitute between products. These findings provide concrete insights that can guide fashion brands’ inventory-management decisions.
1. Introduction
The fashion market is characterised by dynamic and volatile demand conditions, in which new products are released every sales period, rendering older merchandise obsolete – yet, at the same time, the desirability of new collections, and thus the demand for the corresponding products, is uncertain. These conditions make it challenging for retailers to successfully plan their inventories, i.e. to determine the quantity and assortment (e.g. colours and patterns) of items to offer in each sales period (Ho et al. Citation2015; Wang et al. Citation2022). In making such decisions, retailers must further consider another key feature of fashion markets: the high potential for substitutability between products. For example, fashion products are often manufactured in a variety of colours, meaning that if a specific garment that a consumer wishes to buy is out of stock, they might switch and buy the same garment in a different colour (Ergin, Gümüş, and Yang Citation2022).
A consumer’s sensitivity to substitutability may determine the extent to which he or she is likely to engage in such substitution (Caro and Gallien Citation2010; Zhang et al. Citation2020). Willingness to accept substitutes may be particularly high in fast fashion markets, where collections change rapidly over a finite season (Schlapp and Fleischmann Citation2018). In these markets, when consumers are looking for a specific product but find that it is out of stock, they may be faced with a choice between purchasing the product in a different colour or not purchasing it at all – as the product is unlikely to return (Choi and Guo Citation2018).
Herein, taking these considerations into account, we study optimal inventory decisions of fashion retailers aiming to maximise their expected profits. Our work comprises two main components: an analytical modelling component and an empirical study. For the analytical component, we model a market in which two substitutable fashion products are sold. We examine two scenarios, each of which we describe with a single-period inventory model: The first scenario is a centralised setting in which a single retailer offers both substitutable products. Each customer has a preferred product, and, if that product is out of stock, the customer may either purchase the substitute (from the same retailer) or, alternatively, buy nothing. The second scenario is a competitive setting with two competing retailers, each of whom sells one of the two substitutable products. When a customer’s preferred product is out of stock, the customer may choose to buy the substitutable product from the competing retailer or not buy any product at all. In each model, on the basis of a set of given parameter values (e.g. the customer’s willingness to substitute one product for the other, the price and unit cost of each product, etc.), the retailer(s) choose the inventory level(s) that maximise profits. Our work extends the inventory model of Koren, Perlman, and Shnaiderman (Citation2022), which considered only the second of the two scenarios we describe, namely, a competitive setting with two substitutable products, each offered exclusively by a different retailer. We investigate how this scenario compares to the centralised setting in terms of the optimal inventory level for each product, as well as the corresponding profits. This comparison can provide insights into how inventory management strategies might differ between a retailer that is an exclusive carrier of a particular product (e.g. a designer shop or even a chain retailer, such as The Gap, selling its own branded items) versus a retailer that carries products that are available through multiple competing retailers (e.g. products manufactured by national or global brands such as Nike or Adidas).
Next, in the empirical component of this research, we derive the actual demand distributions of two substitutable fashion products offered by a fast-fashion retailer (in our model, the joint demand distribution is represented by a general function). Then, given these demand distributions, as well as other real-world data regarding product prices, we examine how the optimal inventory levels and corresponding expected profits are affected by three parameters: substitution rate (customers’ willingness to purchase a substitute when their preferred product is out of stock), unit selling price, and the correlation between the demand levels of the two products.
The main contributions of this paper are as follows: (i) Closed-form analytical solutions are obtained for optimal inventory levels in centralised and competitive settings; in both cases, we prove that the objective functions are concave, so that a unique optimal solution exists. (ii) We estimate the joint probability of the demand distribution based on data from an online apparel retailer regarding the sales of two substitutable products. (iii) We characterise the impact of stockout-based substitution rate on optimal inventory levels and profits under centralised and competitive cases. A nonintuitive result is the finding that when customers’ flexibility regarding substitution of a particular product is low, the optimal inventory order size of that product is larger in the case of a single retailer than in the competitive setting. The models we develop, as well as the findings of our analysis, can be of value to fashion retailers seeking to plan their inventories in an uncertain market.
2. Literature review
2.1. Background: inventory management in the fashion industry
In general, the main objective of inventory management is to ensure that demand is met in a manner that minimises profit loss – so as to avoid being left with a surplus of unsold goods at the end of the season on the one hand, while preventing a shortage of popular items due to excessive demand (Shen et al. Citation2016). In the fashion industry, effective inventory management entails a complex process of identifying customers’ future tastes and preferences, e.g. by tracking factors that might influence these preferences (Ambilkar et al. Citation2022; Ko, Costello, and Taylor Citation2019; Singh and Verma Citation2018). On the basis of this information about consumer preferences, coupled with information regarding the current state of the market, as well as historical data (which is commonly used in trend forecasting; see Loureiro, Miguéis, and da Silva Citation2018), designers and manufacturers decide which items and how many to produce, and retailers decide what to stock (DuBreuil and Lu Citation2020; Shokouhyar et al. Citation2021; Yamazaki, Shida, and Kanazawa Citation2016). Yet, ultimately, decisions about the production and stocking of multitudes of new designs are based on incomplete information that cannot provide a definitive indication of products’ future success (Ren, Chan, and Siqin Citation2020; Wen, Choi, and Chung Citation2019).
Using historical data for the purpose of trend forecasting is a common tool (Loureiro, Miguéis, and da Silva Citation2018). In the empirical portion of our study, we similarly incorporate sales history to obtain an understanding of how the demand for two substitutable products is expected to behave.
2.2. Inventory management models with product substitution
Starting from a seminal paper written by Parlar (Citation1988), extensive research has been devoted to modelling various inventory management problems in the presence of product substitution. A review by Shin et al. (Citation2015) identifies four criteria according to which models in this research stream can be classified. The first criterion is the modelling objective and, more broadly, the type of problem being addressed – e.g. assortment planning, inventory decisions, capacity planning, or pricing decisions. A second criterion identified by Shin et al. (Citation2015) is the identity of the party who initiates the substitution (the ‘decision-maker’). Specifically, substitution can be initiated either by the firm (as in the case of a company that manufactures a high-end product and decides to offer a similar, lower-end product to a different customer segment) or by the customer (e.g. when a customer faced with multiple substitutable alternatives selects one based on preference). The third criterion relates to the direction of substitutability: one-way (in which product A can be substituted for product B but not vice versa), or two-way (in which products can be substituted in either direction). The fourth criterion is the mechanism of substitution, which, according to Shin et al. (Citation2015), can fall into three main categories: (i) price-based substitution, in which a customer generally prefers a particular product but may select an alternative product because it is cheaper; (ii) assortment-based substitution, in which a store does not carry the customer’s preferred product, and the customer may decide to substitute a product that the store does carry (Martínez-de-Albéniz and Kunnumkal Citation2022); and (iii) inventory stockout-based substitution, in which, when the customer’s preferred product is out of stock, he or she can buy a substitutable product. Transchel (Citation2017) further refers to assortment-based substitution as ‘static’ substitution, as, in this scenario, the consumer’s preferred item is not included in the retailer’s predetermined, static assortment, and the consumer must decide whether to substitute it with an alternative; see Hübner (Citation2017) and Kök, Fisher, and Vaidyanathan (Citation2015). In turn, Transchel (Citation2017) refer to inventory-based substitution as ‘dynamic’, because in this scenario, the consumer’s preferred item is typically included in the retailer’s assortment, but is currently not available (e.g. Chen and Chao Citation2020; Goyal, Levi, and Segev Citation2016; Honhon, Gaur, and Seshadri Citation2010). Stockout-based (dynamic) substitution has been explored in diverse types of inventory-related problems, including pricing decisions in the presence of store capacity constraints (Gümüş, Kaminsky, and Mathur Citation2016), inventory routing and transshipment decisions (Achamrah, Riane, and Limbourg Citation2022; Li and Li Citation2018), inventory distribution processes (Caro and Gallien Citation2010; Wang and Zhang Citation2021), inventory management (Transchel et al., 2017; Perlman Citation2019), and production scheduling (Zeppetella et al. Citation2017).
