Abstract
With the rise of Internet of Things (IoT) technology, the seamless connection between the physical and digital worlds has been realized. This review paper aims to conduct a science mapping review of IoT applications in industrial management and to identify mainstream research topics, research gaps, and future research directions. Using VOSviewer as a visualization tool, 142 articles retrieved from the Scopus database were quantitatively discussed using scientometric analysis. Additionally, a follow-up qualitative discussion was focused on mainstream research topics, existing research gaps, and future research directions as the main research goals. The results revealed influential findings for the co-occurrence of keywords, journals, countries, authors, and documents analyses. Moreover, it was found that the existing research mainly focused on four main research topics including (1) application of IoT in manufacturing based on cyber-physical systems, (2) IoT-related technologies on logistics and supply chain management, (3) The impact of IoT on business models, and (4) Industrial IoT (IIoT) in the context of Industry 4.0. On this basis, the existing research gaps and future research directions are proposed. This review paper would help relevant practitioners and researchers to better understand the existing body of knowledge and lay the foundation for further research.
1. Introduction
Internet of Things (IoT) can be thought of as a global network infrastructure consisting of a multitude of connected devices that rely on sensing, communication, networking, and information processing technologies (Tan and Wang Citation2010; Radanliev et al. Citation2021; Ystgaard et al. Citation2023). These devices have enhanced sensing, computing, and communication capabilities that allow them to communicate, sense, or interact with their internal state or external environment (Caron et al. Citation2016; Ammar, Russello, and Crispo Citation2018). Also, IoT systems are the next revolutionary concept in transforming the internet into a fully integrated network, enabling harmonious interaction between societies, individuals, and intelligent systems (Guo et al. Citation2013; Malik Citation2022). With technologies such as wireless sensor networks (WSN), radio-frequency identification (RFID), and Micro-Electro-Mechanical Systems (MEMS) continuing to mature, IoT expands the ability to access and use information. At the same time, manufacturing efficiency is increased, product quality is improved, product costs and resource consumption are reduced, and more transparent and personalised services are provided to users (Fireteanu Citation2020). IoT technology, one of the world's hottest technology areas, is now gradually being used in various fields.
To date, many industrial companies are striving to transform their digital initiatives and these new technologies are turning the industry upside down on a large scale (Ghosh et al. Citation2022). For example, automated cockpits are becoming increasingly sophisticated and replacing traditional cockpits in airlines (Casner et al. Citation2014). In healthcare, cloud computing can streamline document management procedures, even when accessing patients’ data, making it simple for healthcare providers, including hospitals, clinics, imaging centres, and insurance companies, to distribute medical data, prescription information, radiology, test results, and other pertinent information (Sharma et al. Citation2021). The integration of the new generation of information technology and the industry has accelerated the process of industrial digitisation, networking, and intelligence (Zhou et al. Citation2018; Yang et al. Citation2021). In the process of industrialisation, the application of IoT is also an important way to promote the degree of industrial automation and the continuous improvement of management information. It is of great significance to realise new industrialisation, improve product competitiveness and innovate industrial production, management, and business models (Fireteanu Citation2020). Applications of IoT are related to various aspects of the industry, including supply chain management in manufacturing, such as improving the efficiency of supply chain management by increasing the transparency of information, enhancing the integration of management information systems, and improving big data processing capabilities (Cui et al. Citation2020).
Besides, IoT can optimise the production processes, such as enabling product lifecycle tracking, inspection, storage, and use during product returns in product lifecycle data management (Fang et al. Citation2016; Meng, Wu, and Gray Citation2018). In addition, IoT can enable product and equipment monitoring and management, such as using IoT to collect and inspect real-time data for online monitoring of production processes (Browne Citation2021). What’s more, environmental testing and energy management have extensively utilised IoT technology, such as optimal control, scheduling, forecasting, and coordination of energy assets across regions, enabling transferable use across different energy sectors (O’Dwyer et al. Citation2020). Likewise, industrial safety management has also widely used IoT, such as the use of IoT technology to create a protective barrier of multiple security protections to prevent and delay any cyber-attacks (Mugarza, Flores, and Montero Citation2020). Taken together, IoT can enhance business operations at many levels and can help the industrial sectors to maintain their competitiveness in the market (Javaid et al. Citation2021). Therefore, the application of IoT in the industry is an inevitable trend in the development of industrial information technology.
Although the outstanding capabilities of IoT in various fields are apparent, there are still some problems. On the one hand, in the process of promoting the application of IoT, large enterprises, with their scale effect and strong financial support, can break through the cost constraints and apply IoT to actual production. However, for some small and medium-sized industrial enterprises, the traditional concepts of system integration and custom development cannot be applied to these enterprises and are too costly to build (Okkonen, Hyysalo, and Peltonen Citation2020). On the other hand, the adoption of IoT in developing countries faces the dilemma of needing to improve human and financial support, low level of informatization, and insufficient integration with industrialisation (Mital et al. Citation2018). In addition, the use of IoT comes with unexpected risks associated with big data (Brous, Janssen, and Herder Citation2020). For example, data leaks may reveal sensitive personal information (Skarmeta, Hernandez-Ramos, and Moreno Citation2014; Alghamdi and Bellaiche Citation2023). Lee and Lee (Citation2015) highlighted several inherent vulnerabilities in IoT devices, such as insecure web interfaces and the lack of encryption and authentication of transmissions that can pose a threat to information security. Information technology (IT) infrastructure also affects the project process (Lin, Yang, and Shyua Citation2013), new algorithms and technologies are needed for data processing and storage (Weinberg et al. Citation2015; Abdallah, Mnasri, and Nasri Citation2020). Deficiencies in data management are also a key issue limiting IoT development (Stephan et al. Citation2013; Zhang et al. Citation2021). IoT can steer the need for distributed data centre management to improve data processing efficiency and speed up response times (Bradley, Thibodeau, and Ng Citation2014; Mehta, Mittra, and Yadav Citation2018). Despite the relevance of data collected from IoT devices, numerous applications of IoT remain unexplored. This assertion may be due to a large amount of data (i.e. big data), high-frequency updates, and information disparity (Malik et al. Citation2018). In addition to these objective factors, human conditions also play an important role in the development of IoT. There is a lack of support for IoT in organisations (Carcary et al. Citation2018), a lack of knowledge among senior managers (Ferretti and Schiavone Citation2016), a lack of awareness of security privacy issues (Storey Citation2014), and a lack of training of staff in relevant skills (Valmohammadi Citation2016). Overall, IoT may face various problems/constraints in its application, which are yet to be solved.
In terms of future trends, 5G will be the key to the future development of IoT. Gartner (Citation2021) reported that the number of 5G patent applications increased fivefold from 2015 to 2021, demonstrating the market's expectation of the current and future potential of 5G, and these figures are likely to increase in recent years. 5G networks open new opportunities for manufacturers, including accelerating the IoT with faster data transfer rates and more efficient connectivity and communication between devices. But along with these opportunities come cyber security risks (Ahmad et al. Citation2018). Not only will 5G require a physical overhaul of networks containing more IoT devices, but we will also see a shift from hardware to software networks. However, given that these networks involve the collection of large amounts of sensitive data shared between an increasing number of connected devices, the software will introduce new network vulnerabilities and exacerbate existing unforeseen risks. In addition, machine learning, edge computing, and flexible supply chains will also be an important part of the future development of IoT. Therefore, the rapid development of IoT is an inevitable trend, and the research prospects are very broad.
Over the past decade, as digital transformation technologies have accelerated, numerous IoT technologies have been integrated into industry-related projects and the number of publications related to them has been increasing yearly. Several existing review-based studies on the applications of IoT are based on other disciplines. In the medical field, Brites et al. (Citation2022) conducted a literature review on the applications of IoT in identifying diseases through heart sounds and laying the groundwork for future work. In the construction industry, Ghosh, Edwards, and Hosseini (Citation2020) outlined the key drivers of IoT development, particularly the organisational, economic-related barriers, revealing knowledge gaps in contemporary research. In manufacturing, Hipsher and Duffy (Citation2021) conducted a literature review based on articles related to IoT in manufacturing, finding great advances and potential weaknesses for the manufacturing industry. In the logistics industry, Katoch (Citation2022) identified the role of IoT in supply chain management and found potential research areas. Moreover, Gupta (Citation2021) explored the status and trends of the scientific literature on IoT as a whole, identifying publication trends, the most productive authors, institutions, journals, and countries. Given the numerous existing review-based studies, there is no study on the review of IoT in the field of industrial management. As such, there is a research gap in the state-of-the-art review related to industrial management. As the scope of IoT applications in the industry expands, the demand for literature analysis in this area is increasing. The relevant literature needs to be collated to help practitioners access information easily and to identify future trends for relevant scholars. Therefore, it is necessary to conduct a science mapping of IoT applications in industrial management.
Given the above, this review-based study aims to conduct a science mapping review of IoT applications in industrial management and to identify mainstream research topics, research gaps, and future research directions. The specific objectives of this review study include:
Analyze the annual research publication trends and peer-reviewed journals on IoT applications in industrial management.
Conduct a scientometric analysis on co-occurrence analysis of keywords, authors, countries, citation networks, and document analysis.
Identify mainstream research topics on IoT applications in industrial management.
Recommend future research directions on IoT applications in industrial management.
The remainder of the review paper is as follows. Section 2 discusses the research methodology. Section 3 reports the scientometric analysis results on co-occurrence analysis of keywords, authors, countries, citation networks, and document analysis, while Section 4 highlights the mainstream topics, research gaps, research trends on IoT applications in industrial management, and contributions to theory, practice, and policy. Lastly, the conclusions are summarised in Section 5.
2. Research methods
This review study uses a four-step approach to summarise research on the application areas of IoT in industrial management by indexing the existing literature in the Scopus database. The findings would provide academics and industry practitioners with an in-depth understanding of IoT in the research area of industrial management. In this study, an interpretivist epistemology with facets of positivism (Saunders, Lewis, and Thornhill Citation2007) was used to conduct a state-of-the-art review, where an in-depth understanding, scope, and validity of published articles were selected based on a four-step methodological approach. The four-step approach includes: (1) literature search, (2) literature selection, (3) scientometric analysis, and (4) qualitative discussion. Figure shows an overview of the four-step approach.
Figure 1. An overview of the four-step approach.
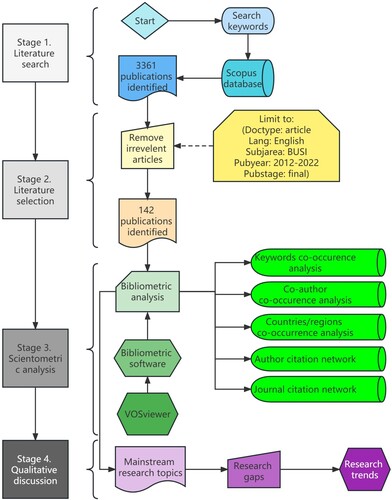
2.1. Literature search
To analyze the data using scientometric analysis, the search was conducted in the Scopus database. Scopus has become the standard for research in the field of scientometric and bibliometric analyzes (Rose and Kitchin Citation2019). It is also a highly influential and comprehensive citation database of multidisciplinary articles and is extremely useful for interdisciplinary research (Zhong et al. Citation2019). Compared with other available digital resources (e.g. Web of Science), Scopus covers more journals and more recent publications than them (Chadegani et al. Citation2013). Scopus is also widely regarded as a comprehensive and reliable database. World-class journals from well-known publishers can be searched in the Scopus database (Katoch Citation2022). Also, Scopus’ indexing process is faster than other databases (Meho and Rogers Citation2008). Therefore, it has also been widely used in previous review studies (Hosseini et al. Citation2018; Wu et al. Citation2022; Antwi-Afari et al. Citation2023). To retrieve literature samples, the search was conducted in the Scopus database by using keywords. A total of 3,361 documents were initially found in the TITLE-ABS-KEY (internet AND of AND things) AND TITLE-ABS-KEY (industrial AND management).
