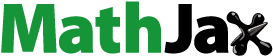
ABSTRACT
Learning-by-doing is an important concept in economics as it is one of the main driving forces of economic progress. However, corporate learning is a slow process, so outsourcing manufacturing can override the long-term benefits of accumulating productivity knowledge. Semiconductor industry is also strongly featured by the learning effect, however, due to high capital requirements of building fabs, there are companies that fully outsource, while others try to keep production in-house. The chosen business model now gets further importance due to bottlenecks and supply issues perceived in the industry since the COVID-19 outbreak. Accordingly, we model the make-or-buy decision problem of a firm that decides on the optimal mix of outsourcing and in-house production, considering productivity gain via learning-by-doing and the economic impact of resilience. By presenting numerical simulations, we aim to capture the pre-pandemic and the current state of the semiconductor industry. Our model highlights that tendencies of being fabless were reasonable in the past. Although recent industry conditions have made in-house production more beneficial, this optimal strategy may be fragile due to changes of relative production costs or the extent of economic impact of chip shortages, which may underline the relevance of government incentives aiming to revitalise semiconductor industry.
1. Introduction
In 1956, the Nobel prize was received by Bell Labs scientists John Bardeen, Walter Brattain, and William Shockley for their research on semiconductors and the invention of the transistor. Sixty-five years later, the industry developed upon their discovery accounts for USD 600 billion and chips and microelectronics are pivotal elements of numerous devices, ranging from home appliances and cars to entertainment and telecommunication devices. With the evolution of the industry, two pure production models emerged: integrated device manufacturers (IDM) kept production in-house, i.e. close to R&D and development facilities, while the fabless companies offshored it to contract manufacturers called foundries to gain competitive advantage through lower operation and capital expenses. Even though market leaders are IDMs like Intel and Samsung, the fabless model is also widespread, the Taiwanese foundry TSMC being the third largest firm by revenue, and second largest one by capacity. Accordingly, despite semiconductors have become one of the most traded goods in the international economy in the past decades, combined share of U.S., Europe, and Japan in production dropped by 40 percentage points between 1995 and 2020, resulting in an equivalent rise of capacities in China, Taiwan, and South Korea.
Semiconductor industry was also no exception to the turmoil experienced in global supply chains recently, moreover, today there is increased attention to shortages of chips as product lines in numerous industries had to stop or operate at reduced speed. As buying from third-party suppliers became more and more difficult, increasing lead times spilled over-dependent industries, resulting in further delays. It seems to be an obvious solution to extend capacities to meet excess demand, though, building up factories from scratch usually takes years. Until then, OEMs are obliged to find a solution to ease their dependency on semiconductors. For example, in the automotive industry, where one car typically contains thousands of chips, vehicles are now assembled without some extra features, like USB ports or seat heating (Walsh Citation2022).
Governments are also keen to find a solution. ‘There is no digital without chips’, said Ursula von der Leyen, president of the European Commission in her State of the Union speech in 2021, addressing the importance of the semiconductor industry and the issue of lagging behind the Asian countries regarding installed capacities (EC Citation2021). CHIPS and Science Act was signed into law in the U.S. in 2022, which also aims to strengthen domestic manufacturing, design, and research in the American semiconductor industry. Despite these, gaining back Western dominance seems extremely challenging. First, the industry is characterised by extremely high capital intensity, requirement of high-skilled workers and rapid technological change. Second, the technological and organisational knowledge accumulated via learning-by-doing results in a ‘success-breeds-success’ process making the situation of lagging companies or economies even harder.
Although the semiconductor industry has been widely investigated in the literature, to the best of our knowledge, make-or-buy decisions considering learning effect have not yet been discussed particularly. To fill this research gap, we first introduce a theoretical model that captures the dynamics and consequences of make-or-buy decisions in the semiconductor industry. Second, we impute industrial data like learning rate, purchasing price, life cycle length, manufacturing and fixed costs, initial production history, salvage value of accumulated productivity knowledge, and economic impact of chip shortages into the model that makes us able to evaluate the pre-pandemic and the current state of the industry. Accordingly, our findings may be applicable in strategic decision-making, such as investment and capacity planning, while incorporating resilience into our model provides further insights on supply chain vulnerabilities and flexibility of production strategies. Our results may be also applied in shaping government policies and incentives that support domestic semiconductor production. Furthermore, as the theoretical model is generally applicable to similar industries, the results may be relevant for other businesses such as consumer electronics and pharmaceutical products.
The rest of the paper is structured as follows. The next section provides a brief overview of the literature, followed by the characteristics of the semiconductor industry. The model is introduced in the fourth section, while section five presents the dynamic optimisation problem. The numerical simulations describing the industry are found in section six, followed by discussion of the results. The last section gives conclusion and lists some ideas for further research.
2. Literature review
Our research belongs to the broad stream of modelling learning-by-doing, presented thoroughly by Jaber (Citation2006), however, considering learning effect in make-or-buy decisions is less common. In this regard, Deng, Guan, and Xu (Citation2021) developed a model to assess the learning effect in case of two competing original equipment manufacturers (OEM) and a common contract manufacturer (CM). The authors showed that it may be beneficial for competitors to assign the same CM as the increased level of production (and the higher intensity of learning) can result in lower contract prices. Gray, Tomlin, and Roth (Citation2009) introduce a two-period strategic model with a buyer and a supplier to analyse make-or-buy decisions about a single product or component. Xiao and Gaimon (Citation2013) study a game-theoretic model similar to Gray, Tomlin, and Roth (Citation2009), but they add the future value of production experience to capture its value in developing new products. They give conditions under which the buyer pursues a partial outsourcing solution whereby the buyer is able to exploit the immediate reduction of production costs thanks to outsourcing to a supplier and enjoy the future benefits from production at the same time. Vörös (Citation2013) also accounts for the salvage value of production history, assuming the accumulated productivity knowledge can be sold at the end of the planning period. Anderson and Parker (Citation2002) show in their analytical and numerical analysis that companies may be better off without outsourcing if product modularity is low and the component outsourced is a highly integral part of the product. Yeung, Lai, and Yee (Citation2007) highlight the role of organisational learning in the productivity of firms, showing evidence that performance growth through learning is unlikely to happen for manufacturers who produce only mature products or follow a low-cost strategy, i.e. outsource the production of products with low modularity. Considering interdependence between product development and production, several studies argue that offshoring manufacturing may result in the decrease of innovation capabilities (e.g. Mazzola, Bruccoleri, and Perrone Citation2019). However, its extent may depend on the process modularity and the maturity of the applied technology (Longauer, Hauck, and Vasvári Citation2023). For example, although technology is evolving rapidly in case of semiconductors, transaction cost from chip design to fabrication is low (Shin, Kraemer, and Dedrick Citation2016), thus, locating design near manufacturing is not a must (Pisano and Shih Citation2012). By investigating entrepreneurial activity in the semiconductor industry, Funk and Luo (Citation2015) found also that specialisation, standardisation between design and production further contributed to the rise of start-ups and may also explain how the outsourcing companies along with contract manufacturers can compete in terms of innovation and applying leading-edge technology (e.g. Fitch Citation2020).
The semiconductor industry is explored from several other aspects in the literature, like knowledge creation (Paier, Dünser, and Unger Citation2017), knowledge transfer (Appleyard Citation1996; Liao and Hu Citation2007), pricing (Asmat Citation2021), capacity planning (Biwer and Arikan Citation2018), or supply chain configuration (Chiang et al. Citation2007). The evolution of the industry is described in detail by Scott and Angel (Citation1987), Brown and Linden (Citation2005), Bown (Citation2020), and Thorbecke (Citation2021), while Mathews (Citation1997) and Chang and Wu (Citation2021) investigate the Taiwanese developments. Learning effect is analysed thoroughly by Dick (Citation1991) and Irwin and Klenow (Citation1994) and found that manufacturing costs of semiconductors typically drop by 20 percent as production quantity doubles. Terwiesch and Bohn (Citation2001) add that beyond learning-by-doing, the importance of learning by experimentation (engineering trials) is also important. Regarding the semiconductor manufacturing, Hatch and Mowery (Citation1998) and Manda and Uzsoy (Citation2021) show that learning is indeed not solely a function of production volume and it may be enhanced by involving engineering labour, especially in case of product transitions. Make-or buy and SCM considerations in the industry are captured by Mönch, Uzsoy, and Fowler in their series of three papers, covering areas such as strategic network design (Mönch, Uzsoy, and Fowler Citation2018a), demand and capacity planning (Uzsoy, Fowler, and Mönch Citation2018) or master planning and demand fulfilment (Mönch, Uzsoy, and Fowler Citation2018b). Ramani, Ghosh, and Sodhi (Citation2022) explored how disruptions in the semiconductor supply chain affected the automotive industry. In this regard, some articles emphasise the role of flexibility and redundancy of supply chains to minimise expected cost and maximise service delivery (Kamalahmadi, Shekarian, and Parast Citation2022; Shekarian and Parast Citation2021). Furthermore, Chien and Kuo (Citation2013) investigate the role of make-or-buy decisions in short-term capacity planning, while Wu and Chien (Citation2008) discuss outsourcing decisions of assembly functions. Section 3 provides additional details on how make-or-buy decisions shaped the industry in recent decades.
