ABSTRACT
The metaverse and Web 3.0 have created a new digital world with specific properties and behaviours replicating and influencing the behaviours and processes of physical entities. This study aims to advance our understanding of how the metaverse will impact supply chain and operations management (SCOM). Using elements of a structured literature search and building on the concepts of cyber-physical systems, digital supply chain twins, cloud supply chains, and Industry 4.0/Industry 5.0, we propose a framework for metaverse SCOM encompassing multiple socio-technological dimensions. We conclude that further metaverse developments could result in a co-existence of physical SCOM, metaverse SCOM, and SCOM for coordination of the physical and metaverse worlds. We offer a structured future research agenda pointing to new research questions and topics stemming from metaverse-driven visibility, computational power for data analytics, digital collaboration, and connectivity. New research areas can emerge for the novel metaverse SCOM processes and decision-making areas (e.g. joint demand forecasting for metaverse and physical products, digital inventory allocation in the metaverse, integrated production planning for the metaverse and physical worlds, and pricing and contracting for digital products), as well as new performance measures (e.g. virtual customer experience level, availability of digital products, and digital resilience and sustainability).
1. Introduction
The metaverse creates a new world where every physical entity (e.g. people, products, and enterprises) has a digital twin. The entities in the metaverse are not just digital replicas of some physical entities; rather, they are equipped with artificial intelligence possessing and changing their own properties and behaviours and even influencing the behaviours and processes of physical entities. The metaverse is expected to completely change our lives in the very near future, much faster than we can think of now.
What is the metaverse? The term ‘metaverse’ was coined in 1992 in Neal Stephenson’s literary work Snow Crash (Stephenson Citation1992), where it was visualised as a black spherical planet accessible to users through terminals with integrated virtual reality capabilities through which users could appear as avatars (The Economist Citation2020). According to Maersk (Citation2022), ‘[t]he metaverse is the next evolution of the Internet. It’s a fusing of the digital and physical worlds powered by technologies, including virtual and augmented reality, blockchain, artificial intelligence, and the Internet of things that connects smart devices’. Moreover, Lovich (Citation2022) defines the metaverse as ‘a combination of the virtual reality and mixed reality worlds accessed through a browser or headset, which allows people to have real time interactions and experiences across distance.’
The metaverse develops fast. Digital technology leaders like Nvidia with Omniverse and Facebook with Meta have invested deeply in metaverse solutions (Huynh-The et al. Citation2023). The SupplyOn supplier collaboration platform (Holzwarth, Staib, and Ivanov Citation2022) and the Catena-X data ecosystem have also been developed in the automotive industry, allowing for the creation of digital product passports and improving the sustainability and resilience of supply chains from the perspective of the ecosystem (Catena Citation2022). For instance, Siemens and BMW have developed smart manufacturing platforms using cloud technology (Open Manufacturing Citation2022; Siemens Citation2022). Furthermore, manufacturing companies like Puma, Nike, Gucci, and Adidas have started using the idea of the metaverse in marketing and e-commerce to interact with customers, allowing them to view or even buy digital versions of their products (Barrera and Shah Citation2023). However, the role of the metaverse in supply chain and operations management (SCOM) remains underexplored (Kathiala Citation2022).
Mourtzis et al. (Citation2022) claim that the metaverse represents a new era in Internet connectivity, characterised by interactivity, simulation, a decentralised environment, and persistent reality facilitated by the next evolution of the Internet (also known as Web 3.0) to combine the digital and physical worlds. Lee and Kundu (Citation2022) point to conceptual similarities between the metaverse and cyber-physical systems, the application of which to manufacturing has been discussed by Panetto et al. (Citation2019). A survey by Accenture (Citation2022a) found that 64% of supply chain management executives believe that the metaverse will have an impact on their organisations.
Analysis of the existing literature shows that the metaverse’s impacts on SCOM go beyond the technological dimension: the metaverse is not only a technology but a complex socio-technological phenomenon. In this setting, a scientific approach is needed to reflect on the chances, barriers, and challenges that the development of the metaverse will impose on SCOM.
Thus, this study aims to advance our understanding as to how the metaverse will influence SCOM. In particular we are interested in exploring the following research questions (RQ):
RQ1: How will the metaverse impact SCOM in the physical world?
RQ2: What will be the potential SCOM processes and decision-making areas in the metaverse?
RQ3: What will be the mutual impacts of the co-existence of physical and metaverse SCOM?
To provide some guidelines and structure for further research on metaverse SCOM and the interrelations between physical and metaverse SCOM, we discuss a future research agenda, namely that future research can explore new opportunities for SCOM that stem from the metaverse, i.e. visibility, computational power for data analytics, digital collaboration, and connectivity. At the same time, we show that new SCOM activities can appear specifically dedicated to the metaverse and lead to novel SCOM processes and decision-making areas (e.g. joint demand forecasting for metaverse and physical products, digital inventory allocation in the metaverse, integrated production planning for the metaverse and physical worlds, and pricing and contracting for digital products), as well as new performance measures (e.g. virtual customer experience level, availability of digital products, and digital resilience and sustainability).
The remainder of this paper is organised as follows. In Section 2, the results of a SCOPUS search for metaverse literature related to SCOM are presented. Drawing upon keyword analysis, we propose the metaverse SCOM framework in Section 3. In Section 4, future research questions and new topics that focus on the metaverse are discussed. We conclude in Section 5 by summarising the major insights of this study and pointing to some future extensions of them.
2. Analysis of the main topics in the research on the metaverse and SCOM
To understand the state of the art in research on the metaverse and SCOM, we first ran a SCOPUS search organised as follows:
TITLE-ABS-KEY (metaverse) AND (LIMIT-TO (DOCTYPE, “ar”)) AND (LIMIT-TO (SUBJAREA, “ENGI”) OR LIMIT-TO (SUBJAREA, “BUSI”) OR LIMIT-TO (SUBJAREA, “DECI”))
We carefully analysed the keywords identified by SCOPUS and structured them based on the 7-element digital twin framework (Ivanov Citation2023b). Accordingly, we propose the following seven elements to be included in the metaverse SCOM framework: technology, people, management, organisation, scope, task, and modelling (Table ).
Table 1. Metaverse SCOM elements.
