Abstract
ChatGPT and generative artificial intelligence (Gen-AI) are transforming firms and supply chains. However, the empirical literature reporting the benefits, challenges, and outlook of these nascent technologies in operations and supply chain management (OSCM) is limited. This study surveys current projects and perceptions of these technologies in US (n = 119) and UK (n = 181) supply chains. We found that projects range from proof-of-concept to full implementation, with a main focus on operational gains, such as improved customer satisfaction, cost minimisation, and process efficiencies. The main challenges concern data, technological and organisational issues. Expected benefits are dominated by cost savings and enhanced customer experience, but also include increased automation and sustainability. Industries were found to cluster around six groups according to perceived benefits and implementation challenges. Our findings contribute to the emerging literature on Gen-AI use in OSCM, and to management practice by mapping the benefits, challenges, outlook, and maturity level of Gen-AI projects in supply chains.
1. Introduction
Artificial intelligence (AI) has a long tradition in production, operations and supply chain management (OSCM) research (Elliott and Griffiths Citation1990; Vujosevic Citation1994) and has recently become a key topic following significant advances in computational power (Dey et al. Citation2023; Jauhar et al. Citation2023; Kusiak Citation2020; Merhi and Harfouche Citation2023; Wong et al. Citation2022). AI tools are already used in various OSCM activities, including demand forecasting, inventory management, risk management (Pournader et al. Citation2021), predictive maintenance, quality control, process optimisation, task scheduling (Rai et al. Citation2021), sales, maintenance, and operations (Helo and Hao Citation2022). Indeed, AI has been shown to create competitive advantages (Helo and Hao Citation2022; Pournader et al. Citation2021; Sharma et al. Citation2022), and improve decision-making (Pournader et al. Citation2021). In the future, AI integration could enable ‘autonomous’ supply chains, with the ability to be self-aware, self-governing, self-determining, and self-optimising (Helo and Hao Citation2022).
The recent exploits of ChatGPT have brought generative artificial intelligence (Gen-AI) to the fore (Peres et al. Citation2023). The media have marvelled at its capacity to replicate and surpass human intelligence, notably demonstrated by its ability to pass graduate-level examsFootnote1, such as the United States Medical Licensing Exam (Tiffany et al. Citation2022), the Uniform Bar Exam (Katz et al. Citation2023), and an MBA level Operations Management exam (Terwiesch Citation2023). Scholars have greeted its abilities with both excitement and apprehension (Jo Citation2023). The emergence of ‘conversational’ generative AI technologies such as ChatGPT allows for easier and more complex interaction with users (Rožanec et al. Citation2023) and promises faster and more widespread adoption compared to traditional, programming based techniques. Today, Gen-AI is predicted to add trillions of dollars in value annually to the global economy (Chui et al. Citation2023), and the global market size for Gen-AI technologies and services is projected to reach $126.5 billion by 2031 (Allied Market Research Citation2023).
While much has been written about the promises of Gen-AI and its ability to mimic human decision-making, the intentions, obstacles, and challenges of its adoption and use in OSCM have received little scholarly attention (e.g. Badini et al. Citation2023; Fosso Wamba et al. Citation2023; Hendriksen Citation2023; Peres et al. Citation2023; Wang, Liu, and Shen Citation2023). And yet, the practitioner literature has continually highlighted the transformative potential of Gen-AI (Deighton Citation2023), with one promising area being operations and supply chain management (Dolgui and Ivanov Citation2023; Telling Citation2023; Triantafyllidis and Kuepper Citation2023; Zagorin Citation2023). Industry reports have described the business value of these technologies, as well as the required capabilities, associated risks (Deloitte Citation2023; EY Citation2023), scalability challenges (PWC Citation2023), ethical concerns, technology governance and sustainability issues (EY Citation2023). Chui et al. (Citation2023) even heralded 2023 as the ‘breakout year’ for Gen-AI.
Further scholarly research is urgently required to better understand the adoption and diffusion of Gen-AI technologies across industries in OSCM. This study is an initial effort in this direction by exploring how companies are currently using Gen-AI, the benefits and challenges it presents, and the future envisioned for these technologies. More explicitly, this study intends to answer the following research questions:
How are companies using Gen-AI in OSCM?
What challenges do companies face implementing Gen-AI in OSCM?
What are the future expected benefits of using Gen-AI in OSCM?
How does adoption of Gen-AI in OSCM differ between industries?
The paper is organised as follows. The following section provides a literature review of Gen-AI and develops several propositions to guide our research. The research methods used in data collection and analysis are then discussed in section three. The results are presented in section four, followed by a discussion in section five. Section six concludes the paper.
2. Literature review
2.1. Gen-AI and OSCM
Gen-AI refers to algorithms that can generate new content such as images, videos, documents, and audio based on the data they were trained on (Dwivedi et al. Citation2023; Peres et al. Citation2023). The main difference between Gen-AI and traditional AI is that the former can ‘generate novel content, rather than simply analysing and acting on existing data’ (Gozalo-Brizuela and Garrido-Merchan Citation2023). Also, traditional AI requires explicit programming to execute tasks, whereas Gen-AI may generate content based on human-machine ‘conversations’. Nonetheless, Gen-AI is still built on AI technologies such as neural network and deep learning algorithms. For example, ChatGPT uses large language models (LLM) based on a large number of parameters to train and learn about relations between data and make predictions about what content should be generated. Consequently, Gen-AI requires access to vast collections of data that are mainly sourced from the internet (Huang, Wang, and Yang Citation2023; Webb, Holyoak, and Lu Citation2023).
Gen-AI enabled OSCM could lead to a paradigm shift in the field (Jackson, Saenz, and Ivanov Citation2023) and provide several distinct advantages such as improved sourcing assessment, supply chain risk mitigation, procurement and inventory accuracy, logistics coordination, and customer relations, and more sustainable and ethically conscious OSCM practices (Richey et al. Citation2023). These technologies also hold promise for enhanced decision-making processes, faster real-time responses to customer queries, and improved customer behaviour forecasting (Precedence Research Citation2023).
2.2. Adoption and use of AI and Gen-AI
The high operational and strategic potential of Gen-AI has led some practitioners and scholars to go so far as to suggest that Gen-AI is the ‘next big thing for business transformation’ (Gascoigne Citation2023). However, past IT innovation studies tell us that the acceptance of any technological innovation is mainly driven by organisational perceptions of business value based on an assessment of the benefits and challenges of adoption (e.g. Keating et al. Citation2010).
A large number of studies have examined the different uses of AI technologies (Wamba et al. Citation2021), the benefits that accrue from them (Merhi and Harfouche Citation2023), and the challenges encountered during adoption (Kamoonpuri and Sengar Citation2023). AI is also seen as an integral part of Industry 4.0 and beyond with manifold opportunities within digital transformations (Ivanov et al. Citation2021). However, empirical studies on the uses, benefits and challenges of Gen-AI in OSCM are lacking (Fosso Wamba et al. Citation2023). Table illustrates some of the possible benefits and challenges of Gen-AI based on publicly available business use cases.
Table 1. Illustrations of Gen-AI projects and outcomes.
Following an exploratory descriptive research design (Bickman, Rog, and Hedrick Citation2009; Punch Citation2013), we draw on diffusion of innovation theory (Rogers Citation1983) inter alia, to formulate a set of research propositions to guide our research.
2.2.1. Diffusion of innovation theory: a theoretical prism
Diffusion of innovation theory provides a theoretical prism to explore and describe the nascent use of Gen-AI in organisations. Past IT innovation studies tell us that the acceptance of any technological innovation is mainly driven by organisational perceptions of business value based on an assessment of the specific benefits and challenges related to its adoption (Keating et al. Citation2010; Mintzberg, Raisinghani, and Théorêt Citation1976).
The IT innovation literature has notably examined the reasons behind organisational innovation, its facilitators and inhibitors, and the methods used to foster it (Fichman Citation2000; Fosso Wamba and Chatfield Citation2009; Keating et al. Citation2010). This literature can be classified into two main streams: (a) adoption studies that focus on differences in organisational innovativeness by examining, for example, the characteristics of an organisation as well as the timing and the extent of innovation adoption; (b) diffusion studies that examine the ‘rate, pattern, and extent of technology diffusion’ (Fichman Citation2000, 110) across a given population.
Multiple factors have been found to influence the diffusion of any given innovation, including technological characteristics (e.g. compatibility, complexity, relative advantage, trialability, observability (Rogers Citation1983)), organisational characteristics (e.g. organisational readiness (Lee and Shim Citation2007), organisational culture (Fichman Citation2000), and top management support (Lu et al. Citation2021)), and environmental characteristics (e.g, competitive pressure (El-Haddadeh et al. Citation2021), standards (Fichman Citation2000), government support (Lu et al. Citation2021), and regulations (El-Haddadeh et al. Citation2021)).