In light of the recent industrial incentives to revitalise semiconductor manufacturing in developed regions, our study also relates to reshoring literature. The motives of partial or full relocation of previously offshored activities can be various, e.g. recognition of a managerial mistake (e.g. Gray et al. Citation2013; Kinkel and Maloca Citation2009), reaction to the change in external environment (e.g. Barbieri et al. Citation2019; Moretto, Patrucco, and Harland Citation2020) or a shift in strategy (Di Mauro et al. Citation2018). However, if outsourcing becomes prevailing in an industry, industrial commons (that incorporate technical skills and expertise, technology, supplier base, know-how) may disappear, which makes execution of a reshoring strategy harder (e.g. Pisano and Shih Citation2009). Gurtu, Searcy, and Jaber (Citation2016) also point out that bringing production home requires significant investment until productivity knowledge recovers, which eroded due to decreasing volume or ceasing production. This is the reason why simply abandoning Asian manufacturing powerhouses is difficult: now they own most industrial commons necessary for competitive manufacturing (Longauer, Hauck, and Vasvári Citation2023; Sheffi Citation2020). Srai and Ané (Citation2016) also point out that resources and proximity to markets are more important for reshoring firms than home market mindset driven by government initiatives; accordingly institutional factors in combination with the traditional drivers of relocation decisions should be considered in shaping government policies.
3. Research context: the semiconductor industry
Semiconductors are considered to provide one of key technologies with strategic importance for a broad range of industries (Paier, Dünser, and Unger Citation2017) and are subject to high competition (Chang and Wu Citation2021) and technological complexity (Mönch, Uzsoy, and Fowler Citation2018a). Most semiconductors are integrated circuits and chips, which are responsible for computing, data processing, storage, and transmission. Accordingly, they are vital components of today’s digital world, let it be about consumer electronics, automotive or military. Chips are made from thin wafer layers that may contain up to 100 million transistors per square mm, making the production of semiconductors extremely complex: the 400–1400 steps of manufacturing require high precision in a super tiny scale and outmost sterile conditions (Varas et al. Citation2021). Accordingly, semiconductors are highly complex products in terms of both design and manufacturing: companies spend 20 percent of their total revenue on R&D (Aubry and Renou-Maissant Citation2014), while building a wafer fabrication site (fab) costs USD 10–20 billion and takes several years. Accordingly, capital expenses dominate labour costs in fabrication, thus, low labour costs seemed to be less important in strategic decisions (Brown and Linden Citation2005).
In recent decades, the world economy, particularly manufacturing industries have become globalised that led to a certain division of labour among different regions. Functions requiring high-skilled staff like R&D and product development are generally located in developed countries, while low value-added functions like production or packaging are moved to low-cost regions. The semiconductor industry was no exemption and make-or-buy decisions gave birth to two distinct production models. On the one hand, integrated device manufacturer (IDM) companies generally stick to in-house manufacturing combined with product development, while fabless companies remained focused on R&D and contracted out their production to third-party manufacturers called foundries, primarily in China or Taiwan. Well-known IDM companies are Intel and Samsung, while fabless companies include AMD, Nvidia, or Apple. The market leader foundry is the Taiwanese TSMC that produced more than 13 million 12″ equivalent wafers, i.e. 13 percent of the global wafer capacity in 2021 (Knometa Research Citation2022), providing chips to more than five hundred customers. The advantages of making chips instead of buying are obvious: close relationship with R&D enhances product development and companies are less reliant on abroad suppliers. However, extension of capacities requires enormous capital investments, which combined with demand uncertainties easily leads to oversized capacities (Biwer and Arikan Citation2018; Chang and Wu Citation2021). Fabless companies decouple product development from manufacturing, having foundries to make their products according to their design. Due to the lower capital requirements, going fabless became widespread in the industry (Bown Citation2020; Mönch, Uzsoy, and Fowler Citation2018a). For example, AMD decided to sell its manufacturing arm in the late 2000s; moreover, even IDMs are relying on excess overseas capacities in peak times (Brown and Linden Citation2005). Foundries generally do not have brands or products on their own at all; they focus on pure manufacturing based on the design provided by fabless partners; thus, they spend much less on R&D.Footnote1 Shin, Kraemer, and Dedrick (Citation2016) underlines that business models behave differently in terms of innovation capabilities: while fabless companies may spend more on R&D, IDMs are able to convert their R&D spending into more net profit.
Today, chips are among the most traded products in the world economy, and manufacturing powerhouses in Taiwan, China, and South Korea produce more than 50 percent of semiconductors. Majority of the chips are designed in developed countries like the U.S., Japan, or the EU, whose share in production dropped by 40 percentage points between 1995 and 2020, possessing only 35 percent of world’s wafer producing capacities in 2020 (Table ).Footnote2 Due to the high capital investment intensity, the main driver of moving capacities to Asia was primarily the lower fixed costs achieved via tax advantages (Brown and Linden Citation2005). For example, in case of manufacturing leading-edge technology like advanced logic units, the total cost of ownership (TCO) is estimated to be 22 percent lower in Taiwan and South Korea than in the U.S., while China may offer an additional 15 percent of advantage (Varas et al. Citation2020).
Table 1. The recent geographical distribution of semiconductor industry, percent.
In recent years, chip shortages showed how dependent national economies are on the semiconductor industry: with the current capacities, supply is struggling to fulfil the excess demand, causing significant delays, backlogs, and increased prices in related industries.Footnote3 The reasons are various: due to COVID-19 lockdowns, several industries put their chip orders on hold (e.g. car manufacturing), which resulted in reallocation of supplies to other customers like consumer electronics manufacturers, who were facing increased demand these times. Some argue that the emerging ‘trade war’ between U.S. and China also contributed to the reduction of the available capacities.Footnote4 Moreover, accelerating digital transformation and smart technologies (e.g. IoT) result in an additional need for different semiconductor products (like integrated circuits, microchips). Due to high investment and lead time requirements of building new fabs and capacities, it is expected that shortages of chips may last for years. Until then, OEMs are forced to deal with increasing prices and the lack of semiconductors. Easing demand for chips may be quick wins: recoding software to make chips to do more work or simply offering products without certain features (like USB ports or seat heating in cars). In the long run, additional onshore capacities are supposed to decrease dependency on suppliers in Far-East countries (Ramani, Ghosh, and Sodhi Citation2022; Walsh Citation2022).
The shortages of semiconductors have also been addressed by policymakers, having realised that they are too dependent on the supply of the Asian semiconductor industry. The European Chips Act will aim to strengthen R&D, extend capacity, and develop workforce; by doing so, the EU aims to increase its share in semiconductor production to 20 percent by 2030 (EC Citation2022). In the U.S., the CHIPS and Science Act was signed into law in 2022, which allocates more than USD 50 billion for R&D, labour, and manufacturing development (The White House Citation2022) and also includes an investment tax credit of 25 percent on capital expenses in the industry. Intel and the memory manufacturer Micron already announced to invest into new fabs (for USD 20 and 40 billion, respectively), and TSMC is also building a leading-edge fab for USD 12 billion in Arizona (Reuters Citation2022). Besides, there are several other ongoing government initiatives worldwide that foster semiconductor manufacturing, including China, Taiwan, India, Korea, and Japan, which further highlights the importance of the sector in domestic economies (SIA Citation2022).