2.2. Literature selection
The search results were refined by selecting literature from the last decade (2012-2022). The publication search was restricted to the zone of business, management, and accounting. Since academic journal articles undergo rigorous peer review and many authors publish their works in scholarly journals, the search results are limited to journal articles and articles written in English (Olawumi, Chan, and Wong Citation2017). Notably, journal articles often provide more comprehensive and higher quality information than other types of publications (Zhong et al. Citation2019). After initial screening, a total of 142 documents were retained in the literature sample. The complete research string is as follows:
(TITLE-ABS-KEY (internet AND of AND things) AND TITLE-ABS-KEY (industrial AND management)) AND (LIMIT-TO (PUBYEAR ≥ 2012) AND (LIMIT-TO (PUBYEAR ≤ 2022)) AND (LIMIT-TO (PUBSTAGE, ‘final’)) AND (LIMIT-TO (DOCTYPE, ‘ar’)) AND (LIMIT-TO (SUBJAREA, ‘BUSI’)) AND (LIMIT-TO (LANGUAGE, ‘English’)) AND (LIMIT-TO (SRCTYPE, ‘j’)).
2.3. Scientometric analysis
The third step of the review was a scientometric analysis using VOSviewer. It is a software tool for building visual scientometric networks. It provides text-mining capabilities and can also be used to construct and visualise co-occurrence networks of key terms extracted from the body of scientific literature. VOSviewer automatically identifies terms and builds scientometric maps from web data (Colares et al. Citation2020). It is informative, graphically simple to understand, and easy to analyze from multiple perspectives. The main benefits of VOSviewer over other scientometric software include its capacity for graphical presentation, adaptability for large-scale data, and versatility in adapting to different databases and sources in different formats (Van Eck and Waltman Citation2010).
Scientometric analysis refers to the cross-cutting science of mathematical and statistical methods for the quantitative analysis of all knowledge vectors (Pritchard Citation1969) and is considered to be a very useful aid for decision-making activities such as setting research priorities and tracking scientific and technological developments (Mejia et al. Citation2021). Scientometric analysis is used to conduct keywords co-occurrence analysis, co-author co-occurrence analysis, countries/regions co-occurrence analysis, author citation network, and journal citation network to find keywords, authors, countries, journals, and documents that contribute high quality work in the field. In addition, it establishes links between numerous literature samples to make an objective assessment of the content and structural characteristics of the literature (Moosavi et al. Citation2021).
2.4. Qualitative discussion
This section discusses the research contributions of IoT in industrial management based on the outputs of the scientometric analysis, building on the previous literature, and identifying current research gaps as well as limitations. Scientometric analysis of keywords, authors, country co-occurrence analysis, and document analysis was used to explore in-depth discussion of the mainstream topics of research on the applications of IoT in industrial management and recommend future research directions according to research trends for scholars in related industries to extend research works in this field.
3. Results
3.1. Annual publication trend
Figure presents the annual publication trends of research articles in the studied area. From 2012 to 2022, there is an overall upward trend in articles related to the applications of IoT in industrial management. Although the development was flat between 2012 and 2016, this was mainly related to the popularity of IoT and the degree of industrialisation. However, with the emergence of Industry 4.0 and the introduction of Industrial Internet of Things (IIoT), the development path of industrial manufacturing intelligence and digitalisation was clarified, and the digital-for-transformation of various industries was promoted. It has thus welcomed a great deal of attention from scholars and a growing number of studies related to it, with a sharp increase in the number of articles published from 2017 onwards. Although there is an inflection point in 2020 due to political, economic, social, health, and technological reasons, the overall trend is still on an upward trend. The highest number of publications was in 2021 with 40 relevant articles, a 60% rise compared to 2020. The next highest percentage increase was in 2019, with 32 relevant articles published, up 113.33% compared to 2018. Notably, this review study only retrieved articles published in the first seven months of 2022 and used MATLAB to conduct a linear regression of the number of articles published. It was found that 37 articles are expected to be published in 2022 and 42 articles are expected to be published in 2023. Overall, the application of IoT in industrial management as a popular research topic is predicted to continue to rise in related research results in the next few years.
3.2. Journal sources
Based on the 142 retrieved articles from Scopus, it was found that the research articles on IoT in industrial management in the last decade are mainly published in 84 journals. Table shows the top 15 journal sources. These journal sources cover a wide range of areas (e.g. engineering and technology), reflecting the richness, diversity, and multidisciplinary style of research into the applications of the IoT in industrial management. Among them, 23 journals had published two or more articles, accounting for 27.38%, and the publication of articles was relatively scattered. These journals are mainly from India, the United Kingdom, and the United States, which shows a higher level of interest in the studied topic in these countries. The journal with the most published articles is the International Journal of Production Research, with a total of 10 articles, accounting for 7.09% of the total number of published articles. This journal publishes papers on innovation management, product design, manufacturing processes, production, and logistics systems. In addition, manufacturing strategy, policy development and evaluation, and the contribution of new technologies are also major concerns of this journal. However, the proportion of high-level journals is low, and there is much room for improvement in the level of research papers and interdisciplinary research.
Table 1. Top 15 selected journal sources.
3.3. Keywords co-occurrence analysis
Keyword co-occurrence networks focus on understanding the connections between keywords in the literature by examining the knowledge components and knowledge structures of the scientific/technical domain. In a keyword co-occurrence network, keywords serve as nodes of the network, and the co-occurrence relationship between keywords constitutes the connection between nodes. The number of co-occurrences of a word pair in different articles is the weight of the link connecting the word pair. By analyzing the structure and intensity of links between keywords that exist in the literature, networks formed in this way can reveal significant knowledge components and insights. They can also represent the cumulative knowledge of an area (Radhakrishnan et al. Citation2017). Firstly, ‘Author Keywords’ and ‘Fractional Counting’ were selected according to the recommendations of Eck and Waltman (Citation2014). Secondly, the minimum number of occurrences of a keyword was set to 3. Out of a total of 593, 30 keywords met the threshold. The next step was to remove all the keywords that were semantically identical and repetitive, including IIoT, Industry 4.0, IoT, internet of things (IoT), fourth industrial revolution, cyber-physical systems, management, digitalisation, and manufacturing. After a second screening, 21 keywords were selected.
Figure illustrates the keywords co-occurrence analysis. The keywords as shown in Figure are grouped into six clusters, with keywords in the same cluster often having a closer internal relationship. For example, articles related to supply chain management often require the use of cloud computing, big data, and artificial intelligence (AI) as technical support. The distance between keywords and the connecting lines indicates their interconnectedness. For example, IIoT, digital technologies and digital transformation; smart manufacturing and sustainability are closely related to each other.
Figure 3. Keywords co-occurrence network of IoT applications in industrial management.
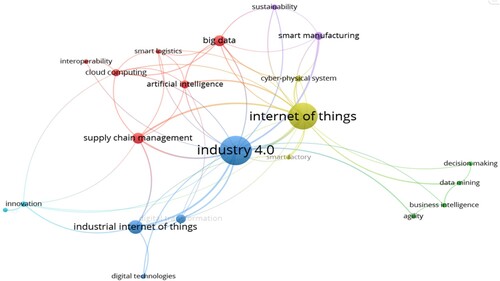
Table depicts the top 15 keywords arranged by their average normalised citations. According to Table , the keywords that appear frequently include ‘Industry 4.0’, ‘Internet of things’ and ‘Big data’. The keywords ‘Industrial internet of things’ and ‘Supply chain management’ also frequently appeared. ‘Industry 4.0’ is the most frequently used keyword, which indicates that the continued development of Industry 4.0 in the context of Industry 4.0 will result in a large-scale, fast-growing IoT market, while the application of IoT is also crucial for the realisation of Industry 4.0. In the third occurrence position (although couldn’t appear in Table ), ‘Big data’ as an IoT-enabled technology is leveraging the value of the IoT and in turn stimulating demand for its use. IIoT, a sub-category of IoT, is also more focused on industrial applications. It can be observed that the keywords with the highest average citation and average normalised citation are not necessarily with the highest occurrence. Average normalised citation top keywords include ‘Cloud computing’, ‘Interoperability’ and ‘Digital transformation’. Research has shown that studies focus on the impact of advanced technologies on the industrial sector (Xu, Xu, and Li Citation2018) and the contribution made by IoT in the digital transformation process (Butschan et al. Citation2019). In addition, the most novel research key topics and trends in research directions can be found in the published keywords from average publication year. The most current keyword is ‘Decision making’, followed by ‘Digital technologies’. Relatively traditional keywords include ‘Interoperability’ and ‘Business intelligence’.
Table 2. Top 15 keywords in IoT applications in industrial management.
From the observation of Figure and Table , the following keywords in the following directions can represent the current mainstream research directions for IoT in industrial management:
Application of IoT in the supply chain. Digitisation presents significant opportunities for supply chains (Seyedghorban et al. Citation2020), leveraging the remote data collection, analysis and management capabilities offered by IoT to optimise the transport management structure of logistics, improve customer experience and customer satisfaction, and minimise transport costs (Bhargava et al. Citation2022). Soni et al. (Citation2022) also suggested that IoT technologies can have a significant impact on achieving sustainable supply chain finance for small and medium-sized manufacturing enterprises by improving information processing capabilities.
IIoT technologies play an increasingly important role in industrial management. IIoT have given rise to a new generation of real-time smart factories (Kiel, Arnold, and Voigt Citation2017), more efficient transport conditions (Harris, Wang, and Wang Citation2015), better inventory management (Mathaba et al. Citation2017) and higher levels of customer satisfaction (Jie et al. Citation2015). And how to identify, analyze and address the ensuing risks and challenges is also one of the research priorities.
IoT technologies play a key role in Industry 4.0. IoT systems are one of the key technologies for the successful adoption of Industry 4.0 (Javaid et al. Citation2021). As Industry 4.0 is driven by the need to gain real-time insight and information throughout the manufacturing process, it inherits advanced technologies such as IoT to improve automation and technological systems (Łabędzka Citation2021). At the same time, IoT technologies are the technological backbone of the entire Industry 4.0 agenda because of their ability to increase transparency (Colli et al. Citation2021).
3.4. Network of countries/regions
After screening, the minimum number of documents of a country is set at 3 and the minimum number of citations of a country is set at 6. Of 48 countries, 20 met the threshold. Table depicts the countries/regions analysis of IoT applications in the field of industrial management. In terms of the number of publications, India had the highest with 26 articles, representing 18.31% of the total number of included articles. As such, it reveals India's position at the forefront of theoretical contributions to this field. The USA appeared second with 21 articles, accounting for 14.79% of the total number of included articles. This was followed by China (19 articles), the UK (18 articles), and Germany (13 articles). As a result, developed economies are more prominent than developing economies, with the total number of articles higher in North America and Europe than in Asian and African countries. This indicates that developed economies play an important role in scientific research in the field of IoT applications in industrial management. Concurrently, the average publication year also shows that research on this topic in various countries has been concentrated in the last two years, which leads to the conclusion that this research topic has remained a popular area of study for researchers, and there is an interest in this research topic in all countries/regions.
Table 3. Top 15 countries/regions in IoT applications in industrial management.
3.5. Co-author co-occurrence analysis
According to the articles retrieved from Scopus database, only 11 authors who have published more than two articles were screened and presented in Table . Based on the number of published articles, the University of Erlangen-Nuremberg in Germany is the research institution with the highest publications. Malaysia and India had the next most prolific authors. From the eleven authors, a co-authorship network was created based on their publications. The co-authorship network can be thought of as the structure of an interpersonal social network, where a node represents an author and the links between nodes represent authors’ co-authorship relationships. Each of the 11 authors was analyzed to calculate the total link strength with other authors, where larger circles represent high number of publications by authors, while smaller circles correspond to a smaller number of publications by authors. Among these eleven authors, the author with the highest total link strength is Voigt, K. I. with a link strength of 4. This author also obtained the highest total citations, indicating that Voigt, K. I. has made a relatively high contribution to research in this field. Table shows that the authors collaborated with one another on their respective articles. Although each of the eleven authors had different co-authorship relationships, authors from the same institution were more closely associated. Since only 11 out of 444 authors have published more than two articles, the co-authorship relationship is not particularly evident. After setting the minimum number of authors to 1, it was found that there wasn’t a large collaborative network, with only 12 out of 142 selected articles being single authored. The main reason is due to the wide range of application areas and the interdisciplinary design knowledge, showing lot of contents and data analyzes. Collaboration among authors will not only shorten the research time, but also help authors to share different ideas with one another.
Table 4. Contributing authors in IoT applications in industrial management.