Rogers (Citation1983) identified five adopter categories. Innovators (2.5% of adopters) are always interested in new ideas and the first to adopt an innovation. They play ‘an important role in the diffusion process: that of launching the new idea in the system by importing the innovation from outside of the system’s boundaries’ (p.248). Early adopters (13.5%) represent the second set of adopters after the innovators and are quick to adopt an innovation. They are usually the ones that help trigger the critical mass when they adopt an innovation. The early majority (34%) adopt just before an average member of the population, but they typically take a while to fully embrace the innovation. The late majority (34%) consistently adopt innovations with caution and skepticism, often only after the average member of a system has done so. Laggards (16%) are usually the last in a population to adopt an innovation as they tend to be very suspicious of innovations.
2.2.2. Research propositions
We formulate four research propositions based on the extant scholarly literature, practitioner and industry publications, and the theoretical prism of diffusion of innovation theory.
The first proposition concerns initial projects and benefits. Compared to prior IT-enabled OSCM, Gen-AI is expected to improve productivity and efficiency (Fosso Wamba et al. Citation2023). The technology notably has the potential to solve the productivity paradox as discussed for example in Brynjolfsson (Citation1993) and Brynjolfsson, Rock, and Syverson (Citation2018). For example, it could allow for a more reliable and efficient interface between suppliers, shippers, truckers, and warehouses (Mizell-Pleasant Citation2023). Brynjolfsson, Li, and Raymond (Citation2023) also report that Gen-AI increases operational productivity and positive customer sentiment by transforming the way novice and low-skilled workers learn.
The first wave of Gen-AI implementation in the supply chain is expected to focus on task automation to more efficiently execute repetitive tasks at operational and managerial levels (Gravier Citation2023; Wiles Citation2023). These benefits have been largely described in the practioner literature. For example, Young (Citation2023) reported an increase in Gen-AI solutions by logistics companies, essentially in customer support processes. Likewise, Zagorin (Citation2023) highlighted the potential process efficiencies and cost savings from the adoption of Gen-AI in procurement activities. Lamba (Citation2023) and Telling (Citation2023) reported the use of Gen-AI to more efficiently manage supply chains and minimise disruption risks. We, therefore, propose that:
P1. Initial projects and benefits in OSCM will focus on task automation, efficiencies, and improved customer service.
P2. Current challenges to Gen-AI implementation in OSCM will concern AI models, technologies, and data access.
P3. Expected benefits from future Gen-AI projects in OSCM will include gains accruing from business model transformations.
P4. The rate of adoption of Gen-AI in OSCM will vary by industry.
3. Research method
To examine our research propositions we used an exploratory descriptive approach which focuses on revealing patterns in data rather than on testing theory based hypotheses (Helfat Citation2007; Oxley, Rivkin, and Ryall Citation2010). This approach is appropriate when an area is relatively new or unexplored (Punch Citation2013) and when little or no theory is available to fully explain the phenomenon (Hambrick Citation2007; Ven Citation2016; Ven et al. Citation2015). Exploratory descriptive research seeks to make causal propositions by exploring and describing links between variables that contribute to building a foundation for understanding relationships before formally testing them through explanatory research. Exploratory descriptive research designs have been successfully employed in OSCM research to study issues such as the use of visual management tools (Jaca et al. Citation2014), manufacturing strategy (Dangayach and Deshmukh Citation2001), lean production (Jasti and Kodali Citation2015) and the adoption of lean six sigma (Samarrokhi, Jenab, and Weinsier Citation2015).
An exploratory design is ‘indispensable in the early stages of studying a phenomenon’ (Malhotra and Grover Citation1998) and this is notably the case for new and highly mediatised technologies. The limited practical and theoretical understanding of Gen-AI (Susarla et al. Citation2023) motivated our methodological approach. Furthermore, several calls have been made in the literature for more empirical observations and descriptions of AI adoption and use (Dwivedi et al. Citation2023; Richey et al. Citation2023).
The exploratory descriptive research process used in the study is presented in Figure .
Figure 1. Descriptive research workflow.
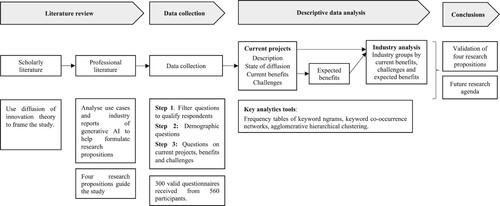
3.1. Data collection and analysis
A questionnaire survey was used to study the benefits and challenges of Gen-AI for OSCM. The instrument consisted of twelve questions and is reproduced in Appendix 1. A market research service provider managed data collection.Footnote2 Of the 560 individuals that agreed to participate in the study, 300 returned valid questionnaires (response rate of 54%). Table presents the characteristics of the sample.
Table 2. Demographic and professional profile of the sample.
The typical respondent was male, between 26 and 49 years old, and held a university-level qualification. On average, they had worked between two to ten years at their company, occupied a middle management position and worked in the area of strategy, operations, human resources, or marketing. The most observed industries were information and communication, professional, scientific, and technical activities, financial and insurance activities, and human health and social work activities.
4. Results
Data analysis involved studying the responses to open questions concerning current Gen-AI projects, and perceived benefits and challenges. We used bi-gram and co-word network analysis of keywords, and hierarchical cluster analysis of industry groups.
4.1. Current Gen-AI projects in OSCM
Respondents were asked to describe their current use of Gen-AI for OSCM. The responses were cleaned and analysed using the R programming language, particularly the packages dplyr, tidyr, and tidytext. Bi-grams were then computed to identify the most frequently used combinations of words. A bi-gram is a string of two adjacent words used in the same sentence by a respondent. Two word strings were considered appropriate to capture compound nouns (e.g. demand forecasting) and verb-noun collections (e.g. reduce costs) that respondents may use to describe their current projects. The 50 most frequent bi-grams were then manually inspected and cleaned, and similar bi-grams were grouped together. The ten most frequently observed applications of Gen-AI are reported in Table .
Table 3. Current project description bi-grams.
Current Gen-AI projects mainly concerned supply chain and operational issues of inventory management, demand forecasting, resource planning, process efficiencies, and time and cost savings. For example, a manager from the UK construction industry described the following project in inventory optimisation:
ChatGPT can be trained on historical inventory data to generate recommendations for optimising inventory levels. By analyzing past sales data and predicting future demand, the model can suggest optimal stocking levels for different products at different times.
We will be using ChatGPT to interact with our customers. If our customers have an issue or concern, they can interact with ChatGPT to find a solution.
First ranked projects varied by industry, with some privileging customer relations (e.g. accommodation and food service, financial and insurance, and public administration and defence), operational efficiencies and savings (e.g. agriculture, forestry and fishing, human health and social work, and real estate), or business intelligence and forecasting (e.g. arts, entertainment and recreation, information and communication, and wholesale and retail trade).
Table 4. Top three current projects by industry.
4.1.1. The current state of Gen-AI adoption
Respondents were then asked to identify the stage of development of their Gen-AI projects. The results are reported in Figure .
Figure 2. Current projects by development stage.
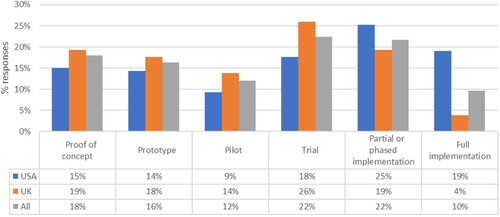
One-third of projects were in an experimental proof-of-concept or prototype phase, one-third were being trialled or tested in a pilot phase, and one-third of projects had already been partially or fully implemented. Table presents the most frequently reported projects and the main industries by project stage.
Table 5. Current project by stage and industry.
The main projects in the experimental phase (i.e. proof-of-concept, prototype) were demand forecasting, inventory management, and process automation. The accommodation and food service activities industry reported the highest proportion of projects in this early phase of Gen-AI adoption.
The most frequently reported projects in the test phase (i.e. pilot or trial) concerned quality control, inventory management, and customer service. Companies in the administrative and support service activities industry reported most projects in this phase.
An IT director at a UK educational institution described the trialled use of ChatGPT for customer service:
We are currently in trials with a government backed project to test and to see how effective the systems are in place. We are constantly monitoring these systems to evaluate how effective they are both for us and our customers. We are doing this to move forward into a new and exciting future within our industry.
A purchasing manager at a water supply, sewerage and waste management concern reported using ChatGPT in the tendering process:
AI being used mainly in inventory management to improve planning and scheduling. ChatGPT is used operationally to support the tendering process, generating tender detail, contracts, etc., using historical data.
We can compare these rates of Gen-AI deployment to adopter categories from diffusion of innovation theory. Companies that have fully implemented Gen-AI correspond to Rogers (Citation1983) innovators category (10% of companies). The early adopters include those companies that have partially implemented Gen-AI projects (22%), while the other 68% of companies make up the early majority.