4. Model development
4.1. Problem description and motivation
Semiconductor industry is widely exposed to outsourcing and offshoring decisions, though it has several unique features. According to the literature, the main driver of going to or supply from low-cost countries was the high capital requirement to install new capacities, which were largely subsidised in certain developing countries. Consequently, more than 70 percent of wafer capacities are now located in the Far-East; and Taiwan has almost twice as many capacities installed as the U.S. Foundries made the business of fabless companies a lot easier, who might avoid enormous capital expenses and difficulties of capacity planning, and they could focus on high value-added activities like R&D and chip design. Despite this, outsourcing has not become dominant in the industry: several market leader companies still stick to in-house production to harvest synergies from product development and manufacturing, pursuing further technological advantage. However, design and manufacturing prove to be more modular in the case of chip manufacturing (Shin, Kraemer, and Dedrick Citation2016), enabling fabless companies along with foundries to compete with IDMs in innovation; it seems that the learning effect succeeding from extended capacities outweighs the benefits of design-manufacturing synergies, and ‘success-breeds-success’ process makes competitors catch up even harder (Grimes and Du Citation2022). Recent industrial turbulences and chip shortages highlighted that without having own manufacturing arm, companies and entire sectors may be heavily dependent on Asian manufacturing powerhouses, making even national economies vulnerable. Resilience and availability of semiconductors have gained importance over production or fixed cost, giving birth to government incentives aiming to build new onshore capacities and revitalise the semiconductor industry.
In this regard, we introduce a simple model to capture the dynamics and consequences of make-or-buy decisions in the semiconductor industry. We incorporate the trade-off between short-term cost reduction, the productivity gain via learning-by-doing, and the economic impact of resilience, e.g. consequences of chip shortages. In the numerical section, by filling the model with industrial data we further explore the reasons why the current state of global chip-making is different compared to the pre-pandemic era. Even though semiconductor industry is of high impact and demonstrates various production models, according to our knowledge, outsourcing decisions considering learning-by-doing have not been modelled or discussed before in the literature.
4.2. Model formulation
We introduce a dynamic make-or-buy decision model of a manufacturing company that runs a production process which is featured by learning-by-doing effect, i.e. learning happens during production resulting in higher productivity in the long run. We suppose that output per unit of time – denoted by – is fixed,Footnote5 which enables us to concentrate on the effects of learning on the costs of the firm (see e.g. Xiao and Gaimon Citation2013). The firm’s objective is to decide on the proportion of the volume (i.e. what percentage of
) to be produced in-house, which we denote by the decision variable
at time
(in short, in-house production ratio). If
, then the firm outsources production to a supplier for a contract-specific unit price denoted by
(case of ‘buy’). Based on Dick (Citation1991), we also suggest that this purchasing price is set by considering the learning effect arising at foundries. As semiconductors have high value compared to their weight, we assume that shipment cost is relatively low and included in unit price
. To capture resilience, we introduce the
parameter, representing economic loss due to shortage or delayed shipment of goods. Accordingly, total cost of ‘buy’ at time
reaches
. In line with the common assumptions in literature (see, e.g. Gilbert, Xia, and Yu Citation2006; Xu et al. Citation2013) we suppose that there is no quality difference between the output produced in-house and by supplier, and both companies have sufficient capacities and inputs to produce
quantity.
If , then the firm produces the entire amount in-house and the resulting costs depend on its production history (case of ‘make’). Production costs decrease as cumulative in-house output increases, that is, productivity depends on the production experience the firm has gained in the past (see, e.g. Dick Citation1991; Vörös Citation2021). The strength of this learning effect is of course dependent on the added value and complexity of the given product and underlying production process. In the semiconductor industry the effect is estimated to be around 20 percent, meaning that each doubling of the cumulative output results in a 20 percent decrease of unit production cost (Irwin and Klenow Citation1994).Footnote6 The decrease in unit cost is the result of rising output yields as the firm continually refines its production process and performs the manufacturing steps more efficiently resulting in fewer defective items (Dick Citation1991). In the model, we denote the cumulative in-house output by
and introduce the following equation of motion describing its evolution based on Vörös (Citation2021):
(1)
(1) Let us model the production unit cost function by
, which has the following properties (Dick Citation1991):
(2)
(2) Assumption (i) states that unit production cost is
if the firm does not have any production experience, assumption (ii) sets a lower bound on the unit production cost, while according to assumption (iii) unit production cost declines with cumulative output at a decreasing rate. As fixed costs may be relevant in certain industries (especially in the semiconductor industry), we also introduce the fixed cost of production as per unit of time – denoted by
– and suppose that it is proportional to the ratio of in-house production chosen by the firm. The total cost of ‘make’ at time
is thus
We apply an additional concept rarely used in the literature, namely the future value of production experience to capture its role in the design and development of future products and processes (Vörös Citation2013; Xiao and Gaimon Citation2013). As in-house production increases productivity through learning-by-doing, the firm may be able to develop and manufacture new product generations more effectively. We denote the salvage value of this additional productivity by , which aims to capture the future value of producing one unit of output in-house.
In some cases, the firm may be better off with partial outsourcing () if it is feasible and most favourable from costs’ perspective (case of ‘make-and-buy’). Finally, let the length of the decision interval be
which denotes the product’s life cycle, during which discount rate
is constant (
). Notations used in the model are listed in Table .
Table 2. List of notations.
Despite the fact that the motivation comes from the semiconductor industry, the theoretical model is generally applicable for other industries or products with the same settings, i.e. (1) there is excess demand for the product or the output is given and constant; (2) the value of products is high compared to weight, making shipment cost relatively low; (3) quality is consistent and standard, no matter if production is carried out in-house or by a supplier; and (4) design and manufacturing functions are modular, i.e. locating manufacturing close to product development is not a must. According to Pisano and Shih (Citation2012), beyond semiconductor industry, consumer electronics and active pharmaceutical products are good examples to meet these criteria. Moreover, the numerical section also demonstrates that filling the model with industry-specific data may give floor to further analysis.
5. The dynamic optimisation problem
Using the previously introduced notations and assumptions, we can finally formulate the dynamic cost minimisation problem given in (3). Aim of the firm is to choose an optimal path that minimises its make-or-buy costs. The firm produces some
proportion itself with a unit cost of
and the rest (
) is done by the supplier who sells the items on contract price
. The firm also faces
fixed costs in case of in-house production, and
unit economic loss in case of outsourcing. At the end of the product life cycle, the firm may benefit from having increased its productivity knowledge expressed by term
, because development of next product generations is more cost-effective or because the firm sells business at a whole. The firm decides on the in-house production ratio, i.e.
is decision variable, while
level of cumulative output is state variable. The firm commits to its in-house production ratio path at
, that is at the beginning of the product life cycle.
(3)
(3)
We solve the problem by applying the methodology of optimal control. The current-value Hamiltonian equivalent to problem (3) is the following:
(4)
(4) where
is the dynamic Lagrange multiplier, which is function of time, like
and
, but for the sake of simplicity we omitted the time index. Lagrange multiplier
shows how much cost the firm can save from a marginal increase in the level of cumulative output thanks to learning-by-doing at current value. The optimality conditions required to solve the optimisation problem are the following (Chiang Citation2000):
(4a)
(4a)
(4b)
(4b)
(4c)
(4c)
(4d)
(4d) where an asterisk indicates optimal value. Condition (4a) states that the production ratio must be chosen so that the Hamiltonian is maximised (Pontryagin’s maximum principle). Condition (4b) describes the evolution of the state variable, while condition (4c) describes the evolution of the Lagrange multiplier. Finally, condition (4d) introduces the transversality condition to predetermine the terminal value of the Lagrange multiplier. As the planning period ends in
, the firm benefits from any increase of cumulative output as much as the
salvage value.
From condition (4c) an expression for can be derived when we multiply both sides of the differential equation with
and then calculate the definite integral of the resulting expression with respect to time:
where
is the constant of integration. From (4d) it follows that
, and we get:
(5)
(5) where we reintroduced time index. The dynamic Lagrange multiplier is defined as the sum of two factors: the first term is the salvage value of an additional unit of output produced in-house, while the second term is the impact of productivity knowledge on total costs over the remainder of the planning period, both discounted to period
. The sign of the Lagrange multiplier is influenced by the
derivative function. According to our assumptions in (2)
, the Lagrange multiplier can never be negative. Substituting (5) into (4c), for the dynamics of the Lagrange multiplier we get:
(6)
(6) In the absence of discounting or if the discount rate is very low (i.e.
) the Lagrange multiplier cannot increase in time, because
, meaning that the cost–benefit resulting from the learning effects diminishes as time goes by.