3.6. Author citation network
To conduct the author citation network, the minimum number of articles and the minimum number of citations for an author were set at 1 and 70, respectively. In the end, out of 368 authors, 58 met the threshold. Figure presents the authors’ citation network analysis. As depicted in Figure , Hahn G. J., Fatorachian H., Barbaray R., Frozza R., and Choy K. L. have robust total link strength and strong connections with other authors. Therefore, it may be concluded that the articles published by these authors in this research field are more valuable to serve as a reference guide. Regarding cited authors, the higher the number of citations, the higher the competency of an author is known in the field of study, and the more valuable is the cited article. Table presents the authors citation analysis. As shown in Table , the top 15 most cited authors have all published articles in the last five years from a contemporary perspective. As such, the published articles in this field are more current and the increase in citations in recent years is evident of a growing trend in research in this field. By comparing the average normalised citations, the most cited authors include Wäger M., Warner K. S. R., Xu E. L, Xu L. D., Fatorachian H., and Kazemi H. These authors are more interested in how companies in traditional industries can efficiently use IoT technologies in the context of industrialisation of companies and factories, finding the best solutions to avoid errors and delays.
Figure 4. Network of authors citation analysis.
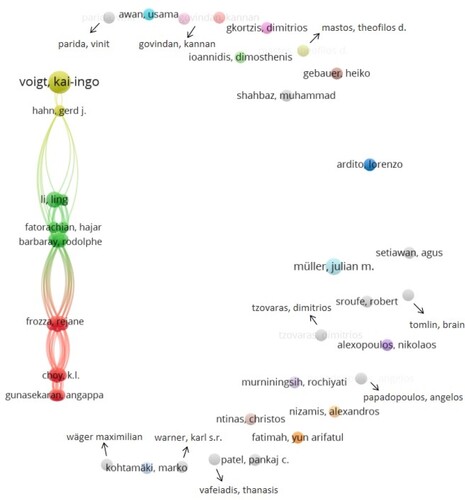
Table 5. Top 15 contributing authors in IoT applications in industrial management.
3.7. Journal citation network
Average citations indicate the citation level of a journal, reflecting its quality and academic value, while the average normalised citations are more comparable. By using VOSviewer, the minimum number of documents and the minimum number of citations of a source were set at 1 and 7, respectively. Out of 74 sources, 18 journal sources met the threshold. Table presents the top 15 journals ranked by their average normalised citations. As shown in Table , Production Planning & Control had the highest average normalised citations, with research articles focusing on operation management in all industries. Included articles published in this journal focus on research related to industrial needs, with a bias towards industrial engineering management, and management science and engineering to assist and guide managers and future researchers. As far as the research areas of the included articles are concerned, the articles related to IoT and industrial management are all associated with supply chain and logistics management. In the second place was the Business Process Management Journal, an academic journal of process management, which focuses on the field of quality management. Figure illustrates the network of journals citation analysis. In conjunction with Figure , the International Journal of Production Research, which had the highest number of articles published in this field (see Table ) also received the highest number of documents, total citations, and average citations (see Table ), and focuses on manufacturing, operations management, and logistics decision making. Their focus is on how to break through and innovate in new business models in the field of IoT and industrial management and how IoT technologies have had an impact on supply chain management, product lifecycle management, warehouse management, equipment management, and process management in the context of Industry 4.0. It also points out to managers which indicators deserve more attention in daily management. In terms of the time dimension, the applications of IoT in industrial management is a relatively new research direction under major journals, apart from the International Journal of RF Technologies.
Figure 5. Network of journals citation analysis.
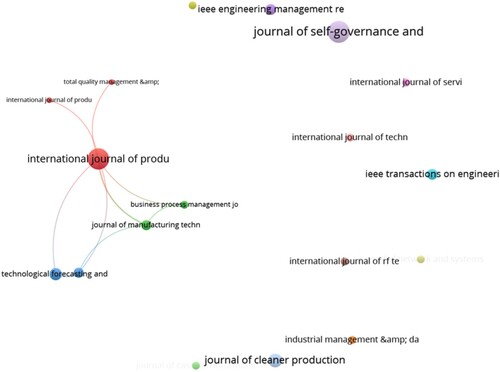
Table 6. Top 15 contributing journals in IoT applications in industrial management.
3.8. Document analysis
The higher the number of citations, the higher the scholarly value of an article/document. After screening, the minimum number of citations for a document was set at 30. Out of 142 documents, 28 met the threshold. Among them, Moeuf et al. (Citation2018), Lee et al. (Citation2018), Hahn (Citation2020), Kipper et al. (Citation2020), Reaidy, Gunasekaran, and Spalanzani (Citation2015), Xu, Xu, and Li (Citation2018), Wang, Ong, and Nee (Citation2018) and Ding et al. (Citation2021) have strong links. Most of these articles focused on the applications of IoT in logistics and supply chain management, generally incorporating logistics and supply chain management into one of the most important application areas of IoT technology. Table illustrates the document analysis of IoT applications in industrial management. As shown in Table , the most cited document was Lee and Lee (Citation2015), probably due to its early publication date and comprehensive introduction of IoT technology, which is extremely informative. However, the normalised citation for this document was the lowest, showing a value of 1.83. The ranking of normalised citations shows that articles focusing on Industry 4.0 and supply chain management are more popular, suggesting that they constitute the hot research topics and trends in this studied domain. The top-ranked article by Warner and Wäger (Citation2019) proposed a process model comprising of nine micro foundations to reveal the generic contingency factors that trigger, enable, and hinder the building of dynamic capabilities for digital transformation. On the other hand, other published articles focused on exploratory innovation strategy (Müller, Buliga, and Voigt Citation2021), cyber-physical systems (Fatorachian and Kazemi Citation2021), cloud computing (Ardito et al. Citation2018), IIoT (Kiel, Arnold, and Voigt Citation2017) and smart manufacturing (Moeuf et al. Citation2018).
Table 7. Top 15 cited documents in IoT applications in industrial management.
4. Discussion
4.1. Mainstream research topics of IoT applications in industrial management
Following the scientometric analysis and scientific mapping of the selected literature samples, this review paper identifies mainstream research topics of IoT applications in industrial management through a qualitative discussion of the existing literature. This was combined with the keyword clusters and led to the following four mainstream research topics.
4.1.1. Application of IoT in manufacturing based on cyber-physical systems
Due to the intricate nature of systems and the fluctuating environment, smart manufacturing companies should have a coherent operating model in which IoT-based management processes can significantly improve production efficiency (Tucker Citation2021). Therefore, smart manufacturing factories based on information-physical systems can be established in conjunction with mobile devices in the processes of product design, time management, quality management, and equipment operation (Wade and Vochozka Citation2021). The main components range from intelligent design and production to visual monitoring of the production process.
In terms of intelligent design and production, the integration of IoT and manufacturing can achieve intelligent product design. On the one hand, the integration of digital information and physical entities can achieve real-time interaction between physical and virtual space (Nota, Peluso, and Lazo Citation2021). On the other hand, the integration of IoT and manufacturing can achieve the intelligence of the products themselves, i.e. the integration of modern information technology into the products, so that the products can achieve more intelligent functions to meet the diversified needs of consumers and shape personalised products (Lee et al. Citation2018). Combining them with machine learning technology can also minimise the time and cost of customised and mass production, helping manufacturing companies to maintain their competitiveness (Rai et al. Citation2021). For example, digital twin technology can build intelligent model structures, or twins, from real-time data (Golovina et al. Citation2020). The developed digital twin can detect possible inefficiencies in products and help companies to upgrade their manufacturing processes. In addition, the Graduation Intelligent Manufacturing System developed by Guo et al. (Citation2020) can reduce the complexity and uncertainty for achieving real-time information sharing and real-time production planning, integrating an effective production and operation management system.
Concurrently, real-time data collection and monitoring of the production process by using IoT technology can make production timelier and more accurate, whilst the dynamics and agility of management can be greatly enhanced. Information about products and equipment can be collected at any time during the product life cycle, maximising the efficiency of implementation (Hamid et al. Citation2022). Examples of collected information include yield information, exception information, etc. The use of IoT technology in a data-driven mode enables an automated collection of full status data throughout the production process for instant application of data and closed-loop control (Wade and Vochozka Citation2021). It enables self-enhancement, self-diagnosis, and predictive maintenance of equipment (Nota, Peluso, and Lazo Citation2021). It also facilitates the quality management of products and troubleshooting of equipment by company managers (Gordon Citation2021). Moreover, it emphasised the importance of maintenance, repair, and operation management (Li et al. Citation2019). Furthermore, it helps to leverage existing data management techniques to manage structured data (Ge et al. Citation2020). The use of IoT technology has enabled the transformation of traditional functional product design to personalised product design and transition from traditional manufacturing to flexible manufacturing and intelligent management.
4.1.2. The contribution of IoT technologies to logistics and supply chain management
For intelligent logistics systems, IoT technology can automatically collect logistics information through sensing technology. With the advancement of mobile internet technology, the collected logistics information can also be transmitted to a database through wireless networks, enhancing real-time data collection and sharing of information (Lee et al. Citation2018). It also provides information to managers to make real-time adjustments which can lead to dynamic control of operations in all aspects of logistics (Popescu et al. Citation2021).
IoT technology enables the construction of intelligent traceability network systems for products. Product traceability systems play a huge role in tracking, identification, enquiry, information collection and management of goods (Oh Citation2019). Bhargava et al. (Citation2022) proposed the concept of IoT-ILTMF (Interconnected Logistics Transportation and Management Framework) for collecting real-time logistics data. Track consumption and inventory status through sensor-based measurement devices were used to optimise supply chain management (Kaur and Kaur Citation2018). In a situation of supply chain disruption risk, real-time logistic data can be used to quickly track down all orders that have exceeded their delivery lead time to identify problematic transports, and then use digital technology to simulate possible solutions (Ivanov, Dolgui, and Sokolov Citation2019). IoT system modelling framework with implementation architecture was proposed by Tu, Lim, and Yang (Citation2018) to optimise the traceability network system by enabling the tracking of products and different parts of the same product along the manufacturing supply chain.
Visualisation network systems are also an integral part of logistics management. With the development of big data, complex systems and intelligent sensors are optimised in terms of information collection (Javaid et al. Citation2021). The visualisation network system of logistics process is based on satellite positioning technology, RFID technology, sensing technology, and other technologies (Fatorachian and Kazemi Citation2021). Especially for heavy-duty vehicles used for transportation, real-time vehicle parameter monitoring is realised in the process of logistics activities and monitoring of all suppliers’ activities (Rane and Narvel Citation2021) to provide the foundation for transport security. At present, most logistics companies or enterprises are equipped with intelligent vehicle mounted IoT systems, which can realise the positioning and real-time monitoring of vehicles, etc., and initially realise the transparent and visual management of logistics operations.
Finally, IoT technologies are also indispensable for the construction of smart logistics centres. Reaidy, Gunasekaran, and Spalanzani (Citation2015) proposed an IoT-based bottom-up warehouse management method by using ambient intelligence and RFID technology to improve the competitiveness of warehouses in dynamic environments. IoT-based smart warehousing will be more efficient, visual, accurate, and secure. These logistics centres use IoT technology to connect objects to objects, connect operators to objects in the warehouse and use embedded environmental sensors to track and monitor connected items (Ding et al. Citation2021). Lee et al. (Citation2018) proposed how to combine warehouse management systems with fuzzy logic techniques to select the most appropriate order picking method, thus improving the efficiency of the order picking process. Specifically, 5G technology can be used for scheduling and routing decisions in tailed supply chain and logistics solutions to meet the diverse needs of customers, as well as for improving companies’ supply chain and logistics efficiency (Dolgui and Ivanov Citation2022). In addition, IoT technology can simplify the storage process and optimise storage resources. Decentralised decision-making can be implemented in central warehouse management systems via IoT technology (Ding et al. Citation2021).