Notably, some studies modelling inventory management may fall into multiple categories for a particular criterion in Shin et al.’s (Citation2015) taxonomy. In particular, some simultaneously consider multiple types of inventory-related decisions – e.g. inventory quantity and pricing – and, in some cases, multiple forms of substitution. Examples include the works of Besbes and Sauré (Citation2016) Zhao and Zhao (Citation2016), who modelled a scenario in which retailers compete in both assortment and prices and must choose which product assortment to offer, given display constraints. Dong et al. (Citation2020) developed an inventory-pricing model in which a retailer who offers two substitutable products seeks to select the prices and inventory levels for those products so as to maximise expected profits. Recently, Hammami et al. (Citation2022) studied inventory decisions for a retailer offering substitutable products that differ in delivery time and price, under hybrid distribution (i.e. rapid delivery from the retailer’s stock versus slower delivery via drop shipping).
According to the taxonomy described above, the models developed in the current research can be categorised as inventory planning (i.e. order quantity) decision models under dynamic, inventory stockout-based substitution, in which the customer initiates the substitution decision, and substitutability is bidirectional. Specifically, this research develops single-period inventory-management models. Similarly to Parlar (Citation1988), we seek to identify optimal order quantities in a market with two substitutable products. However, Parlar (Citation1988) assumed that the demand for each product is independent of the demand for the other product, while we consider a general joint probability density function of the demand. Our model is further enriched with sales data from an online apparel retailer offering substitutable products (a sneaker in different colours). In a numerical analysis based on these data, we are able to fit a specific joint demand function, estimate the correlation between the demand level of the two products, and perform sensitivity analysis of the optimal inventory levels and profits to this parameter.
As noted in the introduction, our study addresses both a centralised scenario of product substitution – in which consumers accept product substitutes offered by the same retailer – and a scenario in which a decision to accept a substitute for an out-of-stock product entails a decision to patronise a different store. Prior studies have examined either the former or the latter. For example, Li et al. (Citation2023) examined how demand spills over to substitute products (specifically, products of different sizes) offered by the same retailer. Ergin, Gümüş, and Yang (Citation2022), in turn, examined the dynamics of substitution across stores, and found that the likelihood of such substitution is strongest when customers first observe the lack of stock, and decreases over time. Ovezmyradov and Kurata (Citation2019) modelled customers’ responses to the stockout of fashion products and showed how a retailer can maximise profit by offering substitutable products at multiple stores. Other models considered two phases of selection; the consumer first chooses where to shop (i.e. brick and mortar store or online store), and subsequently selects the product (e.g. Gupta, Ting, and Tiwari Citation2019; Wan et al. Citation2018). To our knowledge, our work is among the few to compare how, in the presence of stockout-based substitution, optimal inventory decisions in a multi-retailer setting differ from those in a centralised setting.
3. Models
In what follows, we develop and analyse two separate inventory models representing different scenarios in a market in which two substitutable products are sold (product 1 and product 2), and substitution is of a dynamic inventory stockout-based nature. That is, a customer may choose to substitute one product for the other only in the event that her preferred product is out of stock. In each model, a retailer (or retailers) must set the inventory level(s) of the product(s) they offer so as to maximise expected profit. The first model (subsection 3.1) focuses on a centralised setting, in which a single retailer sells both substitutable products. Such centralisation can occur in cases in which a retailer sells its own branded products that are not available through competing retailers, or, as suggested by Parlar (Citation1988), in cases in which one retailer takes over another, or establishes a collaboration with another retailer. In the latter arrangement, neither retailer incurs any penalties if the ‘competitor’ fulfils the demand of the other retailer. The second model (subsection 3.2), based on the work of Koren, Perlman, and Shnaiderman (Citation2022), examines a competitive setting with two retailers, each offering only one of the two products. In subsection 3.3, the two models are compared, and we examine the impact of customers’ substitution rate on the retailers’ optimal inventory levels.
The notations and the decision variables used in the models are as follows:
i – indicator for a specific product, i ε {1,2}; in the two-retailer model, the term ‘retailer i’ denotes the retailer that offers product i.
Qi – Inventory level of product i (a decision variable)
Xi – Demand for product i (a random variable with realisation xi)
f – The joint probability density function of X1 and X2
pi – Unit selling price of product i
ci – Order cost of product i
si – Salvage value of product i
δi – The stockout-based substitution rate, defined as customers’ probability (i.e. their willingness) to buy product 3-i if product i is out of stock, and given that product 3 – i is available in the market.
π – Retailer’s profit; in the two-retailer model, πi denotes the profit of retailer i.
3.1. Inventory model for a single retailer
Consider a single retailer who sells both types of substitutable products, i.e. product 1 and product 2.
The retailer, who orders all inventory prior to the selling period, seeks to choose optimal order quantities and
that maximise her profit π, given the values of the other variables. See sequence of events in Figure .
Figure 1. Sequence of events for centralised setting.
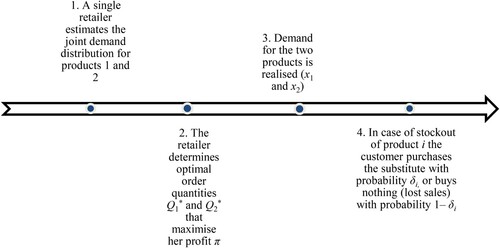
3.1.1. Profit outcomes for different values of inventory and demand
We first identify four general scenarios regarding the retailer’s profit (a random variable) for specific values of the inventory levels Q1 and Q2, defined in relation to the random demand levels X1 and X2.
If X1≤ Q1 and X2≤ Q2, each customer receives his or her preferred product, and the retailer's profit is
(1)
(1)
If X2> Q2 and X1≤ Q1–δ2(X2 – Q2), the inventory of product 2 does not meet the demand for that product. On the other hand, the inventory level of product 1 satisfies both the original demand for that product and the demand among customers who prefer product 2 but find that it is unavailable, and are willing to buy product 1 instead. Thus, the retailer’s profit is:
(2)
(2)
Similarly, if X1> Q1 and X2≤ Q2–δ1(X1 – Q1),
(3)
(3)
Otherwise, all the inventory is sold, and the profit is equal to
(4)
(4)
3.1.2. Expected profit maximisation
Based on (1)–(4), the retailer's expected profit is
(5)
(5)
To maximise the expected profit Z, we solve the following problem:
(6)
(6)
In Lemma 3.1 below, it is indicated that under the following conditions
(7)
(7)
Problem (6) is concave. One of the first two terms of (7) is necessarily satisfied. It is reasonable to assume that the other is also met, as the two products are of similar types, and, therefore, the ratios {p1/p2, p2/p1} are often close to 1.
Lemma 3.1:
The objective function (5) is strictly concave in [0,∞)×[0,∞) under the assumptions (7).
Proof:
See Appendix A.
According to Lemma 3.1, problem (6) is concave. Consider the following two conditions:
(8)
(8)
as well as
(9)
(9)
Since the right-hand side of (8) is the reciprocal of the right-hand side of (9), at least one of them is greater than or equal to 1. Thus, it is necessary for at least either (8) or (9) to be satisfied. Note that pi – ci is the margin the retailer gains from selling product i. If, for example, p1 – c1 > p2 – c2 the margin from product 1 is higher than the margin from product 2, so that (8) is always satisfied.
It will now be shown that if both conditions (8) and (9) are met, then the retailer orders products of both types to maximise the expected profit (Proposition 3.1). On the other hand, if one condition is not satisfied, it is optimal to order products of only one type (Proposition 3.2 below).
Proposition 3.1:
Given that both (8) and (9) are met, the solution of (6), denoted by (Q1*,Q2*), satisfies the following equations:
(10)
(10)
Furthermore, both Q1* and Q2* are positive.
Proof:
See Appendix A.