4.2. The benefits and obstacles of Gen-AI in OSCM
Respondents were asked to identify five current benefits, challenges, and expected future benefits of Gen-AI technologies. Once again, bi-grams were generated and analysed, and are reported in the following subsections.
4.2.1. The current benefits of Gen-AI
The ten most cited benefits of Gen-AI in OSCM are presented in Table .
Table 6. Top ten current benefits.
The first ranked benefit from Gen-AI adoption was improved customer satisfaction. For example, an IT specialist from the US described how Gen-AI can lead to an enhanced customer experience:
ChatGPT can provide customers with quick and accurate responses to their queries, leading to improved customer satisfaction and loyalty.
ChatGPT can identify cost-saving opportunities by analyzing supplier performance, identifying areas of inefficiency in the supply chain, and automating procurement processes. These cost savings can translate into higher profitability for the business.
Gen-AI was also reported to enhance decision-making and process management through a more accurate understanding of patterns, trends, and real-time insights. For example, a manager from a US marketing services company described the decision-making benefits from Gen-AI:
Increased market agility and responsiveness, allowing marketing companies to adjust to new trends and customer preferences quickly.
Co-word analysis involves identifying the co-occurrences of keywords within a corpus and representing them in the form of a network. Each node is a keyword, and a node's size reflects its frequency within the corpus. Links indicate co-occurrences of keywords and the thickness of a link reflects the number of co-occurrences within the corpus. The distance between two keywords in the network indicates the relatedness regarding co-occurrence links. In general, the closer two nodes are to each other, the stronger their relatedness. Related nodes are grouped into coloured clusters.
The results are presented in Figure . Four main themes emerged: productivity, speed and cost savings (yellow, orange, and green clusters); accuracyFootnote3 and automation (blue clusters); demand management and customer service (red and brown clusters); and communication (purple cluster).
This network representation shows that productivity, accuracy, cost, and speed benefits are often related, as are supply chain operations, optimisation, inventory management, demand forecasting, and customer service. Such relationships were often illustrated in project descriptions. For example, a senior vice president from the US wholesale, retail and repair industry reported that:
Gen-AI will be trained on historical sales data to forecast demand for different product lines and sizes accurately. This can help optimise inventory levels, minimise stockouts, and reduce overstocking.
4.2.2. The expected benefits of Gen-AI in OSCM
The ten most cited expected benefits from the use of Gen-AI in OSCM are presented in Table .
Table 7. Top ten future benefits.
Several expected benefits were also reported as current benefits by managers, including customer satisfaction, cost savings, improved efficiency, inventory management, and decision-making. However, two new benefits were expected from the use of Gen-AI in the future, notably increased automation, and improved sustainability. These two benefits were both highly cited and ranked in 4th and 5th position respectively. Also, while access to real-time information was cited as a current benefit (8th rank), it was expected to be a future benefit by an even greater number of managers (3rd rank).
Co-word analysis was then performed using VOSViewer to map connections between all expected benefits. The results are presented in Figure .
Two major and opposing themes can be observed from the co-occurrence map of expected future benefits: productivity improvements (including time, speed, staff, and reduction), and sustainability (including agility, collaboration, safety, and risk). Three smaller themes in the network are cost savings, innovation, and supply chain operations (including real-time insights, prediction models, and scenarios).
Not surprisingly, the theme of sustainability is transversal, and is related to keywords of operations, reductions, abilities, and communication. The following pilot project described by a senior developer from the professional, scientific, and technical activities industry illustrates the sustainability benefits of Gen-AI:
For us ChatGPT and Gen-AI review material and product records to provide a summary used for logistics in the warehouse to avoid bottlenecks. We recently implemented this, and it allows us to analyze the data from various sources along the supply chain to minimise downtime and identify patterns for areas of improvement. It also allows us to track our sustainability in a way that we had not been able to before we implemented this. Another way that we use it in our supply chain management is to reduce our waste.
4.2.3. The challenges of Gen-AI in OSCM
Managers were asked to describe five current challenges related to the adoption and use of Gen-AI in OSCM. The results are reported in Table .
Table 8. Top ten challenges.
The main challenges concerned issues related to data (i.e. data quality, data privacy, data security) and the Gen-AI technologies themselves (i.e. model complexity, choice and use of 3rd party platforms). For example, a manager from the agriculture, forestry and fishing industry lamented the difficulty of obtaining reliable volumes of supply chain data:
Gen-AI models require large amounts of high-quality data to learn from. However, supply chain data can be fragmented, inconsistent, and difficult to obtain, which can limit the accuracy and effectiveness of Gen-AI models.
Retraining IT to support something that is not fully understood. Retraining staff to use the ChatGPT solution.
The co-occurrence of keywords was then studied using VOSViewer to detect relationships between challenges. The results are presented in Figure .
Two main and opposing themes emerged from the keyword network: employee adoption and use, data quality, biases and ethical considerations. These are consistent with the challenges previously identified in Table .
4.3. Industry clusters by Gen-AI benefits and challenges
Industries were clustered into similar groups according to reported current and future benefits and implementation challenges. The thirty most frequent bi-grams were first extracted and ranked for all nineteen industries. Each bi-gram was then coded using an open coding procedure, resulting in a topology of nine current benefits, eight challenges and eleven future benefits. Industries were then clustered using an agglomerative hierarchical clustering algorithm for categorical variables.Footnote4 Six clusters were identified based on the resulting dendrogram (reproduced in Appendix 2).
The industries by cluster are presented in Table . The number of respondents per cluster in the original dataset is shown in brackets. Each cluster has been named to facilitate analysis.
Table 9. Industries by cluster.
The first cluster (‘services’) was the largest and included nine service and retail industries. The second cluster (‘building & support’) comprised three secondary sector industries. The administrative and support services industry also included companies that provide services to the construction and manufacturing industries (e.g. rental and leasing, security, logistics). The third cluster (‘land use & other’) was also comprised of three industries, two of which specifically concern land-based resource use. One industry was in each of the fourth (‘arts’) and sixth (‘real estate’) clusters. The fifth cluster (‘extraction’) included industries that extract, process and sell fossil fuels and minerals.
Industry clusters differed according to the importance of four current benefits (i.e. automation, cost savings, customer satisfaction, and decision support), two challenges (i.e. data quality and avalilability, legacy systems)Footnote5, and two future benefits (i.e. business development, and efficiency, optimisation and productivity). The key benefits and challenges by cluster are presented in Table .
Table 10. Benefits and challenges by cluster.
There were no significant differences between clusters in demographic (i.e. gender, age, educational level) and professional variables (i.e. area of decision-making responsibility, number of years working in the organisation, management level).Footnote6
While benefits and challenges varied between clusters, there were similarities: most clusters identified efficiency, optimisation, productivity and cost savings as current benefits from using Gen-AI. Industries in clusters one and six also frequently cited improved customer satisfaction, industries in clusters three and four cited automation benefits, and industries in clusters one and two reported enhanced decision support.
Challenges relating to staff resistance, accuracy, biases and errors, and required training and skills were also cited by most clusters. Customer satisfaction was mentioned by industries in most clusters as an important, if not the main expected future benefit from using Gen-AI. Most clusters also expected to make efficiency, optimisation and productivity gains. Each industry cluster is now described and illustrated in turn.
4.3.1. Cluster one: the ‘service’ cluster
The ‘service’ cluster was the largest, comprised of nine industries representing over 70% of respondents in the dataset. A range of benefits from current Gen-AI projects were reported and highly ranked by managers in these industries, the most frequent being customer satisfaction and cost savings. Efficiency, optimisation and productivity gains, and time and error reduction were also cited.
For example, a general manager in a US accommodation and food services concern described the multiple benefits of a partially implemented Gen-AI project:
Linking suppliers and distributors so everyone is aware of changes, shortages, and increases. AI is also used in client relations, as a way to track trends, needs, collecting data, and keeping up with other competitors in the market.
4.3.2. Cluster two: the ‘building & support’ cluster
The ‘building & support’ cluster was comprised of three industries and represents 15% of companies in the dataset. The main reported benefits from the use of Gen-AI were greater efficiency, optimisation and productivity, enhanced decision-making, and cost savings.
For example, an architect in the construction industry described the multiple benefits of a Gen-AI project in its trial phase:
Gen-AI is used in my construction industry to optimise planning and scheduling, minimise material waste, and reduce costs. For example, AI algorithms can analyse construction project data to identify potential bottlenecks and optimise the scheduling of resources to ensure timely delivery of materials and completion of the project. Additionally, Gen-AI is also used to optimise inventory levels by forecasting demand, thereby reducing the risk of stock outs and minimising the need for costly safety stock. Overall, the application of Gen-AI helps us improve efficiency and reduce costs, ultimately leading to increased profitability.