4.1.3. The impact of IoT on business models
IoT in the business world can uncover new insights from data. The adoption of IoT has revolutionised how data is processed and has improved the way businesses use collected data. Warner and Wäger (Citation2019) highlighted the importance of building digital sensing capabilities, suggesting that strategic agility is a key dynamic capability for companies to capture the latest trends and avoid potential existential threats. With the emergence of new business lines, enterprises not only can develop products, but also integrate business intelligence into daily management (Polyvyanyy et al. Citation2017). To improve the competitiveness of companies, IoT enables new paths of innovation in the form of continuous engagement and value-added services (Schroeder et al. Citation2019). Notably, innovative technologies promote absorptive capacity in companies. When seeking new partners, companies must review their business transactions and knowledge acquisition (Müller, Buliga, and Voigt Citation2021). In this context, companies are more concerned with the needs of their customers, the agility of their production systems, the safety and quality of their products and services and after-sales service. Digitalisation and Industry 4.0 technology can have an impact on disruptive profit models, service models, channel models, and organisational models (Ivanov et al. Citation2021; Choi et al. Citation2022). Within companies, managers are more interested in innovative work behaviours (Santoso, Abdinagoro, and Arief Citation2019) and human resource practices (Vereycken et al. Citation2021) that would help employees to adapt to evolving technologies. In this way, a more interoperable, virtualised, modular, flexible, and personalised business can be created. As such, companies must deal with their human resources (Santoso, Abdinagoro, and Arief Citation2019), planning and execution (Hahn Citation2020), operations and processes (Colli et al. Citation2021), systems and mechanisms (Veile et al. Citation2020), organisation and structures (Eryarsoy et al. Citation2022), as well external factors such as markets (Martinez Citation2019) and customers (Asthana and Dwivedi Citation2020). The integration of IoT can improve management capabilities in multiple dimensions, both internally and externally.
4.1.4. IIoT in the context of Industry 4.0
In contrast to IoT, IIoT technologies are industry focused IoT (Bhargava et al. Citation2022). IIoT technologies emphasise on automation, networking, integration and represent a major technological advancement in the application of next-generation information technology. From a business perspective, the emergence of IIoT has had a huge impact on the already established business models of manufacturing companies, particularly through production and process optimisation in customer production systems (Kiel, Arnold, and Voigt Citation2017). Innovations in this business model include converting products into services, using predictive maintenance technology to monitor and analyze the best time for maintenance and providing proactive maintenance and service for customers. In the manufacturing industry, previous studies have provided a detailed analysis of the possibilities for IIoT applications at the shop floor level and within individual manufacturing sites, discussing how IIoT can support the tracking of material flows from the production site downstream to the customer (Deflorin, Scherrer, and Schillo Citation2021). Besides, IIoT can pose several challenges to traditional standardisation due to its complexity, dynamism, and acceleration of technological progress, as such, managers should identify and analyse IIoT's challenges (Eryarsoy et al. Citation2022). Given the challenges of IIoT to traditional standardisation, new solutions and standards are needed for organisational development (Koch and Blind Citation2020). This is a problem that must be addressed by the subsequent development of industry.
4.2. Research gaps of IoT applications in industrial management
IoT technologies play an important role in industrial production and manufacturing, energy conservation and emission reduction, product informatization, operations and management. With the gradual expansion of the application fields of IoT, the problems/challenges of IoT technology in different fields are also increasingly revealed. For example, the challenges of intelligent logistics of IoT include the technical problems of RFID and WSN, the expansion of IoT and the limitation on technical capabilities, the standardisation of IoT, the issue of data collection and processing from IoT, as well as the security and privacy issues of IoT. Figure summarises the main research gaps of IoT applications in industrial management.
Figure 6. Research gaps of IoT in industrial management.
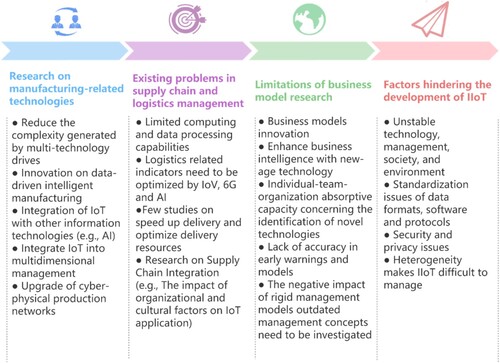
4.2.1. Research on manufacturing-related technologies
In recent years, several technologies have emerged to continuously promote the development of intelligent manufacturing and IoT. The main applications include cyber-physical systems, AI, machine/deep learning algorithms, etc. Sophisticated AI and machine learning algorithms are designed to take advantage of existing technologies to keep machines progressing over longer durations (Gordon Citation2021). One of the key application directions is predictive maintenance. New approaches are needed to reduce the complexity of manufacturing management systems, driven by multiple technologies. Li et al. (Citation2019) showed a management framework that includes online production management, offline maintenance management, and maintenance bill of materials management, which can further integrate multiple technologies to simultaneously implement break-down maintenance management and preventive maintenance management. In practical applications, smart factories can improve sustainability by using AI data-driven IoT systems for real-time monitoring (Tucker Citation2021). In addition, it can strengthen data collection, equipment management, and intelligent analysis of connected equipment to diagnose the operation of manufacturing systems (Kovacova and Lewis Citation2021). Current research has shown that AI-based decision-making methods are helpful for processing data generated during production (Andrei et al. Citation2016). In the future, more fine-grained data models can be built to help managers monitor each stage of a process, providing granular data to support stage optimisation (Peng et al. Citation2021). Further research should consider AI-based decision-making algorithms (Popescu et al. Citation2021), cyber-physical production networks in sustainable smart manufacturing (Lăzăroiu, Kliestik, and Novak Citation2021), and the higher levels of cooperation on manufacturing network coordination mechanisms (Deflorin, Scherrer, and Schillo Citation2021). In addition, innovative research on data-driven intelligent manufacturing needs to be conducted (Wade and Vochozka Citation2021). Integrating IoT technology into multidimensional management resources such as quality management, material management and planning management, can help to establish a multidimensional model of project management and extend it to other areas of the project management knowledge system.
4.2.2. Existing problems in supply chain and logistics management
In supply chain and logistics management, IoT technology makes the transportation process traceable and monitorable, however, compared to the control of the delivery process, few studies have investigated how to speed up delivery and optimise delivery resources through IoT (Ding et al. Citation2021). Meanwhile, in the construction of information systems for logistics centres, IoT technologies have made significant contributions to the fields of warehousing safety, inventory management, and order picking efficiency (Lee et al. Citation2018). Among them, the minimisation of space allocation and travel distance is very important to improve warehouse performance. However, due to the limited computing power and data processing capacity, some problems cannot be solved by IoT at present, including the selection of optimal delivery routes (Tsang et al. Citation2018), optimisation of storage strategy, transportation, truck loading (Vanderroost et al. Citation2017), and RFID technology issues (Ding et al. Citation2021). In addition, satisfying both demand and supply at the cheapest cost is inseparable from the optimisation of transportation routes. With the continuous development of IoT, different industries refine the technology in different fields and applications. For example, the emergence of ‘Internet of Vehicles’ (IoV) technology has reduced the time and cost of logistics to a certain extent and improved safety during transportation (Shen, Fantacci, and Chen Citation2020). By leveraging 6G, performance can be further improved for faster and smoother data exchange (Bhargava et al. Citation2022). Further research could make use of AI technologies to optimise decisions on vehicle cargo matching, vehicle routing, charging and parking, as well as optimising all logistics-related metrics such as emissions and costs (Dong et al. Citation2021). In addition, machine learning algorithms can be used to perform resource allocation simulations to achieve optimal recognition, allocation, and prediction (Nota, Peluso, and Lazo Citation2021). From another perspective, supply chain partners can provide higher value and increased competitive advantage to customers through the integration of supply chains. Hence, future research could also focus on investigating the organisational and cultural factors that influence supply chains (Fatorachian and Kazemi Citation2021). Effective and efficient management can be achieved through the coordinated management of business processes within and between organisations (Dong et al. Citation2021).
4.2.3. Limitations of business model research
IoT can transform not only products, but the entire business model of a company (Tervonen et al. Citation2018). By including innovative and personalised services provided by enterprises, new ways of cooperation and changes in internal management models could be realised (Kiel, Arnold, and Voigt Citation2017). In the context of IoT, Schroeder et al. (Citation2019) proposed that digital service providers can leverage performance and operational insights to create entirely new value propositions. It is also possible to increase the market penetration of IoT technologies by developing new and innovative business models (Dong et al. Citation2021). Yet, little work has been done to implement business intelligence using advanced technologies to improve the agility of the various sub-processes of project procurement management (Rane and Narvel Citation2021). In addition, Müller, Buliga, and Voigt (Citation2021) discussed the impact of absorptive capacity on innovation strategies during technological transition, which affects the redesign of existing business models. However, further research is needed on how individual absorptive capacity affects team-level absorptive capacity, and how the latter affects the identification of new technologies for organisational absorptive capacity. Moreover, the samples selected by existing research faced some limitations. In the process of investigation, it is necessary to reasonably reflect the proposed investigation results in the case of differences in countries, cultures, and organisations. From the perspective of risk management, the ability of IoT to monitor data in real-time is often used in risk management (Kausar Fatima et al. Citation2019) to keep track of risk conditions for preventive rescue. The early warning method based on GA-SVR and ACO-SVR proposed by Wang and Xue (Citation2022) can make effective predictions for resource-based clusters, but the accuracy of the early warning and the accuracy of the model need to be further improved. In addition, not all companies have the organisational and financial capacity to anticipate potential risks (Łabędzka Citation2021). The predictive capabilities of IoT can also be used in future research to establish relationships between available resources and future demands in enterprises and to establish appropriate early warning mechanisms (Awan, Sroufe, and Shahbaz Citation2021). Not only that, many scholars have continued to study the favourable direction of transformation in management models, but in the environment of enterprise management innovation, no studies have been conducted to analyze the negative effects of rigid management models and outdated management concepts.
4.2.4. Factors hindering the development of IIoT
Due to the low timeliness and intelligence of traditional systems, the emergence of IIoT technology has promoted the development of intelligence and efficiency in the industrial field (Hossain and Muhammad Citation2016). Li et al. (Citation2018) pointed out that IIoT technologies are superior to traditional IoT in terms of scalability, flexibility, centralised management, service quality, real-time, and reliability. However, there are still some defects in the application of IIoT, which hinder the wider applications of IIoT. For example, system performance and platforms are not yet stable enough (Gao Citation2021), management uncertainty (Birkel and Hartmann Citation2020), social uncertainty (Shah, Bolton, and Menon Citation2020), and environmental uncertainty (Wang and Wang Citation2017). In addition, the widespread use of IIoT depends on the standardisation of data formats, software, and protocols. Due to the huge barriers to perception, communication, and feedback of information caused by heterogeneity (Razzaque et al. Citation2015), future research on the standardisation process is needed so that multiple formats and technologies can meet the different requirements for machine-to-machine communication between connected devices (Koch and Blind Citation2020), and to develop feasible policies based on norms and standard specifications (Tay, Alipal, and Lee Citation2021). IIoT may pose cybersecurity-related risks to management (Bhattacharya and Chatterjee Citation2021) and may reduce company performance (Birkel and Hartmann Citation2019). IoT data largely depend on secure networks. When numerous IoT devices are connected, the data samples are transmitted through the networks, whether it is the sensor end, the device end, or the application end, there could be risks to information security. For example, the privacy issues caused by increasing the frequency of high-dimensional data have not been solved due to differential privacy protection (Wang et al. Citation2020). As such, these shortcomings might lead to poor utility, trust relationships between physical objects, unauthorised users, and high complexity (Yao et al. Citation2021; Radanliev and De Roure Citation2023). Where AI is deployed in IoT devices, the possibility of data leakage can be effectively reduced (Bagaa et al. Citation2020; Radanliev and De Roure Citation2022). The implications of these issues for management, as well as exploring ways to avoid these risks and finding opportunities to turn them into value creation, will continue to be the subject of our future research.
4.3. Research trends of IoT applications in industrial management
Based on co-occurrence of keywords analysis, qualitative discussion of mainstream research topics and research gaps, Figure shows the research framework for future research directions of IoT applications in industrial management.
Figure 7. Future research directions of IoT in industrial management.