According to condition (4a), the firm chooses an in-house production ratio that maximises the Hamiltonian. Let us rewrite condition (4a):
(4a*)
(4a*) Clearly, the Hamiltonian is maximised by
if expression
is positive on the whole
interval, and it is minimised by
if the expression is negative. Therefore, we can summarise the optimal decision as follows:
(7)
(7) According to (7), given the optimal value of
and
, the relation between the long run cost-benefits of learning-by-doing
and the difference in unit costs between the two strategies, (i.e. make and buy,
) determines whether it is more reasonable to outsource (
) or to produce in-house (
) at time
. As the
function is nonnegative, learning can decrease the future production costs of the firm. If these learning benefits are strong enough, it may be rational for the firm to choose in-house production even if outsourcing was more cost-efficient in the short run, i.e.
holds. In (7), the equality case corresponds to an internal solution, when partial outsourcing may arise, but this is an instable case. As the system evolves continuously, even if this case occurs, it is only temporary and never permanent. To show this, let us analyse the dynamic properties of the problem. The evolution of the relation in (7) determines the dynamics of the firm’s make-or-buy decision. Since the term
is constant, the dynamics of the model depends on the evolution of term
:
(8)
(8) where we made use of differential Equations (1) and (6). Since (8) is nonnegative, once the firm decided to choose in-house production, it will stick to it and not switch to outsourcing. On the other hand, if the firm has initially decided to choose outsourcing, it is possible that the firm switches to in-house production, as it may become less advantageous relative to outsourcing over time. This latter case would mean a dynamic strategy in the sense that the firm changes its make-or-buy choice from ‘buy’ to ‘make’ at some point in time. The motivation of the firm to choose the ‘make’ strategy after a while is that this way it may benefit from having increased productivity in the design and development of the next product generations, which we capture by the salvage value of in-house output, i.e.
.
6. Numerical simulations
In this section, we examine the model by means of numerical simulations using data taken from the semiconductor industry (see Table ). To model the effect of learning on production costs we suppose a traditional power function that satisfies the properties given in (2) and is widely used in the literature (e.g. Anderson and Parker Citation2002; Vörös Citation2021; Xiao and Gaimon Citation2013):
(9)
(9) where
is the unit production cost without any prior production history (i.e.
), and
determines the learning rate – the rate at which costs fall with each doubling of the cumulative in-house output – based on expression
(Irwin and Klenow Citation1994). A cost function like (9) demonstrates that learning is most important in the early stages of production (Jarmin Citation1994), as costs decrease faster at the beginning and then diminish as cumulative output increases and technology gets mature.
Table 3. List of parameter values.
Our baseline scenario is the pre-pandemic state of the industry, which is primarily based on data from 2014. Selecting this year is mainly due to data availability issues of firm level capacities. As accessing semiconductor market data is particularly difficult (Aubry and Renou-Maissant Citation2014; Shin, Kraemer, and Dedrick Citation2016), in some cases, we had to rely on business reports, journals, or other sources of information. Our reference value is the purchasing cost per wafer, calculated from foundry sales data and capacities. Production cost is estimated from an IDM’s cost of goods sold per wafer.Footnote7 Total fixed cost is coming from the capital investment requirement of 16/12 nm node technology estimated by Khan and Mann (Citation2020, 44), launched by TSMC in 2014. The estimation of productivity knowledge salvage value is based on the recent acquisition of Tower Semiconductor by Intel.Footnote8 We initially considered a 10-year length product life cycle,Footnote9 and both fixed cost per year and salvage value were calculated by using this timeframe. Initial production history, and discount rate is counted as zero, while learning rate is set to 20 percent, based on Irwin and Klenow (Citation1994). For the sake of simplicity, the annual capacity is considered to be one in both cases (i.e. ) and fixed costs are normalised to this reference capacity.
To capture the dynamics of the industry, we constructed a post-pandemic scenario, where we amended several parameter values. The gap between production costs relative to purchasing price has narrowed according to several reports (e.g. Scheper Citation2022; Varas et al. Citation2020). We also accounted for the shorter life cycles by reducing it to seven years. We amended total fixed cost based on the total value and capacity of the TSMC’ 5 nm node factory under construction in Arizona (Reuters Citation2022). We consider the economic impact of semiconductor non-availability to be zero in the first scenario, meaning no shortages or delays in lead time experienced or significantly affected sales. In the post-pandemic era, however, lack of semiconductors caused disruptions in dependent industries, e.g. Rowsell (Citation2022) estimated that chip shortages cost the U.S. economy USD 240 billion. Projecting this data on the global wafer capacity installed in 2021, the impact of shortage is an additional 0.5478 cost per wafer, relative to purchasing price in our model.Footnote10 Due to the increase in interest rates after the pandemic, we amended the discount rate to 4 percent.Footnote11
Figure plots make-or-buy strategies estimated by numerical simulations. Graphs on the left-hand side describe the pre-pandemic era, while the ones on the right show current conditions. On each graph, a dot marks the optimal strategy for the industry in the given year. The aim of presenting series of graphs was to illustrate the role of different parameter values on the optimal solutions and to compare the situation before and after the pandemic. In general, we can conclude that outsourcing was the dominating optimal strategy before the pandemic, though currently in-house production seems to be the best practice. Main reasons include the significant increase in economic impact of shortages and the decrease of unit production cost relative to purchasing cost. Before going into details, let us add that the currently dominating optimal ‘make’ strategies are typically close to the threshold to choosing the ‘buy’ option.
Figure 1. Optimal ‘make-or-buy’ strategies in the semiconductor industry.
Graphs A1 and A2 show that chances of in-house production are growing with decreasing fixed and production costs. The borderline between ‘make’ and ‘buy’ strategies was roughly in the middle of the graph. Though, the current conditions shifted the edge between strategies upwards, making in-house production the optimal strategy. Let us note that the current combination of production and fixed cost would have resulted in outsourcing in the pre-pandemic scenario, as it can be seen marked with a square.
The role of unit production cost and the economic impact of shortages are illustrated in Graphs B1 and B2. In the baseline scenario, when production costs were relatively low and the industry did not face supply chain disruptions, choosing outsourcing was a straightforward decision. Our model suggests, however, that today it is more obvious to produce in-house. We gain similar results when considering the impact of shortages in terms of product life cycle (Graphs C1 and C2).
It has already been shown in the first pair of graphs that higher fixed costs would not influence the ‘make’ strategy in the current scenario. Similarly, Graphs D1 and D2 present that in fact, salvage value of productivity knowledge also does not affect the optimal strategy. Graphs E1 and E2 illustrate how initial production history determines outsourcing decisions in terms of product life cycle. We found that previous manufacturing experience would have been important only if the product life cycle went beyond 12 years, otherwise, buying was a straightforward strategy. In the post-pandemic era, however, in-house production is advised, even for fabless companies that lack production history. Considering the slope of the edge between strategies, previous manufacturing experience plays much smaller role in the current scenario.
The relationship between learning rate and production cost is plotted by Graphs F1 and F2. In the pre-pandemic scenario, making would be beneficial only if purchasing price was similar to the cost of in-house manufacturing. In the current scenario, market conditions made outsourcing less favourable. However, without the drop of relative production costs, the optimal strategy would be ‘buying’, very close to the edge. Finally, Graphs G1 and G2 show that discount rates have slight but discouraging effect on ‘make’ strategy, and considering the current state of the industry, ‘make’ may pay off, even in the recent era of increasing rates.
7. Discussion
Our general model showed two straightforward consequences on how learning-by-doing can affect make-or-by decisions. First, even if outsourcing is cost-efficient in the short run, in the long run it may be rational to choose in-house production, as production costs drop by time, and accumulated productivity knowledge may have market value at the end of the planning period. Second, considering the learning effect might lead to the revision of previous outsourcing decisions. These are important aspects of the semiconductor industry as numerous industries are suffering nowadays from the disruption of supply chains, growing chip shortages, and from the dependence on Asian manufacturing powerhouses; from this aspect, going fabless and outsourcing production to the Far-East seems to be a myopic strategy. According to our numerical simulations, however, outsourcing was an obvious decision in the pre-pandemic era, even if we consider the effect of learning-by-doing. Although the cost advantage of Asian foundries was mainly due to government incentives, according to our simulations, subsidising manufacturing via incentives on capex or labour could hardly lead to keep manufacturing onshore: Graph A1 shows that for any value of fixed costs, or even if the gap between production costs and purchasing price was reduced to a third, the optimal strategy remained outsourcing. The same applies to those firms who had production history (like AMD) or who had even considered the salvage value of productivity knowledge accumulated via manufacturing.