4.3.3. Cluster three: the ‘land use & other’ cluster
Three industries belonged to the ‘land use & other’ cluster, representing 8% of respondents in the dataset. The most frequently cited benefits include improved decision support and automation.
For example, a compliance manager from the US water supply, sewerage, and waste management industry described the automation and decision support benefits from AI:
For our customers, implementing supply chain AI solutions, the benefits of ChatGPT and Gen-AI are vast, including improved automated workflow and decision-making, guided scenarios and simulations, and intelligent risk management.
Currently our company has implemented Gen-AI to analyse all of the documentation throughout the sales process, from purchasing orders to additional services required and invoicing documentation. This has currently been through two testing phases on certain projects and has now been rolled out as a trial across the business for analysis by the whole team. We are hoping that by utilising this tool we'll be able to identify any patterns that we have been missing previously in order to capitalise on any gaps or take advantage of current market trends. Additionally, we have just begun to utilise an email routing system and automated AI communication system to help with customer-business relations.
4.3.4. Cluster four: the ‘arts’ cluster
The fourth cluster (‘arts’) was comprised of companies in the arts, entertainment and recreation industry. The main benefits derived from Gen-AI use included task automation, cost savings and business development.
For example, a US business owner described how a fully implemented Gen-AI project automates operations:
I use ChatGPT to do keyword research. When I have a product in mind that I want to sell I have ChatGPT come up with long-tail keywords to better position my products in Google. I have also used ChatGPT to write descriptions of my products that include keywords. Even more recently I have been using Gen-AI to create graphic mockups to help me visualise how I want certain products to look.
4.3.5. Cluster five: the ‘extraction’ cluster
The ‘extraction’ cluster was comprised of companies in the electricity, gas, steam and air conditioning supply, and the mining and quarrying industries. The main benefits accruing from Gen-AI use were efficiency, optimisation and productivity gains, reduced time and errors, and cost savings. Expected benefits from the future use of Gen-AI included business development opportunities, process efficiencies, and enhanced customer satisfaction.
For example, a sales coordinator from the UK mining and quarrying industry described expected future benefits for customer service from Gen-AI:
We are gradually implementing (or attempting to) AI in operations. By introducing this to our customers we hope they can get a faster and easier update on the status of their jobs with us than by using the conventional customer service route. This service will enable us to provide 24 h updates. We also hope it will have an effect on our profits. And reduce the time wasted by supply chain operations.
4.3.6. Cluster six: the ‘real estate’ cluster
The ‘real estate’ cluster was the sixth and final industry cluster In the dataset. The current benefits reported from the use of Gen-AI included gains in efficiency, optimisation and productivity, increased customer satisfaction, and content creation.
For example, a UK-based property manager described how Gen-AI is currently used for content creation:
Currently using it for marketing purposes. We use it to describe our spaces to potential tenants on Loopnet. We also use it to generate emails that we send to our clients.
ChatGPT is used in our company to update listing language as well as writing posts for our blog. By utilising AI, we can cut costs and reduce the time needed to generate these types of information.
5. Discussion
This study aimed to improve our understanding of the uses, benefits, and challenges of using Gen-AI in OSCM. The exploratory descriptive design, employing open questions and mixed methods of qualitative analysis, allowed us to uncover the main benefits enjoyed and expected by organisations, and the major challenges they face. The following discussion examines our results in light of our research propositions, identifies several scientific and managerial implications, and considers the study’s limitations and opportunities for future research.
5.1. Confrontation of results with research propositions
The results support our first research proposition that initial projects and benefits in OSCM will focus on task automation, efficiencies, and improved customer service. We found that current projects mainly concerned supply chain and inventory management issues, demand forecasting, resource planning, process efficiencies, time and cost savings. Several projects were customer oriented, aimed at improving service, satisfaction, and experience. Our findings show a strong alignment with the emerging academic and professional literature on Gen-AI. For instance, one of the primary objectives of early Gen-AI adopters is greater customer satisfaction through process efficiencies (Fosso Wamba et al. Citation2023; Hendriksen Citation2023; Zagorin Citation2023) and improved services (Young Citation2023). However, cluster analysis revealed a more nuanced answer to this first proposition: groups of industries differed according to the importance of four current benefits, including automation, cost savings, customer satisfaction, and the use of Gen-AI in decision support.
These results are consistent with diffusion of innovation theory: the ability of Gen-AI to enhance methods and processes relative to existing arrangements is the main driver of adoption. Rogers (Citation1983, 15) defines relative advantage as ‘the degree to which an innovation is perceived as better than the idea it supersedes’. Companies adopted Gen-AI technologies to improve supply chain performance (as defined by Beamon (Citation1999)), by better using resources (e.g. optimised inventory management), producing outputs (e.g. improved resource planning) and reacting to uncertainty (e.g. enhanced demand forecasting).
Other innovation characteristics as described by Rogers (Citation1983) may have also influenced Gen-AI adoption. Firstly, the strong media attention that generative AI has received (Bartholomew and Mehta Citation2023) may have increased the observability (16) of its capabilities and relative advantages. By reporting on business cases and the results of trials and experimentations, the press and the professional literature has made it easier to ‘observe relative advantages of the new technology’ (Rogers Citation1983). Secondly, the conversational nature of some generative AI technologies (e.g. ChatGPT) may have reduced the perceived complexity (15) of these new technologies (Rožanec et al. Citation2023).
We also found support for our second research proposition that current challenges to Gen-AI implementation in OSCM will concern AI models, technologies, and data access. Our results confirmed this proposition and revealed that data challenges (i.e. data quality, data privacy, and data security) and technological reliability were the main concerns of supply chain industry practitioners. Keyword co-occurrence analysis also revealed the importance of employee adoption and use, data quality, biases and ethical considerations. Again, these findings support the AI scholarly and professional literatures. For example, Cubric (Citation2020) conducted a tertiary study of thirty systematic literature reviews on AI adoption in business and management and reported three types of barriers to adoption: economic (e.g. cost), technical (e.g. data availability) and social (e.g. lack of knowledge). While we also found these three types of factors in the top ten challenges faced by organisations, technical and social issues ranked higher than economic factors, with ‘initial time and costs’ only ranked ninth. The predominance of technical and social factors is consistent with the professional and scholarly literature on Gen-AI in OSCM (e.g. Fosso Wamba et al. Citation2023; Hendriksen Citation2023), that has notably highlighted the challenges concerning the availability of qualified human resources (e.g. EY Citation2023).
These challenges may also be explained by diffusion of innovation theory. Potential adopters assess the compatibility of an innovation with ‘existing values, past experiences, and needs’ (Rogers Citation1983, 15). Challenges posed by legacy systems, employee reactions and ethical concerns may all influence the time that organisations take to ‘break down the organisational and cultural barriers that stand in AI’s way’ (Fountaine, McCarthy, and Saleh Citation2019, 4). Resolving these issues could enhance ‘the collaboration of human and artificial intelligence’ and move OSCM processes and activities closer to the promises of ‘Industry 5.0’ (Ivanov Citation2023).
It is also possible that the challenges and benefits of Gen-AI vary with the solutions deployed by organisations. For example, ‘Inspectorio CAPA recommender’ launched as the ‘world’s first generative-AI tool for supply chain management’Footnote7 has the ability to ‘assist brands, retailers, suppliers, factories and others’ improve the efficiency and effectiveness of their quality control processes. The system identifies the root-causes of defects or non-conformities in products, and makes recommendations for corrective and preventive actions (Mayer Citation2023). On the other hand, CoPilot, a conversational Gen-AI-enabled freight management platform that allows shippers to generate interactive reports, maps, and charts based on their shipping data may present different benefts and challenges.Footnote8
The results partially support our third proposition that expected benefits from future Gen-AI projects in OSCM will include gains accruing from business model transformations. Opportunities for business development or new business models were not frequently reported across the dataset. However, they were observed for industries in clusters three, four and five. One explanation for this result may be the mix of Gen-AI maturity levels and experiences between the companies in our dataset. As we are currently in the early use of generative AI technologies, some companies may need to move beyond tests and trials and begin actively using Gen-AI to better envisage possible changes to business models (Wells and Nieuwenhuis Citation2018).
The majority of expected future benefits were the same as those currently enjoyed by adopters, and mainly concerned operating efficiencies and customer experience. In addition, some organisations expect Gen-AI to improve the sustainability of OSCM processes. This result is consistent with the extant OSCM literature that has reported how the adoption of digital technologies is associated with more sustainable practices and outcomes (Schilling and Seuring Citation2023). For example, Gen-AI technologies may help reduce the uncertainties related to transitions to circular supply chains (Ghadge, Wurtmann, and Seuring Citation2020) and the risks of climate change (Schilling and Seuring Citation2023). According to a recent practitioner study, Gen-AI could enhance supply chain sustainability through improved supplier selection, risk mitigation, product design, and life-cycle assessment (Simons Citation2023).