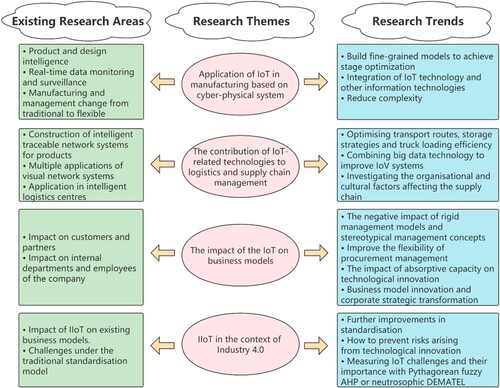
The mainstream research topics and research gaps of IoT applications in industrial management do not exist in isolation but are closely linked with one another. For example, the application of IoT in intelligent manufacturing makes the real-time data collection and monitoring of IoT technologies in the production process more timely and reliably. In addition, the visualisation network system of logistics process is based on satellite positioning technology, RFID technology, sensing technology, and other technologies, which can closely track and monitor suppliers’ activities. Through IoT innovation technology, the relationships between enterprises and suppliers are facilitated, which can affect the business model of enterprises. With the extensive application of IoT, more challenges will occur. For example, IIoT may create multiple challenges to traditional standardisation. In the future, standard-setting organisations will be required to seek new solutions. Therefore, several directions of future research in the corresponding fields can be foreseen, including:
Control of existing risk factors in the process of technological innovation, research, and development of enterprises, so as to reduce the risks in technological innovation.
Integration of IoT technologies with other forms of data analytics and digital technologies (e.g. Al, blockchain, cloud computing, sensing technologies, robotics, etc.).
How to speed up deliveries and optimise transport processes through IoT technology, especially through IoV.
The agility of project procurement management can be further improved and extended to other areas of the project management body of knowledge.
A comparative study of innovative business models in industries and other sectors.
The negative impact of rigid management models and stereotypical management concepts.
Enhancing corporate absorptive capacity through IoT technologies, mainly at the individual and team levels, and integrated with innovation strategies.
The study of the standardisation of IIoT and their analytical methods.
IIoT challenges and their importance can be measured from a hierarchy using algorithms such as Pythagorean fuzzy AHP or neutrosophic DEMATEL (Eryarsoy et al. Citation2022).
4.4. Contributions to theory
This review paper aims to conduct a science mapping review of IoT applications in industrial management and to identify mainstream research topics, research gaps, and future research directions. Although extant studies have analyzed the applications of IoT in industrial management and put forward some meaningful research directions, it is still necessary to propose a theoretical framework for future research directions on the applications of IoT through the results of scientometric analysis and explore its progress in industrial management. As such, the present review study evaluated the development applications of IoT in the studied area since 2012, analyzed the impact of authors, countries, keywords, and documents, discussed the main research topics, and provided a theoretical framework for future research directions of IoT in industrial management. Through our analysis and the study of extant literature, the principles, techniques, and applications of IoT have been integrated into every aspect of industrial production and reproduction. It has freed itself from the limitations of the original traditional production and helped to improve automated management. Along with the in-depth research on production management and IoT technologies in industries, the boundaries between industrial engineering, computer science, information science, and management are becoming increasingly blurred, providing a theoretical basis for the discovery of new research areas and disciplines.
4.5. Contributions to practice and policy
This is the first state-of-the-art review on the applications of IoT in industrial management by adopting a science mapping approach. Therefore, the findings of this study provide an in-depth understanding of the state-of-the-art applications and future needs of IoT research for researchers, policymakers, and practitioners in various industries. For example, the results of influential journals, keywords, authors, and countries would serve as a reference guide for other researchers when searching for literature within the studied area. Also, the identified main research topics would provide a useful reference for practitioners and policymakers to explore the practical applications of IoT in industrial management. Moreover, the future research directions would help other researchers to extend the research developments while contributing to the extant body of knowledge. Lastly, since IoT can be adopted in several industries (e.g. manufacturing, healthcare, construction, etc.), the recommended research directions can be explored by researchers in different fields, thus, promoting the continued application scenarios of IoT technologies.
4.6. Limitations of the study and future research directions
Every study has limitations in its methodology and study design, which affect the conclusions drawn from the study. For this study, there are still some shortcomings worth noting. First, the literature search was conducted using Scopus database. A single database was chosen in this study to avoid the removal of duplicates from multiple databases. Also, it may be difficult to combine several database formats into a single science mapping tool (i.e. VOSviewer). However, it should be noted that the analyzed data cannot cover all relevant research results. Second, at the time of literature screening, this study was limited to articles written in English language and subject areas such as business, management, and accounting, thus, excluding other languages and innovative research in other fields. Third, some discrepancies in the process of data processing with VOSviewer have not been resolved. In future studies, researchers can further use literature from other databases (such as Web of Science, Science Direct), including other languages and subject areas to conduct similar research.
5. Conclusions
This review paper aims to conduct a state-of-the-art review of IoT applications in industrial management, and to identify mainstream research topics, research gaps in the existing literature, and future research directions. By searching for articles between the years from 2012 to 2022 (only before July 2022), 142 documents were retrieved from the Scopus database. Scientometric analysis provides a theoretical basis and a method for innovative research. First, research articles in the studied field have been increasing in the past ten years. With the help of continuous innovation of IoT technology, this upward trend may continue in recent years. Mining influential papers and authors against the reported results would help future researchers to collaborate with other authors, globally. The results of this study indicate that the International Journal of Production Research is the journal with the largest contribution of published articles among other journals. Voigt, K. I. and Wäger M., as highly cited authors, have laid a solid foundation for continued research in this field. From a global perspective, there is an imbalance in publications across different countries/regions. Literature from developed countries are more valuable because these countries have advanced and well-known institutional settings. Finally, according to the cluster analysis of keywords, four main research areas about IoT applications in industrial management were identified, including manufacturing, logistics, business model transformation, and IIoT.
The main conclusion drawn from scientometric techniques is that IoT applications are growing rapidly in various fields of industry, and in recent years researchers have shifted their research focus to 1) application of IoT in manufacturing based on cyber-physical systems 2) IoT-related technologies to logistics and supply chain management 3) IoT on business models 4) application of IIoT in the context of Industry 4.0. In addition, this review study suggests future research directions for researchers in related areas, allowing more scholars to participate in this field. Overall, the findings of this study would serve as a useful reference for researchers and practitioners to explore further research and practical applications of IoT in industrial management.
Acknowledgments
The authors are grateful to the Department of Mechanical, Aerospace and Civil Engineering, University of Manchester, UK for supporting this research. We are also grateful to the Editor and Reviewers for their comments in improving the quality of this paper. This paper forms part of the first author’s research project where similar research backgrounds, but different scopes and/or analyzes may have been published.
Data availability statement
The data that support the findings of this study are available from the corresponding author upon reasonable request.
Disclosure statement
No potential conflict of interest was reported by the author(s).
Additional information
Notes on contributors
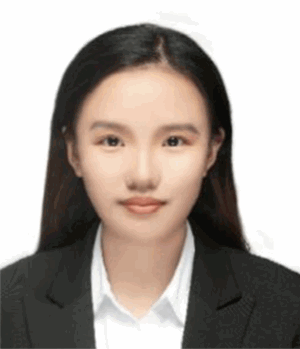
Xiaoshao Mu
Xiaoshao Mu obtained her Master of Science degree in the Department of Mechanical, Aerospace and Civil Engineering, University of Manchester, majoring in Management of Projects. She completed her Bachelor of Science degree in Financial Mathematics from Shanxi University of Finance and Economics, China in 2021. Her research interests include Engineering Management, Supply Chain Management, Industry 4.0 Technologies, Digital Transformation (e.g. Big Data, Internet of Things, Cloud Computing), and Mathematical Modelling (e.g. Securities, Investment, Insurance).
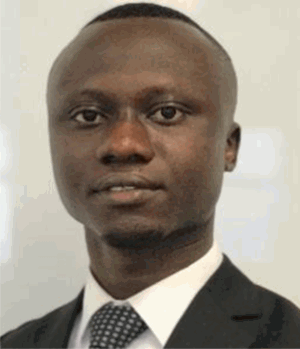
Maxwell Fordjour Antwi-Afari
Dr Maxwell Fordjour Antwi-Afari is currently a Lecturer in Construction & QS at the Department of Civil Engineering, College of Engineering and Physical Sciences, Aston University, Birmingham, B4 7ET, UK. He obtained his Ph.D. in Construction Information Technology from the Department of Building and Real Estate at The Hong Kong Polytechnic University. Maxwell also received his MPhil in Building Technology and worked as a Graduate Assistant at the Department of Building Technology, Kwame Nkrumah University of Science and Technology (KNUST), Kumasi-Ghana in 2015. He attained his Bachelor of Science degree in Construction Technology and Management (First Class Honours) from KNUST, Kumasi-Ghana in 2012. His research interests focus on construction management and engineering, construction health and safety, construction ergonomics, digital technologies and innovations (e.g. building information modelling, wearable sensors, internet-of-things, digital twins, robotics), construction informatics (e.g. machine learning, deep learning), technology transfer in construction and biomechanical analysis.
References
- Abdallah, W., S. Mnasri, and N. Nasri. 2020, September. “Emergent IoT Wireless Technologies Beyond the Year 2020: A Comprehensive Comparative Analysis.” 2020, International Conference on Computing and Information Technology (ICCIT-1441) (pp. 1-5). IEEE. doi:10.1109/ICCIT-144147971.2020.9213799.
- Ahmad, I., T. Kumar, M. Liyanage, J. Okwuibe, M. Ylianttila, and A. Gurtov. 2018. “Overview of 5G Security Challenges and Solutions.” IEEE Communications Standards Magazine 2 (1): 36–43. doi:10.1109/MCOMSTD.2018.1700063.
- Akpan, I. J., D. Soopramanien, and D. H. Kwak. 2021. “Cutting-edge Technologies for Small Business and Innovation in the era of COVID-19 Global Health Pandemic.” Journal of Small Business & Entrepreneurship 33 (6): 607–617. doi:10.1080/08276331.2020.1799294.
- Alghamdi, R., and M. Bellaiche. 2023. “An Ensemble Deep Learning Based IDS for IoT Using Lambda Architecture.” Cybersecurity 6 (1): 5. doi:10.1186/s42400-022-00133-w.
- Ammar, M., G. Russello, and B. Crispo. 2018. “Internet of Things: A Survey on the Security of IoT Frameworks.” Journal of Information Security and Applications 38: 8–27. doi:10.1016/j.jisa.2017.11.002.
- Andrei, F., A. B. Siegling, A. M. Aloe, B. Baldaro, and K. V. Petrides. 2016. “The Incremental Validity of the Trait Emotional Intelligence Questionnaire (TEIQue): A Systematic Review and Meta-Analysis.” Journal of Personality Assessment 98 (3): 261–276. https://doi.org/10.1080/00223891.2015.1084630.
- Antwi-Afari, M. F., H. Li, A. H. S. Chan, J. Seo, S. Anwer, H. Y. Mi, Z. Wu, and A. Y. L. Wong. 2023. “A Science Mapping-Based Review of Work-Related Musculoskeletal Disorders among Construction Workers.” Journal of Safety Research ahead-of-print (ahead-of-print), doi:10.1016/j.jsr.2023.01.011.
- Ardito, L., A. M. Petruzzelli, U. Panniello, and A. C. Garavelli. 2018. “Towards Industry 4.0.” Business Process Management Journal 25 (2): 323–346. doi:10.1108/BPMJ-04-2017-0088.
- Asthana, S., and A. Dwivedi. 2020. “Performance Measurement of India-Based Third Party Logistics Sector: An Empirical Study of User Versus Provider Perspectives.” Production Planning & Control 31 (2-3): 259–272. doi:10.1080/09537287.2019.1631467.
- Awan, U., R. Sroufe, and M. Shahbaz. 2021. “Industry 4.0 and the Circular Economy: A Literature Review and Recommendations for Future Research.” Business Strategy and the Environment 30 (4): 2038–2060. doi:10.1002/bse.2731.
- Bagaa, M., T. Taleb, J. B. Bernabe, and A. Skarmeta. 2020. “A Machine Learning Security Framework for IoT Systems.” IEEE Access 8: 114066–114077. doi:10.1109/ACCESS.2020.2996214.
- Bhargava, A., D. Bhargava, P. N. Kumar, G. S. Sajja, and S. Ray. 2022. “Industrial IoT and AI Implementation in Vehicular Logistics and Supply Chain Management for Vehicle Mediated Transportation Systems.” International Journal of System Assurance Engineering and Management 13 (1): 673–680. doi:10.1007/s13198-021-01581-2.