No doubt, being fabless was highly beneficial for firms as they were not forced to invest into expensive wafer fabrication sites, moreover, they were shielded from demand uncertainties. In addition, as design and production activities are modular, decoupling of manufacturing from R&D did not result in disadvantage in terms of the applied technology. Meanwhile, foundries were able to build significant capacities, which may have helped them to decrease production cost via learning-by-doing. On the one hand, this was beneficial for both fabless and foundry companies, in line with the theoretical model developed by Deng, Guan, and Xu (Citation2021). On the other hand, ‘success-breeds-success’ process favouring incumbent manufacturers made entering the manufacturing market extremely difficult.
Despite all the advantages, offshoring to overseas locations comes with some risks either related to transportation, quality, protection of intellectual rights, or supply issues. The semiconductor industry faced the realisation of these, and the arising shortages of chips showed how easily these turbulences can spill over to other industries. Capacities take years to be built up, so firms are forced to adapt to lack of chips. However, it seems that companies possessing own manufacturing capacities are better off, and they may be less affected by delays or increased lead times. Accordingly, we included a proxy for economic impact of shortages into our model, making the simulations able to capture the current state of the industry. The optimal strategy seems to be in-house manufacturing, which is in line with the ongoing trends related to revitalisation of onshore chip making. Our model, however, provides further implications. First, as Graph A2 illustrates, the optimal strategy is also exposed to production cost. This is in line with the findings of Varas et al. (Citation2020), who found that labour costs have now higher importance in selecting fab location, besides capital expenditures and government incentives. Interestingly, Brown and Linden (Citation2005) did not assign much importance on labour cost, which highlights how economic environment has changed in the recent decade: considering the current relative production costs in the pre-pandemic scenario, the optimal strategy would be still outsourcing, suggesting labour cost had less importance in the past. Second, the negative economic impact of shortages may project further price increases,Footnote12 which can result in a reduction of the relative cost of production (further strengthening the strategy of in-house production). However, should the extent of chip shortages ease, purchasing price relative to production costs would not increase further, and this may push the firm towards outsourcing (Graph B2). The same applies if product life cycle shortens (Graph C2): below six years, ‘buying’ is the optimal strategy, assuming the current economic impact of shortages. Accordingly, outsourcing may become again the optimal strategy in time. Third, salvage value of productivity knowledge remained less important in make-or-buy decisions (Graph D2). Fourth, our model suggests that as long as the product life cycle is above six years, even fabless companies may consider establishing a manufacturing arm. However, starting or restoring manufacturing activities from zero requires large investment to establish proper capital and labour foundations of competitive production (Gurtu, Searcy, and Jaber Citation2016).
The presented research provides interesting insights into the semiconductor industry, even though, it has certain limitations, mainly stemming from the nature of the model. Due to the current shortages and backlogs of semiconductors, including potential demand-side effects is not vital. However, the capacity constraint means some restrictions, as it assumes that there is a persistent excess demand for semiconductor products. Otherwise, the company would be able to decide on the level of output rather than operating at full capacity, which would provide some control over the learning effect and indirectly the costs through the production volume. We also assumed that the company knows the exact length of the product life cycle in advance and optimise accordingly (e.g. technology roadmaps), however, this assumption may also be too strict in the recent business environment.
8. Conclusion
Since the COVID-19 pandemic, semiconductor industry has been the bottleneck for numerous dependent industries, and shortages of chips resulted in operating of conveyor belts at a lower speed. The reasons are obvious: excess demand can be fulfilled by installing new capacities, which may take several years and costs billions of dollars. In this study, we modelled the make-or-buy decision problem of a firm considering the learning-by-doing effect arising from production. We constructed numerical simulations to capture the pre- and post-pandemic state of the industry and show how chip-making has dynamically changed over time.
The theoretical model has two simple takeaways. First, should outsourcing be cost-efficient in the short run, it still may be rational to choose in-house production in the long run. Second, considering learning effect and resilience may lead to the revision of previous outsourcing decisions and even to insourcing production.
Excess dependence on Asian manufacturing powerhouses like Taiwan is mainly due to the emergence of the fabless-foundry model in the 2000s, where government subsidies for fab construction and manufacturing enabled production at lower costs, which in turn attracted orders from overseas. At the same time, fabless companies were able to manage demand uncertainties and get rid of the capital requirements. Considering recent economic turmoil in supplying chips, these outsourcing decisions might seem extremely myopic. However, our simulation shows that being fabless was a well-founded decision and even government incentives could not reverse this process.
The recent economic impact of chip shortages has been shocking, resulting in the growing need of onshoring semiconductor production. Government initiatives were launched or even signed into law in the developed world to revitalise manufacturing of semiconductors. Our model shows that in-house production may be beneficial in the current state of the industry, even without government incentives (see Srai and Ané Citation2016). However, we add that the current state of the industry is fragile, and the optimal strategy is close to the edge of ‘buying’. For example, decreasing the extent of shortages may push companies again to choose outsourcing. This may happen sooner than we think; in the second half of 2022, there were already signs of a decline in demand for final products, like PCs or smartphones (de Laubier Citation2022) and growing inventories (Culpan Citation2022; Dobberstein Citation2022) which also indicates a falling need for semiconductors. Returning to the status quo may be against industrial and political interest, which may be considered in shaping government policies on the semiconductor industry.
We also conclude that even fabless companies without any production history may be advised to start manufacturing gradually. Extension of capacities will also be crucial for companies with in-house production in reduction of production cost via learning effect.Footnote13 However, execution may be not as easy as theory suggest. Decreasing extent of manufacturing results in diminishing of industrial commons, which makes building new capacities harder. For example, it is reported that TSMC is facing difficulties in establishing a proper supply chain for their new fab in Arizona (Patterson Citation2022).
Even though we aimed to provide a full picture of the semiconductor industry, there are several options for further research. First, we assumed that the firm produces at full capacity and aims to minimise costs, which is true in the current situation of excess demand towards semiconductors. Including the demand curve in the model, however, may provide a better understanding on business decisions in those times when companies are not facing serious shortages and backlogs; this would also give the opportunity to take the dynamics of product price and product quality into account (Vörös Citation2019). Second, the model and the simulations considered the same learning rate within the industry. As learning effect may vary across countries and firms (Dick Citation1991; Jaber Citation2006), and may also be improved e.g. by learning by experimentation (Manda and Uzsoy Citation2021; Terwiesch and Bohn Citation2001) or by cross-stage learning (Longauer, Hauck, and Vasvári Citation2023), it would be interesting to investigate what are the model implications of the different channels of learning. We assumed that a firm’s productivity knowledge could only increase, but it can also decline, e.g. due to the forgetting effect caused by high turnover of employees (Gurtu, Searcy, and Jaber Citation2016). Furthermore, during periods of outsourcing, the company’s production knowledge may completely disappear, that is, including learning and forgetting effects jointly in the model like in Anderson and Parker (Citation2002) would enrich the scope.
Our investigations have shown that several aspects must be considered when deciding on investments and capacity planning, i.e. to outsource or produce in-house. These aspects and conditions might differ from industry to industry. Based on data of the semiconductor industry, we have seen that changes in the conditions can have a significant impact on the optimal strategy as the ‘buy’ strategy was dominating in the pre-pandemic era, whereas the current situation suggests producing in-house. As the optimal strategy is typically close to the threshold to outsourcing, the current optimum can be subject to change even in a shorter period. Thus, we can conclude that winners will be the ones adapting to changes quickly. Resilience in this sense can either mean flexibility of production strategies, i.e. a firm can switch from the pre-pandemic ‘buy’ strategy to ‘make’ in the current situation and change back again if necessary. The other interpretation of resilience can be accepting the current suboptimal situation until outsourcing will be optimal again. As establishing a factory means high amount of sunk costs when turning from ‘make’ to ‘buy’, salvage value of productivity knowledge will play a more important role than considered before.
Disclosure statement
No potential conflict of interest was reported by the author(s).
Data availability statement
Data sharing is not applicable to this article as no new data were created or analysed in this study.
Additional information
Funding
Notes on contributors
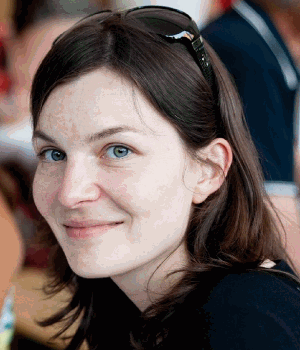
Dóra Longauer
Dóra Longauer is an Assistant Professor of Operations Management at the Management Science Department of the Faculty of Business and Economics, University of Pécs, Hungary. She holds a doctorate degree in Economics from the same university. Her research interests include economic applications of network theory and supply chain related decisions of firms.