It can be observed in Table that the existing research on the metaverse and SCOM covers a broad socio-technological-economic spectrum. On the one hand, our analysis allows us to identify the key digital technologies enabling the metaverse. On the other hand, the key role of people and the human-machine interface becomes evident through the keywords represented in Table , which was built based on Figure and supplemented by some additional items based on our expert review. The metaverse enables new organisational forms and management capabilities (e.g. visibility and interaction). A large variety of artificial intelligence-based modelling methods supports decision-making tasks in forecasting, manufacturing, and contracting in different system scopes.
3. Metaverse SCOM framework
Based on the keyword analysis, we can now propose a metaverse SCOM framework (Figure ).
The metaverse SCOM framework is based on the 7-element digital twin framework proposed by Ivanov (Citation2023b). The seven major dimensions are people, organisation, management, technology, modelling, scope, and task. In Figure , we combine keywords identified by the SCOPUS search with our integrative analysis of the relevant frameworks such as digital twins (Badakhshan and Ball Citation2023; Berti and Finco Citation2022; Burgos and Ivanov Citation2021; Frazzon, Freitag, and Ivanov Citation2021; Huang, Wang, and Yan Citation2022; Negri, Fumagalli, and Macchi Citation2017), cloud and digital supply chain (Ivanov, Dolgui, and Sokolov Citation2022; MacCarthy and Ivanov Citation2022; Zhang, MacCarthy, and Ivanov Citation2022), collaborative networks (Camarinha-Matos and Afsarmanesh Citation2005), reconfigurable supply chain (Dolgui, Ivanov, and Sokolov Citation2020), cloud manufacturing (Moghaddam and Nof Citation2018), open manufacturing (Kusiak Citation2020), Physical Internet (Pan et al. Citation2017), and Industry 4.0/Industry 5.0 (Choi et al. Citation2022b; Ivanov Citation2022a; Tang and Veelenturf Citation2019; Winkelhaus and Grosse Citation2020Yin, Stecke, and Li Citation2018; Zennaro et al. Citation2019).
Further, in Figure we illustrate the extension of traditional SCOM understanding as ‘a cross-department and cross-enterprise integration and coordination of material, information, and financial flows to transform and use the supply chain resources in the most rational way along the entire value chain, from raw material suppliers to customers’ (Ivanov, Tsipoulanidis, and Schönberger Citation2021b, 9) towards a triple-SCOM view wherein physical, metaverse, and physical-metaverse SCOMs co-exist.
Figure depicts that physical and metaverse worlds are connected through digital technology such as augmented/virtual reality, blockchain, artificial intelligence, the Internet of things, 5G/Edge computing, ERP (enterprise resource planning), big data analytics, and simulation (Brintrup et al. Citation2020; Cai, Choi, and Zhang Citation2021; Chabanet et al. Citation2023; Cui, Li, and Zhang Citation2022; Dolgui and Ivanov Citation2022; Dubey et al. Citation2021; Elmachtoub and Grigas Citation2022). Smart devices and sensors in physical products along with 3D printers represent other data sources for the metaverse. In the metaverse, digital customers (i.e. avatars) act in the digital markets where digital products are offered and sold using digital money (probably, a mix of physical and digital products can be considered too). Managers use digital collaboration spaces for sourcing, production, and logistics coordination. Also, digital stores, factories, and warehouses represent the supply chain in the metaverse, which can be digital replicas of physical stores, factories, and warehouses, or represent new, additional entities which do not exist in the physical supply chain.
4. Open research questions
In this section, we outline open research questions related to the metaverse SCOM.
4.1. Area 1: scope and task
The scope of the metaverse SCOM will cover digital products, digital factories and warehouses, the digital supply chain, and digital ecosystems. The metaverse supply chain is not just a digital replica of a physical supply chain: the digital and physical supply chains evolve autonomously but co-jointly. When we assume that a digital twin is a digital replica of a physical supply chain, then the metaverse is more than a digital twin. On the one hand, the metaverse enhances decision-making support and analytics applications for physical SCOM. On the other hand, the digital and physical supply chains mutually influence each other (Liu, Jiang, and Jiang Citation2020; Lv et al. Citation2022).
For example, the increased popularity of a product in the metaverse can lead to an increased demand for this product in the physical supply chain. A timely recognition of these trends through data analytics can help supply chain managers to prepare for the peak load. The metaverse data analysis can also be used for the introduction of new products into the market and decisions on initial order quantity – for example, a product can be first introduced in the metaverse, and the sales/inventory data from the digital supply chain can be used to set up the physical supply chain processes. In another example, a product shortage in the physical supply chain can be substituted by an increased supply of this product in the metaverse so that customers (or their avatars) who cannot buy the physical product could obtain it in its digital form. This is a novel context for supply chain resilience management.
Assuming that people will have more and more activities to partake in the metaverse, we can expect new SCOM activities specifically dedicated to the metaverse and leading to the appearance of novel SCOM processes and decision-making areas. For example, joint demand forecasting for metaverse and physical products belongs to a new research area. Since digital products will also require some storage place in the metaverse, digital inventory allocation in the metaverse can arise as a novel optimisation context. Pricing and contracting for digital products as well as new performance measures (e.g. virtual customer experience level and availability of digital products, as well as digital resilience and sustainability) can motivate new research. Through digital analytics, testing and forecasting customer and supplier behaviours can be used for demand, inventory, and capacity planning. Circular SCOM can receive a new perspective combining digital and physical reverse flows (Meier, Gruchmann, and Ivanov Citation2023).
Inventory management research can also be innovated through the metaverse. For example, one group of customers might like to have both physical and digital products, another group only physical, and another only digital – for example, a luxury car, which can be too expensive in real life can be purchased in the metaverse. Competition between digital and physical products can lead to interesting new problem settings in pricing and inventory management. In some cases, digital products can even be wanted more than physical ones – a new setting for revenue management. Furthermore, sourcing and production planning in the metaverse SCOM can be adjusted through digital collaboration spaces with improved delivery visibility and coordination. In addition, physical products might be increasingly supplemented by some digital services, and digital products can include some physical add-ons. In this setting, sourcing and production planning can encounter novel and counter-intuitive decision-making problems.
4.2. Area 2: management
As indicated in Figure , three SCOMs could exist when the metaverse becomes an important part of everyday life – SCOM for physical world, SCOM for digital world, and SCOM for coordinating physical and digital worlds. The metaverse can be used for decision-making support in physical SCOM through enhanced management capabilities such as visibility, computational power for data analytics, digital collaboration, and connectivity (Dolgui and Ivanov Citation2022; Ivanov et al. Citation2021a).