- Bhattacharya, S., and A. Chatterjee. 2021. “Digital Project Driven Supply Chains: A new Paradigm.” Supply Chain Management: An International Journal 27 (2): 283–294. doi:10.1108/SCM-12-2020-0641.
- Birkel, H. S., and E. Hartmann. 2019. “Impact of IoT Challenges and Risks for SCM.” Supply Chain Management: An International Journal 24 (1): 39–61. doi:10.1108/SCM-03-2018-0142.
- Birkel, H. S., and E. Hartmann. 2020. “Internet of Things – the Future of Managing Supply Chain Risks.” Supply Chain Management: An International Journal 25 (5): 535–548. doi:10.1108/SCM-09-2019-0356.
- Bradley, T., P. Thibodeau, and V. Ng. 2014. “The Internet of Things Threats and Challenges.” Network World Asia 11 (1): 16–18.
- Brites, I. S., L. M. Silva, J. L. Barbosa, S. J. Rigo, S. D. Correia, and V. R. Leithardt. 2022. “Lecture Notes in Networks and Systems.” In International Conference on Information Technology & Systems 414: 356–388. doi:10.1007/978-3-030-96293-7_32.
- Brous, P., M. Janssen, and P. Herder. 2020. “The Dual Effects of the Internet of Things (IoT): A Systematic Review of the Benefits and Risks of IoT Adoption by Organizations.” International Journal of Information Management 51: 101952. doi:10.1016/j.ijinfomgt.2019.05.008.
- Browne, M. 2021. “Artificial intelligence data-driven internet of things systems, real-time process monitoring, and sustainable industrial value creation in smart networked factories.” International Journal of Information Management 51 (2): 101952–101931. doi:10.1016/j.ijinfomgt.2019.05.008.
- Butschan, J., S. Heidenreich, B. Weber, and T. Kraemer. 2019. “Tackling Hurdles to Digital Transformation-The Role of Competencies for Successful Industrial Internet of Things (IIoT) Implementation.” International Journal of Innovation Management 23 (4): 1950036. doi:10.1142/S1363919619500361.
- Carcary, M., G. Maccani, E. Doherty, and G. Conway. 2018. “Exploring the Determinants of IoT Adoption: Findings from a Systematic Literature Review.” In International Conference on Business Informatics Research 330: 113–125. doi:10.1007/978-3-319-99951-7_8.
- Caron, X., R. Bosua, S. B. Maynard, and A. Ahmad. 2016. “The Internet of Things (IoT) and its Impact on Individual Privacy: An Australian Perspective.” Computer law & Security Review 32 (1): 4–15. doi:10.1016/j.clsr.2015.12.001.
- Casner, S. M., R. W. Geven, M. P. Recker, and J. W. Schooler. 2014. “The Retention of Manual Flying Skills in the Automated Cockpit.” Human Factors: The Journal of the Human Factors and Ergonomics Society 56 (8): 1506–1516. doi:10.1177/0018720814535628.
- Chadegani, A. A., H. Salehi, M. M. Yunus, H. Farhadi, M. Fooladi, M. Farhadi, and N. A. Ebrahim. 2013. “A Comparison Between two Main Academic Literature Collections: Web of Science and Scopus Databases,” Vol. 9, No. 5, pp.18-26. doi:10.5539/ass.v9n5p18.
- Choi, T. M., A. Dolgui, D. Ivanov, and E. Pesch. 2022. “OR and Analytics for Digital, Resilient, and Sustainable Manufacturing 4.0.” Annals of Operations Research 310 (1): 1–6. doi:10.1007/s10479-022-04536-3.
- Colares, G. S., N. Dell'Osbel, P. G. Wiesel, G. A. Oliveira, P. H. Z. Lemos, F. P. da Silva, C. A. Lutterbeck, L. T. Kist, and ÊL Machado. 2020. “Floating Treatment Wetlands: A Review and Bibliometric Analysis.” Science of the Total Environment 714: 136776. doi:10.1016/j.scitotenv.2020.136776.
- Colli, M., J. N. Uhrenholt, O. Madsen, and B. V. Waehrens. 2021. “Translating Transparency Into Value: An Approach to Design IoT Solutions.” Journal of Manufacturing Technology Management 32 (8): 1515–1532. doi:10.1108/JMTM-06-2020-0225.
- Cui, L., M. Gao, J. Dai, and J. Mou. 2020. “Improving Supply Chain Collaboration Through Operational Excellence Approaches: An IoT Perspective.” Industrial Management & Data Systems 122 (3): 565–591. doi:10.1108/IMDS-01-2020-0016.
- Deflorin, P., M. Scherrer, and K. Schillo. 2021. “The Influence of IIoT on Manufacturing Network Coordination.” Journal of Manufacturing Technology Management 32 (6): 1144–1166. doi:10.1108/JMTM-09-2019-0346.
- Ding, Y., M. Jin, S. Li, and D. Feng. 2021. “Smart Logistics Based on the Internet of Things Technology: An Overview.” International Journal of Logistics Research and Applications 24 (4): 323–345. doi:10.1080/13675567.2020.1757053.
- Dolgui, A., and D. Ivanov. 2022. “5G in Digital Supply Chain and Operations Management: Fostering Flexibility, end-to-end Connectivity and Real-Time Visibility Through Internet-of-Everything.” International Journal of Production Research 60 (2): 442–451. doi:10.1080/00207543.2021.2002969.
- Dong, C., A. Akram, D. Andersson, P. O. Arnäs, and G. Stefansson. 2021. “The Impact of Emerging and Disruptive Technologies on Freight Transportation in the Digital era: Current State and Future Trends.” The International Journal of Logistics Management 32 (2): 386–412. doi:10.1108/IJLM-01-2020-0043.
- Eck, N. J. V., and L. Waltman. 2014. “Visualizing Bibliometric Networks.” In Measuring Scholarly Impact, 285–320. doi:10.1007/978-3-319-10377-8_13.
- Eryarsoy, E., H. S. Kilic, S. Zaim, and M. Doszhanova. 2022. “Assessing IoT Challenges in Supply Chain: A Comparative Study Before and During- COVID-19 Using Interval Valued Neutrosophic Analytical Hierarchy Process.” Journal of Business Research 147: 108–123. doi:10.1016/j.jbusres.2022.03.036.
- Fang, C., X. Liu, J. Pei, W. Fan, and P. M. Pardalos. 2016. “Optimal Production Planning in a Hybrid Manufacturing and Recovering System Based on the Internet of Things with Closed Loop Supply Chains.” Operational Research 16 (3): 543–577. doi:10.1007/s12351-015-0213-x.
- Fatimah, Y. A., K. Govindan, R. Murniningsih, and A. Setiawan. 2020. “Industry 4.0 Based Sustainable Circular Economy Approach for Smart Waste Management System to Achieve Sustainable Development Goals: A Case Study of Indonesia.” Journal of Cleaner Production 269: 122263. doi:10.1016/j.jclepro.2020.122263.
- Fatorachian, H., and H. Kazemi. 2021. “Impact of Industry 4.0 on Supply Chain Performance.” Production Planning & Control 32 (1): 63–81. doi:10.1080/09537287.2020.1712487.
- Ferretti, M., and F. Schiavone. 2016. “Internet of Things and Business Processes Redesign in Seaports: The Case of Hamburg.” Business Process Management Journal 22 (2): 271–284. doi:10.1108/BPMJ-05-2015-0079.
- Fireteanu, V. V. 2020, June. “Agile Methodology Advantages When Delivering Internet of Things Projects.” In 2020: 12t. h International Conference on Electronics, Computers and Artificial Intelligence (ECAI), pp. 1-5. IEEE. doi:10.1109/ECAI50035.2020.9223172.
- Gao, Y. 2021. “Using Artificial Intelligence Approach to Design the Product Creative on 6G Industrial Internet of Things.” International Journal of System Assurance Engineering and Management 12 (4): 696–704. doi:10.1007/s13198-021-01065-3.
- Gartner. 2021. “Infographic: Patent Growth Insights,” 5G Technology, 2021. Available at: https://www.gartner.com/en/documents/4008201 (Accessed 11 May 2022).
- Ge, J., F. Wang, H. Sun, L. Fu, and M. Sun. 2020. “Research on the Maturity of big Data Management Capability of Intelligent Manufacturing Enterprise.” Systems Research and Behavioral Science 37 (4): 646–662. doi:10.1002/sres.2707.
- Ghosh, A., D. J. Edwards, and M. R. Hosseini. 2020. “Patterns and Trends in Internet of Things (IoT) Research: Future Applications in the Construction Industry.” Engineering, Construction and Architectural Management 28 (2): 457–481. doi:10.1108/ECAM-04-2020-0271.
- Ghosh, S., M. Hughes, I. Hodgkinson, and P. Hughes. 2022. “Digital Transformation of Industrial Businesses: A Dynamic Capability Approach.” Technovation 113: 102414. doi:10.1016/j.technovation.2021.102414.
- Golovina, T., A. Polyanin, A. Adamenko, E. Khegay, and V. Schepinin. 2020. “Digital Twins as a new Paradigm of an Industrial Enterprise.” International Journal of Technology 11 (6): 1115–1124. doi:10.14716/ijtech.v11i6.4427.
- Gordon, A. 2021. “Internet of Things-Based Real-Time Production Logistics, big Data-Driven Decision-Making Processes, and Industrial Artificial Intelligence in Sustainable Cyber-Physical Manufacturing Systems.” Journal of Self-Governance and Management Economics 9 (3): 61–73. doi:10.22381/jsme9320215.
- Guo, D., M. Li, R. Zhong, and G. Q. Huang. 2020. “Graduation Intelligent Manufacturing System (GiMS): An Industry 4.0 Paradigm for Production and Operations Management.” Industrial Management & Data Systems 121 (1): 86–98. doi:10.1108/IMDS-08-2020-0489.
- Guo, B., D. Zhang, Z. Wang, Z. Yu, and X. Zhou. 2013. “Opportunistic IoT: Exploring the Harmonious Interaction Between Human and the Internet of Things.” Journal of Network and Computer Applications 36 (6): 1531–1539. doi:10.1016/j.jnca.2012.12.028.
- Gupta, N. 2021. “Trends in IoT research: A bibliometric and science mapping analysis of internet of things.” Library Philosophy and Practice (e-journal), 6096. https://digitalcommons.unl.edu/libphilprac/6096.
- Hahn, G. J. 2020. “Industry 4.0: A Supply Chain Innovation Perspective.” International Journal of Production Research 58 (5): 1425–1441. doi:10.1080/00207543.2019.1641642.
- Hamid, Abd, M. S. R. Masrom, N. R. and Mazlan, and N. A. B. 2022. “Supply Chain Digitalization and Operational Performance.” International Journal of Asian Business and Information Management 13 (2): 1–16. doi:10.4018/IJABIM.298000.
- Harris, I., Y. Wang, and H. Wang. 2015. “ICT in Multimodal Transport and Technological Trends: Unleashing Potential for the Future.” International Journal of Production Economics 159: 88–103. doi:10.1016/j.ijpe.2014.09.005.
- Hipsher, T. A., and V. G. Duffy. 2021, July. “Integration of Internet of Things Devices in Manufacturing Workspaces: A Systematic Literature Review.” In International Conference on Human-Computer Interaction, Vol. 13097, LNCS, pp. 476-493. Springer, Cham. doi:10.1007/978-3-030-90966-6_33.
- Hossain, M. S., and G. Muhammad. 2016. “Cloud-assisted Industrial Internet of Things (IIoT) – Enabled Framework for Health Monitoring.” Computer Networks 101: 192–202. doi:10.1016/j.comnet.2016.01.009.
- Hosseini, M. R., I. Martek, E. K. Zavadskas, A. A. Aibinu, M. Arashpour, and N. Chileshe. 2018. “Critical Evaluation of off-Site Construction Research: A Scientometric Analysis.” Automation in Construction 87: 235–247. doi:10.1016/j.autcon.2017.12.002.
- Ivanov, D., A. Dolgui, and B. Sokolov. 2019. “The Impact of Digital Technology and Industry 4.0 on the Ripple Effect and Supply Chain Risk Analytics.” International Journal of Production Research 57 (3): 829–846. doi:10.1080/00207543.2018.1488086.
