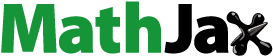
Abstract
Despite a general lack of examples showcasing seamless implementation of industry 4.0, talks of a human-centric, resilient, and sustainable industry 5.0 have already begun. But are the managers and workers of manufacturing enterprises today even ready for that? Digital transformation is still slow-going, especially within small- and medium-sized companies. Supported by an existing framework and interpretive structural model for digitalisation, we argue that a big part of digital transformation depends on the workers' competencies. Guided by the research question: How can the approach to competence development in SMEs explain patterns of slow digital transformation?, we investigate 30 manufacturing SMEs pursuing digitalisation. While manufacturing companies are aware of the need for additional competencies, few are actively working towards obtaining them. We expand on a theoretical model for smart manufacturing determinants and argue how a scaffold is needed to support SMEs in the early stages of digitalisation to increase understanding of core concepts, i.e. Industry 4.0 concepts and available technologies, data handling, integration capability, upskilling and training, and strategic management capabilities. We propose that a scaffolding approach to competence development can enable knowledge acquisition and the formation of digital transformation strategies.
1. Introduction
Since the introduction of industry 4.0 as a German strategic initiative in 2011 (Kagermann et al. Citation2013), both large- and small-sized manufacturing companies have worked towards leveraging or redefining their production facilities through digital transformation efforts (Horváth and Szabó Citation2019; Møller et al. Citation2023). Typically, the goal is a more interconnected and efficient production by exploiting data through new technologies (Xu, Xu, and Li Citation2018) and leveraging the information to respond in real-time both on the factory and supply chain level (also referred to as smart manufacturingFootnote1 Kusiak (Citation2018)). This interconnectivity requires well-thought-out system architectures capable of supporting horizontal integration across value and supply chains and vertical integration throughout the entire organisation from front-line staff to the top floor management (Pivoto et al. Citation2021). It is a significant and complex feat to manage and one that many SMEs have yet to overcome.
Researchers have tried to understand these complexities, map out the barriers that manufacturing companies experience, and pinpoint focus areas needed to advance digital maturity towards industry 4.0 (Larsen, Magnusson, and Lassen Citation2022). A few common barriers identified are resource availability (Horváth and Szabó Citation2019; Kamble, Gunasekaran, and Sharma Citation2018), lack of knowledge (Masood and Sonntag Citation2020; Stentoft et al. Citation2021), lack of skills (Estensoro et al. Citation2022; Horváth and Szabó Citation2019), and lack of technological and strategical expertise (Moeuf et al. Citation2020), where critical success factors include a skilled workforce through training and exploitation of data management (Moeuf et al. Citation2020; Stentoft et al. Citation2021). While SMEs have good opportunities for internal collaboration and employee involvement, they meet considerable barriers when it comes to their human resources (Horváth and Szabó Citation2019; Masood and Sonntag Citation2020; Matt et al. Citation2020; Stentoft et al. Citation2021). The skills of the industrial workers need to be on par with the new technology. Otherwise, the potential productivity gains will be left unfulfilled if workers cannot operate the technology (Lassen and Waehrens Citation2021; Moldovan Citation2019). Thus, it is evident that the digitalisation challenge is not purely technological. In light of this, we argue that manufacturing enterprises interested in digital transformation should address competencies early in the process. This, in turn, requires visionary and strategic managers of enterprises who both understand the possibilities of digitalisation and the importance of their human capital (Liboni et al. Citation2019). In this context, SMEs are particularly interesting to research because they make up a large proportion of the manufacturing industry (99.8% in Europe Gorgels et al. Citation2022) but are very slow to adopt smart technology (Buer et al. Citation2020; Lassen and Waehrens Citation2021). This means that SMEs are missing out on increased productivity through industry 4.0 solutions (Szász et al. Citation2020), especially if they do not invest in relevant knowledge and skills (Cirillo et al. Citation2023).
In this article, we seek to understand better the reasons for the slow digital transformation of SMEs in manufacturing industries. We move beyond merely identifying the particular characteristics of barriers and aim to explain how the approach to competence development in SMEs relates to the slow digital transformation we observe. We do so by examining empirical results from 30 Danish small and medium manufacturing enterprises pursuing smart manufacturing. We approach this matter through the lens of a human-centric perspective following the emerging suggestion of an Industry 5.0 approach. In particular, we seek to answer the question: How can the approach to competence development in SMEs explain patterns of slow digital transformation?