Through supply chain mapping, it becomes possible to obtain more accurate, real-time data on lead-times, delays, deliveries, shortages, physical locations of containers and trucks, and costs (MacCarthy, Ahmed, and Demirel Citation2022). Forecasted known-unknown becomes knowable. For example, in a metaverse ‘collaboration room’, supply chain managers could
review expected sales forecasts, projected production plans and possible supplier limitations that could affect manufacturing volume. They could also see, on an immersive supply chain network map, exactly where inventory is, virtually walk through key ports to identify possible shipping delays because of congestion, and model possible alternatives to keep products moving to the right stores and shelves. (Accenture Citation2022b)
Sustainability management can also be improved through transparency about carbon emissions, visibility about the entire product life cycle, and associated environmental footprints. The digital supply chain can help in tracing the upstream suppliers to ensure that suppliers do not use child labour (e.g. by using blockchain or some product-tracking technologies) and produce products according to sustainability standards and laws.
4.3. Area 3: technology
Huynh-The et al. (Citation2023) point to six major technological elements of the metaverse, i.e. a digital twin (cyber-physical interface), neural interface (brain-computer interface), machine vision (virtual/augmented reality), networking (e.g. multi-access edge computing), blockchain (data collection, storage, sharing, and management), and natural language processing (e.g. text-to-speech processing). Bhandal et al. (Citation2022) point to the Internet of things, blockchain, artificial intelligence and data analytics, augmented and virtual reality, and Industry 4.0 as digital twin enablers. In addition, through 3D printing, production can be triggered by customers themselves. Customers can also design products and have them produced on demand (Boute et al. Citation2022; Peron et al. Citation2022). This will have implications on supply chain complexity and environmental footprints, along with increased customer satisfaction. Finally, digital platforms and supplier collaboration portals will be used to ensure collaboration and communication in Industry 5.0 (Holzwarth, Staib, and Ivanov Citation2022; Reim, Andersson, and Eckerwall Citation2023; Sawik Citation2022). End-to-end visibility, which is so important for both proactive and reactive decision-making, is supported across the supply chain by ERP systems, blockchain, and T&T systems (Choi et al. 2022a; Li et al. Citation2022; MacCarthy and Ivanov Citation2022; Roeck, Sternberg, and Hofmann Citation2020).
Digital twins can be enabled by technologies of different scopes (Boyes and Watson Citation2022; Jahani, Jain, and Ivanov Citation2023 Nguyen et al. Citation2022;). CAD/CAM (computer aided design/computer aided manufacturing) systems are applied at the product level, while MES and ERP systems enable the building of the digital twins of processes and organisations. At the supply chain level, special software such as anyLogistix in combination with external data sources (e.g. data from logistics service providers, weather data, financial market data) are used to build supply chain digital twins (Burgos and Ivanov Citation2021; Ivanov and Dolgui Citation2021). Future research areas highlight both a technical understanding of system integration and interoperability and management conceptualisation of the needs and limits of data-driven decision-making support. Technologies allow for the integration of models with external data sources and ensure interactions with other digital twins.
A specific, and very important part of future research on digital twins will be related to human-artificial intelligence collaboration. According to Ivanov (Citation2023c), three levels of digital twins can be classified: digital twin, cognitive digital twin, and intelligent digital twin. The latter type of digital twin is based on human-artificial intelligence collaboration and will therefore be relevant to the metaverse SCOM (Ivanov Citation2023b).
One particular area of human-artificial intelligence collaboration in the metaverse will be related to generative AI artificial intelligence (e.g. ChatGPT). Generative AI is expected to have a profound impact on the physical and digital supply chains taking over (or supporting) a large variety of SCOM tasks such as demand forecasting, routing optimisation, process monitoring, and risk control. All the activities related to prediction, optimisation, and anomaly/failure detection in SCOM will use generative AI.
Finally, cybersecurity issues are of utmost importance for metaverse SCOM. Multiple technologies and users of the metaverse may lead to increased SCOM cyber threats, resulting in various new cybersecurity challenges. For example, real-time metaverse SCOM applications may require new countermeasures against the new cyber threats.
4.4. Area 4: people
The metaverse will change the work and role of people in SCOM. Automatic responses with minimal human intervention, new standards for working places and remote work, collaboration of people (virtual meeting platforms), and human-robot collaboration are just some examples of this change (Rožanec et al. Citation2022; Saghafian, Tomlin, and Biller Citation2022; Sheu and Choi Citation2022; Sun et al. Citation2022). The metaverse is being developed and used by people, and at the same time it changes human behaviours and SCOM decision-making.
Decisions in SCOM depend on the expertise of the manager, the knowledge and skills they exhibit, and their access to real-time data and information (Sgarbossa et al. Citation2020). The metaverse can help managers providing decision-making support by acquiring real-time data and simulating the potential outcomes of certain decisions (e.g. alternative recovery policies after a disruption or changes in an environmental footprint due to a supply chain redesign). Digital twins can also consider the level of competence in making decisions (e.g. placing orders in an inventory control system). Most centrally, the metaverse offers real-time, data-driven decision-making support.
Further research is needed to examine the impacts of continuous access to real-time data on managerial decision-making. In addition, behavioural aspects of data-driven decision-making and cognitive biases in human-artificial intelligence interactions belong to the novel topics that will emerge when digital twins can be explored in SCOM research (Fahimnia et al. Citation2019; Fu et al. Citation2022; Sun et al. Citation2022). At the manufacturing system level, human-robot collaboration is one of the central digital twin-related future research topics (Sheu and Choi Citation2022). Mourtzis et al. (Citation2022) stress the human-centric perspective of the metaverse, echoing the integration of human-centricity, resilience, and sustainability into the Industry 5.0 framework (Ivanov Citation2022a).