- Ivanov, D., C. S. Tang, A. Dolgui, D. Battini, and A. Das. 2021. “Researchers’ Perspectives on Industry 4.0: Multi-Disciplinary Analysis and Opportunities for Operations Management.” International Journal of Production Research 59 (7): 2055–2078. doi:10.1080/00207543.2020.1798035.
- Javaid, M., A. Haleem, R. P. Singh, and R. Suman. 2021. “Significant Applications of big Data in Industry 4.0.” Journal of Industrial Integration and Management 06 (4): 429–447. doi:10.1142/S2424862221500135.
- Jie, Y. U., N. Subramanian, K. Ning, and D. Edwards. 2015. “Product Delivery Service Provider Selection and Customer Satisfaction in the era of Internet of Things: A Chinese e-Retailers’ Perspective.” International Journal of Production Economics 159: 104–116. doi:10.1016/j.ijpe.2014.09.031.
- Katoch, R. 2022. “IoT Research in Supply Chain Management and Logistics: A Bibliometric Analysis Using Vosviewer Software.” Materials Today: Proceedings 56: 2505–2515. doi:10.1016/j.matpr.2021.08.272.
- Kaur, J., and P. D. Kaur. 2018. “CE-GMS: A Cloud IoT-Enabled Grocery Management System.” Electronic Commerce Research and Applications 28: 63–72. doi:10.1016/j.elerap.2018.01.005.
- Kausar Fatima, S., S. Gauhar Fatima, S. Abdul Sattar, and D. K. A. Sheela. 2019. “A Monitoring Scheme with IoT and Sensor Expertise.” International Journal of Advanced Research in Engineering and Technology 10 (2): 271–277. doi:10.34218/IJARET.10.2.2019.027.
- Kiel, D., C. Arnold, and K. I. Voigt. 2017. “The Influence of the Industrial Internet of Things on Business Models of Established Manufacturing Companies – A Business Level Perspective.” Technovation 68: 4–19. doi:10.1016/j.technovation.2017.09.003.
- Kipper, L. M., L. B. Furstenau, D. Hoppe, R. Frozza, and S. Iepsen. 2020. “Scopus Scientific Mapping Production in Industry 4.0 (2011–2018): A Bibliometric Analysis.” International Journal of Production Research 58 (6): 1605–1627. https://doi.org/10.1080/00207543.2019.1671625.
- Koch, C., and K. Blind. 2020. “Towards Agile Standardization: Testbeds in Support of Standardization for the IIoT.” IEEE Transactions on Engineering Management 68 (1): 59–74. doi:10.1109/TEM.2020.2979697.
- Kohtamäki, M., V. Parida, P. C. Patel, and H. Gebauer. 2020. “The Relationship Between Digitalization and Servitization: The Role of Servitization in Capturing the Financial Potential of Digitalization.” Technological Forecasting and Social Change 151: 119804. doi:10.1016/j.techfore.2019.119804.
- Kovacova, M., and E. Lewis. 2021. “Smart Factory Performance, Cognitive Automation, and Industrial big Data Analytics in Sustainable Manufacturing Internet of Things.” Journal of Self-Governance and Management Economics 9 (3): 9–11. doi:10.22381/jsme9320211.
- Łabędzka, J. 2021. “Industry 4.0 - Policy-Based Approaches to Efficient Implementation in SMEs.” Engineering Management in Production and Services 13 (4): 72–78. doi:10.2478/emj-2021-0032.
- Lăzăroiu, G., T. Kliestik, and A. Novak. 2021. “Internet of Things Smart Devices, Industrial Artificial Intelligence, and Real-Time Sensor Networks in Sustainable Cyber-Physical Production Systems.” Journal of Self-Governance and Management Economics 6 (1): 20–30. doi:10.22381/jsme9120212.
- Lee, I., and K. Lee. 2015. “The Internet of Things (IoT): Applications, Investments, and Challenges for Enterprises.” Business Horizons 58 (4): 431–440. doi:10.1016/j.bushor.2015.03.008.
- Lee, C. K., Y. Lv, K. K. H. Ng, W. Ho, and K. L. Choy. 2018. “Design and Application of Internet of Things-Based Warehouse Management System for Smart Logistics.” International Journal of Production Research 56 (8): 2753–2768. doi:10.1080/00207543.2017.1394592.
- Li, X., D. Li, J. Wan, C. Liu, and M. Imran. 2018. “Adaptive Transmission Optimization in SDN-Based Industrial Internet of Things with Edge Computing.” IEEE Internet of Things Journal 5 (3): 1351–1360. doi:10.1109/JIOT.2018.2797187.
- Li, L., M. Liu, W. Shen, and G. Cheng. 2019. “A Novel Performance Evaluation Model for MRO Management Indicators of High-end Equipment.” International Journal of Production Research 57 (21): 6740–6757. doi:10.1080/00207543.2019.1566654.
- Lin, C. C., C. H. Yang, and J. Z. Shyua. 2013. “A Comparison of Innovation Policy in the Smart Grid Industry Across the Pacific: China and the USA.” Energy Policy 57: 119–132. https://doi.org/10.1016/j.enpol.2012.12.028.
- Malik, A. 2022. “A Social Relationship-Based Energy Efficient Routing Scheme for Opportunistic Internet of Things.” ICT Express, doi:10.1016/j.icte.2022.10.002.
- Malik, K. R., Y. Sam, M. Hussain, and A. Abuarqoub. 2018. “A Methodology for Real-Time Data Sustainability in Smart City: Towards Inferencing and Analytics for big-Data.” Sustainable Cities and Society 39: 548–556. doi:10.1016/j.scs.2017.11.031.
- Martinez, F. 2019. “Process Excellence the key for Digitalisation.” Business Process Management Journal 25 (7): 1716–1733. doi:10.1108/BPMJ-08-2018-0237.
- Mathaba, S., M. Adigun, J. Oladosu, and O. Oki. 2017. “On the use of the Internet of Things and Web 2.0 in Inventory Management.” Journal of Intelligent & Fuzzy Systems 32 (4): 3091–3101. doi:10.3233/JIFS-169252.
- Meho, L. I., and Y. Rogers. 2008. “Citation counting, citation ranking, and h-index of human-computer interaction researchers: a comparison of Scopus and Web of Science.” Journal of the American Society for Information Science and Technology 59 (11): 1711–1726. doi:10.1002/asi.20874.
- Mehta, G., G. Mittra, and V. K. Yadav. 2018, September. “Application of IoT to Optimize Data Center Operations.” In 2018, International Conference on Computing, Power and Communication Technologies (GUCON) (pp. 738-742). IEEE. doi:10.1109/GUCON.2018.8674939.
- Mejia, C., M. Wu, Y. Zhang, and Y. Kajikawa. 2021. “Exploring Topics in Bibliometric Research Through Citation Networks and Semantic Analysis.” Frontiers in Research Metrics and Analytics 6: 742311. doi:10.3389/frma.2021.742311.
- Meng, Z., Z. Wu, and J. Gray. 2018. “RFID-based Object-Centric Data Management Framework for Smart Manufacturing Applications.” IEEE Internet of Things Journal 6 (2): 2706–2716. doi:10.1109/JIOT.2018.2873426.
- Mital, M., V. Chang, P. Choudhary, A. Papa, and A. K. Pani. 2018. “Adoption of Internet of Things in India: A Test of Competing Models Using a Structured Equation Modeling Approach.” Technological Forecasting and Social Change 136: 339–346. doi:10.1016/j.techfore.2017.03.001.
- Moeuf, A., R. Pellerin, S. Lamouri, S. Tamayo-Giraldo, and R. Barbaray. 2018. “The Industrial Management of SMEs in the era of Industry 4.0.” International Journal of Production Research 56 (3): 1118–1136. doi:10.1080/00207543.2017.1372647.
- Moosavi, J., L. M. Naeni, A. M. Fathollahi-Fard, and U. Fiore. 2021. “Blockchain in Supply Chain Management: A Review, Bibliometric, and Network Analysis.” Environmental Science and Pollution Research, 1–15. doi:10.1007/s11356-021-13094-3.
- Mugarza, I., J. L. Flores, and J. L. Montero. 2020. “Security Issues and Software Updates Management in the Industrial Internet of Things (Iiot) era.” Sensors 20 (24): 7160. doi:10.3390/s20247160.
- Müller, J. M., O. Buliga, and K. I. Voigt. 2021. “The Role of Absorptive Capacity and Innovation Strategy in the Design of Industry 4.0 Business Models - A Comparison Between SMEs and Large Enterprises.” European Management Journal 39 (3): 333–343. doi:10.1016/j.emj.2020.01.002.
- Nota, G., D. Peluso, and A. T. Lazo. 2021. “The Contribution of Industry 4.0 Technologies to Facility Management.” International Journal of Engineering Business Management 13: 184797902110241. doi:10.1177/18479790211024131.
- O’Dwyer, E., I. Pan, R. Charlesworth, S. Butler, and N. Shah. 2020. “Integration of an Energy Management Tool and Digital Twin for Coordination and Control of Multi-Vector Smart Energy Systems.” Sustainable Cities and Society 62: 102412. doi:10.1016/j.scs.2020.102412.
- Oh, A. S. 2019. “Designing smart supplier chain management model under big data and internet of things environment.” International Journal of Recent Technology and Engineering 8 (2): 290–294. doi:10.35940/ijrte.B1055.0782S619.
- Okkonen, P., J. Hyysalo, and E. Peltonen. 2020, April. “Public and Open Internet of Things for Smart Cities: The SME Perspective.” In 2020, IEEE 36th International Conference on Data Engineering Workshops (ICDEW), pp. 48-55. IEEE. doi:10.1109/ICDEW49219.2020.000-8.
- Olawumi, T. O., D. W. Chan, and J. K. Wong. 2017. “Evolution in the Intellectual Structure of BIM Research: A Bibliometric Analysis.” Journal of Civil Engineering and Management 23 (8): 1060–1081. doi:10.3846/13923730.2017.1374301.
- Olsen, T. L., and B. Tomlin. 2020. “Industry 4.0: Opportunities and Challenges for Operations Management.” Manufacturing & Service Operations Management 22 (1): 113–122. https://doi.org/10.1287/msom.2019.0796.
- Peng, C., T. Peng, Y. Liu, M. Geissdoerfer, S. Evans, and R. Tang. 2021. “Industrial Internet of Things Enabled Supply-Side Energy Modelling for Refined Energy Management in Aluminium Extrusions Manufacturing.” Journal of Cleaner Production 301: 126882. doi:10.1016/j.jclepro.2021.126882.
- Polyvyanyy, A., C. Ouyang, A. Barros, and W. M. van der Aalst. 2017. “Process Querying: Enabling Business Intelligence Through Query-Based Process Analytics.” Decision Support Systems 100: 41–56. doi:10.1016/j.dss.2017.04.011.
- Popescu, G. H., S. Petreanu, B. Alexandru, and H. Corpodean. 2021. “Internet of Things-Based Real-Time Production Logistics, Cyber-Physical Process Monitoring Systems, and Industrial Artificial Intelligence in Sustainable Smart Manufacturing.” Journal of Self-Governance & Management Economics 9 (2): 52–62. doi:10.22381/jsme9220215.
- Pritchard, A. 1969. “Statistical Bibliography or Bibliometrics.” Journal of Documentation 25 (4): 348–349.
- Radanliev, P., and D. De Roure. 2022. “Advancing the Cybersecurity of the Healthcare System with Self-Optimising and Self-Adaptative Artificial Intelligence (Part 2).” Health and Technology 12 (5): 923–929.
- Radanliev, P., and D. De Roure. 2023. “New and Emerging Forms of Data and Technologies: Literature and Bibliometric Review.” Multimedia Tools and Applications 82 (2): 2887–2911.
- Radanliev, P., D. De Roure, R. Nicolescu, M. Huth, and O. Santos. 2021. “Artificial Intelligence and the Internet of Things in Industry 4.0.” CCF Transactions on Pervasive Computing and Interaction 3: 329–338. doi:10.1007/s42486-021-00057-3.
