Abstract
This paper proposes a novel intelligent scheduling method based on deep reinforcement learning (DRL) to solve the multi-objective steelmaking-continuous casting (SCC) scheduling problem, under time-of-use (TOU) tariffs for the first time. The intelligent scheduling system architecture is designed, and a mathematical model is established to minimise the total sojourn time and electricity cost. To effectively reduce production costs by avoiding peak periods of electricity consumption, the ‘start time’ of the system is generated based on the Markov Decision Process (MDP), and heuristic scheduling rules related to power cost are used as the action space, with corresponding reward functions designed according to the characteristics of these two objectives. To satisfy the continuous casting which is a particular SCC constraint, a backward strategy is developed. Additionally, a branching duelling double deep Q-network (BD3QN) is adapted to guide action selection and avoid blind search in the iteration process, and then applied to real-time scheduling. Numerical experiments demonstrate that the proposed method outperforms comparison algorithms in terms of solution quality and CPU times by a large margin.
Data availability statement
The authors confirm that the data supporting the findings of this study are available within the article [and/or] its supplementary materials.
Disclosure statement
No potential conflict of interest was reported by the author(s).
Additional information
Funding
Notes on contributors
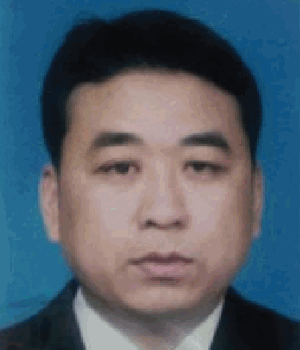
Ruilin Pan
Ruilin Pan received the Ph.D. degree in Enterprise Management from Dalian University of Technology, Dalian, China, in 2010. He is currently a Professor of Operations Management with the School of Management Science and Engineering, Anhui University of Technology, Anhui, China. His research interests include industrial data science, machine learning, and reinforcement learning. He has published papers in journals such as Annals of Operations Research, Swarm and Evolutionary Computation, Journal of Intelligent Manufacturing, European Journal of Operational Research, and Computers & Industrial Engineering.
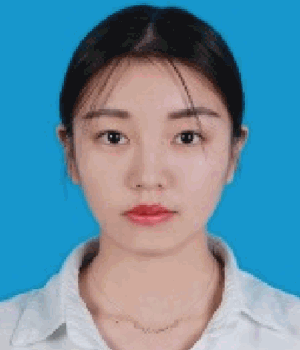
Qiong Wang
Qiong Wang received the M.E. degree in Management Science and Engineering from Anhui University of Technology, Anhui, China, in 2022. Her research interests include operations planning and scheduling problems in production, mathematical modelling, optimisation and heuristic methods.
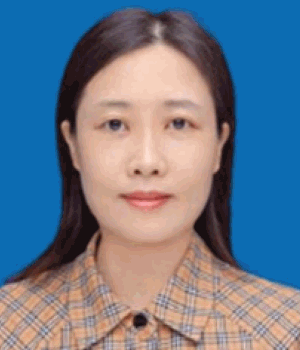
Jianhua Cao
Jianhua Cao received the Ph.D. degree in Business Administration from Zhejiang University of Technology, Hangzhou, China, in 2022. She is currently an Associate Professor of Operations Management with the School of Management Science and Engineering, Anhui University of Technology, Anhui, China. Her research interests include operations research and optimisation, production scheduling and machine learning. She has published papers in journals such as Annals of Operations Research, Swarm and Evolutionary Computation, Transportation Letters, and Computers & Industrial Engineering.
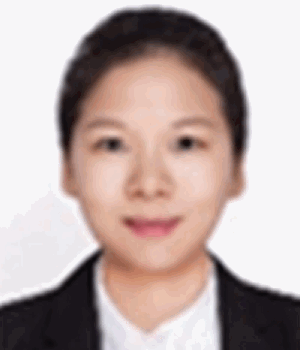
Chunliu Zhou
Chunliu Zhou received the Ph.D. degree in Enterprise Management from the Dalian University of Technology, Dalian, China, in 2020. She is currently a Lecturer at the Department of Industrial Engineering, Anhui University of Technology, Anhui, China. Her research interests include production planning and control, product data management, and data-driven process management. She has published papers in journals such as Advanced Engineering Informatics and Industrial Engineering and Management.