We now consider the case in which either (8) or (9) is not met. Consider inequality (8). The difference p1 – c1, is the retailer's net profit from a customer who prefers product 1 and receives that product, whereas δ1(p2 – c2), is equivalent to the retailer's expected net profit from a customer who prefers product 1 and does not receive it, but is willing and able to buy product 2 (i.e. product 1 is stocked out whereas product 2 exists in the inventory). When the inequality of (8) is satisfied, the retailer is motivated to hold a positive inventory level of product 1, so that customers receive their first-choice product. However, if (8) is not met, it is profitable for the retailer to forgo offering product 1 and instead to sell product 2 to the share δ1 of product 1’s customers who are willing to purchase product 2. Hence, the optimal inventory level of product 1 is equal to zero. According to a similar logic, if (9) is not met, then the retailer carries only product 1 and does not hold a positive inventory level of product 2. In other words, from a practical perspective, the retailer is better off ordering inventory of only a single product when the margin of that product is higher than the expected margin gained due to stockout-based substitution. From a mathematical perspective, the following proposition holds:
Proposition 3.2:
(i) If condition (9) is not satisfied, then Q2* = 0, and Q1* > 0 such that
(11)
(11) (ii) If condition (8) is not met, then Q1* = 0, and Q2* > 0 such that
(12)
(12)
Proof:
See Appendix A.
Note that the reasoning underlying Proposition 3.2 does not apply in a competitive setting, in which a retailer who runs out of a customer’s preferred product cannot gain any profit from that customer – as the customer will choose to purchase a substitute (if at all) from a competing retailer. We discuss this case in detail in section 3.2.
3.1.3. Characterisation of the optimal order quantities and expected profit as a function of parameter values
Proposition 3.3:
For every , the optimal order quantity Qi* increases in δ3-i. If for every xi ≥ 0, the joint density function f(x1,x2) decreases in x3-i, then Qi* decreases in δi.
Proof:
See Appendix A.
In practice, if a customer is willing to substitute and buy his or her less-preferred product, it is not necessary for the retailer to hold a high level of inventory of the more-preferred product. We note that the assumption in Proposition 3.3 that f(x1,x2) decreases in x3-i is a sufficient condition for Qi* to decrease in δi. However, there are conditions under which the optimal order quantity of product i decreases in δi even when this assumption is not met (see section 4.3.1 for examples). Appendix A provides a formal characterisation of these conditions.
Regarding the retailer’s expected profit, we expect Z to increase in each of the substitution rates δi. From a practical perspective, a higher value of a stockout-based substitution rate δ1 or δ2 indicates that customers are more flexible, meaning that the retailer is more likely to be able to gain profit from customers regardless of their product preferences. Formally:
Proposition 3.4:
The expected profits of the retailer increase in both δ1 and δ2.
Proof:
See Appendix A.
According to (10), the optimal order quantities depend on the ratios and
. Moreover, according to (10), the optimal order quantities are affected by the ratio
, as long as both (8) and (9) are satisfied; namely, both Q1* and Q2* are positive. To satisfy the first two terms of (7), we assume that
Proposition 3.5:
Let ,
and
.
The optimal order quantity Q1* increases in rp for fixed values of r1 and r2.
The optimal order quantity Q2* decreases in rp for fixed values of r1 and r2.
Proof:
See Appendix A.
The retailer's profit from each unit sold is the difference between selling price and salvage value, which determines whether it is profitable to sell the product. The ratio between these two terms () determines the profitability of a product. Clearly, a retailer will increase inventory levels when a product is more profitable.
3.2. Inventory model for two competing retailers
Here, in line with Koren, Perlman, and Shnaiderman (Citation2022), we consider a scenario with two substitutable products, but in this case, each product is offered by a different (competing) retailer. In the competitive scenario, the retailers both order their inventory simultaneously, prior to the start of the selling period (i.e. before demand realisation). Each retailer i aims to order the inventory level Qi that maximises his or her expected profit, defined below. The sequence of events is summarised in Figure .
Figure 2. Sequence of events for competitive setting.
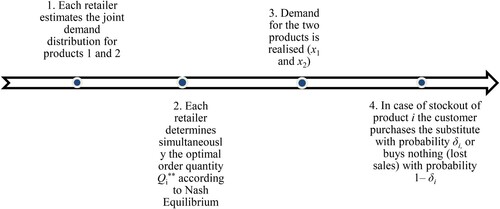
3.2.1. Profit outcomes for different values of inventory and demand
As in the centralised case, we first identify four general profit outcome scenarios for specific values of the inventory levels Q1 and Q2, defined in relation to the random demand levels X1 and X2.
If X1≤ Q1 and X2≤ Q2, each customer purchases the product that he or she prefers, and the corresponding profit for retailer i is
(13)
(13)
If X2> Q2 and X1≤ Q1–δ2(X2 – Q2), then retailer 2’s inventory does not meet the demand for product 2, whereas retailer 1’s inventory level satisfies both the demand for product 1 and the demand among customers who prefer product 2 yet found that it was unavailable and are willing to buy product 1 instead. Thus, each retailer’s profit is as follows:
(14)
(14)
According to the same logic, if X1 > Q1 and X2 ≤ Q2 – δ1(X1 – Q1), then
(15)
(15)
Otherwise, all the inventory is sold and the profit of retailer i is equal to
(16)
(16)
3.1.2. Expected profit maximisation
Let Zi(Qi| Q3-i) denote the total expected profit of retailer i, given Q3-i. According to (13)–(16), Z1 and Z2 can be expressed as follows:
(17)
(17)
and
(18)
(18)
Recall that the two retailers order their products simultaneously, prior to demand realisation. Thus, they set inventory levels Q1** and Q2** that maximise their expected profits, according to the following Nash equilibrium:
(19)
(19)
Lemma 3.2:
The functions Z1(Q1|Q2) and Z2(Q2|Q1) are concave in Q1 and in Q2, respectively.
Proof
: See Appendix A.
In the following Proposition, it is shown that there is a unique Nash solution for the optimal inventory levels. According to Lemma 3.2, and similarly to the case in Parlar (Citation1988), we have shown in Appendix A (A38) that the derivative of retailer 1’s reaction curve is always strictly smaller than the derivative of retailer 2’s reaction curve.
Proposition 3.6:
There exist unique optimal inventory levels Q1** > 0 and Q2** > 0, which are obtained by solving the following equations:
(20)
(20)
Proof:
See Appendix A.
Proposition 3.6 stipulates that each retailer orders a positive inventory level of his or her product; this proposition makes intuitive sense, given that a retailer cannot make a profit without ordering any inventory of the product s/he sells.
3.2.3. Characterisation of the optimal order quantities as a function of the substitution rates
Proposition 3.7:
For every , the optimal order quantity Qi** decreases in δi and increases in δ3-i.
Proof:
See Appendix A.
It indeed seems plausible that the optimal order quantity Qi** should grow when δ3-i increases, as an increase in δ3-i implies that more customers of retailer 3 – i are likely to be willing to purchase from retailer i in the event that their preferred product is unavailable. Proposition 3.7 further stipulates that as δi increases, Qi** decreases. This phenomenon results, in part, from the fact that an increase in δi triggers an increase in Q3-i**, which means that fewer customers who prefer product 3 – i will face stockouts and patronise retailer i. Thus, from a practical perspective, the decrease in Qi** associated with an increase in δi should cover the loss of customers who switch from product 3 – i, but it should not be so great that it prevents retailer i from fulfilling the demand of customers who prefer product i. Accordingly, the negative effect of δi on the optimal order quantity Qi** is not expected to be substantial – as we indeed show in our numerical study in subsection 4.3 below (see Figures a and b).
Figure 3. Effect of δ1 on the optimal inventory levels Q1 (a) and Q2 (b).
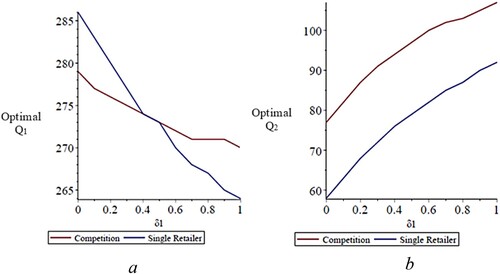
3.3. Comparison of the centralised and competitive models
It can be assumed, without loss of generality, that p1 – c1 > p2 – c2 (i.e. product 1 is more profitable and (8) is always satisfied). In what follows, we show that for small values of δ1, the optimal inventory level of product 1 in the single-retailer model is greater than that corresponding to the competition model. On the other hand, the optimal inventory level of product 2 is higher in the case of competition.
Proposition 3.8:
When δ1 is low and close to 0, then Q1* > Q1** and Q2* < Q2**.
Proof:
See Appendix A.