- Radhakrishnan, S., S. Erbis, J. A. Isaacs, and S. Kamarthi. 2017. “Novel Keyword co-Occurrence Network-Based Methods to Foster Systematic Reviews of Scientific Literature.” PloS one 12 (3): e0172778. doi:10.1371/journal.pone.0172778.
- Rai, R., M. K. Tiwari, D. Ivanov, and A. Dolgui. 2021. “Machine Learning in Manufacturing and Industry 4.0 Applications.” International Journal of Production Research 59 (16): 4773–4778. doi:10.1080/00207543.2021.1956675.
- Rane, S. B., and Y. A. Narvel. 2021. “Leveraging the Industry 4.0 Technologies for Improving Agility of Project Procurement Management Processes.” International Journal of System Assurance Engineering and Management 12 (6): 1146–1172. doi:10.1007/s13198-021-01331-4.
- Razzaque, M. A., M. Milojevic-Jevric, A. Palade, and S. Clarke. 2015. “Middleware for Internet of Things: A Survey.” IEEE Internet of Things Journal 3 (1): 70–95. doi:10.1109/JIOT.2015.2498900.
- Reaidy, P. J., A. Gunasekaran, and A. Spalanzani. 2015. “Bottom-up Approach Based on Internet of Things for Order Fulfillment in a Collaborative Warehousing Environment.” International Journal of Production Economics 159: 29–40. doi:10.1016/j.ijpe.2014.02.017.
- Rose, M. E., and J. R. Kitchin. 2019. “Pybliometrics: Scriptable Bibliometrics Using a Python Interface to Scopus.” SoftwareX 10: 100263. doi:10.1016/j.softx.2019.100263.
- Santoso, H., S. B. Abdinagoro, and M. Arief. 2019. “The Role of Digital Literacy in Supporting Performance Through Innovative Work Behavior: The Case of Indonesia’s Telecommunications Industry.” International Journal of Technology 10 (8): 1558–1566. doi:10.14716/ijtech.v10i8.3432.
- Saunders, M., P. H. Lewis, and A. D. Thornhill. 2007. “Research Methods.” Business Students, 4th edition Pearson Education Limited, England 6 (3): 1–268.
- Schroeder, A., A. Ziaee Bigdeli, C. Galera Zarco, and T. Baines. 2019. “Capturing the Benefits of Industry 4.0: A Business Network Perspective.” Production Planning & Control 30 (16): 1305–1321. doi:10.1080/09537287.2019.1612111.
- Seyedghorban, Z., H. Tahernejad, R. Meriton, and G. Graham. 2020. “Supply Chain Digitalization: Past, Present and Future.” Production Planning & Control 31 (2-3): 96–114. doi:10.1080/09537287.2019.1631461.
- Shah, S., M. Bolton, and S. Menon. 2020, January. “A Study of Internet of Things (IoT) and its Impacts on Global Supply Chains.” In 2020, International Conference on Computation, Automation and Knowledge Management (ICCAKM), pp. 245-250. IEEE. doi:10.1109/ICCAKM46823.2020.9051474.
- Sharma, D. K., R. S. K. Boddu, N. K. Bhasin, S. S. Nisha, V. Jain, and M. K. Mohiddin. 2021, October. “Cloud Computing in Medicine: Current Trends and Possibilities.” In 2021, International Conference on Advancements in Electrical, Electronics, Communication, Computing and Automation (ICAECA), pp. 1-5. IEEE. doi:10.1109/ICCAKM46823.2020.9051474.
- Shen, X., R. Fantacci, and S. Chen. 2020. “Internet of Vehicles [Scanning the Issue].” Proceedings of the IEEE 108 (2): 242–245. doi:10.1109/JPROC.2020.2964107.
- Skarmeta, A. F., J. L. Hernandez-Ramos, and M. V. Moreno. 2014, March. “A Decentralized Approach for Security and Privacy Challenges in the Internet of Things.” In 2014, IEEE world forum on Internet of Things (WF-IoT), pp. 67-72. IEEE. doi:10.1109/WF-IoT.2014.6803122.
- Soni, G., S. Kumar, R. V. Mahto, S. K. Mangla, M. L. Mittal, and W. M. Lim. 2022. “A Decision-Making Framework for Industry 4.0 Technology Implementation: The Case of FinTech and Sustainable Supply Chain Finance for SMEs.” Technological Forecasting and Social Change 180: 121686. doi:10.1016/j.techfore.2022.121686.
- Stephan, E. G., T. O. Elsethagen, A. S. Wynne, C. Sivaraman, M. C. Macduff, L. K. Berg, and W. J. Shaw. 2013, October. “A Linked Fusion of Things, Services, and Data to Support a Collaborative Data Management Facility.” In 9th IEEE International Conference on Collaborative Computing: Networking, Applications and Worksharing. pp. 579-584. IEEE. doi:10.4108/icst.collaboratecom.2013.254158.
- Storey, A. 2014. “There's Nothing ‘Smart’ About Insecure Connected Devices.” Network Security 2014 (7): 9–12. doi:10.1016/S1353-4858(14)70069-8.
- Tan, L., and N. Wang. 2010, August. “Future Internet: The Internet of Things.” In 2010: 3rd international conference on advanced computer theory and engineering (ICACTE), Vol. 5, pp. V5376-V5380. IEEE. doi:10.1109/ICACTE.2010.5579543.
- Tay, S. I., J. Alipal, and T. C. Lee. 2021. “Industry 4.0: Current Practice and Challenges in Malaysian Manufacturing Firms.” Technology in Society 67: 101749. doi:10.1016/j.techsoc.2021.101749.
- Tervonen, J. K., J. Hautamäki, M. Heikkilä, and V. Isoherranen. 2018. “Survey of Business Excellence by Knowledge Gathering for Industrial Internet-of-Things Applications.” International Journal of Management and Enterprise Development 17 (4): 388–410. doi:10.1504/IJMED.2018.096256.
- Tsang, Y. P., K. L. Choy, C. H. Wu, G. T. S. Ho, H. Y. Lam, and V. Tang. 2018. “An Intelligent Model for Assuring Food Quality in Managing a Multi-Temperature Food Distribution Centre.” Food Control 90: 81–97. doi:10.1016/j.foodcont.2018.02.030.
- Tu, M., M. K. Lim, and M. F. Yang. 2018. “IoT-based production logistics and supply chain system–Part 1: Modeling IoT-based manufacturing supply chain.” Industrial Management & Data Systems 118 (1): 65–95. doi:10.1108/IMDS-11-2016-0503.
- Tucker, G. 2021. “Sustainable Product Lifecycle Management, Industrial big Data, and Internet of Things Sensing Networks in Cyber-Physical System-Based Smart Factories.” Journal of Self-Governance and Management Economics 6 (1): 9–19. doi:10.22381/jsme9120211.
- Valmohammadi, C. 2016. “Examining the Perception of Iranian Organizations on Internet of Things Solutions and Applications.” Industrial and Commercial Training 48 (2): 104–108. doi:10.1108/ICT-07-2015-0045.
- Vanderroost, M., P. Ragaert, J. Verwaeren, B. De Meulenaer, B. De Baets, and F. Devlieghere. 2017. “The Digitization of a Food Package’s Life Cycle: Existing and Emerging Computer Systems in the Logistics and Post-Logistics Phase.” Computers in Industry 87: 15–30. doi:10.1016/j.compind.2017.01.004.
- Van Eck, N., and L. Waltman. 2010. “Software Survey: VOSviewer, a Computer Program for Bibliometric Mapping.” Scientometrics 84 (2): 523–538. doi:10.1007/s11192-009-0146-3.
- Veile, J. W., M. C. Schmidt, J. M. Müller, and K. I. Voigt. 2020. “Relationship Follows Technology! How Industry 4.0 Reshapes Future Buyer-Supplier Relationships.” Journal of Manufacturing Technology Management 32 (6): 1245–1266. doi:10.1108/JMTM-09-2019-0318.
- Vereycken, Y., M. Ramioul, S. Desiere, and M. Bal. 2021. “Human Resource Practices Accompanying Industry 4.0 in European Manufacturing Industry.” Journal of Manufacturing Technology Management 32 (5): 1016–1036. doi:10.1108/JMTM-08-2020-0331.
- Wade, K., and M. Vochozka. 2021. “Artificial Intelligence Data-Driven Internet of Things Systems, Sustainable Industry 4.0 Wireless Networks, and Digitized Mass Production in Cyber-Physical Smart Manufacturing.” Journal of Self-Governance and Management Economics 9 (3): 48–60. doi:10.22381/jsme9320214.
- Wang, X., S. K. Ong, and A. Y. Nee. 2018. “A Comprehensive Survey of Ubiquitous Manufacturing Research.” International Journal of Production Research 56 (1-2): 604–628. doi:10.1080/00207543.2017.1413259.
- Wang, X. V., and L. Wang. 2017. “A Cloud-Based Production System for Information and Service Integration: An Internet of Things Case Study on Waste Electronics.” Enterprise Information Systems 11 (7): 952–968. doi:10.1080/17517575.2016.1215539.
- Wang, Y., and W. Xue. 2022. “Sustainable Development Early Warning and Financing Risk Management of Resource-Based Industrial Clusters Using Optimization Algorithms.” Journal of Enterprise Information Management 34 (4-5): 1374–11391. doi:10.1108/JEIM-03-2021-0152.
- Wang, R., Y. Zhu, C. C. Chang, and Q. Peng. 2020. “Privacy-preserving High-Dimensional Data Publishing for Classification.” Computers & Security 93: 101785. doi:10.1016/j.cose.2020.101785.
- Warner, K. S., and M. Wäger. 2019. “Building Dynamic Capabilities for Digital Transformation: An Ongoing Process of Strategic Renewal.” Long Range Planning 52 (3): 326–349. doi:10.1016/j.lrp.2018.12.001.
- Weinberg, B. D., G. R. Milne, Y. G. Andonova, and F. M. Hajjat. 2015. “Internet of Things: Convenience vs. Privacy and Secrecy.” Business Horizons 58 (6): 615–624. doi:10.1016/j.bushor.2015.06.005.
- Wu, Z., K. Deng, C. Chen, H. Li, M. F. Antwi-Afari, and Y. Wang. 2022. “Status quo and Future Trends of BIM-Based Coordination Research: A Critical Review.” Journal of Civil Engineering and Management 28 (6): 469–484. doi:10.3846/jcem.2022.16928.
- Xu, L. D., E. L. Xu, and L. Li. 2018. “Industry 4.0: State of the art and Future Trends.” International Journal of Production Research 56 (8): 2941–2962. doi:10.1080/00207543.2018.1444806.
- Yang, T., X. Yi, S. Lu, K. H. Johansson, and T. Chai. 2021. “Intelligent Manufacturing for the Process Industry Driven by Industrial Artificial Intelligence.” Engineering 7 (9): 1224–1230. doi:10.1016/j.eng.2021.04.023.
- Yao, X., F. Farha, R. Li, I. Psychoula, L. Chen, and H. Ning. 2021. “Security and Privacy Issues of Physical Objects in the IoT: Challenges and Opportunities.” Digital Communications and Networks 7 (3): 373–384. doi:10.1016/j.dcan.2020.09.001.
- Ystgaard, K. F., L. Atzori, D. Palma, P. E. Heegaard, L. E. Bertheussen, M. R. Jensen, and K. De Moor. 2023. “Review of the Theory, Principles, and Design Requirements of Human-Centric Internet of Things (IoT).” Journal of Ambient Intelligence and Humanized Computing 14: 2827–2859. doi:10.1007/s12652-023-04539-3.
- Zhang, H., X. Zhang, Z. Guo, H. Wang, D. Cui, and Q. Wen. 2021. “Secure and Efficiently Searchable IoT Communication Data Management Model: Using Blockchain as a new Tool.” IEEE Internet of Things Journal, doi:10.1109/JIOT.2021.3121482.
- Zhong, B., H. Wu, H. Li, S. Sepasgozar, H. Luo, and L. He. 2019. “A Scientometric Analysis and Critical Review of Construction Related Ontology Research.” Automation in Construction 101: 17–31. doi:10.1016/j.autcon.2018.12.013.
- Zhou, J., P. Li, Y. Zhou, B. Wang, J. Zang, and L. Meng. 2018. “Toward new-Generation Intelligent Manufacturing.” Engineering 4 (1): 11–20. doi:10.1016/j.eng.2018.01.002.