Abstract
The transformation of production mode leads to the need to strictly ensure the order in-time delivery. The global control ability of manufacturing workshops has become a necessary capability for enterprises. The real-time accurate Order Remaining Completion Time (ORCT) prediction allows managers to master the production schedule fluctuation, which provides criteria for workshop management. Due to the widespread deployment of Internet of Things (IoT) devices in the workshop, the difficulty of systematic processing and analysis of multi-dimensional heterogeneous data has been the main pain point of ORCT prediction. To tackle these problems, a Manufacturing Big Data (MBD)-driven ORCT prediction method based on Salp Swarm Algorithm (SSA) and Bidirectional Long Short-Term Memory (BiLSTM) is proposed. An attribute selection algorithm combined with max-relevance and min-redundancy and regularisation is used to mine key data from MBD. An SSA-BiLSTM model is proposed to achieve efficient and accurate ORCT prediction. The prediction model is supported by BiLSTM, and the hyperparameters are optimised by SSA. Finally, a case study about ORCT prediction in the IoT-enabled manufacturing workshop is presented. The result verifies that the proposed ORCT prediction method has obvious advantages over the other three traditional methods in accuracy and efficiency.
Disclosure statement
No potential conflict of interest was reported by the author(s).
Data availability statement
Raw data were generated at Navicat 15 for MySQL. Derived at a supporting the findings of this study are available from the corresponding author Zhu on request.
Additional information
Funding
Notes on contributors
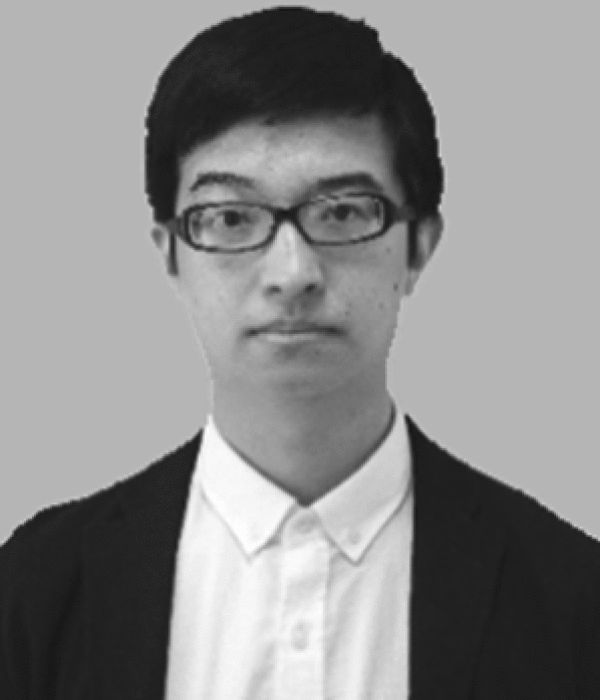
Haihua Zhu
Haihua Zhu was born in Ningbo, Zhejiang, China in 1985. He received the Ph.D. degree in mechanical engineering and automation from Nanjing University of Science and Technology, Nanjing, Jiangsu, China, in 2013.From 2009 to 2011, he was a Research Scholar with the University of Greenwich, London, UK. He is currently an Associate Professor with the College of Mechanical and Electrical Engineering, Nanjing University of Aeronautics and Astronautics, Nanjing, Jiangsu, China. His research interest includes smart manufacturing systems, digital twin, and industrial internet of things.
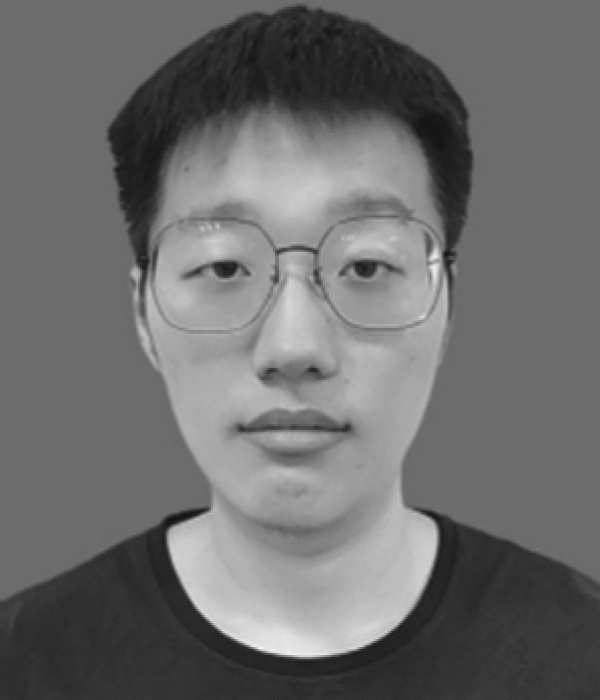
Jianjie Wang
Jianjie Wang was born in Beijing, China in 1999. He received the B.S. degree in mechanical engineering from Nanjing University of Aeronautics and Astronautics, Nanjing, Jiangsu, China, in 2022. He is currently pursuing the M.S. degree in mechanical engineering at Nanjing University of Aeronautics and Astronautics, Nanjing, Jiangsu, China. His research interest includes big data, network collaborative production, and industrial artificial intelligence.
