ABSTRACT
Pick-Up Points (PUPs) represent an alternative delivery option for online purchases. Parcels are delivered at a reduced cost to PUPs and wait until being picked up by customers or returned to the original warehouse if their sojourn time is over. When the chosen PUP is overloaded, the parcel may be refused and delivered to the next available PUP on the carrier tour. This paper presents and compares forecasting approaches for the load of a PUP to help PUP management companies balance delivery flows and reduce PUP overload. The parcel life-cycle has been taken into account in the forecasting process via models of the flow of parcel orders, the parcel delivery delays, and the pick-up process. Model-driven and data-driven approaches are compared in terms of load-prediction accuracy. For the considered example, the best approach (which makes use of the relationship of the load with the delivery and pick-up processes) is able to predict the load up to 4 days ahead with mean absolute errors ranging from 3.16 parcels (1 day ahead) to 8.51 parcels (4 days ahead) for a PUP with an average load of 45 parcels.
Acknowledgments
We thank Professor Valdério Anselmo Reisen for his valuable comments.
Data Availability Statement
The data that support the findings of this study are openly available in Github at https://github.com/cabani/ForecastingParcels.
Disclosure statement
No potential conflict of interest was reported by the author(s).
Notes
1 Based on the statistical analysis in Appendix A, 80 % of the parcels are picked up two days after their delivery, and only 1 % are still in the PUP six days after their delivery. The considered simplifying assumption about only marginally impacts the load prediction results.
Additional information
Notes on contributors
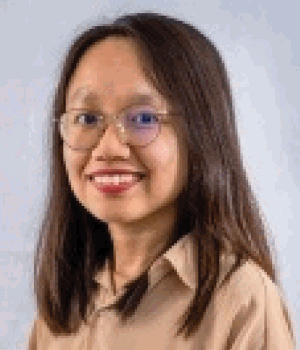
Thi-Thu-Tam Nguyen
Tam Nguyen received her PhD degree in Computer Science from Paris Saclay University, Orsay, France in 2023. Her research interests include statistical and machine learning based models applied to time series analysis and forecasting.
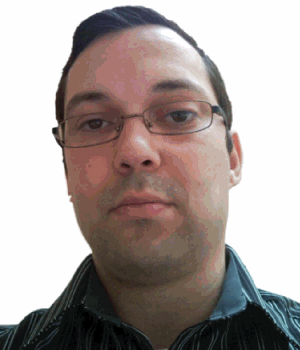
Adnane Cabani
Adnane Cabani received his Ph.D. degree in Computer Science from INSA Rouen Normandie in December 2007. He obtained his HDR, French Accreditation to Direct Research, in March 2021 from University of Rouen Normandy. He is a Professor of Computer Science at ESIGELEC/IRSEEM. Currently, he is the head of the Software Engineering & Digital Transformation Master's programme and a member of the steering committee of the federative structure in logistics SFLog FED 4230. He has co-authored 70 research papers, conference proceedings, books, and standards in the areas of Intelligent Transport Systems and Logistics.
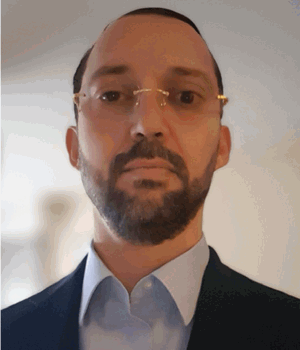
Iyadh Cabani
Iyadh Cabani received his Ph.D. degree in Computer Science from INSA Rouen Normandie in 2007. He obtained the Executive Certificate, BigData for Digital Business from CentraleSupelec in 2022. He has been a reviewer for several years in IEEE conferences and journals in the field of image processing. In addition, he held the positions of Chief Data Officer and Data Protection Officer within the Pickup Group (a subsidiary of La Poste Group). He is now Chief Executive Officer of uUsUu SAS specialising in the field of consulting on the themes of complex program management, data-centric information systems, and artificial intelligence.
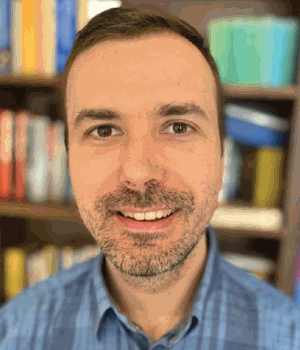
Koen De Turck
Koen De Turck is an associate professor at the TELIN department of the Faculty of Engineering and Architecture at Ghent University since October 2021. From 2015 to 2021, he was an associate professor (MCF) at the Telecommunications Department of CentraleSupélec and a researcher at the Laboratoire des Signaux et Systémes, Gif-sur-Yvette. His research interests include stochastic modelling of telecommunication systems, queueing theory, scaling methods, and asymptotic analysis. These techniques are applied to a number of domains, notably including wireless networks, and in particular, energy efficiency and bandwidth reutilisation. He is also interested random graph methods, stochastic learning and designing efficient simulation algorithms.
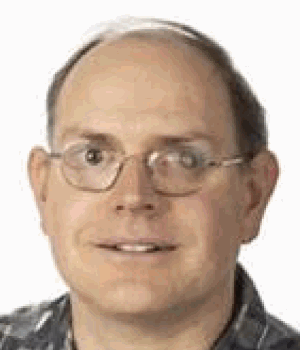
Michel Kieffer
Michel Kieffer is a full professor in signal processing for communications at Université Paris-Saclay and a researcher at the Laboratoire des Signaux et Systémes, Gif-sur-Yvette. His research interests are in signal processing for multimedia, communications, and networking, distributed source coding, network coding, joint source-channel coding and decoding, joint source-network coding. Michel Kieffer is co-author of more than 200 contributions in journals and in conference proceedings. He filed 12 patents and is co-author of 2 books: Applied Interval Analysis published by Springer-Verlag in 2001, and Joint source-channel decoding: A cross layer perspective with applications in video broadcasting published by Academic Press in 2009. He serves as associate editor of Signal Processing since 2008 and of the IEEE Transactions on Communications from 2012 to 2016. From 2011 to 2016, Michel Kieffer was a junior member of the Institut Universitaire de France.