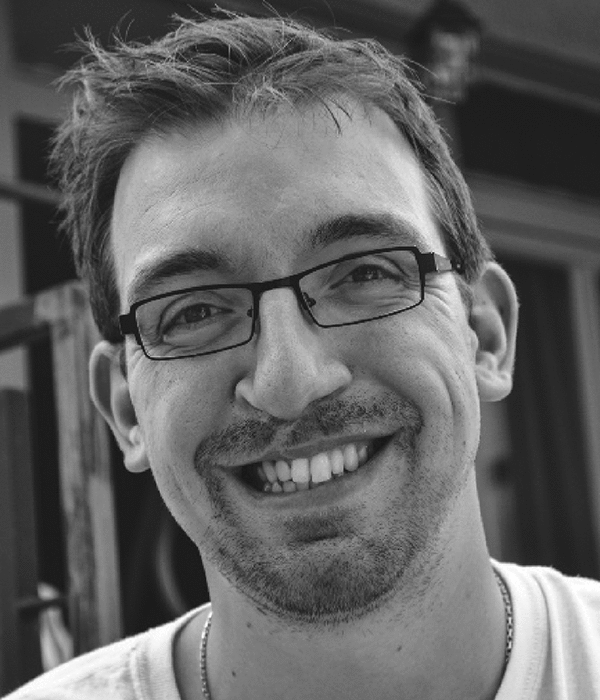
Tamás Vasvári
Tamás Vasvári is a Research Fellow at the Faculty of Business and Economics, University of Pécs, Hungary. He holds a doctorate degree in Economics from the same university. His research interests cover value chain upgrading, the role of multinational companies in the CEE region, and economic development through industrialisation.
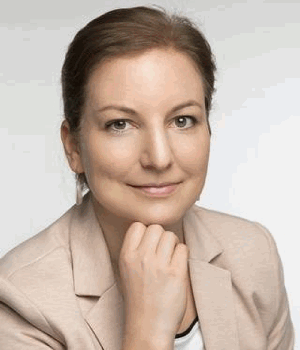
Zsuzsanna Hauck
Zsuzsanna Hauck is an Associate Professor of Operations Management at the Management Science Department of the Faculty of Business and Economics, University of Pécs, Hungary. She holds a doctorate degree and habilitation in Business Administration from the same university. Her research interests include OM-related topics like inventory modelling, quality control, and optimal control.
Notes
1 For example, TSMC’s R&D expenditures compared to total sales were roughly the half of Intel’s or AMD’s spending (7.9 percent compared to 19.2 and 17.3 percent, respectively) in 2021.
2 Accordingly, the value chain has become geographically fractured: the software for design and IP cores are primarily coming from the U.S., manufacturing equipment is delivered from Europe to East Asia, where most of the wafer fabrication is made. Packaging and assembly are mostly done in China, then products are delivered to end users.
3 The average lead time of semiconductor products was below 10–15 weeks before 2021 and it rose to more than 25 weeks by the early 2022 (Peng Citation2022).
4 Due to issues regarding protection of intellectual property in China, the U.S. imposed a 25 percent tariffs on semiconductor imports in 2018 (Bown Citation2020), while U.S. firms were limited to sell semiconductors to Chinese firms like Huawei or SMIC from 2019 (Thorbecke Citation2021). Obviously, these steps reduced the available capacities for companies in both countries.
5 This is reasonable due to recent excess demand for semiconductors.
6 The results of Irwin and Klenow (Citation1994) are still point of reference in the literature, e.g. recently applied by Asmat (Citation2021) in his simulations. Further industry-specific examples are discussed by Jaber (Citation2006).
7 For foundry and IDM data, we considered TSMC and Intel annual reports, respectively. Capacities are coming from IC Insight (Citation2016).
8 Agreed price (without premium) per annual wafer capacity of Tower Semiconductor (Gallagher Citation2022). The pre-pandemic parameter value is deflated by CPI.
9 After eight years, 16/12 nm technology still represents 13 percent of total revenue at TSMC. It is also reported that several semiconductor manufacturers revised and disclosed positive changes regarding the useful life of their machinery (Usvyatsky and Pakaluk Citation2016).
10 We are aware that this is a rough calculation (e.g. the projection could be more precise if only the U.S. consumption of semiconductors were considered). However, the real economic impact may be still under-estimated: e.g. AlixPartners (Citation2021), estimated that the economic impact of chip shortages is USD 210 billion for the automotive industry alone.
11 As of 31 March 2023, the effective federal funds rate in the US is 4.75–5.00 percent, the interest rate in the euro area is 3.50 percent, while it is 4.25 percent in the UK. (https://tradingeconomics.com/country-list/interest-rate).
12 TSMC raised prices by 20 percent in 2021, which was followed by an additional increase of 5–8 percent in 2022 (Sevilla Citation2022; Ting-Fang and Li Citation2022).
13 The acquisition of Tower Semiconductor by Intel is good example how an IDM may expand its capacities and penetrate into the foundry market.
References
- AlixPartners. 2021. “Shortages Related to Semiconductors to Cost the Auto Industry $210 Billion in Revenues This Year”, Says New Alix Partners Forecast. Press release, September 12, 2021. https://www.alixpartners.com/media-center/press-releases/press-release-shortages-related-to-semiconductors-to-cost-the-auto-industry-210-billion-in-revenues-this-year-says-new-alixpartners-forecast/.
- Anderson, E. G., and G. G. Parker. 2002. “The Effect of Learning on the Make/Buy Decision.” Production and Operations Management 11 (3): 313–339.
- Appleyard, M. M. 1996. “How Does Knowledge Flow? Interfirm Patterns in the Semiconductor Industry.” Strategic Management Journal 17 (S2): 137–154. https://doi.org/10.1002/smj.4250171112.
- Asmat, D. 2021. “Collusion Along the Learning Curve: Theory and Evidence from the Semiconductor Industry.” The Journal of Industrial Economics 69 (1): 83–108. https://doi.org/10.1111/joie.12235.
- Aubry, M., and P. Renou-Maissant. 2014. “Semiconductor Industry Cycles: Explanatory Factors and Forecasting.” Economic Modelling 39: 221–231. https://doi.org/10.1016/j.econmod.2014.02.039.
- Barbieri, P., E. Stefano, L. Fratocchi, and R. Golini. 2019. “Relocation of Second Degree: Moving Towards a New Place or Returning Home?” Journal of Purchasing and Supply Management 25 (3): 100525. https://doi.org/10.1016/j.pursup.2018.12.003.
- Biwer, S., and E. Arikan. 2018. “Capacity Planning Challenges in a Global Production Network with an Example from the Semiconductor Industry.” M. Rabe, A.A. Juan, N. Mustafee, A. Skoogh, S. Jain, and B. Johansson (eds.). Proceedings of the 2018 Winter Simulation Conference. 3386–3396.
- Bown, Ch.P. 2020. “How the United States Marched the Semiconductor Industry Into its Trade War with China.” East Asian Economic Review 24 (4): 349–388. https://doi.org/10.11644/KIEP.EAER.2020.24.4.384.
- Brown, C., and G. Linden. 2005. “Offshoring in the Semiconductor Industry: A Historical Perspective.” Brookings Trade Forum, Offshoring White-Collar Work (2005). 279–333. https://www.jstor.org/stable/25058769#metadata_info_tab_contents.
- Chang, B.-G., and K.-S. Wu. 2021. “The Nonlinear Relationship Between Financial Flexibility and Enterprise Risk-taking During the COVID-19 Pandemic in Taiwan’s Semiconductor Industry.” Oeconomia Copernicana 12 (2): 307–333. https://doi.org/10.24136/oc.2021.011.
- Chiang, A. C. 2000. Elements of Dynamic Optimization. Illinois: Waveland Press Inc.
- Chiang, D., R.-S. Guo, A. Chen, M.-T. Cheng, and Ch-B. Chen. 2007. “Optimal Supply Chain Configurations in Semiconductor Manufacturing.” International Journal of Production Research 45 (3): 631–651. https://doi.org/10.1080/00207540600792499.
- Chien, Ch-F, and R.-T. Kuo. 2013. “Beyond Make-or-Buy: Cross-company Short-Term Capacity Backup in Semiconductor Industry Ecosystem.” Flexible Services and Manufacturing Journal 25 (3): 310–342. https://doi.org/10.1007/s10696-011-9113-4.