4.5. Area 5: organisation
Technology determines organisation. The metaverse will not only mirror physical SCOM organisations but also create and develop new business and operational models. Through digital twins, novel organisational constructs such as digital manufacturing, cloud supply chains, and collaborative platforms will arise (Huang, Wang, and Yan Citation2022; Sharma et al. Citation2022). Examination of the metaverse-driven transformations in the organisation of SCOM can be conducted in future research areas where impactful and substantial contributions can be made. In addition, digital twins can lead to new organisational structures and a redistribution of decision-making competencies across departments. Metaverse solutions can also be applied to factory design and planning through simulation of their digital twins. In the created virtual simulation environments, processes and flows can be represented, analysed, and improved. Furthermore, new organisational forms (e.g. cloud supply chains, intertwined supply networks, ecosystems) and new categories in SCOM such as creator economy, discovery, and digital experience could appear too (Ivanov and Dolgui Citation2020).
In the context of viability, digital technology allows for the implementation of the viable supply chain model (Ivanov Citation2022a; Ivanov and Keskin Citation2023; Ruel et al. Citation2021). Visibility, reconfigurable manufacturing systems, and additive manufacturing, along with analytics and digital collaboration tools, are vital for viable manufacturing and supply chains. In light of the increasing resource shortages in physical supply chains due to component (e.g. semiconductors) shortages, workforce variability, energy blackouts, and inflation (Hägele, Grosse, and Ivanov Citation2023; Ivanov and Dolgui Citation2022b), the importance of viable supply chains and the metaverse will continue to grow in the future.
Following Ivanov (Citation2022a), ‘the Viable Supply Chain Model is based on adaptable structural network designs for situational supply-demand allocations and, most importantly, the establishment and control of adaptive mechanisms for transitions between the structural designs’. Moreover, supply chain viability and the ecosystem view have been synthesised through the lens of the human-centred ecosystem perspective by Ivanov and Dolgui (Citation2022a). In addition, the reconfigurable supply chain framework can be considered a part of future Industry 5.0 developments (Dolgui, Ivanov, and Sokolov Citation2020; Ivanov Citation2023a). Dolgui, Ivanov, and Sokolov (Citation2020) note that by
supplementing the reconfigurable manufacturing concept (Battaïa et al. Citation2020; Zennaro et al. Citation2019), the reconfigurable supply chain adds three specific features: active behaviour of network elements, networking effects across multiple structures and their dynamics (i.e. organizational, information, financial, technological, energy), and network complexity (i.e. multi-echelon supply chains). The reconfigurable supply chains are characterized by structural and process variety, which is beneficial for supply chain resilience.
4.6. Area 6: modelling
Analytics capabilities offered through the metaverse can hardly be imagined now to a full extent. Modelling in the metaverse will be based on shifting historical data-based forecasting for supply chains and operational planning methods towards real time, data-driven decisions (Figure ).
Optimisation, discrete-event simulation, neural networks, machine and reinforcement learning, agent-based modelling, and system dynamics allow for the implementation of the full variety of descriptive, predictive, and prescriptive algorithms in SCOM (Cavalcante et al. Citation2019; Choi et al. Citation2022a; Dolgui and Proth Citation2010; Fu et al. Citation2022; Kusiak Citation2023; Rai et al. Citation2021; Rolf et al. Citation2022). While real-time data-driven models constitute a narrow view of digital twins (i.e. a digital twin as a standalone software package), in a broader sense, digital twins can be considered as a combination of different information systems and models. Seamless digital and physical integration can become the centric element of the SCOM metaverse. For example, imagine a product that knows its location, inventory status, price, and costs. Using edge computing, an algorithm would trigger automatic replenishment, routing, pricing, and demand prediction decisions, thus enhancing margins, product availability, on-time delivery, and overall profitability. New computational capacities for supply chain and operations analytics and the use of synthetic data along with the industrial Internet of things can be used to predict customer and supplier behaviours in terms of demand recognition and delivery accuracy. Future research can shed more light on the transition from offline to real-time data-driven modelling, revealing its value and barriers through improved end-to-end visibility in the supply chain.
5. Conclusion
The metaverse and Web 3.0 represent new and strong triggers for the further evolution of SCOM. They not only create a new, digital world with specific properties and behaviours replicating the behaviours and processes of physical entities, but also influence physical SCOM. Despite some fragmented literature that focuses on the metaverse and SCOM, there is a lack of understanding as to how the metaverse will impact SCOM in the physical world and what the potential SCOM processes and decision-making areas in the metaverse and the mutual impacts of the co-existence of physical and metaverse SCOM will be.
Driven by these questions, our study aimed to advance our understanding of how the metaverse will impact SCOM by drawing on cyber-physical systems, digital twins, cloud and digital supply chains, and Industry 4.0/Industry 5.0 concepts. With regard to the first research question, we proposed a framework for metaverse SCOM encompassing seven socio-technological dimensions, i.e. organisation, management, people, technology, scope, task, and modelling.
Concerning the second research question, our study indicates that new research areas can appear which are specifically dedicated to the metaverse and novel SCOM processes and decision-making areas (e.g. joint demand forecasting for metaverse and physical products, digital inventory allocation in the metaverse, integrated production planning for the metaverse and physical worlds, and pricing and contracting for digital products), as well as new performance measures (e.g. virtual customer experience level, availability of digital products, and digital resilience and sustainability).
In answering the third question, our analysis shows that in the future we can expect a co-existence of physical SCOM, metaverse SCOM, and SCOM for the coordination of the physical and metaverse worlds. We offered a structured future research agenda pointing to new research questions and topics stemming from metaverse-driven visibility, computational power for data analytics, digital collaboration, and connectivity. Further research on digital technology in SCOM will contribute to the coordination of physical and metaverse supply chains and operations.
Acknowledgements
The authors thank two anonymous reviewers whose comments helped us enormously in improving the paper.
Data availability statement
Data related with this paper is available with authors and will be available upon reasonable request.
Disclosure statement
No potential conflict of interest was reported by the author(s).