Finally, we found support for our fourth research proposition that the rate of adoption of Gen-AI in OSCM will vary by industry. There were several similarities and differences in project maturity between industries: four industries reported that projects were still in the proof-of-concept or prototype phase, four were mainly in the test phase, and six reported that most projects were either fully or partially implemented. This result is consistent with findings in the diffusion of innovation (MacVaugh and Schiavone Citation2010) and OSCM literatures (Ivanov et al. Citation2021) that technology adoption varies across industries.
5.2. Research implications
Our study has numerous research implications. Firstly, it is the first to provide rich descriptions of how companies are using Gen-AI in OSCM across multiple industries. Our results contribute to the emerging literature on the adoption of AI (Dora et al. Citation2022; Merhi and Harfouche Citation2023; Olan et al. Citation2022), and Gen-AI technologies (Chang and Kidman Citation2023; Euchner Citation2023; Susarla et al. Citation2023; Zhang Citation2023). It also responds to recent calls for more empirical studies of Gen-AI adoption and use (Fosso Wamba et al. Citation2023; Li, Yue, and Zhao Citation2023).
Secondly, the emerging literature has already highlighted the limited practical and theoretical understanding of Gen-AI systems (Susarla et al. Citation2023) by scholars, practitioners, and policymakers. Our findings address this gap by providing a rich view of Gen-AI adoption and use. For example, the six industry clusters enrich our understanding of the benefits, challenges, and the future outlook of Gen-AI across different supply chains. Future studies could consider a more structured approach towards creating clusters, based for example on major SCM functions and activities (e.g. inventory management, product life-cycle management) or established operations management theories such as the theory of performance frontiers or the theory of swift (Schmenner and Swink Citation1998; Seuring Citation2009).
Thirdly, at a company level, our findings suggest that most Gen-AI OSCM projects focus on operational performance (e.g. demand forecasting, resource planning, process efficiencies) and require different types of resources to meet the challenges of adoption and use (e.g. human skills, organisation infrastructure, AI and data-driven culture). Future research could develop novel frameworks to explain and guide Gen-AI deployment.
Fourthly, our study highlighted industry differences in the timing of Gen-AI adoption and use. Future studies could explore the reasons for these differences. A finer analysis of industry specific adoption processes could improve our understanding of the role that social systems and innovation characteristics play in Gen-AI adoption.
Finally, diffusion of innovation theory could also be used to explain how, why, and at what rate Gen-AI technologies are spreading across different national cultures. Such studies could explore the need for culturally sensitive applications, policies and regulations to encourage or discourage the development of Gen-AI.
5.3. Managerial implications
Our study has several managerial implications. Firstly, our results provide a list of benefits and challenges faced by organisations when adopting Gen-AI. This list may serve as a checklist for managers across industries considering investing in these technologies.
Secondly, our findings suggest that the main benefits for organisations using Gen-AI in OSCM are enhanced customer satisfaction, cost reduction and improved efficiency. While these objectives are aligned with an operational ‘optimisation’ strategy in this first wave of adoption, supply chain managers and decision-makers should also consider the opportunities of using Gen-AI to support their strategic priorities. For instance, managers could use Gen-AI to identify opportunities in new markets, forecast demand, improve sustainability and transform business models.
Thirdly, managers should also consider the challenges of adopting Gen-AI technologies, notably relating to data (i.e. quality, privacy, and security issues) and the technology itself (i.e. model complexity, use of third party platforms). These issues would ideally be addressed during project feasibility studies prior to adoption.
Finally, our findings show different benefits, challenges and maturity levels between industries, alerting decision-makers to carefully consider the industrial specificities of their Gen-AI projects.
5.4. Limitations
The results of this study should be interpreted in light of several limitations. Firstly, given the novelty of generative AI and the strong media attention it has attracted, respondents may have had difficulty clearly defining reasonable future uses and challenges of these technologies. Also, respondents may have confused Gen-AI and traditional-AI based systems. Secondly, as this study was descriptive and exploratory, our research propositions should now be tested with explanatory research designs. Thirdly, data was collected over a short period of time. Given the fast moving nature of these technologies, future studies could use case studies (e.g. Chien et al. Citation2020) or longitudinal research to capture changes in perceptions and expectations over time.
6. Conclusion
Our study explored and described how and why companies are using Gen-AI in their OSCM, the challenges they face, and the benefits they expect from future use. Our study makes a number of contributions to this emerging field. Firstly, projects were equally spread between early stage experimentation, pilot phase trials and tests, and partial or full implementation. Secondly, in this early stage of Gen-AI, most applications were focused at the operational level, looking to improve customer satisfaction, and achieve cost savings and process efficiencies. Thirdly, our findings suggest that data quality, technological issues and organisational barriers are major challenges for adopting organisations. Finally, we found that the benefits, the challenges, and the rate of Gen-AI adoption in OSCM varies between industries. These findings enrich and advance the OSCM, AI and emerging Gen-AI literatures, and they provide practitioners with a structured understanding of the possible benefits and challenges of Gen-AI adoption.
Our results point to a number of promising avenues for research on Gen-AI in OSCM. Scholars could investigate how Gen-AI can help address OSCM issues of sustainability; how to overcome challenges relating to data quality and access; how to align organisational resources, both technological and human with Gen-AI projects; and how to generate new business value with Gen-AI in OSCM, beyond improved efficiency and customer service. Future research could also examine differences according to firm size, and in perceptions between junior employees and senior executives.
Disclosure statement
No potential conflict of interest was reported by the author(s).
Data availability statement
The data that support the findings of this study are available from the corresponding author upon reasonable request.
Additional information
Notes on contributors
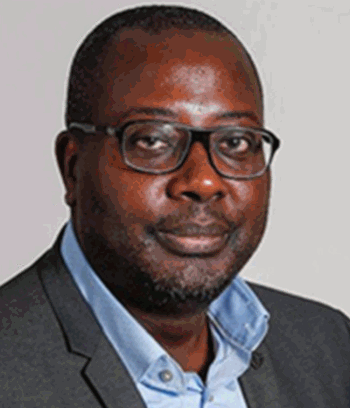
Samuel Fosso Wamba
Samuel Fosso Wamba is a Full Professor in Information Systems and Data Science and the Associate Dean for Research at TBS Education, Toulouse, France. He is also a Distinguished Visiting Professor at The University of Johannesburg, South Africa. He earned his Ph.D. in industrial engineering at the Polytechnic School of Montreal, Canada. His research interests include information technology adoption and use, business analytics, artificial intelligence for business, the Internet of Things, and metaverse for business.
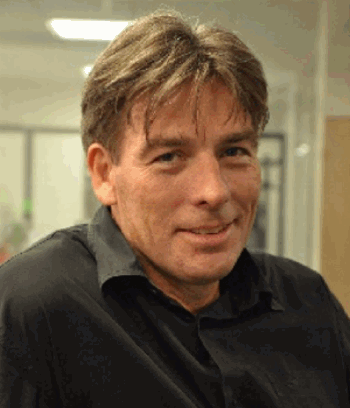
Cameron Guthrie
Cameron Guthrie is an Associate Professor in decision and system sciences at Toulouse Business School. He earned his Ph.D. in management science at the Université de Paris Panthéon-Sorbonne. His current research focuses on technology adoption, social innovation, wicked problems, and complex problem-solving. He has published papers in various journals, including European Journal of Information Systems, Technological Forecasting and Social Change, Journal of Retailing and Consumer Services, Knowledge Management Research & Practice, Business Process Management Journal, Industrial Relations, and Journal of Management History.
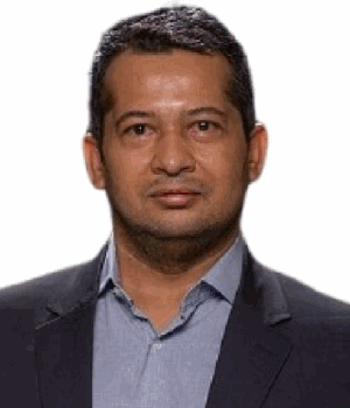
Maciel M. Queiroz
Maciel M. Queiroz is an Associate Professor of Operations and Supply Chain Management at FGV EAESP, Brazil, and Latin/South America Regional Ambassador of the Academy of Management OSCM Division. Maciel is an Associate Editor in the International Journal of Management Reviews and the International Journal of Logistics Management. He ranks in the World's Top 2% of most cited scientists Stanford/Scopus-Elsevier, 2022 and 2023.