- Culpan, T. 2022. “A New Normal is Dividing the Global Chip Industry.” The Economic Times, August 17, 2022. https://economictimes.indiatimes.com/small-biz/trade/exports/insights/a-new-normal-is-dividing-the-global-chip-industry/articleshow/93606836.cms?from=mdr.
- de Laubier, Ch. 2022. “Why the Global Chip Market is Seizing Up Despite Massive Investments.” Le Monde, September 28, 2022. https://www.lemonde.fr/en/economy/article/2022/09/28/why-the-global-chip-market-is-seizing-up-despite-massive-investments_5998492_19.html.
- Deng, S., X. Guan, and J. Xu. 2021. “The Coopetition Effect of Learning-by-Doing in Outsourcing.” International Journal of Production Research 59 (2): 516–541. https://doi.org/10.1080/00207543.2019.1696493.
- Dick, A. R. 1991. “Learning by Doing and Dumping in the Semiconductor Industry.” Journal of Law and Economics 34 (4): 131–159.
- Di Mauro, C., L. Fratocchi, G. Orzes, and M. Sartor. 2018. “Offshoring and Backshoring: A Multiple Case Study Analysis.” Journal of Purchasing and Supply Management 24 (2): 108–134. https://doi.org/10.1016/j.pursup.2017.07.003.
- Dobberstein, L. 2022. “TSMC Reports Record Profits as Customers Hoard Chips.” The Register, July 15, 2022. https://www.theregister.com/2022/07/15/tsmc_record_profits/.
- EC. 2021. 2021 State of the Union Address by President von der Leyen. Strasbourg, September 15, 2021. https://ec.europa.eu/commission/presscorner/detail/ov/SPEECH_21_4701.
- EC. 2022. “European Chips Act.” https://ec.europa.eu/info/strategy/priorities-2019-2024/europe-fit-digital-age/european-chips-act_en.
- Fitch, A. 2020. “Intel’s Success Came With Making Its Own Chips. Until Now.” Wall Street Journal, Nov 6 2020. https://www.wsj.com/articles/intel-chips-cpu-factory-outsourcing-semiconductor-manufacturing-11604605618.
- Funk, J. L., and J. Luo. 2015. “Open Standards, Vertical Disintegration and Entrepreneurial Opportunities: How Vertically-Specialized Firms Entered the U.S. Semiconductor Industry.” Technovation 45–46: 52–62. https://doi.org/10.1016/j.technovation.2015.07.001.
- Gallagher, D. 2022. Intel Pays Up for Foundry Expertise. The Wall Street Journal, February 15, 2022. https://www.wsj.com/articles/intel-pays-up-for-foundry-expertise-11644954114?mod=article_inline.
- Gilbert, S., Y. Xia, and G. Yu. 2006. “Strategic Outsourcing for Competing OEMs That Face Cost Reduction Opportunities.” IIE Transactions 38 (11): 903–915. https://doi.org/10.1080/07408170600854644.
- Gray, J. V., K. Skowronski, G. Esenduran, and M. J. Rungtusanatham. 2013. “The Reshoring Phenomenon: What Supply Chain Academics Ought to Know and Should do.” Journal of Purchasing and Supply Management 49 (2): 27–33.
- Gray, J. V., B. Tomlin, and A. V. Roth. 2009. “Outsourcing to a Powerful Contract Manufacturer: The Effect of Learning-by-Doing.” Production and Operations Management 18 (5): 487–505. https://doi.org/10.1111/j.1937-5956.2009.01024.x.
- Grimes, S., and D. Du. 2022. “China’s Emerging Role in the Global Semiconductor Value Chain.” Telecommunications Policy 46 (2). https://doi.org/10.1016/j.telpol.2020.101959.
- Gurtu, A., C. Searcy, and M. Y. Jaber. 2016. “Effects of Offshore Outsourcing on a Nation.” Sustainable Production and Consumption 7: 94–105. https://doi.org/10.1016/j.spc.2016.06.001.
- Hatch, N. W., and D. C. Mowery. 1998. “Process Innovation and Learning by Doing in Semiconductor Manufacturing.” Management Science 44 (11-part-1): 1461–1477. https://doi.org/10.1287/mnsc.44.11.1461.
- IC Insight. 2016. “Samsung, TSMC Remain Tops in Available Wafer Fab Capacity.” Research Bulletin, January 6, 2016. https://www.icinsights.com/news/bulletins/Samsung-TSMC-Remain-Tops-In-Available-Wafer-Fab-Capacity/.
- IC Insight. 2021. “Taiwan Maintains Edge as Largest Base for IC Wafer Capacity.” Research Bulletin. July 13, 2021. https://www.icinsights.com/news/bulletins/Taiwan-Maintains-Edge-As-Largest-Base-For-IC-Wafer-Capacity/.
- Irwin, D. A., and P. J. Klenow. 1994. “Learning-by-Doing Spillovers in the Semiconductor Industry.” Journal of Political Economy 102: 1200–1227.
- Jaber, M. Y. 2006. “Learning and Forgetting Models and Their Applications.” In Handbook of Industrial and Systems Engineering, edited by A. B. Badiru. Boca Raton: Taylor and Francis, 708.
- Jarmin, R. 1994. “Learning by Doing and Competition in the Early Rayon Industry.” The RAND Journal of Economics 25: 441–454. https://doi.org/10.2307/2555771.
- Kamalahmadi, M., M. Shekarian, and M. M. Parast. 2022. “The Impact of Flexibility and Redundancy on Improving Supply Chain Resilience to Disruptions.” International Journal of Production Research 60 (6): 1992–2020. https://doi.org/10.1080/00207543.2021.1883759.
- Khan, M. S., and A. Mann. 2020. “AI Chips: What They Are and Why They Matter.” Center for Security and Emerging Technology, April 2020. 72p. https://cset.georgetown.edu/publication/ai-chips-what-they-are-and-why-they-matter/.
- Kinkel, S., and S. Maloca. 2009. “Drivers and Antecedents of Manufacturing Offshoring and Backshoring – A German Perspective.” Journal of Purchasing & Supply Management 15 (3): 154–165. https://doi.org/10.1016/j.pursup.2009.05.007.
- Knometa Research. 2022. “Top Five Leaders Continue Expanding Share of Global IC Fab Capacity.” https://www.knometa.com/news/?post=top-five-leaders-continue-expanding-share-of-global-ic-fab-capacity&tag=global-wafer-capacity.
- Liao, Sh-H, and T-Ch. Hu. 2007. “Knowledge Transfer and Competitive Advantage on Environmental Uncertainty: An Empirical Study of the Taiwan Semiconductor Industry.” Technovation 27 (6–7): 402–411. https://doi.org/10.1016/j.technovation.2007.02.005.
- Longauer, D., Zs Hauck, and T. Vasvári. 2023. “Make-or-Buy Strategies in a Multi-Stage Manufacturing Process and the Role of Learning Effect in Relocation Decisions.” Computers & Industrial Engineering, 109259. https://doi.org/10.1016/j.cie.2023.109259.
- Manda, A. B., and R. Uzsoy. 2021. “Managing Product Transitions with Learning and Congestion Effects.” International Journal of Production Economics 239: 108190. https://doi.org/10.1016/j.ijpe.2021.108190.
- Mathews, J. A. 1997. “A Silicon Valley of the East: Creating Taiwan’s Semiconductor Industry.” California Management Review 39 (4): 25–55. https://doi.org/10.2307/41165909.
- Mazzola, E., M. Bruccoleri, and G. Perrone. 2019. “The Curvilinear Effect of Manufacturing Outsourcing and Captive-Offshoring on Firms’ Innovation: The Role of Temporal Endurance.” International Journal of Production Economics 211: 197–210. https://doi.org/10.1016/j.ijpe.2019.02.010.
- Mönch, L., R. Uzsoy, and J. W. Fowler. 2018a. “A Survey of Semiconductor Supply Chain Models Part I: Semiconductor Supply Chains, Strategic Network Design, and Supply Chain Simulation.” International Journal of Production Research 56 (13): 4524–4545. https://doi.org/10.1080/00207543.2017.1401233.
- Mönch, L., R. Uzsoy, and J. W. Fowler. 2018b. “A Survey of Semiconductor Supply Chain Models Part III: Master Planning, Production Planning, and Demand Fulfilment.” International Journal of Production Research 56 (13): 4565–4584. https://doi.org/10.1080/00207543.2017.1401234.