Proposition 3.8 can be explained according to the following logic. If δ1 is small and close to 0, then customers who prefer product 1 are generally unwilling to buy product 2 in the event of a stockout of product 1. Recall that we assume that the profit from product 1 is greater than the profit from product 2. Ordering a quantity of product 1 inventory is always preferable; therefore, Q1 is significantly greater in a single-retailer setting than in a competitive setting, i.e. Q1* > Q1**. On the other hand, Q2 is significantly greater in a competitive setting than in a single retailer (centralised) setting, i.e. Q2* < Q2**.
It will now be informally demonstrated that when δ1 is large and close to 1, the optimal order quantity of product 2 is greater under the competition model than under the centralised model. In turn, the optimal order quantity of product 1 depends on the (fixed) value of δ2.
Let δ1 = 1 and 0 ≤ δ2 ≤ (p2 – c2)/(p1-c1). From (20) and (10) we obtain, respectively,
(21)
(21)
And
(22)
(22)
According to (21) and (22), Q1*(1, δ2) and Q2*(1, δ2) seem to be lower than Q1**(1, δ2) and Q2**(1, δ2), respectively.
Now let (p2 – c2)/(p1 – c1) < δ2 ≤ 1; then Q2*(1, δ2) = 0<Q2**(1, δ2), and according to (11),
(23)
(23)
From (23) as well as the first term of (21), Q1*(1, δ2) > Q1**(1, δ2).
Next, we will consider the case in which δ2 is small and close to 0, meaning that when product 2 is out of stock, customers are generally unwilling to switch and buy product 1.
Proposition 3.9:
When δ2 is small and close to 0, then Q1*< Q1** and Q2* > Q2**.
Proof:
See Appendix A.
When δ2 is small and close to 0, then customers who prefer product 2 have very low flexibility to buy product 1. The implications of this inflexibility for the products’ optimal order quantities are more significant in the single-retailer model than in the competitive model. Specifically, in a single-retailer setting, Q2 must be large enough to accommodate almost all the demand for product 2, as customers who prefer this product are likely to be lost. Moreover, Q2* should satisfy both the original demand for product 2 and the demand among customers who do not receive product 1 and are prepared to buy product 2; therefore, Q2* > Q2**.
It will now be demonstrated that when δ2 exceeds a certain threshold, the optimal inventory level of product 1 is greater in the case of a single retailer than in the competitive setting. For product 2, the opposite holds. Clearly, such a situation would be expected to occur once δ2 exceeds the threshold (p2-c2)/(p1-c1), since in this case Q2* vanishes and Q1* jumps. However, as presented in Proposition 3.10, these relationships hold even for values of δ2 that are lower than (p2 – c2)/(p1 – c1) – but not too small.
Proposition 3.10:
There exists a threshold Δ, 0 < Δ < (p2-c2)/(p1-c1), such that Q1* > Q1** and Q2* < Q2** for every Δ <δ2 ≤ 1 and every δ1.
Proof:
See Appendix A.
Proposition 3.10 implies that when the substitution rate for a particular product is relatively high – i.e. exceeds a certain threshold – the optimal inventory order size of the alternative product is larger in the case of a single retailer than in a competitive setting.
4. Empirical study
In the second part of our research, we used real-world data to (i) derive the joint sales distribution of two substitutable products; and (ii) obtain concrete insights regarding the ways in which the optimal inventory levels and expected profits are affected by the values of the model parameters (through a sensitivity analysis). To obtain our data, we collaborated with an online fast fashion retailer that sells clothing and footwear for women and men. The retailer provided us with daily-level data regarding a year’s worth of sales for a sneaker that was available in two colours. To protect the retailer’s anonymity, we do not present our collected data set in full. We further note that, as elaborated in what follows, the data set used in our analysis excludes several days with high volatility in sales, corresponding to major holidays (e.g. New Year's Day and Black Friday).
4.1. Sales data
Our data set included the daily sales for each of the two sneaker versions (colours), denoted product 1 and product 2, for the period between January 2020 and December 2020. We were also provided with the products’ unit prices and order costs. Table 1 presents summary statistics for the sales data, excluding major holidays. On average, product 1 sold 776.69 units per day, and product 2 sold 275.48 units per day. The median and mode are equal and very close to the mean for each colour.
Table 1. Summary statistics of sales data (number of product units sold per day).
First, for each of the two products, we conducted Kolmogorov–Smirnov tests (with a level of significance α = 0.05) to determine whether the sales distributions were close to normal. We carried out this test twice for each product. The first time, we included all sales data, including major holidays that usually have high sales volatility (365 days). The second time, we excluded major holidays (using data for 355 days). When the data did not include major holidays, test results indicated that the sales distribution for product 1 was normal (p = 0.077), whereas the distribution of sales for product 2 was not normal (p close to 0), with positive skewness and kurtosis (0.127 and 0.085 respectively). To normalise the data, we took the square root of the sales of product 2 (resulting in a normal distribution, p > 0.05). To ensure comparability of the data for the two products, we also took the square root of the sales of product 1 and reapplied the Kolmogorov–Smirnov (excluding major holidays); we once again obtained a p-value greater than 0.05, indicating a normal distribution (see Appendix B1). Thus, in what follows, we assume that the daily square root sales of product 1 and of product 2 are each normally distributed, with statistics given in Table 2.
Table 2. Summary statistics of sales (number of product units sold per day) after square root transformation.
4.2. Joint demand distribution
We denote by x1 and x2 the daily sales of products 1 and 2, respectively. In line with prior models addressing inventory management of (fast) fashion products (e.g. Caro and Gallien Citation2010; Kamanchi et al. Citation2020; Lucci, Schiraldi, and Varisco Citation2016), as well as stockout-based substitution (e.g. Martínez-de-Albéniz and Kunnumkal Citation2022), we assume that x1 and x2 are Poisson-distributed random variables, with time-dependent means. Our aim is to estimate these means as a function of the day of the week and the date in the year. A salient feature of the Poisson distribution is that, for a Poisson-distributed variable X with a mean that is not too small, is approximately normally distributed with standard deviation 0.5 (see Lemma 3 in Appendix B1). Since sales may occur in batches, the constant standard deviation may be higher. As will be seen in what follows, goodness-of-fit tests provide ample justification for our assumption that x1 and x2 are Poisson-distributed.
To estimate variables of interest – namely, the standard deviations σ1 and σ2, the correlation coefficient ρ, and the time-dependent mean functions μ1(t) and μ2(t) of the bivariate normal pair ( – we fit a periodic function to the square root of the sales, based on a yearly and a daily period. Specifically, we used a multiple linear regression model with trigonometric elements. The explanatory variables (predictors) were sine and cosine variables corresponding to the period of one week, and sine and cosine variables corresponding to the period of one year.
The coefficients for the two regression models are presented in Appendix B2. For product 1 (Table B.2.1 in Appendix B2), the predictors explain 51% of the variance in (the square root of the) sales (Multiple R = 0.71, R2 = 0.51, Adjusted R2 = 0.51). We then obtain:
(24)
(24)
where
,
, and t is the time measured in days.
For product 2 (Table B.2.2 in Appendix B2), all predictors together explain 45% of the sales variation (Multiple R = 0.67, R2 = 0.45, Adjusted R2 = 0.44). We then obtain:
(25)
(25)
where
,
and t is the time measured in days.
The values µi (for each product i) obtained by the above regressions ((24)-(25)) are depicted in .
Table 3. Parameters for model.
Next, we analysed the residuals (i.e. actual transformed sales minus µi, ). For each product, we performed a Kolmogorov–Smirnov test (at the level of significance α = 0.05), and found that the distribution of the residuals was adequately normal (p-value greater than 0.05). Then, we performed the Jarque-Bera (J-B) test to test joint normality (Jarque and Bera Citation1980). In our case, the J-B value for product 1 was 0.272, and the value for product 2 was 0.21 (see Appendix B1, Figure B1.3). As the null hypothesis in the J-B test was not rejected, we proceeded to evaluate the empirical covariance matrix of the residuals (2 columns and 355 rows), to estimate σ1 and σ2. Specifically, for product 1 we obtained σ1 = 4.19; and for product 2 we obtained σ2 = 3.31. The value of the correlation coefficient for the joint distribution, denoted by ρ, is 0.5689. Because fast fashion products are characterised by a short life cycle and short storage life, zero salvage value can be assumed (Chen, Tian, and Yang Citation2014). These data are summarised in Table 3. Footnote1
4.3. Sensitivity analysis
In this section, we apply our models to the data obtained from the retailer, including the parameter values derived in the previous section (see Table 3). Specifically, we explore how the optimal inventory level for each product, as well as the retailers’ expected profits, are affected by adjustments to the following three parameters: the probability of substitution (δ), the unit selling price (p), and the correlation coefficient (ρ(, which affects the joint probability distribution function.