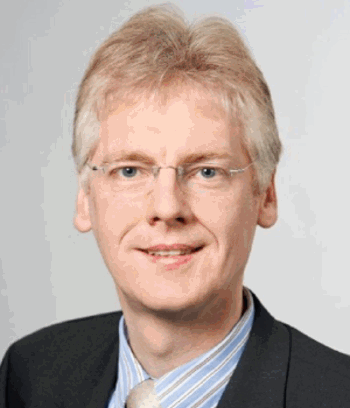
Stefan Minner
Stefan Minner is a Full Professor for Logistics and Supply Chain Management at the School of Management, Technical University of Munich (TUM). His research interests are in global supply chain design, transportation optimisation and inventory management using Operations Research and Machine Learning methods. The work was published in many peer reviewed journals, including Management Science, Manufacturing & Service Operations Management, Operations Research, Production and Operations Management, Transportation Science, Transportation Research Part B, European Journal of Operational Research, Computers and Operations Research and OR Spectrum. For his research output, he is currently listed among the top 1% business professors in German speaking countries by Wirtschaftswoche and among the top 2% researchers worldwide in a citation-based ranking by Stanford University. He serves on several editorial boards of logistics and operations journals. Currently, Stefan Minner is the Editor-in-Chief of the International Journal of Production Economics and of Sustainable Manufacturing and Service Economics. Stefan Minner is a fellow of the International Society for Inventory Research (ISIR) and is currently vice-chairman of the scientific advisory board of the German Logistics Association (BVL), and a member of the Research Committee of the European Logistics Association (ELA).
Notes
2 The questionnaire was administered by Prolific in S1 2023.
3 The large blue node beneath the yellow ‘productivity’ node.
4 The daisy() function from the cluster package was used in R to perform the analysis. A dissimilarity matrix was computed using the Gower dissimilarity measure (Gower Citation1971).
5 p-value < 0.1
6 p-value > 0.1
References
- Agrawal, Ajay, Joshua Gans, and Avi Goldfarb. 2022. “ChatGPT and How AI Disrupts Industries.” HBR Digital Article.
- Allied Market Research. 2023. “Generative AI Market Research, 2031.” 261.
- Badini, Silvia, Stefano Regondi, Emanuele Frontoni, and Raffaele Pugliese. 2023. “Assessing the Capabilities of ChatGPT to Improve Additive Manufacturing Troubleshooting.” Advanced Industrial and Engineering Polymer Research 6 (3): 278–287. https://doi.org/10.1016/j.aiepr.2023.03.003.
- Bartholomew, Jem, and Dhrumil Mehta. 2023, May 26. “How the Media is Covering ChatGPT.” Columbia Journalism Review.
- Beamon, Benita M. 1999. “Measuring Supply Chain Performance.” International Journal of Operations & Production Management 19 (3): 275–292. https://doi.org/10.1108/01443579910249714
- Bickman, Leonard, and Debra J Rog. 2009. “Applied Research Design: A Practical Approach.” In Handbook of Applied Social Research Methods 2, edited by Leonard Bickman and Debra J. Rog, 3–43. Los Angeles: Sage.
- Brynjolfsson, Erik. 1993. “The Productivity Paradox of Information Technology.” Communications of the ACM 36 (12): 66–77. https://doi.org/10.1145/163298.163309
- Brynjolfsson, Erik, Danielle Li, and Lindsey R. Raymond. 2023. “Generative AI at Work.” National Bureau of Economic Research Working Paper Series, No. 31161.
- Brynjolfsson, Erik, Daniel Rock, and Chad Syverson. 2018. “Artificial Intelligence and the Modern Productivity Paradox: A Clash of Expectations and Statistics.” In The Economics of Artificial Intelligence: An Agenda, edited by Ajay Agrawal, Joshua Gans, and Avi Goldfarb, 23–57. Chicago: University of Chicago Press.
- Chang, Chew-Hung, and Gillian Kidman. 2023. “The Rise of Generative Artificial Intelligence (AI) Language Models - Challenges and Opportunities for Geographical and Environmental Education.” International Research in Geographical and Environmental Education 32 (2): 85–89. https://doi.org/10.1080/10382046.2023.2194036.
- Chien, Chen-Fu, Stéphane Dauzère-Pérès, Woonghee Tim Huh, Young Jae Jang, and James R. Morrison. 2020. “Artificial Intelligence in Manufacturing and Logistics Systems: Algorithms, Applications, and Case Studies.” International Journal of Production Research 58 (9): 2730–2731. https://doi.org/10.1080/00207543.2020.1752488.
- Chui, Michael, Eric Hazan, Roger Roberts, Alex Singla, Kate Smaje, Alex Sukharevsky, Lareina Yee, and Rodney Zemmel. 2023. The Economic Potential of Generative AI: The Next Productivity Frontier. San Francisco: McKinsey & Company.
- Chui, Michael, Lareina Yee, Bryce Hall, Alex Singla, and Alexander Sukharevsky. 2023. The State of AI in 2023: Generative AI’s Breakout Year. San Francisco: McKinsey & Company.
- Cubric, Marija. 2020. “Drivers, Barriers and Social Considerations for AI Adoption in Business and Management: A Tertiary Study.” Technology in Society 62: 101257. https://doi.org/10.1016/j.techsoc.2020.101257.
- Dangayach, G. S., and S. G. Deshmukh. 2001. “Manufacturing Strategy: Literature Review and Some Issues.” International Journal of Operations & Production Management 21 (7): 884–932. https://doi.org/10.1108/01443570110393414.
- Deighton, K. 2023. “Companies Tap Tech Behind ChatGPT to Make Customer-Service Chatbots Smarter.” The Wall Street Journal, Accessed 26 May. https://www.wsj.com/articles/companies-tap-tech-behind-chatgpt-to-make-customer-service-chatbots-smarter-11674509622.
- Deloitte. 2023. Generative AI is all the Rage. Dublin: Deloitte AI Institute.
- Dey, Prasanta Kumar, Soumyadeb Chowdhury, Amelie Abadie, Emilia Vann Yaroson, and Sobhan Sarkar. 2023. “Artificial Intelligence-Driven Supply Chain Resilience in Vietnamese Manufacturing Small- and Medium-Sized Enterprises.” International Journal of Production Research, https://doi.org/10.1080/00207543.2023.2179859.
- Dolgui, Alexandre, and Dmitry Ivanov. 2023. “Metaverse Supply Chain and Operations Management.” International Journal of Production Research, https://doi.org/10.1080/00207543.2023.2240900.
- Dora, Manoj, Ashwani Kumar, Sachin Kumar Mangla, Abhay Pant, and Muhammad Mustafa Kamal. 2022. “Critical Success Factors Influencing Artificial Intelligence Adoption in Food Supply Chains.” International Journal of Production Research 60 (14): 4621–4640. https://doi.org/10.1080/00207543.2021.1959665.
- Dwivedi, Yogesh K., Nir Kshetri, Laurie Hughes, Emma Louise Slade, Anand Jeyaraj, Arpan Kumar Kar, Abdullah M. Baabdullah, et al. 2023. ““So What if ChatGPT Wrote it?” Multidisciplinary Perspectives on Opportunities, Challenges and Implications of Generative Conversational AI for Research, Practice and Policy.” International Journal of Information Management 71: 102642. https://doi.org/10.1016/j.ijinfomgt.2023.102642.
- El-Haddadeh, Ramzi, Mohamad Osmani, Nitham Hindi, and Adam Fadlalla. 2021. “Value Creation for Realising the Sustainable Development Goals: Fostering Organisational Adoption of big Data Analytics.” Journal of Business Research 131: 402–410. https://doi.org/10.1016/j.jbusres.2020.10.066.
- Elliott, D. J. M., and B. J. Griffiths. 1990. “A low Cost Artificial Intelligence Vision System for Piece Part Recognition and Orientation.” International Journal of Production Research 28 (6): 1111–1121. https://doi.org/10.1080/00207549008942779.
- Euchner, Jim. 2023. “Generative AI.” Research-Technology Management 66 (3): 71–74. https://doi.org/10.1080/08956308.2023.2188861.
- EY. 2023. Unleashing the Potential of Generative AI: A Game-Changer for P&C Insurance Claims. Dallas: EY.
- Fichman, R. G. 2000. “The Diffusion and Assimilation of Information Technology Innovations.” In Framing the Domains of IT Management: Projecting the Future Through the Past, edited by Robert Zmud, 105–128. Cincinnati, OH: Pinnaflex Educational Resources.
- Fosso Wamba, S., and A. T. Chatfield. 2009. “A Contingency Model for Creating Value from RFID Supply Chain Network Projects in Logistics and Manufacturing Environments.” European Journal of Information Systems 18 (6): 615–636. https://doi.org/10.1057/ejis.2009.44
- Fosso Wamba, Samuel, Maciel M Queiroz, Charbel Jose Chiappetta Jabbour, and Chunming Shi. 2023. “Are Both Generative AI and ChatGPT Game Changers for 21st-Century Operations and Supply Chain Excellence?” International Journal of Production Economics 265: 109015. https://doi.org/10.1016/j.ijpe.2023.109015.
