ABSTRACT
Dynamic task scheduling problem in cloud manufacturing (CMfg) is always challenging because of changing manufacturing requirements and services. To make instant decisions for task requirements, deep reinforcement learning-based (DRL-based) methods have been broadly applied to learn the scheduling policies of service providers. However, the current DRL-based scheduling methods struggle to fine-tune a pre-trained policy effectively. The resulting training from scratch takes more time and may easily overfit the environment. Additionally, most DRL-based methods with uneven action distribution and inefficient output masks largely reduce the training efficiency, thus degrading the solution quality. To this end, this paper proposes an improved DRL-based approach for dynamic task scheduling in CMfg. First, the paper uncovers the causes behind the inadequate fine-tuning ability and low training efficiency observed in existing DRL-based scheduling methods. Subsequently, a novel approach is proposed to address these issues by updating the scheduling policy while considering the distribution distance between the pre-training dataset and the in-training policy. Uncertainty weights are introduced to the loss function, and the output mask is extended to the updating procedures. Numerical experiments on thirty actual scheduling instances validate that the solution quality and generalization of the proposed approach surpass other DRL-based methods at most by 32.8% and 28.6%, respectively. Additionally, our method can effectively fine-tune a pre-trained scheduling policy, resulting in an average reward increase of up to 23.8%.
Disclosure statement
No potential conflict of interest was reported by the author(s).
Data Availability Statement
The data that support the findings of this study are available from the corresponding author, [Lin Zhang], upon reasonable request.
Notes
1 Fine-tuning in DRL means that continuously training a pre-trained policy with online access to the environment, and the pre-training is usually implemented offline with datasets collected by a random policy. Unlike supervised learning which is usually trained offline, DRL relies upon online interactions with the environment to adjust its policy.
Additional information
Funding
Notes on contributors
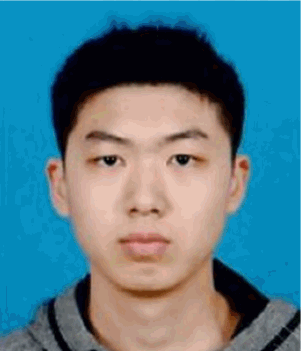
Xiaohan Wang
Xiaohan Wang is a Ph.D. student in School of Automation Science and Electrical Engineering at Beihang University. He received B.S. degree from Beihang University in 2019. He received the recommended test-free qualification for Ph.D. in 2018 and obtained the outstanding graduate of Beihang University in 2019. His research interests include dynamic scheduling, deep reinforcement learning, surrogate-assisted evolutionary algorithms, and planning in cloud manufacturing.
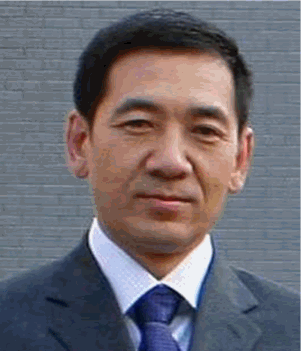
Lin Zhang
Lin Zhang received the B.S. degree in 1986 from the Department of Computer and System Science at Nankai University, China. He received the M.S. degree and the Ph.D. degree in 1989 and 1992 from the Department of Automation at Tsinghua University, China, where he worked as an associate professor from 1994. He served as the director of CIMS Office, National 863 Program, China Ministry of Science and Technology, from December 1997 to August 2001. From 2002 to 2005 he worked at the US Naval Postgraduate School as a senior research associate of the US National Research Council. Now he is a full professor in Beihang University. He is an associate Editor-in-Chief of “International Journal of Modeling, Simulation, and Scientific Computing”. His research interests include cloud manufacturing, system modeling and simulation, and software engineer-ing. Prof. Zhang is an IEEE senior member and a director of board of SCS.
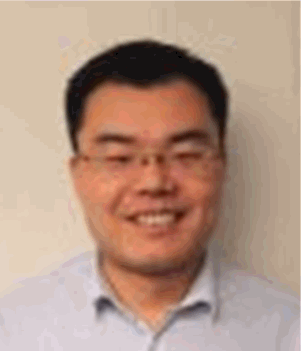
Yongkui Liu
Yongkui Liu received the Ph.D. degree from Xidian University, Xi'an, China, in 2010. He is currently an Associate Professor with the School of Mechano Electronic Engineering, Xidian University. His current research interests include deep reinforcement learning, scheduling, and control in cloud manufacturing.
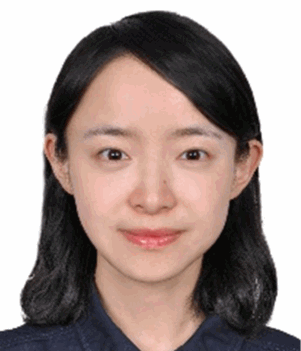
Yuanjun Laili
Yuanjun Laili received the B.S., M.S., and Ph.D. Degree from the School of Automation Science and Electrical Engineering at Beihang University. She is an Assistant Professor of the School of Automation Science and Electrical Engineering, Beihang University. She is a member of IEEE Robotics and Automation Society and SCS (The Society For Modeling and Simulation International). She is also an Associate Editor of “International Journal of Modeling, Simulation, and Scientific Computing”. She has won the “Young Talent Lift Project” supported by China Association for Science and Technology and the “Young Simulation Scientist Award” from SCS. Her main research interests are in the areas of intelligent optimization, modeling and simulation of manufacturing systems.