Additional information
Notes on contributors
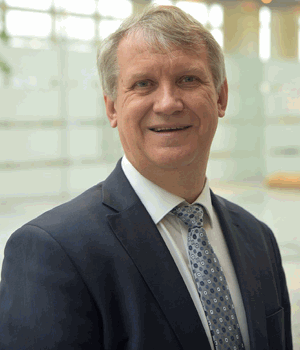
Alexandre Dolgui
Alexandre Dolgui is an IISE Fellow, Distinguished Professor, and the Head of Automation, Production and Computer Sciences Department at the IMT Atlantique, France. His research focuses on manufacturing line design, production planning and supply chain optimisation. His main results are based on the exact mathematical programming methods and their intelligent coupling with heuristics and metaheuristics algorithms. He is the co-author of 5 books, the co-editor of 27 books or conference proceedings, the author of over 300 refereed journal papers, as well as over 400 papers in conference proceedings. He is an Area Editor of Computers & Industrial Engineering, past Associate Editor of International Journal of Systems Science, IEEE Transactions on Industrial Informatics and Omega. He is Member of the Editorial Boards for 27 other journals, including the International Journal of Production Economics. He is an Active Fellow of the European Academy for Industrial Management, Member of the Board of the International Foundation for Production Research, former Chair of IFAC TC 5.2 Manufacturing Modelling for Management and Control (2011–2017, currently a vice-chair), Member of IFIP WG 5.7 Advances in Production Management Systems, IEEE System Council Analytics and Risk Technical Committee. He is the Editor-in-Chief of the International Journal of Production Research (IJPR).
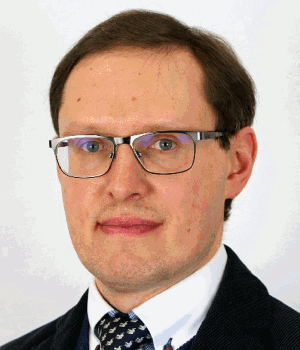
Dmitry Ivanov
Dmitry Ivanov is a professor of supply chain and operations management at Berlin School of Economics and Law. He serves at the school as an Academic director of M.A. Global Supply Chain and Operations Management and B.Sc. International Sustainability Management as well as a Deputy Director of Institute for Logistics. His publication list includes around 400 publications, including over 130 papers in international academic journals and leading textbooks Global Supply Chain and Operations Management and Introduction to Supply Chain Resilience. His main research interests and results span resilience, viability and ripple effect in supply chains, risk analytics, and digital twins. Author of the Viable Supply Chain Model and founder of the ripple effect research in supply chains. Recipient of IISE Transactions Best Paper Award 2021, Best Paper and Most Cited Paper Awards of IJPR (2018, 2019, 2020, 2021), OMEGA Best Paper Award 2022, Clarivate Highly Cited Researcher Award (2021, 2022). He co-edits IJISM and is an associate editor of the IJPR and OMEGA. He is Chairman of IFAC TC 5.2 ‘Manufacturing Modelling for Management and Control’.
References
- Accenture. 2022a. Accessed January 12, 2023. https://www.accenture.com/us-en/insights/technology/technology-trends-2022.
- Accenture. 2022b. Accessed January 12, 2023. https://www.accenture.com/us-en/blogs/business-functions-blog/metaverse-supply-chain-networks.
- Aldrighetti, R., D. Battini, and D. Ivanov. 2023. “Efficient Resilience Portfolio Design in the Supply Chain with Consideration of Preparedness and Recovery Investments.” Omega 117: 103841.
- Aldrighetti, R., D. Battini, D. Ivanov, and I. Zennaro. 2021. “Costs of Resilience and Disruptions in Supply Chain Network Design Models: A Review and Future Research Directions.” International Journal of Production Economics 235: 108103. https://doi.org/10.1016/j.ijpe.2021.108103.
- Badakhshan, E., and P. Ball. 2023. “Applying Digital Twins for Inventory and Cash Management in Supply Chains under Physical and Financial Disruptions.” International Journal of Production Research 61 (15): 5094–5116.
- Barrera, K. G., and D. Shah. 2023. “Marketing in the Metaverse: Conceptual Understanding, Framework, and Research Agenda.” Journal of Business Research 155A: 113420. https://doi.org/10.1016/j.jbusres.2022.113420
- Battaïa, O., L. Benyoucef, X. Delorme, A. Dolgui, and S. Thevenin. 2020. “Sustainable and Energy Efficient Reconfigurable Manufacturing Systems.” Reconfigurable Manufacturing Systems: From Design to Implementation, Springer, 179–191. https://doi.org/10.1007/978-3-030-28782-5_9.
- Berti, N., and S. Finco. 2022. “Digital Twin and Human Factors in Manufacturing and Logistics Systems: State of the Art and Future Research Directions.” IFAC-PapersOnLine 55 (10): 1893–1898. https://doi.org/10.1016/j.ifacol.2022.09.675.
- Bhandal, R., R. Meriton, R. E. Kavanagh, and A. Brown. 2022. “The Application of Digital Twin Technology in Operations and Supply Chain Management: A Bibliometric Review.” Supply Chain Management 27 (2): 182–206. https://doi.org/10.1108/SCM-01-2021-0053.
- Boute, R. N., S. M. Disney, J. Gijsbrechts, and J. A. Van Mieghem. 2022. “Dual Sourcing and Smoothing under Nonstationary Demand Time Series: Reshoring with Speedfactories.” Management Science 68 (2): 1039–1057. https://doi.org/10.1287/mnsc.2020.3951.
- Boyes, H., and T. Watson. 2022. “Digital Twins: An Analysis Framework and Open Issues.” Computers in Industry 143: 103763. https://doi.org/10.1016/j.compind.2022.103763.
- Brintrup, A., J. Pak, D. Ratiney, T. Pearce, P. Wichmann, P. Woodall, and D. McFarlane. 2020. “Supply Chain Data Analytics for Predicting Supplier Disruptions: A Case Study in Complex Asset Manufacturing.” International Journal of Production Research 58 (11): 3330–3341. https://doi.org/10.1080/00207543.2019.1685705.
- Burgos, D., and D. Ivanov. 2021. “Food Retail Supply Chain Resilience and the COVID-19 Pandemic: A Digital Twin-based Impact Analysis and Improvement Directions.” Transportation Research – Part E: Logistics and Transportation Review 152: 102412. https://doi.org/10.1016/j.tre.2021.102412.
- Cai, Y., T. M. Choi, and J. Zhang. 2021. “Platform Supported Supply Chain Operations in the Blockchain Era: Supply Contracting and Moral Hazards.” Decision Sciences 52 (4): 866–892. https://doi.org/10.1111/deci.12475.
- Camarinha-Matos, L. M., and H. Afsarmanesh. 2005. “Collaborative Networks: A New Scientific Discipline.” Journal of Intelligent Manufacturing 16 (4–5): 439–452. https://doi.org/10.1007/s10845-005-1656-3.
- Catena. 2022. Accessed December 14, 2022. https://catena-x.net/de/.
