Abstract
Distributed manufacturing is gradually becoming the future trend. The fierce market competition makes manufacturing companies focus on productivity and product delivery. The hybrid flow shop scheduling problem (HFSP) is common in manufacturing. Considering the difference of machines at the same stage, the multi-objective distributed hybrid flow shop scheduling problem with unrelated parallel machines (MODHFSP-UPM) is studied with minimum makespan and total tardiness. An improved multi-population genetic algorithm (IMPGA) is proposed for MODHFSP-UPM. The neighbourhood structure is essential for meta-heuristic-based solving algorithms. The greedy job insertion inter-factory neighbourhoods and corresponding move evaluation method are designed to ensure the efficiency of local search. To enhance the optimisation ability and stability of IMPGA, sub-regional coevolution among multiple populations and re-initialisation procedure based on probability sampling are designed, respectively. In computational experiments, 120 instances (including the same proportion of medium and large-scale problems) are randomly generated. The IMPGA performs best in all indicators (spread, generational distance, and inverted generational distance), significantly outperforming existing efficient algorithms for MODHFSP-UPM. Finally, the proposed method effectively solves a polyester film manufacturing case, reducing the makespan and total tardiness by 40% and 60%, respectively.
KEYWORDS:
- Distributed hybrid flow shop with unrelated parallel machines
- Multi-objective scheduling considering total tardiness
- Improved multi-population genetic algorithm
- Greedy job insertion inter-factory neighbourhoods
- Rapid evaluation method for inter-factory neighbourhoods
- Sub-regional coevolution among multiple populations
Disclosure statement
No potential conflict of interest was reported by the author(s).
Additional information
Funding
Notes on contributors
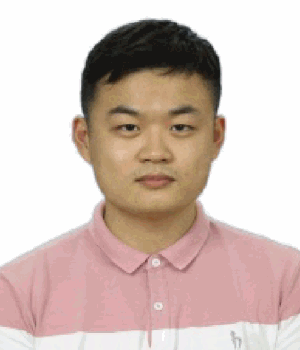
Hanghao Cui
Hanghao Cui received the master’s degree in industrial engineering from Huazhong University of Science and Technology, Wuhan, China, 2021. He is currently pursuing the Ph.D. degree in mechanical engineering with the Huazhong University of Science and Technology. His research interests are in intelligent optimisation algorithms and their application to shop scheduling.
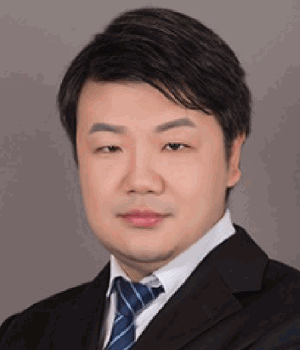
Xinyu Li
Xinyu Li received the Ph.D. degree in industrial engineering from Huazhong University of Science and Technology (HUST), China, 2009. He is a Professor of the Department of Industrial & Manufacturing Systems Engineering, State Key Laboratory of Digital Manufacturing Equipment & Technology, School of Mechanical Science & Engineering, HUST. He had published more than 100 refereed papers. His research interests include intelligent scheduling, machine learning etc.
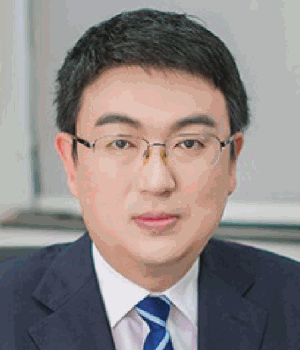
Liang Gao
Liang Gao received the Ph.D. degree in mechatronic engineering from Huazhong University of Science and Technology (HUST), China, 2002. He is a Professor of the Department of Industrial & Manufacturing System Engineering, State Key Laboratory of Digital Manufacturing Equipment & Technology, School of Mechanical Science & Engineering, HUST. He had published more than 470 refereed papers. His research interests include operations research and optimisation, scheduling, big data and machine learning etc. Prof. GAO is an Associate Editor of Swarm and Evolutionary Computation, Journal of Industrial and Production Engineering and. He is co-Editor-in-chief of IET Collaborative and Intelligent Manufacturing.
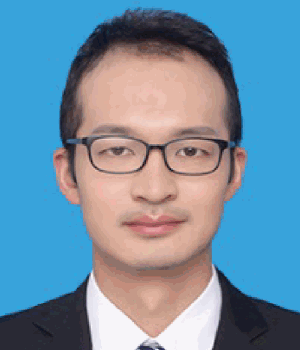
Chunjiang Zhang
Chunjiang Zhang received the B.Eng. and Ph.D. degrees in industrial engineering from Huazhong University of Science and Technology (HUST), Wuhan, China, in 2011 and 2016, respectively. He is currently a lecturer with HUST. His current research interests include evolutionary algorithms, constrained optimisation, multi-objective optimisation, and their applications in production scheduling.