According to Table 3, p1 is larger than p2, and the first two terms of (7) are satisfied for every 0 ≤ δ1 ≤ 1 and 0 ≤ δ2 ≤ 0.9. Therefore, it is assumed that δ2 does not exceed 0.9 and that δ1δ2 is lower than or equal to 0.5, so that problem (6) is concave. Also, p1 – c1 > p2 – c2, that is, condition (8) is satisfied for every 0 ≤ δ1 ≤ 1, whereas (9) is met only if 0 ≤ δ2 ≤ 0.769. Consequently, for the single-retailer model, the optimal inventory level of product 1 is always positive, whereas that of product 2 disappears under the single-retailer model if δ2 exceeds 0.769.
4.3.1. The impact of stockout-based substitution rate on optimal inventory levels and profits
First, we examine the case in which δ2 is fixed at 0.5, and the value of δ1 is varied between 0 and 1 (recall that a higher value of δ1 indicates higher willingness of customers who prefer product 1 to buy product 2 in the event of an inventory shortage). Figure presents the optimal inventory levels of product 1 (Figure a) and of product 2 (Figure b), in both the centralised and competitive settings, as functions of δ1.
In accordance with Propositions 3.3 and 3.7, we observe that in both the centralised and competitive cases, the optimal inventory level of product 1 (Q1* or Q1**) decreases in δ1 (Figure a), and the optimal inventory level of Q2 (Q2* or Q2**) increases in δ1. In other words, as δ1 increases, increasing the inventory level of product 1 is not profitable. Instead, it is preferable to increase the inventory level of product 2. Notably, Q1 decreases more steeply in a single-retailer setting than in a competitive setting, as is mentioned in the discussion following Proposition 3.7 above.
We further observe that, in accordance with Proposition 3.8, when δ1 is small, the optimal order quantity for product 1 is lower in a competitive setting (Q1**) than in a centralised setting (Q1*). Yet, when δ1 is large, the opposite is the case. For product 2, however, the optimal inventory level of product 2 is always higher in a competitive setting than in a centralised setting.
Figure shows the total optimal inventory level (Q1 + Q2) for each setting, as a function of δ1. We observe that the total optimal inventory level increases in both settings, but that it increases more steeply in the competitive setting, eventually surpassing the optimal inventory level in the single-retailer setting.
Figure 4. Effect of δ1 on the total optimal inventory level (Q1 + Q2).
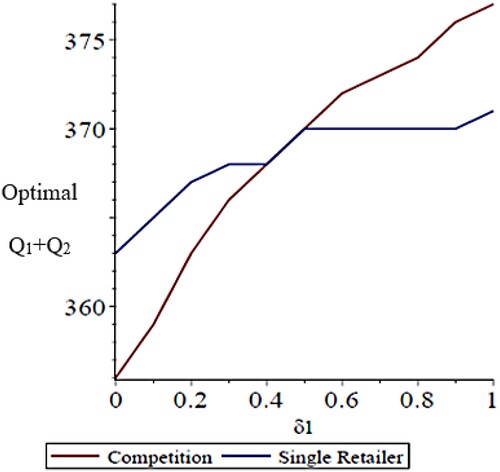
Figure shows the retailers’ expected profits as a function of δ1. The single retailer's total profit is compared to the sum of the profits of the competitive retailers. The single retailer's expected profit increases from 3270 to 3320 (slightly more than 1.5%) as δ1 increases to 0.9 (according to Proposition 3.4). The total expected profit of the two competitive retailers increases from 3230 to 3300 (slightly more than 2%).
Figure 5. Effect of δ1 on the profits of the retailer(s). The graph for the competition scenario represents the sum of profits for the two retailers.
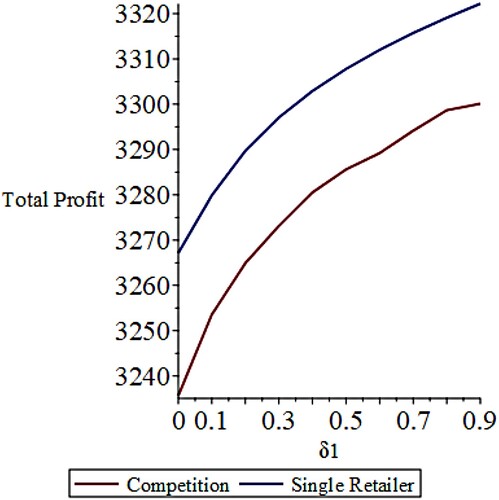
Next, we carry out a similar analysis for the case in which δ1 is fixed at 0.5, and the value of δ2 is varied between 0 and 0.9. Figure shows the optimal order quantities. We observe that Q1* and Q1** increase in δ2 (Figure a), whereas Q2* and Q2** decrease (Figure b), as stated in Propositions 3.3 and 3.7. In this case, the increase or decrease in the single-retailer setting is significantly steeper than in the competitive setting. In particular, while Q2* drops from 105 to 0, the effect of δ2 on Q2**, is negligible (that order quantity decreases from 101 to 98).
Figure 6. Effect of δ2 on the optimal inventory levels Q1 (a) and Q2 (b).
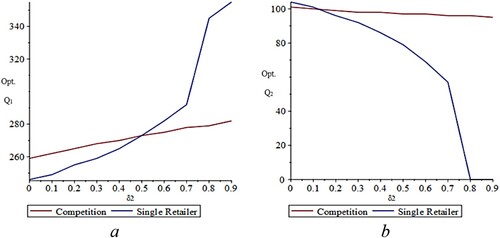
We further observe that, in accordance with Proposition 3.9, when δ2 is small, Q1** > Q1* and Q2* > Q2**. This relationship is reversed when δ2 is close to 1, as Q2* vanishes and Q1* increases. The optimal inventory levels of the two models intersect at δ2 = 0.5 for product 1 and δ2 = 0.15 for product 2.
Figure shows that that total inventory (the sum of the optimal values of Q1 + Q2) increases more steeply in δ2 in the single-retailer setting than in the competitive setting, in line with the steep increase in Q1* observed in Figure a.
Figure 7. Effect of δ2 on the total optimal inventory level (Q1 + Q2).
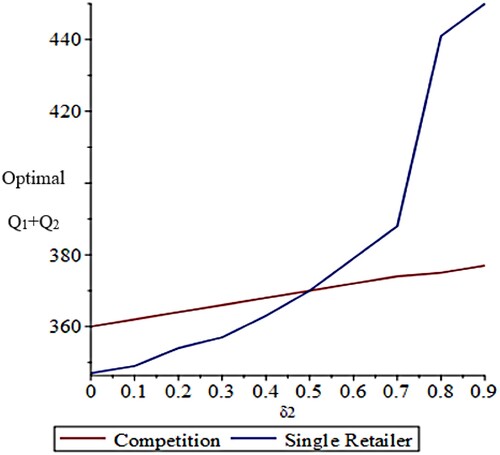
The results above are compatible with the reasoning that, since the profit from product 1 is greater than that of product 2, when δ2 increases (i.e. increasing customers’ flexibility to buy product 1), a retailer who sells product 1 benefits from increasing the inventory level of that product.
Regarding the retailers’ expected profit, Figure shows that the total expected profit of the two competitive retailers increases in δ2 from 3200 to 3350 (almost 4.7%). In a single-retailer setting, the expected profit from both products increases to a greater extent – from 3200 to 3500 (slightly more than 9.3%) as δ2 increases to 0.9.
Figure 8. Effect of δ2 on the profits of the retailer(s). The graph for the competition scenario represents the sum of profits for the two retailers.