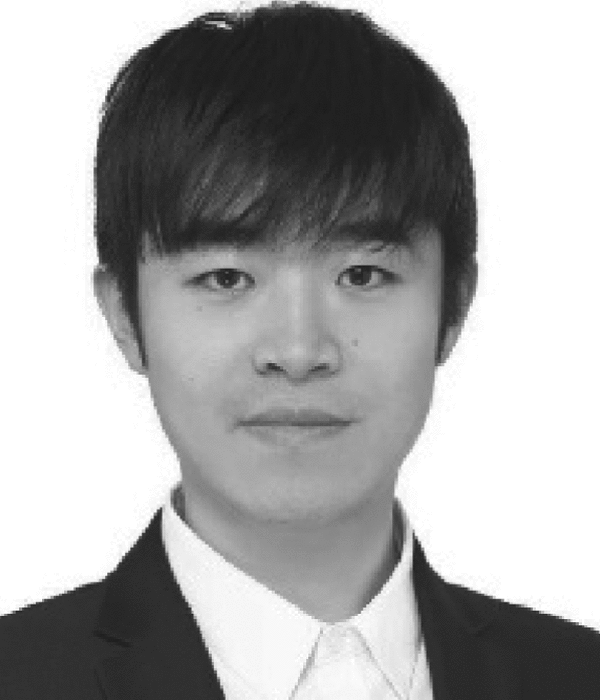
Changchun Liu
Changchun Liu was born in Yangzhou, Jiangsu, China in 1995. He received the B.S. degree in electric engineering from the Yancheng Institute of Technology, Yancheng, China, in 2017, and the M.S. degree in mechanical engineering from the Shanghai Polytechnic University, Shanghai, in 2020. He is currently pursuing the Ph.D. degree in mechanical and electrical engineering at Nanjing University of Aeronautics and Astronautics, Nanjing, Jiangsu, China. His research interest includes big data, industrial internet of things, and industrial artificial intelligence.
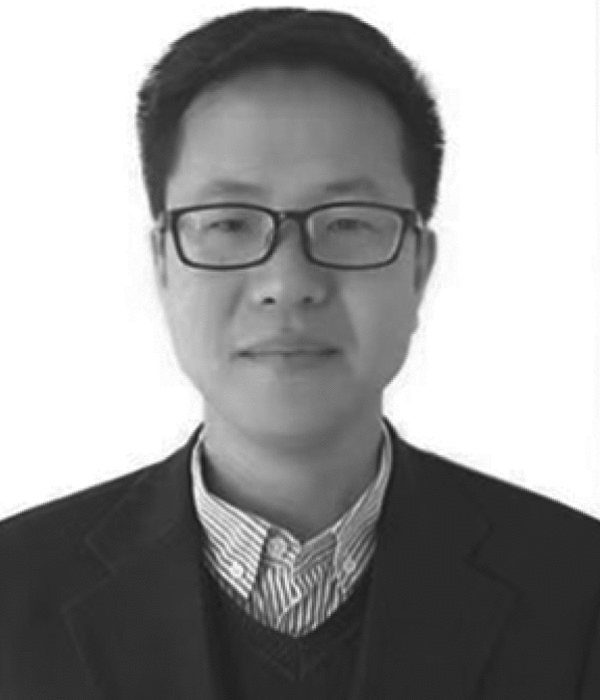
Wei Shi
Wei Shi was born in Weinan, Shaanxi, China in 1981. He is the director of Platform Research and Development Department of Beijing AEROSPACE SMART Manufacturing Technology Development Co., Ltd. He is currently pursuing the Ph.D. degree in mechanical and electrical engineering at Nanjing University of Aeronautics and Astronautics, Nanjing, Jiangsu, China. His research interest includes smart manufacturing systems, industrial internet.
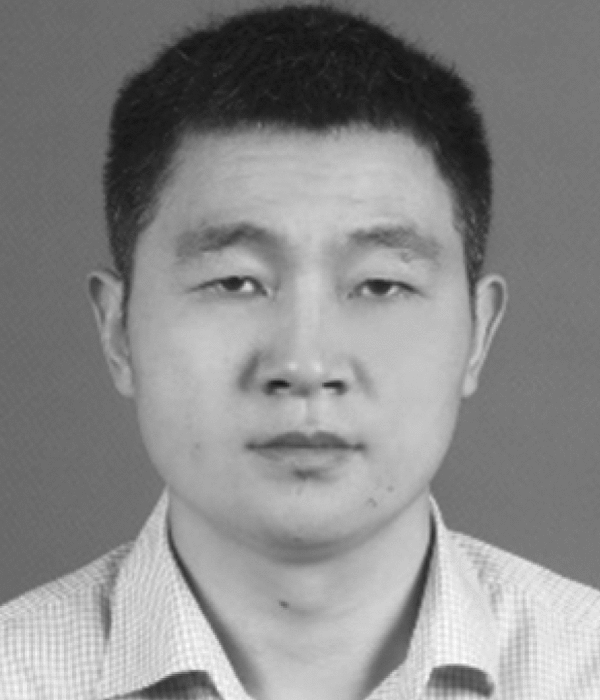
Qixiang Cai
Qixiang Cai received the Ph.D. degree in mechanical and electrical engineering from Nanjing University of Aeronautics and Astronautics, Nanjing, Jiangsu, China, in 2020. He is currently an Associate Researcher of Nanjing University of Aeronautics and Astronautics, Nanjing, Jiangsu, China. His research interest includes smart manufacturing systems, industrial internet and industrial internet of things sensing technology.