ABSTRACT
Automatic determination of manufacturing process sequences for the physical production of given part designs is key to facilitate on-demand cyber manufacturing. In this work, we propose an integrated framework that (i) identifies manufacturing features from 3D part designs using a Graph Neural Network (GNN), (ii) identifies the manufacturing processes necessary to produce all features in the part using a Convolutional Neural Network (CNN) that considers shape, material properties, and quality information, and (iii) outputs an ordered manufacturing sequence that can produce the designed part with the help of sequence mining. Using these methods, the knowledge required to enable automated manufacturing process selection is easily scalable and updatable without requiring manual population of ad-hoc or rule-based descriptions. We present exemplar implementations of the proposed framework by suggesting manufacturing sequences for discrete parts with multiple features. The suggested manufacturing sequences demonstrate the potential of the proposed framework for use in future on-demand cyber manufacturing applications.
SUBJECT CLASSIFICATION CODES:
Data availability statement
The data that support the findings of this study are openly available in the GitHub repository (Zhao Citation2023) at https://github.com/czhao33/Manufacturing-Process-Selection-Using-Knowledge-Learned-from-Design-and-Manufacturing-Data.git.
Disclosure statement
No potential conflict of interest was reported by the author(s).
Additional information
Funding
Notes on contributors
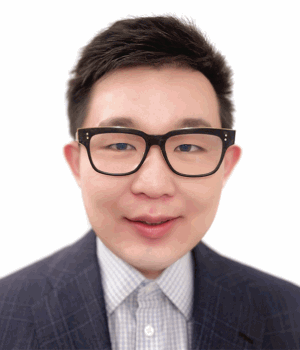
Changxuan Zhao
Changxuan Zhao is a Ph.D. candidate in the George W. Woodruff School of Mechanical Engineering at Georgia Institute of Technology. His current research area focuses on learning manufacturing process capabilities using machine learning methods. He received his MSc and BSc in Mechanical Engineering at Georgia Institute of Technology. His previous research project focused on real-time monitoring of stereolithography processes.
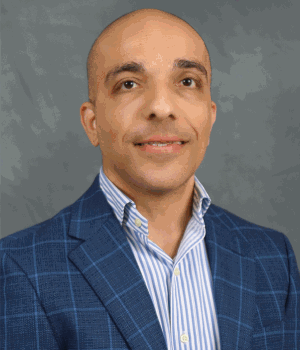
Mahmoud Dinar
Mahmoud Dinar is an assistant professor of mechanical engineer at the University of North Carolina at Charlotte. His research primarily involves implementing a variety of computational frameworks and representations to understand and aid engineering design and manufacturing. Examples from the past include integrating computing apps into Product Data Management tools for an Engineer-to-Order application, and creating an ontology of design for additive manufacturing to support manufacturability analysis of design features. He currently works on using machine learning to glean knowledge of manufacturing process capability in realising designs to support Cyber Manufacturing as a service.
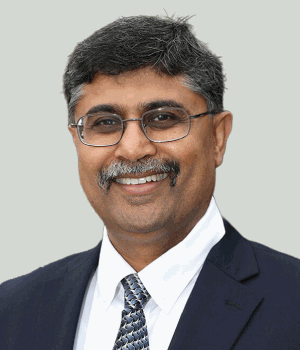
Shreyes N. Melkote
Shreyes N. Melkote is a Morris M. Bryan Jr. Professor in the George W. Woodruff School of Mechanical Engineering at Georgia Institute of Technology. He also serves as Executive Director of the Novelis Innovation Hub at Georgia Tech and as Associate Director of the Georgia Tech Manufacturing Institute. His current research interests are in modelling and simulation of manufacturing processes, application of AI/ML methods to solve complex decision-making problems in manufacturing, and industrial robotics for manufacturing. He is a Fellow of ASME, SME, and CIRP.