- Fosso Wamba, S., M. M. Queiroz, K. Randhawa, and G. Gupta. 2023. “Call for Papers: Generative Artificial Intelligence and the Challenges to Adding Value Ethically.” Technovation 2023.
- Fountaine, Tim, Brian McCarthy, and Tamim Saleh. 2019. “Building the AI-Powered Organization.” Harvard Business Review 97 (4): 62–73.
- Gascoigne, H. 2023. “ChatGPT: The Next Big Thing for Business Transformation?” HobaTech.
- Ghadge, Abhijeet, Hendrik Wurtmann, and Stefan Seuring. 2020. “Managing Climate Change Risks in Global Supply Chains: A Review and Research Agenda.” International Journal of Production Research 58 (1): 44–64. https://doi.org/10.1080/00207543.2019.1629670.
- Gower, John C. 1971. “A General Coefficient of Similarity and Some of its Properties.” Biometrics, 857–871. https://doi.org/10.2307/2528823
- Gozalo-Brizuela, Roberto, and Eduardo C. Garrido-Merchan. 2023. “ChatGPT is not all you need. A State of the Art Review of large Generative AI models.” arXiv preprint arXiv:1703.01319. https://doi.org/10.48550/arXiv.2301.04655.
- Gravier, Michael. 2023. “The great decoupling: The real ChatGPT revolution.” Supply Chain Management Review.
- Hambrick, Donald C. 2007. “The Field of Management's Devotion to Theory: Too Much of a Good Thing?” Academy of Management Journal 50 (6): 1346–1352. https://doi.org/10.5465/amj.2007.28166119
- Helfat, Constance E. 2007. Stylized Facts, Empirical Research and Theory Development in Management. London: Sage Publications Sage UK, 185–192.
- Helo, Petri, and Yuqiuge Hao. 2022. “Artificial Intelligence in Operations Management and Supply Chain Management: An Exploratory Case Study.” Production Planning & Control 33 (16): 1573–1590. https://doi.org/10.1080/09537287.2021.1882690.
- Hendriksen, Christian. 2023. “Artificial Intelligence for Supply Chain Management: Disruptive Innovation or Innovative Disruption?” Journal of Supply Chain Management 59 (3): 65–76. https://doi.org/10.1111/jscm.12304.
- Huang, Allen H., Hui Wang, and Yi Yang. 2023. “FinBERT: A Large Language Model for Extracting Information from Financial Text*.” Contemporary Accounting Research 40 (2): 806–841. https://doi.org/10.1111/1911-3846.12832.
- Inbound Logistics. 2023. “Top 20 AI Applications in the Supply Chain.” Accessed 12 September. https://www.inboundlogistics.com/articles/top-20-ai-applications-in-the-supply-chain/.
- Ivanov, Dmitry. 2023. “The Industry 5.0 Framework: Viability-Based Integration of the Resilience, Sustainability, and Human-Centricity Perspectives.” International Journal of Production Research 61 (5): 1683–1695. https://doi.org/10.1080/00207543.2022.2118892.
- Ivanov, Dmitry, Christopher S Tang, Alexandre Dolgui, Daria Battini, and Ajay Das. 2021. “Researchers’ Perspectives on Industry 4.0: Multi-Disciplinary Analysis and Opportunities for Operations Management.” International Journal of Production Research 59 (7): 2055–2078. https://doi.org/10.1080/00207543.2020.1798035
- Jaca, Carmen, Elisabeth Viles, Daniel Jurburg, and Martín Tanco. 2014. “Do Companies with Greater Deployment of Participation Systems use Visual Management More Extensively? An Exploratory Study.” International Journal of Production Research 52 (6): 1755–1770. https://doi.org/10.1080/00207543.2013.848482
- Jackson, Ilya, Maria Jesus Saenz, and Dmitry Ivanov. 2023. “From Natural Language to Simulations: Applying AI to Automate Simulation Modelling of Logistics Systems.” International Journal of Production Research, https://doi.org/10.1080/00207543.2023.2276811.
- Jasti, Naga Vamsi Krishna, and Rambabu Kodali. 2015. “Lean Production: Literature Review and Trends.” International Journal of Production Research 53 (3): 867–885. https://doi.org/10.1080/00207543.2014.937508
- Jauhar, Sunil Kumar, Shashank Mayurkumar Jani, Sachin S. Kamble, Saurabh Pratap, Amine Belhadi, and Shivam Gupta. 2023. “How to use no-Code Artificial Intelligence to Predict and Minimize the Inventory Distortions for Resilient Supply Chains.” International Journal of Production Research, https://doi.org/10.1080/00207543.2023.2166139.
- Jo, A. 2023. “The Promise and Peril of Generative AI.” Nature 614 (1).
- Kamoonpuri, Sana Zehra, and Anita Sengar. 2023. “Hi, May AI Help you? An Analysis of the Barriers Impeding the Implementation and use of Artificial Intelligence-Enabled Virtual Assistants in Retail.” Journal of Retailing and Consumer Services 72: 103258. https://doi.org/10.1016/j.jretconser.2023.103258
- Katz, Daniel Martin, Michael James Bommarito, Shang Gao, and Pablo Arredondo. 2023. “Gpt-4 passes the bar exam.” Available at SSRN 4389233.
- Keating, B. W., T. R. Coltman, S. Fosso-Wamba, and V. Baker. 2010. “Unpacking the RFID Investment Decision.” Proceedings of the IEEE 98 (9): 1672–1680. https://doi.org/10.1109/JPROC.2010.2052530
- Kusiak, Andrew. 2020. “Convolutional and Generative Adversarial Neural Networks in Manufacturing.” International Journal of Production Research 58 (5): 1594–1604. https://doi.org/10.1080/00207543.2019.1662133.
- Lamba, Jag. 2023. “The Benefits and Risks of Generative AI in Supply Chain Management.” In Supply Chain and Demand Executive.
- Larsen, B., and J. Narayan. 2023 “Generative AI: A Game-Changer That Society and Industry Need to be Ready For.” World Economic Forum. https://www.weforum.org/agenda/2023/01/davos23-generative-ai-a-game-changer-industries-and-society-code-developers/.
- Lee, C. P., and J. P. Shim. 2007. “An Exploratory Study of Radio Frequency Identification (RFID) Adoption in the Healthcare Industry.” European Journal of Information Systems 16 (6): 712–724. https://doi.org/10.1057/palgrave.ejis.3000716
- Li, D., W. T. Yue, and J. L. Zhao. 2023. “Special Issue : ChatGPT and Generative AI in Finance.” Financial Innovation.
- Lu, Liyan, Changyong Liang, Dongxiao Gu, Yiming Ma, Yuguang Xie, and Shuping Zhao. 2021. “What Advantages of Blockchain Affect its Adoption in the Elderly Care Industry? A Study Based on the Technology–Organisation–Environment Framework.” Technology in Society 67: 101786. https://doi.org/10.1016/j.techsoc.2021.101786.
- MacVaugh, Jason, and Francesco Schiavone. 2010. “Limits to the Diffusion of Innovation.” European Journal of Innovation Management 13 (2): 197–221. https://doi.org/10.1108/14601061011040258.
- Malhotra, Manoj K, and Varun Grover. 1998. “An Assessment of Survey Research in POM: From Constructs to Theory.” Journal of Operations Management 16 (4): 407–425. https://doi.org/10.1016/S0272-6963(98)00021-7
- Mayer, M. 2023. “ChatGPT-Driven AI Tool for Supply Chain Management.” Accessed 12 September. https://www.sdcexec.com/software-technology/ai-ar/news/22834220/inspectorio-chatgptdriven-ai-tool-for-supply-chain-management.
- Merhi, Mohammad I., and Antoine Harfouche. 2023. “Enablers of Artificial Intelligence Adoption and Implementation in Production Systems.” International Journal of Production Research, 1–15. https://doi.org/10.1080/00207543.2023.2167014.
- Mintzberg, Henry, Duru Raisinghani, and André Théorêt. 1976. “The Structure of “Unstructured” Decision Processes.” Administrative Science Quarterly 21 (2): 246–275. https://doi.org/10.2307/2392045.
- Mizell-Pleasant, Alexis. 2023. “Alexis Asks: The Potential for ChatGPT in Logistics.” Supply and Demand Chain Executive.
- Olan, Femi, Shaofeng Liu, Jana Suklan, Uchitha Jayawickrama, and Emmanuel Ogiemwonyi Arakpogun. 2022. “The Role of Artificial Intelligence Networks in Sustainable Supply Chain Finance for Food and Drink Industry.” International Journal of Production Research 60 (14): 4418–4433. https://doi.org/10.1080/00207543.2021.1915510.
- Oxley, Joanne E, Jan W Rivkin, Michael D Ryall, and Strategy Research Initiative. 2010. The Strategy Research Initiative: Recognizing and Encouraging High-Quality Research in Strategy. London: Sage Publications Sage UK, 377–386.