- Moretto, A., A. Patrucco, and C. Harland. 2020. “The Dynamics of Reshoring Decisions and the Role of Purchasing.” International Journal of Production Research 58 (19): 5929–5944. https://doi.org/10.1080/00207543.2019.1661534.
- Paier, M. F., M. Dünser, and A. Unger. 2017. “Regional Knowledge Creation in a Global Industry: An Empirical Agent-Based Model of the Austrian Semiconductor Industry” (September 8, 2017). http://doi.org/10.2139/ssrn.3039326.
- Patterson, A. 2022. “TSMC’s Culture Clash at Arizona Fab.” EE Times, March 1, 2022. https://www.eetimes.com/tsmcs-arizona-culture-clash/.
- Peng, I. 2022. “Wait Times for Chip Deliveries Grow Again as Shortages Persist.” Bloomberg, March 11, 2022. https://www.bloomberg.com/news/articles/2022-03-11/wait-times-for-chip-deliveries-grow-again-as-shortages-persist.
- Pisano, G. P., and W. C. Shih. 2009. “Restoring American Competitiveness.” Harvard Business Review 87: 114–125.
- Pisano, G. P., and W. C. Shih. 2012. “Does America Really Need Manufacturing?” Harvard Business Review 90 (3): 94–102.
- Ramani, V., D. Ghosh, and M. S. Sodhi. 2022. “Understanding Systemic Disruption from the Covid-19-induced Semiconductor Shortage for the Auto Industry.” Omega 113. https://doi.org/10.1016/j.omega.2022.102720.
- Reuters. 2022. “Taiwan’s TSMC Progressing Well with Arizona Chip Plant”, Governor Says. Reuters August 31, 2022. https://www.reuters.com/technology/tsmc-making-excellent-progress-with-arizona-chip-plant-state-governor-says-2022-08-31/.
- Rowsell, J. 2022. “How Much Has the Semiconductor Shortage Cost?” Supply Management, May 5, 2022. https://www.cips.org/supply-management/news/2022/may/how-much-has-the-semiconductor-shortage-cost/.
- Scheper, F. 2022. “A Revitalized Semiconductor Industry Will Power Europe’s Digital Future.” World Economic Forum. Sep 2, 2022. https://www.weforum.org/agenda/2022/09/a-revitalized-semiconductor-industry-will-drive-europes-digital-future/.
- Scott, A. J., and D. P. Angel. 1987. “The US Semiconductor Industry: A Locational Analysis.” Environment and Planning 19 (7): 875–912. https://doi.org/10.1068/a190875.
- Sevilla, G. 2022. “TSMC Planning Another Price Increase.” Insider Intelligence, May 13, 2022. https://www.insiderintelligence.com/content/tsmc-planning-another-price-increase.
- Sheffi, Y. 2020. “The New (Ab)Normal: Reshaping Business and Supply Chain Strategy beyond Covid-19.” Transoft Inc. 314.
- Shekarian, M., and M. M. Parast. 2021. “An Integrative Approach to Supply Chain Disruption Risk and Resilience Management: A Literature Review.” International Journal of Logistics Research and Applications 24 (5): 427–455. https://doi.org/10.1080/13675567.2020.1763935.
- Shin, N., K. L. Kraemer, and J. Dedrick. 2016. “R&D and Firm Performance in the Semiconductor Industry.” Industry and Innovation 24 (3): 280–297. https://doi.org/10.1080/13662716.2016.1224708.
- SIA. 2021. “State of the U.S. Semiconductor Industry.” https://www.semiconductors.org/state-of-the-u-s-semiconductor-industry/.
- SIA. 2022. “Global Semiconductor Incentives February, 2022.” https://www.semiconductors.org/wp-content/uploads/2022/02/Global-Semiconductor-Incentives_2-4-2022.pdf.
- Srai, J. S., and C. Ané. 2016. “Institutional and Strategic Operations Perspectives on Manufacturing Reshoring.” International Journal of Production Research 54 (23): 7193–7211. https://doi.org/10.1080/00207543.2016.1193247.
- Terwiesch, Ch, and R. E. Bohn. 2001. “Learning and Process Improvement During Production Ramp-up.” International Journal of Production Economics 70 (1): 1–19. https://doi.org/10.1016/S0925-5273(00)00045-1.
- Thorbecke, W. 2021. “The Semiconductor Industry in the Age of Trade Wars, COVID-19, and Strategic Rivalries.” In Sanjay Kathuria (eds): Age of Ferment: Developments in Asian–European Trade Relations is published by the Konrad-Adenauer-Stiftung’s Regional Economic Programme Asia (SOPAS) 222p.
- Ting-Fang, Ch, and L. Li. 2022. “Chip Giant TSMC Plans Further Price Rises Amid Inflation Concerns.” Financial Times, May 17, 2022. https://www.ft.com/content/a5b1b807-1975-4bcb-be82-b894f56be40c.
- Usvyatsky, O., and J. Pakaluk. 2016. “What Depreciation at Intel Says about Moore’s Law. Audit Analytics, April 4, 2016. https://blog.auditanalytics.com/what-depreciation-at-intel-says-about-moores-law/.
- Uzsoy, R., J. W. Fowler, and L. Mönch. 2018. “A Survey of Semiconductor Supply Chain Models Part II: Demand Planning, Inventory Management, and Capacity Planning.” International Journal of Production Research 56 (13): 4546–4564. https://doi.org/10.1080/00207543.2018.1424363.
- Varas, A., R. Varadarajan, J. Godrich, and F. Yinug. 2020. Government Incentives and US Competitiveness in Semiconductor Manufacturing. BCG x SIA report. September 2020. https://www.semiconductors.org/wp-content/uploads/2020/09/SIA-Summary-of-Government-Incentives-and-US-Competitiveness-in-Semiconductor-Manufacturing-Report.pdf.
- Varas, A., R. Varadarajan, J. Godrich, and F. Yinug. 2021. “Strengthening the Global Semiconductor Supply Chain in an Uncertain Era.” BCG x SIA report. April 2021. https://www.semiconductors.org/strengthening-the-global-semiconductor-supply-chain-in-an-uncertain-era/.
- Vörös, J. 2013. “Multi-period Models for Analyzing the Dynamics of Process Improvement Activities.” European Journal of Operational Research 230 (3): 615–623. https://doi.org/10.1016/j.ejor.2013.04.036.
- Vörös, J. 2019. “An Analysis of the Dynamic Price-Quality Relationship.” European Journal of Operational Research 277: 1037–1045. https://doi.org/10.1016/j.ejor.2019.03.032.
- Vörös, J. 2021. “Production Dynamics in Case of Organizational Learning.” Computers & Industrial Engineering 157: 107340. https://doi.org/10.1016/j.cie.2021.107340.
- Walsh, D. 2022. “How Auto Companies are Adapting to the Global Chip Shortage.” MIT Sloan Jan 21, 2022. https://mitsloan.mit.edu/ideas-made-to-matter/how-auto-companies-are-adapting-to-global-chip-shortage.
- The White House. 2022. “Fact Sheet: CHIPS and Science Act Will Lower Costs, Create Jobs, Strengthen Supply Chains, and Counter China.” August 09, 2022. https://www.whitehouse.gov/briefing-room/statements-releases/2022/08/09/fact-sheet-chips-and-science-act-will-lower-costs-create-jobs-strengthen-supply-chains-and-counter-china/.
- Wu, J.-Z., and Ch-F. Chien. 2008. “Modeling Strategic Semiconductor Assembly Outsourcing Decisions Based on Empirical Settings.” OR Spectrum 30 (3): 401–430. https://doi.org/10.1007/s00291-007-0120-5.
- Xiao, W., and C. Gaimon. 2013. “The Effect of Learning and Integration Investment on Manufacturing Outsourcing Decisions: A Game Theoretic Approach.” Production and Operations Management 22 (6): 1576–1592. https://doi.org/10.1111/poms.12042.
- Xu, X. S., L. Q. Lu, G. Q. Huang, and T. Zhang. 2013. “Scope Economies, Market Information, and Make-or-Buy Decision Under Asymmetric Information.” International Journal of Production Economics 145 (1): 339–348. https://doi.org/10.1016/j.ijpe.2013.05.002.
- Yeung, A., K. Lai, and R. Yee. 2007. “Organizational Learning, Innovativeness, and Organizational Performance: A Qualitative Investigation.” International Journal of Production Research 45 (11): 2459–2477. https://doi.org/10.1080/00207540601020460.