- Cavalcante, I. M., E. M. Frazzon, F. A. Forcellinia, and D. Ivanov. 2019. “A Supervised Machine Learning Approach to Data-driven Simulation of Resilient Supplier Selection in Digital Manufacturing.” International Journal of Information Management 49: 86–97. https://doi.org/10.1016/j.ijinfomgt.2019.03.004.
- Chabanet, S., H. Bril El-Haouzi, M. Morin, J. Gaudreault, and P. Thomas. 2023. “Toward Digital Twins for Sawmill Production Planning and Control: Benefits, Opportunities, and Challenges.” International Journal of Production Research 61 (7): 2190–2213.
- Choi, T. M., A. Dolgui, D. Ivanov, and E. Pesch. 2022a. “OR and Analytics for Digital, Resilient, and Sustainable Manufacturing 4.0.” Annals of Operations Research 310 (1): 1–6. https://doi.org/10.1007/s10479-022-04536-3.
- Choi, T. M., S. Kumar, X. Yue, and H. L. Chan. 2022b. “Disruptive Technologies and Operations Management in the Industry 4.0 Era and Beyond.” Production and Operations Management 31 (1): 9–31. https://doi.org/10.1111/poms.13622.
- Cui, R., M. Li, and S. Zhang. 2022. “AI and Procurement.” Manufacturing & Service Operations Management 24 (2): 691–706. https://doi.org/10.1287/msom.2021.0989.
- Dolgui, A., and D. Ivanov. 2022. “5G in Digital Supply Chain and Operations Management: Fostering Flexibility, End-to-end Connectivity and Real-time Visibility through Internet-of-everything.” International Journal of Production Research 60 (2): 442–451. https://doi.org/10.1080/00207543.2021.2002969.
- Dolgui, A., D. Ivanov, and B. Sokolov. 2020. “Reconfigurable Supply Chain: The X-network.” International Journal of Production Research 58 (13): 4138–4163. https://doi.org/10.1080/00207543.2020.1774679.
- Dolgui, A., and J.-M. Proth. 2010. Supply Chain Engineering: Useful Methods and Techniques. London: Springer.
- Dubey, R., A. Gunasekaran, S. J. Childe, S. F. Wamba, D. Roubaud, and C. Foropon. 2021. “Empirical Investigation of Data Analytics Capability and Organizational Flexibility as Complements to Supply Chain Resilience.” International Journal of Production Research 59 (1): 110–128. https://doi.org/10.1080/00207543.2019.1582820.
- The Economist. 2020. “A Novelist’s Vision of the Virtual World has Inspired an Industry.” Accessed January 4, 2023. https://www.economist.com/technology-quarterly/2020/10/01/a-novelists-vision-of-the-virtual-world-has-inspired-an-industry.
- Elmachtoub, A. N., and P. Grigas. 2022. “Smart ‘Predict, Then Optimize’.” Management Science 68 (1): 9–26. https://doi.org/10.1287/mnsc.2020.3922.
- Fahimnia, B., M. Pournader, E. Siemsen, E. Bendoly, and C. Wang. 2019. “Behavioral Operations and Supply Chain Management–a Review and Literature Mapping.” Decision Sciences 50 (6): 1127–1183. https://doi.org/10.1111/deci.12369.
- Frazzon, E. M., M. Freitag, and D. Ivanov. 2021. “Intelligent Methods and Systems for Decision-making Support: Toward Digital Supply Chain Twins.” International Journal of Information Management 57: 102281. https://doi.org/10.1016/j.ijinfomgt.2020.102281.
- Fu, R., M. Aseri, P. V. Singh, and K. Srinivasan. 2022. ““Un”Fair Machine Learning Algorithms.” Management Science 68 (6): 4173–4195. https://doi.org/10.1287/mnsc.2021.4065.
- Hägele, S., E. Grosse, and D. Ivanov. 2023. “Supply Chain Resilience: A Tertiary Study.” International Journal of Integrated Supply Management 16 (1): 52–81. https://doi.org/10.1504/IJISM.2023.127660.
- Holzwarth, A., C. Staib, and D. Ivanov. 2022. “Building Viable Digital Business Ecosystems with Collaborative Supply Chain Platform SupplyOn.” In Supply Network Dynamics and Control, edited by A. Dolgui, D. Ivanov, and B. Sokolov, 187–210. Cham: Springer.
- Huang, S., G. Wang, and Y. Yan. 2022. “Building Blocks for Digital Twin of Reconfigurable Machine Tools from Design Perspective.” International Journal of Production Research 60 (3): 942–956. https://doi.org/10.1080/00207543.2020.1847340.
- Huynh-The, T., Q. V. Pham, X. Q. Pham, T. T. Nguyen, Z. Han, and D. S. Kim. 2023. “Artificial Intelligence for the Metaverse: A Survey.” Engineering Applications of Artificial Intelligence 117 (A): 105581. https://doi.org/10.1016/j.engappai.2022.105581.
- Ivanov, D. 2021. “Digital Supply Chain Management and Technology to Enhance Resilience by Building and Using End-to-end Visibility During the COVID-19 Pandemic.” IEEE Transactions on Engineering Management. https://doi.org/10.1109/TEM.2021.3095193.
- Ivanov, D. 2022a. “Viable Supply Chain Model: Integrating Agility, Resilience and Sustainability Perspectives – Lessons from and Thinking Beyond the COVID-19 Pandemic.” Annals of Operations Research 319: 1411–1431. https://doi.org/10.1007/s10479-020-03640-6.
- Ivanov, D. 2022b. “Blackout and Supply Chains: Performance, Resilience and Viability Impact Analysis.” Annals of Operations Research, https://doi.org/10.1007/s10479-022-04754-9.
- Ivanov, D. 2023a. “The Industry 5.0 Framework: Viability-based Integration of the Resilience, Sustainability, and Human-centricity Perspectives.” International Journal of Production Research 61 (5): 1683–1695. https://doi.org/10.1080/00207543.2022.2118892.
- Ivanov, D. 2023b. “Conceptualisation of a 7-element Digital Twin Framework in Supply Chain and Operations Management.” International Journal of Production Research, 1–13. https://doi.org/10.1080/00207543.2023.2217291.
- Ivanov, D. 2023c. “Intelligent Digital Twin (iDT) for Supply Chain Stress-testing and Resilience Analysis.” International Journal of Production Economics 263: 108938. https://doi.org/10.1016/j.ijpe.2023.108938.