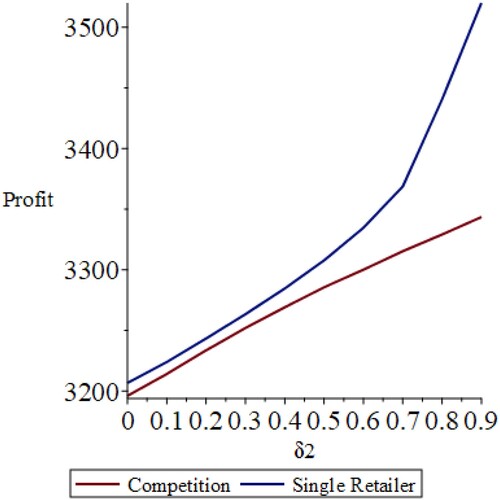
4.3.2. The impact of unit selling price ratio on optimal inventory levels
In this section we examine how the optimal inventory levels are affected by an increase in the unit selling price ratio (p1/p2). In order to maintain the ratio between the unit selling price (p) and order cost (c), we assume that a change in the unit selling price is accompanied by a corresponding change in the order cost. The optimal inventory levels of Q1 and Q2, are presented in Figure a,b, respectively.
Figure 9. Effects of p1/p2 on the optimal inventory levels for Q1 (a) and Q2 (b).
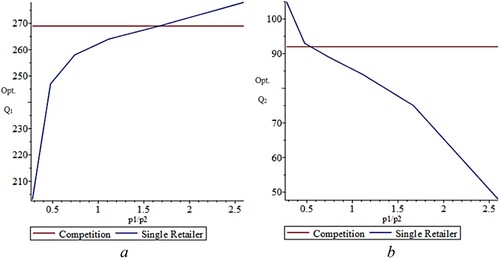
According to Proposition 3.6, in a competitive setting, the optimal inventory level of Qi depends only on ci/pi. Given our assumption that ci/pi remains constant, it is clear that in a competitive setting, Q1** and Q2** do not change as a function of p1/p2. In a single-retailer setting, however, according to Proposition 3.1, Qi* depends on ci/pi and on p3-i/pi. Consequently, the inventory levels change as a function of p1/p2. Q1* increases in p1/p2, whereas Q2* decreases.
4.3.3. The impact of the correlation coefficient on optimal inventory levels
The correlation coefficient ρ is an indication of the extent to which demand for the two products is correlated, in the absence of substitution.
Figures and show, respectively, how the optimal inventory levels and the total inventory level respond to the value of the correlation coefficient. We observe that when the correlation coefficient is positive, the optimal inventory levels of both products are low, due to the risk of having low demand for both products simultaneously. On the other hand, this situation has low probability when the correlation coefficient is negative, and therefore the inventory levels are high.
Figure 10. Effect of correlation coefficient ρ on the inventory levels of Q1 (a) and Q2 (b).
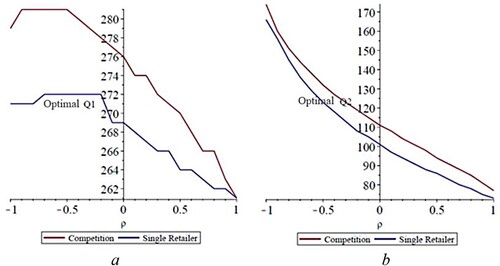
Figure 11. Effect of correlation coefficient ρ on the total optimal inventory (Q1 + Q2).
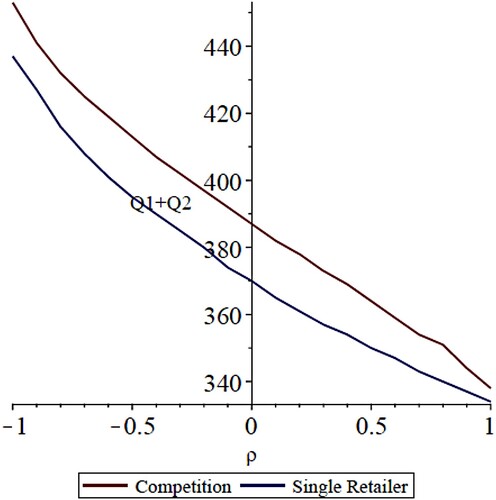
5. Conclusion and managerial implications
This work addressed retailers’ inventory decisions for substitutable products, focusing on the fashion industry, where demand is uncertain, yet consumers are often willing to accept product substitutes when their preferred products are out of stock (e.g. select the same garment in a different colour). We developed single-period models to describe a market in which two substitutable products are sold, and retailers must select the inventory amounts that maximise their expected profits, under stockout-based substitution. We considered both a centralised setting, in which a single retailer offers both products, and a competitive setting, in which each product is offered exclusively by a different retailer.
In both cases, we proved that the objective functions are concave so that a unique optimal solution exists. Moreover, for the centralised setting, we identified conditions under which the retailer benefits from maintaining positive inventory levels for both products, as well as conditions under which it is preferable to stock only one type of product. In each case, we analytically described how the optimal order quantity and expected profits respond to changes in the parameter values, including the substitution rate (i.e. consumers’ willingness to substitute one product for another in the event of a stockout) and product unit prices and costs. We also compared the two settings, identifying scenarios, for example, in which the optimal order quantity for a particular product in the single-retailer setting exceeds that in the competitive setting, or vice versa.
We enriched our model with data from an online apparel retailer regarding the sales of two substitutable products (sneakers in two different colours); these data enabled us to estimate the demand distribution. We subsequently conducted analyses, grounded in empirical data, to derive concrete insights regarding the sensitivity of optimal inventory levels and expected profits to specific parameter values.
Together, the analytical and empirical components of our work converge to the following managerial insights: (i) Optimal inventory levels are significantly affected by customers’ willingness to substitute one product for another in the event that their preferred product is out of stock. Indeed, in the case of a single retailer (centralised setting), there are conditions in which it may be preferable to stock only one type of product (specifically, the more profitable of the two), if consumers are sufficiently willing to substitute that product for their preferred product. In the case of two competing retailers, however, the optimal order quantity for each product is always positive, given that each retailer only offers one type of product, and thus a lack of stock means a lack of profit. (ii) Similarly, when customers’ willingness to compromise is greater, retailers’ expected profit is higher – under both centralised and competitive settings. (iii) Comparing between the two settings, we find that when customers’ flexibility regarding a particular product is low, the optimal inventory order size of that product is larger in the case of a single retailer than in the competitive setting. Conversely, the inventory order size of the other product is larger in the competitive setting than in the centralised case. (iv) When the demand levels for the two products are highly correlated, the optimal inventory levels of both products are low, due to the risk of having very low demand for both products simultaneously.
Future research might extend our work in several ways. One possibility would be to account for lost sales – in which a retailer who runs out of a particular product incurs a penalty when customers leave without making a purchase (and, when possible, buy the product elsewhere). In this case, the solution becomes more complicated, because the objective function depends on the arrival rates of the customers who are interested in buying the product (see preliminary formulation of the model in Appendix C). Another option is to extend our work to encompass a multi-product model that is dynamically solved during a number of different periods (with leftover inventory from previous periods). Such a model would more realistically capture retailers’ decisions in practice, which encompass large numbers of products that may be saleable over multiple periods (e.g. with markdowns on older products as new products enter the market).
Supplemental Material
Download MS Word (174.8 KB)Acknowledgements
The authors would like to thank Isaac Meilijson for his help in estimating the joint demand and the three anonymous reviewers for their constructive comments and suggestions which have significantly improved the paper.
Data availability statement
Data available on request from the authors.
Disclosure statement
No potential conflict of interest was reported by the author(s).