- Peres, Renana, Martin Schreier, David Schweidel, and Alina Sorescu. 2023. “On ChatGPT and Beyond: How Generative Artificial Intelligence may Affect Research, Teaching, and Practice.” International Journal of Research in Marketing 40 (2): 269–275. https://doi.org/10.1016/j.ijresmar.2023.03.001.
- Pournader, Mehrdokht, Hadi Ghaderi, Amir Hassanzadegan, and Behnam Fahimnia. 2021. “Artificial Intelligence Applications in Supply Chain Management.” International Journal of Production Economics 241: 108250. https://doi.org/10.1016/j.ijpe.2021.108250.
- Precedence Research. 2023. Generative AI In Supply Chain Market.
- Punch, Keith F. 2013. Introduction to Social Research: Quantitative and Qualitative Approaches. London: Sage.
- PWC. 2023. Guide to Scaling Generative AI for Your Business. New York: PWC.
- Rai, Rahul, Manoj Kumar Tiwari, Dmitry Ivanov, and Alexandre Dolgui. 2021. “Machine Learning in Manufacturing and Industry 4.0 Applications.” International Journal of Production Research 59 (16): 4773–4778. https://doi.org/10.1080/00207543.2021.1956675.
- Richey, Robert Glenn Jr, Soumyadeb Chowdhury, Beth Davis-Sramek, Mihalis Giannakis, and Yogesh K Dwivedi. 2023. “Artificial Intelligence in Logistics and Supply Chain Management: A Primer and Roadmap for Research.” Journal of Business Logistics 44 (4): 532–549. https://doi.org/10.1111/jbl.12364
- Rogers, E. M. 1983. Diffusion of Innovations. 3rd ed. New York: The Free Press.
- Rožanec, Jože M., Inna Novalija, Patrik Zajec, Klemen Kenda, Hooman Tavakoli Ghinani, Sungho Suh, Entso Veliou, et al. 2023. “Human-centric Artificial Intelligence Architecture for Industry 5.0 Applications.” International Journal of Production Research 61 (20): 6847–6872. https://doi.org/10.1080/00207543.2022.2138611.
- Samarrokhi, Amir, Kouroush Jenab, and Philip D Weinsier. 2015. “The Effects of Lean Production and Six Sigma on Sustainable Competitive Advantage with Moderation of Suitable Resources.” International Journal of Services and Operations Management 21 (1): 112–125. https://doi.org/10.1504/IJSOM.2015.068703
- Schilling, Lara, and Stefan Seuring. 2023. “Linking the Digital and Sustainable Transformation with Supply Chain Practices.” International Journal of Production Research, https://doi.org/10.1080/00207543.2023.2173502.
- Schmenner, Roger, and Morgan Swink. 1998. “On Theory in Operations Management.” Journal of Operations Management 17 (1): 97–113. https://doi.org/10.1016/S0272-6963(98)00028-X.
- Seuring, Stefan. 2009. “The Product-Relationship-Matrix as Framework for Strategic Supply Chain Design Based on Operations Theory.” International Journal of Production Economics 120 (1): 221–232. https://doi.org/10.1016/j.ijpe.2008.07.021.
- Sharma, Rohit, Anjali Shishodia, Angappa Gunasekaran, Hokey Min, and Ziaul Haque Munim. 2022. “The Role of Artificial Intelligence in Supply Chain Management: Mapping the Territory.” International Journal of Production Research 60 (24): 7527–7550. https://doi.org/10.1080/00207543.2022.2029611.
- Simons, Tad. 2023. AI and Supply Chains: The Future is (Almost) Here. New York: Thomson Reuters.
- Susarla, Anjana, Ram Gopal, Jason Bennett Thatcher, and Suprateek Sarker. 2023. “The Janus Effect of Generative AI: Charting the Path for Responsible Conduct of Scholarly Activities in Information Systems.” Information Systems Research 34 (2): 399–408. https://doi.org/10.1287/isre.2023.ed.v34.n2.
- Telling, Oliver. 2023. “Multinationals Turn to Generative AI to Manage Supply Chains.” Financial Times.
- Terwiesch, Christian. 2023. “Would Chat GPT Get a Wharton MBA? A Prediction Based on Its Performance in the Operations Management Course. Philadelphia: Mack Institute for Innovation Management at the Wharton School, University of Pennsylvania.
- Tiffany, H. Kung, Cheatham Morgan, ChatGpt Medenilla Arielle, Sillos Czarina, Leon Lorie De, Elepaño Camille, et al. 2022. “Performance of ChatGPT on USMLE: Potential for AI-Assisted Medical Education Using Large Language Models.” medRxiv:2022.2012.2019.22283643. https://doi.org/10.1101/2022.12.19.22283643.
- Triantafyllidis, Kyriakos, and Daniel Kuepper. 2023. “Beyond the Status Quo: How Generative AI Will Transform Industrial Operations.” In World Economic Forum.
- Van Eck, Nees, and Ludo Waltman. 2010. “Software Survey: VOSviewer, a Computer Program for Bibliometric Mapping.” Scientometrics 84 (2): 523–538. https://doi.org/10.1007/s11192-009-0146-3
- Ven, Andrew H. Van de. 2016. “Happy Birthday, AMD!.” Academy of Management Discoveries 2 (3): 223–225. https://doi.org/10.5465/amd.2016.0090.
- Ven, Andrew H. Van de, Soon Ang, Africa Arino, Peter Bamberger, Curtis LeBaron, Chet Miller, and Frances Milliken. 2015. “Welcome to the Academy of Management Discoveries (AMD).” Academy of Management Discoveries 1 (1): 1–4. https://doi.org/10.5465/amd.2014.0143.
- Vujosevic, R. 1994. “Visual Interactive Simulation and Artificial Intelligence in Design of Flexible Manufacturing Systems.” International Journal of Production Research 32 (8): 1955–1971. https://doi.org/10.1080/00207549408957052.
- Wamba, Samuel Fosso, Ransome Epie Bawack, Cameron Guthrie, Maciel M Queiroz, and Kevin Daniel André Carillo. 2021. “Are we Preparing for a Good AI Society? A Bibliometric Review and Research Agenda.” Technological Forecasting and Social Change 164: 120482. https://doi.org/10.1016/j.techfore.2020.120482
- Wang, Han, Min Liu, and Weiming Shen. 2023. “Industrial-generative pre-Trained Transformer for Intelligent Manufacturing Systems.” IET Collaborative Intelligent Manufacturing 5 (2): e12078. https://doi.org/10.1049/cim2.12078.
- Webb, Taylor, Keith J. Holyoak, and Hongjing Lu. 2023. “Emergent Analogical Reasoning in Large Language Models.” Nature Human Behaviour 7: 1526–1541. https://doi.org/10.1038/s41562-023-01659-w.
- Wells, Peter, and Paul Nieuwenhuis. 2018. “Over the Hill? Exploring the Other Side of the Rogers’ Innovation Diffusion Model from a Consumer and Business Model Perspective.” Journal of Cleaner Production 194: 444–451. https://doi.org/10.1016/j.jclepro.2018.05.144.
- Wiles, Jackie. 2023. “Beyond ChatGPT: The Future of Generative AI for Enterprises.” Gartner.
- Wong, Lai-Wan, Garry Wei-Han Tan, Keng-Boon Ooi, Binshan Lin, and Yogesh K. Dwivedi. 2022. “Artificial Intelligence-Driven Risk Management for Enhancing Supply Chain Agility: A Deep-Learning-Based Dual-Stage PLS-SEM-ANN Analysis.” International Journal of Production Research, https://doi.org/10.1080/00207543.2022.2063089.
- Young, Liz. 2023. “Chatbots Are Trying to Figure Out Where Your Shipments Are.” The Wall Street Journal.
- Zagorin, Edmund. 2023. “How Generative AI Is Transforming Supply Chain And Procurement Roles.” Forbes.
- Zhang, Borui. 2023. “ChatGPT, an Opportunity to Understand More About Language Models.” Medical Reference Services Quarterly 42 (2): 194–201. https://doi.org/10.1080/02763869.2023.2194149.
Appendices
Appendix 1. Questionnaire items
The questionnaire included seven demographic questions and five questions pertaining to generative AI projects. The questions are reproduced below.
Q1 to 7: The respondent was invited to provide his or her gender, age, education level, functional area, seniority, management level, and industry.
Q8: At what stage are you with generative AI projects in OSCM?
Proof-of-concept
Prototype
Pilot
Trial
Partial or phased implementation
Full implementation
Q9: Describe your current use of generative AI in OSCM projects (one to two paragraphs).
Q10: What are the current benefits generated by generative AI in your OSCM? List the top five.
Q11: What are the current challenges related to using generative AI in OSCM? List the top five.
Q12: What are the future benefits expected from using generative AI in your OSCM? List the top five.