- Ivanov, D., and A. Dolgui. 2020. “Viability of Intertwined Supply Networks: Extending the Supply Chain Resilience Angles towards Survivability. A Position Paper Motivated by COVID-19 Outbreak.” International Journal of Production Research 58 (10): 2904–2915. https://doi.org/10.1080/00207543.2020.1750727.
- Ivanov, D., and A. Dolgui. 2021. “A Digital Supply Chain Twin for Managing the Disruptions Risks and Resilience in the era of Industry 4.0.” Production Planning and Control 32 (9): 775–788. https://doi.org/10.1080/09537287.2020.1768450.
- Ivanov, D., and A. Dolgui. 2022a. “Stress Testing Supply Chains and Creating Viable Ecosystems.” Operations Management Research 15: 475–486. https://doi.org/10.1007/s12063-021-00194-z.
- Ivanov, D., and A. Dolgui. 2022b. “The Shortage Economy and its Implications for Supply Chain and Operations Management.” International Journal of Production Research 60 (24): 7141–7154. https://doi.org/10.1080/00207543.2022.2118889.
- Ivanov, D., A. Dolgui, and B. Sokolov. 2022. “Cloud Supply Chain: Integrating Industry 4.0 and Digital Platforms in the ‘Supply Chain-as-a-service’.” Transportation Research – Part E: Logistics and Transportation Review 160:102676. https://doi.org/10.1016/j.tre.2022.102676.
- Ivanov, D., and B. Keskin. 2023. “Post-pandemic Adaptation and Development of Supply Chain Viability Theory.” Omega 116: 102806. https://doi.org/10.1016/j.omega.2022.102806.
- Ivanov, D., C. S. Tang, A. Dolgui, D. Battini, and A. Das. 2021a. “Researchers’ Perspectives on Industry 4.0: Multi-disciplinary Analysis and Opportunities for Operations Management.” International Journal of Production Research 59 (7): 2055–2078. https://doi.org/10.1080/00207543.2020.1798035.
- Ivanov, D., A. Tsipoulanidis, and J. Schönberger. 2021b. Global Supply Chain and Operations Management: A Decision-oriented Introduction into the Creation of Value. 3rd ed. Cham: Springer Nature.
- Jahani, H., R. Jain, and D. Ivanov. 2023. “Data Science and Big Data Analytics: A Systematic Review of Methodologies Used in the Supply Chain and Logistics Research.” Annals of Operations Research. https://doi.org/10.1007/s10479-023-05390-7.
- Kathiala, R. 2022. “Look out Supply Chain—Here comes the Metaverse.” Accessed January 7, 2023. https://www.scmr.com/article/look_out_supply_chain._here_comes_the_metaverse.
- Kusiak, A. 2020. “Open Manufacturing: A Design-for-resilience Approach.” International Journal of Production Research 58 (15): 4647–4658. https://doi.org/10.1080/00207543.2020.1770894.
- Kusiak, A. 2023. “Predictive Models in Digital Manufacturing: Research, Applications, and Future Outlook.” International Journal of Production Research 61 (17): 6052–6062.
- Lee, J., and P. Kundu. 2022. “Integrated Cyber-physical Systems and Industrial Metaverse for Remote Manufacturing.” Manufacturing Letters 34: 12–15. https://doi.org/10.1016/j.mfglet.2022.08.012.
- Li, G., J. Xue, N. Li, and D. Ivanov. 2022. “Blockchain-supported Business Model Design, Supply Chain Resilience, and Firm Performance.” Transportation Research – Part E: Logistics and Transportation Review 163: 102773. https://doi.org/10.1016/j.tre.2022.102773.
- Liu, C., P. Jiang, and W. Jiang. 2020. “Web-based Digital Twin Modeling and Remote Control of Cyber-physical Production Systems.” Robotics and Computer-Integrated Manufacturing 64:101956. https://doi.org/10.1016/j.rcim.2020.101956.
- Lovich, D. 2022. “What is the Metaverse and Why should You Care?” Accessed January 5, 2023. https://www.forbes.com/sites/deborahlovich/2022/05/11/what-is-the-metaverse-and-why-should-you-care/?sh=1784d21b2704.
- Lv, Z., L. Qiao, A. Mardani, and H. Lv. 2022. “Digital Twins on the Resilience of Supply Chain Under COVID-19 Pandemic.” IEEE Transactions on Engineering Management, 1–12. https://doi.org/10.1109/TEM.2022.3195903.
- MacCarthy, B., W. Ahmed, and G. Demirel. 2022. “Mapping the Supply Chain: Why, What and How?” International Journal of Production Economics 250: 108688.
- MacCarthy, B., and D. Ivanov. 2022. “The Digital Supply Chain—Emergence, Concepts, Definitions, and Technologies.” In The Digital Supply Chain, edited by B. MacCarthy and D. Ivanov, 3–14. Amsterdam: Elsevier.
- Maersk. 2022. Accessed January 14, 2023. https://www.maersk.com/insights/digitalisation/how-the-metaverse-will-transform-supply-chain-management.
- Meier, O., T. Gruchmann, and D. Ivanov. 2023. “Circular Supply Chain Management with Blockchain Technology: A Dynamic Capabilities View.” Transportation Research: Part E 176: 103177. https://doi.org/10.1016/j.tre.2023.103177.
- Moghaddam, M., and S. Y. Nof. 2018. “Collaborative Service-component Integration in Cloud Manufacturing.” International Journal of Production Research 56 (1–2): 677–691. https://doi.org/10.1080/00207543.2017.1374574.
- Mourtzis, D., N. Panopoulos, J. Angelopoulos, B. Wang, and L. Wang. 2022. “Human Centric Platforms for Personalized Value Creation in Metaverse.” Journal of Manufacturing Systems 65: 653–659. https://doi.org/10.1016/j.jmsy.2022.11.004.
- Negri, E., L. Fumagalli, and M. Macchi. 2017. “A Review of the Roles of Digital Twin in CPS-based Production Systems.” Procedia Manufacturing 11 (2017): 939–948. https://doi.org/10.1016/j.promfg.2017.07.198.
- Nguyen, T., Q. H. Duong, T. V. Nguyen, Y. Zhu, and L. Zhou. 2022. “Knowledge Mapping of Digital Twin and Physical Internet in Supply Chain Management: A Systematic Literature Review.” International Journal of Production Economics 244: 108381. https://doi.org/10.1016/j.ijpe.2021.108381.