Additional information
Funding
Notes on contributors
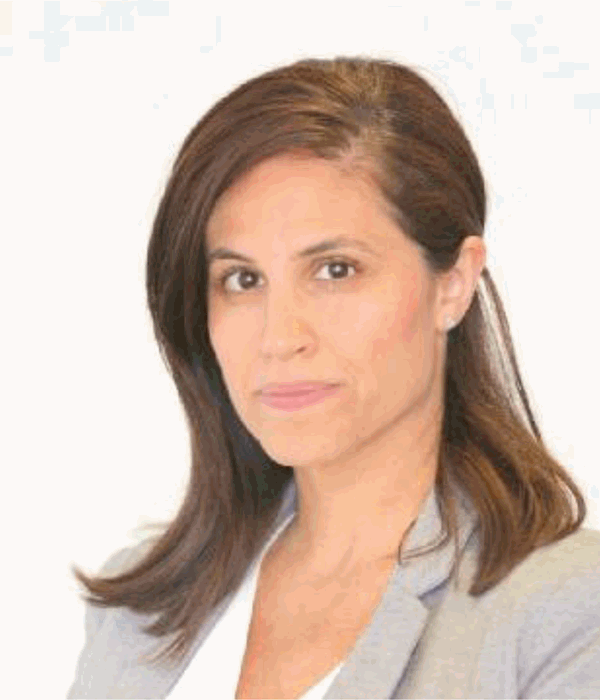
Michal Koren
Michal Koren holds a Ph.D. and an M.A. in Industrial Management, both from the Department of Management, Bar-Ilan University in Israel, and a B.Sc. in Industrial Engineering and Management from Shenkar- Engineering. Design. Art. Currently, she is a faculty member and deputy dean at the School of Industrial Engineering and Management at Shenkar- Engineering. Design. Art. Her research interests include Operations Research, Supply Chain, Machine Learning and Artificial Intelligence.
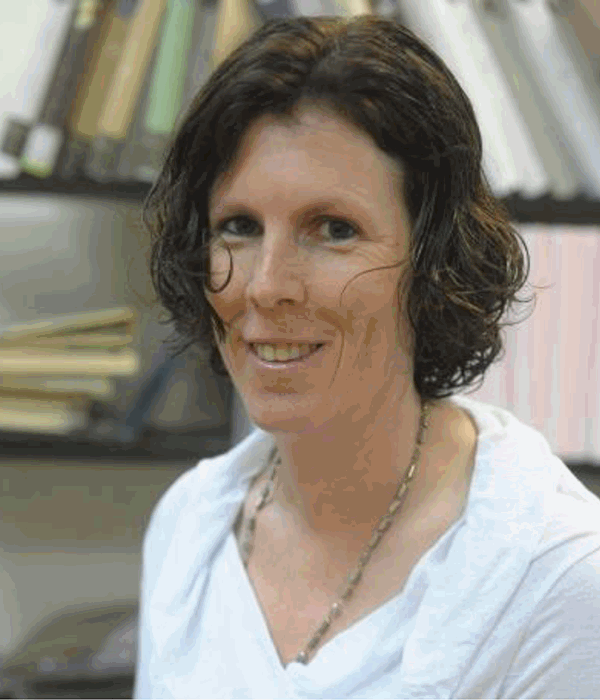
Yael Perlman
Yael Perlman is an Associate Professor of Operations Management in the Department of Management at Bar-Ilan University, Israel. She holds a BSc in Mathematics (TAU) an MBA in Operations Research (TAU) and a PhD in Industrial Engineering (BGU). Yael Perlman develops game theory-based models and queuing theory-based models to investigate strategic decisions of the supply chain members and the effect of intra-supply chain competition on the overall supply chain performance. Her papers have been published in some of the leading journals of Supply Chain Management and Operations Management.
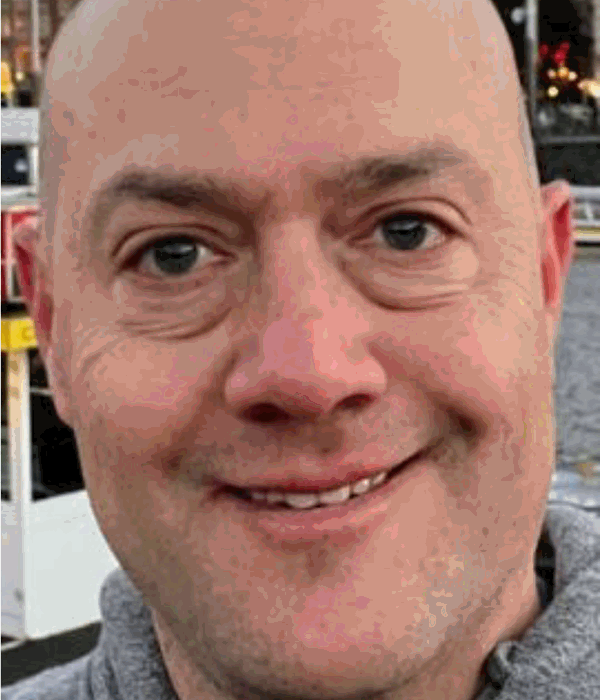
Matan Shnaiderman
Matan Shnaiderman received M.S. and Ph.D. degrees in the Department of Mathematics, Bar-Ilan University in Israel. He is currently Senior Lecturer at the Department of Management, Bar-Ilan University. His research interests include supply-chain and inventory management, information sharing, public transit, frequency setting, energy production and stochastic dynamic programming.
Notes
1 Since we do not have data from two rival retailers, we used the data from in the sensitivity analysis performed in the next subsection as a scenario analysis.
References
- Achamrah, F. E., F. Riane, and S. Limbourg. 2022. “Solving inventory routing with transshipment and substitution under dynamic and stochastic demands using genetic algorithm and deep reinforcement learning.” International Journal of Production Research, 60 (20), 6187–6204.
- Ambilkar, P., V. Dohale, A. Gunasekaran, and V. Bilolikar. 2022. “Product Returns Management: A Comprehensive Review and Future Research Agenda.” International Journal of Production Research 60 (12): 3920–3944. https://doi.org/10.1080/00207543.2021.1933645
- Besbes, O., and D. Sauré. 2016. “Product Assortment and Price Competition Under Multinomial Logit Demand.” Production and Operations Management 25 (1): 114–127. https://doi.org/10.1111/poms.12402
- Caro, F., and J. Gallien. 2010. “Inventory Management of a Fast-Fashion Retail Network.” Operations Research 58 (2): 257–273. https://doi.org/10.1287/opre.1090.0698
- Chen, B., and X. Chao. 2020. “Dynamic Inventory Control with Stockout Substitution and Demand Learning.” Management Science 66 (11): 5108–5127. https://doi.org/10.1287/mnsc.2019.3474
- Chen, W., Tian, Y., & Yang, Y. X. (2014, August). “Decentralized and Centralized Supply Chain Decision-Making of Low Salvage Value Products with Price-postponement Strategy.” In 2014 International Conference on Management Science & Engineering 21th Annual Conference Proceedings, 345–350. IEEE.
- Choi, T. M., and S. Guo. 2018. “Responsive Supply in Fashion Mass Customisation Systems with Consumer Returns.” International Journal of Production Research 56 (10): 3409–3422. https://doi.org/10.1080/00207543.2017.1292065
- Dong, Z. S., W. Chen, Q. Zhao, and J. Li. 2020. “Optimal Pricing and Inventory Strategies for Introducing a new Product Based on Demand Substitution Effects.” Journal of Industrial & Management Optimization 16 (2): 725–739. https://doi.org/10.3934/jimo.2018175
- DuBreuil, M., and S. Lu. 2020. “Traditional vs. big-Data Fashion Trend Forecasting: An Examination Using WGSN and EDITED.” International Journal of Fashion Design, Technology and Education 13 (1): 68–77. https://doi.org/10.1080/17543266.2020.1732482
- Ergin, E., M. Gümüş, and N. Yang. 2022. “An Empirical Analysis of Intra-Firm Product Substitutability in Fashion Retailing.” Production and Operations Management 31 (2): 607–621. https://doi.org/10.1111/poms.13569
- Goyal, V., R. Levi, and D. Segev. 2016. “Near-optimal Algorithms for the Assortment Planning Problem Under Dynamic Substitution and Stochastic Demand.” Operations Research 64 (1): 219–235. https://doi.org/10.1287/opre.2015.1450
- Gupta, V. K., Q. U. Ting, and M. K. Tiwari. 2019. “Multi-period Price Optimization Problem for Omnichannel Retailers Accounting for Customer Heterogeneity.” International Journal of Production Economics 212: 155–167. https://doi.org/10.1016/j.ijpe.2019.02.016
- Gümüş, M., P. Kaminsky, and S. Mathur. 2016. “The Impact of Product Substitution and Retail Capacity on the Timing and Depth of Price Promotions: Theory and Evidence.” International Journal of Production Research 54 (7): 2108–2135. https://doi.org/10.1080/00207543.2015.1108536
- Hammami, R., E. Asgari, Y. Frein, and I. Nouira. 2022. “Time-and Price-Based Product Differentiation in Hybrid Distribution with Stockout-Based Substitution.” European Journal of Operational Research 300 (3): 884–901. https://doi.org/10.1016/j.ejor.2021.08.042
- Ho, W., T. Zheng, H. Yildiz, and S. Talluri. 2015. “Supply Chain Risk Management: A Literature Review.” International Journal of Production Research 53 (16): 5031–5069. https://doi.org/10.1080/00207543.2015.1030467
- Honhon, D., V. Gaur, and S. Seshadri. 2010. “Assortment Planning and Inventory Decisions Under Stockout-Based Substitution.” Operations Research 58 (5): 1364–1379. https://doi.org/10.1287/opre.1090.0805
- Hübner, A. 2017. “A Decision Support System for Retail Assortment Planning.” International Journal of Retail & Distribution Management. 45 (7/8): 808–825. https://doi.org/10.1108/IJRDM-09-2016-0166.
- Jarque, C. M., and A. K. Bera. 1980. “Efficient Tests for Normality, Homoscedasticity and Serial Independence of Regression Residuals.” Economics Letters 6 (3): 255–259. https://doi.org/10.1016/0165-1765(80)90024-5
- Kamanchi, C., G. A. Kumar, N. Sundaram, and C. Bandi. 2020. “An Application of Newsboy Problem in Supply Chain Optimisation of Online Fashion E-Commerce.” arXiv preprint arXiv:2007.02510.
- Ko, E., J. P. Costello, and C. R. Taylor. 2019. “What is a Luxury Brand? A new Definition and Review of the Literature.” Journal of Business Research 99: 405–413. https://doi.org/10.1016/j.jbusres.2017.08.023
- Koren, M., Y. Perlman, and M. Shnaiderman. 2022. “Inventory Management Model for Stockout Based Substitutable Products.” IFAC-PapersOnLine 55 (10): 613–618. https://doi.org/10.1016/j.ifacol.2022.09.467
- Kök, A. G., M. L. Fisher, and R. Vaidyanathan. 2015. “Assortment Planning: Review of Literature and Industry Practice.” In Retail Supply Chain Management. International Series in Operations Research & Management Science, edited by N. Agrawal and S. Smith, vol. 223, 175–236. New York: Springer.
- Li, M., and T. Li. 2018. “Consumer Search, Transshipment, and Bargaining Power in a Supply Chain.” International Journal of Production Research 56 (10): 3423–3438. https://doi.org/10.1080/00207543.2017.1326644
- Li, S., L. X. Lu, S. F. Lu, and S. Huang. 2023. “Estimating the Stockout-Based Demand Spillover Effect in a Fashion Retail Setting.” Manufacturing & Service Operations Management 25 (2): 468–488.
- Loureiro, A. L., V. L. Miguéis, and L. F. da Silva. 2018. “Exploring the use of Deep Neural Networks for Sales Forecasting in Fashion Retail.” Decision Support Systems 114: 81–93. https://doi.org/10.1016/j.dss.2018.08.010
- Lucci, G., M. M. Schiraldi, and M. Varisco. 2016. “Fashion Luxury Retail Supply Chain: Determining Target Stock Levels and Lost Sale Probability.” In 21st Summer School Francesco Turco 2016 (Vol. 13, pp. 192–197). AIDI-Italian Association of Industrial Operations Professors.
- Martínez-de-Albéniz, V., and S. Kunnumkal. 2022. “A Model for Integrated Inventory and Assortment Planning.” Management Science 68 (7): 5049–5067. https://doi.org/10.1287/mnsc.2021.4149
- Ovezmyradov, B., and H. Kurata. 2019. “Effects of Customer Response to Fashion Product Stockout on Holding Costs, Order Sizes, and Profitability in Omnichannel Retailing.” International Transactions in Operational Research 26 (1): 200–222. https://doi.org/10.1111/itor.12511
- Parlar, M. 1988. “Game Theoretic Analysis of the Substitutable Product Inventory Problem with Random Demands.” Naval Research Logistics (NRL) 35 (3): 397–409. https://doi.org/10.1002/1520-6750(198806)35:3<397::AID-NAV3220350308>3.0.CO;2-Z
- Perlman, Y. 2019. “Inventory Levels of Two Stockout-Based Substitutable Products Under Centralized and Competitive Settings.” IFAC-PapersOnLine 52 (13): 1450–1454. https://doi.org/10.1016/j.ifacol.2019.11.403
- Ren, S., H. L. Chan, and T. Siqin. 2020. “Demand Forecasting in Retail Operations for Fashionable Products: Methods, Practices, and Real Case Study.” Annals of Operations Research 291 (1-2): 761–777. https://doi.org/10.1007/s10479-019-03148-8
- Schlapp, J., and M. Fleischmann. 2018. “Multiproduct Inventory Management Under Customer Substitution and Capacity Restrictions.” Operations Research 66 (3): 740–747. https://doi.org/10.1287/opre.2017.1690
- Shen, B., H. L. Chan, P. S. Chow, and K. A. Thoney-Barletta. 2016. “Inventory Management Research for the Fashion Industry.” International Journal of Inventory Research 3 (4): 297–317. https://doi.org/10.1504/IJIR.2016.082325
- Shin, H., S. Park, E. Lee, and W. C. Benton. 2015. “A Classification of the Literature on the Planning of Substitutable Products.” European Journal of Operational Research 246 (3): 686–699. https://doi.org/10.1016/j.ejor.2015.04.013
- Shokouhyar, S., S. Shokoohyar, N. Raja, and V. Gupta. 2021. “Promoting Fashion Customer Relationship Management Dimensions Based on Customer Tendency to Outfit Matching: Mining Customer Orientation and Buying Behaviour.” International Journal of Applied Decision Sciences 14 (1): 1–23. https://doi.org/10.1504/IJADS.2021.112932
- Singh, D., and A. Verma. 2018. “Inventory Management in Supply Chain.” Materials Today: Proceedings 5 (2): 3867–3872. https://doi.org/10.1016/j.matpr.2017.11.641
- Transchel, S. 2017. “Inventory Management Under Price-Based and Stockout-Based Substitution.” European Journal of Operational Research 262 (3): 996–1008https://doi.org/10.1016/j.ejor.2017.03.075
- Wan, M., Y. Huang, L. Zhao, T. Deng, and J. C. Fransoo. 2018. “Demand Estimation Under Multi-Store Multi-Product Substitution in High Density Traditional Retail.” European Journal of Operational Research 266 (1): 99–111https://doi.org/10.1016/j.ejor.2017.09.014
- Wang, Q., and B. Zhang. 2021. “A Guarantee Credit Model with Substitutable Product Competition.” International Journal of Production Research 59 (22): 6919–6940. https://doi.org/10.1080/00207543.2020.1830195
- Wang, S., H. Zhang, F. Chu, and L. Yu. 2022. “A Relax-and-fix Method for Clothes Inventory Balancing Scheduling Problem.” International Journal of Production Research, 1–20. https://doi.org/10.1080/00207543.2022.2145517
- Wen, X., T. M. Choi, and S. H. Chung. 2019. “Fashion Retail Supply Chain Management: A Review of Operational Models.” International Journal of Production Economics 207: 34–55. https://doi.org/10.1016/j.ijpe.2018.10.012
- Yamazaki, T., K. Shida, and T. Kanazawa. 2016. “An Approach to Establishing a Method for Calculating Inventory.” International Journal of Production Research 54 (8): 2320–2331. https://doi.org/10.1080/00207543.2015.1076179
- Zeppetella, L., E. Gebennini, A. Grassi, and B. Rimini. 2017. “Optimal Production Scheduling with Customer-Driven Demand Substitution.” International Journal of Production Research 55 (6): 1692–1706. https://doi.org/10.1080/00207543.2016.1223895
- Zhang, X., M. Yang, J. Su, W. Yang, and K. Qiu. 2020. “Research on Product Color Design Decision Driven by Brand Image.” Color Research & Application 45 (6): 1202–1216. https://doi.org/10.1002/col.22540
- Zhao, Y., and X. Zhao. 2016. “How a Competing Environment Influences Newsvendor Ordering Decisions.” International Journal of Production Research 54 (1): 204–214. https://doi.org/10.1080/00207543.2015.1034330