- Open Manufacturing. 2022. Accessed January 4, 2022. https://open-manufacturing.org/.
- Pan, S., E. Ballot, G. Q. Huang, and B. Montreuil. 2017. “Physical Internet and Interconnected Logistics Services: Research and Applications.” International Journal of Production Research 55 (9): 2603–2609. https://doi.org/10.1080/00207543.2017.1302620.
- Panetto, H., B. Iung, D. Ivanov, G. Weichhart, and X. Wang. 2019. “Challenges for the Cyber-physical Manufacturing Enterprises of the Future.” Annual Reviews in Control 47: 200–213. https://doi.org/10.1016/j.arcontrol.2019.02.002.
- Peron, M., R. Basten, N. Knofius, F. Lolli, and F. Sgarbossa. 2022. “Additive or Conventional Manufacturing for Spare Parts: Effect of Failure Rate Uncertainty on the Sourcing Option Decision.” IFAC PapersOnLine 55 (10): 1141–1146. https://doi.org/10.1016/j.ifacol.2022.09.543.
- Rai, R., M. K. Tiwari, D. Ivanov, and A. Dolgui. 2021. “Machine Learning in Manufacturing and Industry 4.0 Applications.” International Journal of Production Research 59 (16): 4773–4778. https://doi.org/10.1080/00207543.2021.1956675.
- Ralston, P., and J. Blackhurst. 2020. “Industry 4.0 and Resilience in the Supply Chain: A Driver of Capability Enhancement or Capability Loss?” International Journal of Production Research 58 (16): 5006–5019. https://doi.org/10.1080/00207543.2020.1736724.
- Reim, W., E. Andersson, and K. Eckerwall. 2023. “Enabling Collaboration on Digital Platforms: A Study of Digital Twins.” International Journal of Production Research 61 (12): 3926–3942.
- Roeck, D., H. Sternberg, and E. Hofmann. 2020. “Distributed Ledger Technology in Supply Chains: A Transaction Cost Perspective.” International Journal of Production Research 58 (7): 2124–2141. https://doi.org/10.1080/00207543.2019.1657247.
- Rolf, B., I. Jackson, M. Müller, S. Lang, T. Reggelin, and D. Ivanov. 2022. “A Review on Reinforcement Learning Algorithms and Applications in Supply Chain Management.” International Journal of Production Research. https://doi.org/10.1080/00207543.2022.2140221.1.
- Rožanec, J. M., J. Lu, J. Rupnik, M. Škrjanc, D. Mladenić, B. Fortuna, X. Zheng, and D. Kiritsis. 2022. “Actionable Cognitive Twins for Decision Making in Manufacturing.” International Journal of Production Research 60 (2): 452–478. https://doi.org/10.1080/00207543.2021.2002967.
- Ruel, S., J. El Baz, D. Ivanov, and A. Das. 2021. “Supply Chain Viability: Conceptualization, Measurement, and Nomological Validation.” Annals of Operations Research. https://doi.org/10.1007/s10479-021-03974-9.
- Saghafian, S., B. Tomlin, and S. Biller. 2022. “The Internet of Things and Information Fusion: Who Talks to Who?” Manufacturing & Service Operations Management 24 (1): 333–351. https://doi.org/10.1287/msom.2020.0958.
- Sawik, T. 2022. “A Linear Model for Optimal Cybersecurity Investment in Industry 4.0 Supply Chains.” International Journal of Production Research 60 (4): 1368–1385. https://doi.org/10.1080/00207543.2020.1856442.
- Sgarbossa, F., E. H. Grosse, W. P. Neumann, D. Battini, and C. H. Glock. 2020. “Human Factors in Production and Logistics Systems of the Future.” Annual Reviews in Control 49: 295–305. https://doi.org/10.1016/j.arcontrol.2020.04.007.
- Sharma, A., E. Kosasih, J. Zhang, B. Brintrup, and A. Calinescu. 2022. “Digital Twins: State of the art Theory and Practice, Challenges, and Open Research Questions.” Journal of Industrial Information Integration 30: 100383. https://doi.org/10.1016/j.jii.2022.100383.
- Sheu, J. B., and T.-M. Choi. 2022. “Can We Work More Safely and Healthily with Robot Partners? A Human-friendly Robot-human Coordinated Order Fulfillment Scheme.” Production and Operations Management 32: 794–812. https://doi.org/10.1111/poms.13899.
- Siemens. 2022. MindSphere. Accessed January 4, 2022. https://siemens.mindsphere.io/en.
- Stephenson, N. 1992. Snow Crash. New York: Bantam Books.
- Sun, J., D. J. Zhang, H. Hu, and J. A. V. Mieghem. 2022. “Predicting Human Discretion to Adjust Algorithmic Prescription: A Large-scale Field Experiment in Warehouse Operations.” Management Science 68 (2): 846–865. https://doi.org/10.1287/mnsc.2021.3990.
- Tang, C. S., and L. P. Veelenturf. 2019. “The Strategic Role of Logistics in the Industry 4.0 era.” Transportation Research Part E: Logistics and Transportation Review 129: 1–11. https://doi.org/10.1016/j.tre.2019.06.004.
- Winkelhaus, S., and E. H. Grosse. 2020. “Logistics 4.0: A Systematic Review towards a New Logistics System.” International Journal of Production Research 58 (1): 18–43. https://doi.org/10.1080/00207543.2019.1612964.
- Yin, Y., K. E. Stecke, and D. Li. 2018. “The Evolution of Production Systems from Industry 2.0 through Industry 4.0.” International Journal of Production Research 56 (1–2): 848–861. https://doi.org/10.1080/00207543.2017.1403664.
- Zennaro, I., S. Finco, D. Battini, and A. Persona. 2019. “Big Size Highly Customised Product Manufacturing Systems: A Literature Review and Future Research Agenda.” International Journal of Production Research 57 (15–16): 5362–5385. https://doi.org/10.1080/00207543.2019.1582819.
- Zhang, G., B. MacCarthy, and D. Ivanov. 2022. “The Cloud, Platforms, and Digital Twins—Enablers of the Digital Supply Chain.” In The Digital Supply Chain, edited by B. MacCarthy and D. Ivanov, 77–91. Amsterdam: Elsevier.