2. Literature review
Increased attention to the workers and their vital role in digital transformation has led to the concept called industry 5.0 (Breque, Nul, and Petridis Citation2021). Industry 5.0 generally relies on the same technological foundation as industry 4.0, although it focuses more on a human-centric approach (Ibid.). In industry 5.0 the well-being and upskilling of industrial workers are explicitly included in strategic considerations. It is more value-driven than technology-driven like industry 4.0 (Xu et al. Citation2021). Thus it may be seen as an extension to the industry 4.0 agenda rather than a new industrial revolution (Ibid.). Initiatives such as industry 5.0 bring increased focus to competencies for smart manufacturing and the interplay between sustainability, technology and human workers. Hence, it is a more holistic approach to the digital transformation of manufacturing industries. While the vision of industry 5.0 is a step in a more human-centric direction, it is important to remember how industry 5.0 needs the foundation that industry 4.0 provides. Therefore, if the digital transformation of companies pursuing industry 4.0 is slow and stuck in early stages, then industry 5.0 must be presumed basically non-existent. While industry 4.0 shows promise, it has also been criticised for being too focused on technology and less so on the role of the industry workers and how to build up the needed competencies (Lassen and Waehrens Citation2021; Neumann et al. Citation2021). However, neither small nor large companies can escape that industry 4.0 is much about knowledge management. And to this end, there is an inherent relationship between the available competencies and the extent to which a firm can leverage the benefits of digital tools (Capestro and Kinkel Citation2020).
2.1. Competencies for digital transformation
An increasing amount of literature has focused on the need for competencies within smart manufacturing and identified them through questionnaires (Moldovan Citation2019; Motyl et al. Citation2017), literature reviews (Belinski et al. Citation2020; Hecklau et al. Citation2016; Jerman, Pejić Bach, and Bertoncelj Citation2018; Kipper et al. Citation2021) and a few empirical studies (Fareri et al. Citation2020; Mahmood et al. Citation2021). Yet, it is difficult to list a definitive and objective set of competencies as it is not a precisely defined metric (Ananiadou and Claro Citation2009), and the meaning of competence varies across fields (Rangraz and Pareto Citation2021). However, according to Hecklau et al. (Citation2016), there is generally a need for technical, methodological, social, and personal competencies:
Technical competencies are needed to identify and build the needed connectivity. As work increasingly moves to digital platforms, there is a need to develop more profound technical knowledge, allowing workers to move away from purely operational tasks to more strategic and remotely operated tasks. Specialised technical knowledge, coding skills and cyber security awareness are necessary to navigate and create this technical environment.
Methodological competencies are thus needed due to the flat hierarchical structure of smart manufacturing enterprises and increased communication across devices and people. More decision-making power is laid in the hands of the individual worker, which increases the need for process knowledge and the ability to seek out and solve errors through data analysis and interpretation skills.
Social competencies are needed to effectively use the increased communication ability across departments through accessible IT platforms (e.g. Slack or Teams). Social competencies also pertain to navigating cultural differences and international teams. Good communication skills are essential in order to share knowledge among peers, which will become crucial in retaining knowledge in the organisation or making tacit knowledge explicit for it to be implemented in digital processes.
Personal competencies pertains to people's attitude towards change and efforts in learning new knowledge. Industry 4.0 brings a more flexible production, where workers move more fluidly between tasks. It is important to be able to accept this change in how work tasks are more flexibly structured (Hecklau et al. Citation2016).
Many of the needed competence areas fall outside purely technical areas and relate to how people perceive change, approach problem-solving, and the need for a supportive environment around learning. Therefore, almost purely fixating on technical competences may create a bottle-neck for smart manufacturing adoption as foundational competence areas are overlooked.
2.2. Existing models for smart manufacturing adoption
While researchers have advocated for social, personal, and methodological competencies, they seem primarily absent from seminal research streams on industry 4.0. Due to the inherent technical nature of industry 4.0, most literature has focused on enabling technologies, often neglecting human factors (Neumann et al. Citation2021). The vast interconnectivity of factories and supply chains envisioned for industry 4.0 requires vertical and horizontal integration that is capable of tying together a broad range of smart technologies in cyber-physical systems (CPS), which enables smart manufacturing. See Figure for a layered overview of smart technology examples (Ghobakhloo Citation2020). CPS, as such, can be perceived as a technical umbrella term within industry 4.0 that covers a broad area from the backend software, enabling horizontal and vertical data integration to the very frontend interfaces and machines, which are met by manufacturing workers across all layers in an organisation, thus integrating physical systems with communication networks (Pivoto et al. Citation2021). In a CPS, the conventional hierarchical pyramid structure of manufacturing processes and communication transforms into an interconnected cross-layer system, where each layer can communicate in a device-to-device communication setup (Antonino et al. Citation2019; Flores, Xu, and Lu Citation2020). It is an intricate system which needs careful planning. Therefore, different technical and theoretical reference architecture models have emerged to standardise and streamline the complexity of digital transformation, as summarised by both Pivoto et al. (Citation2021) and Nakagawa et al. (Citation2021), see Table .
Figure 1. A layered overview of smart technology consisting of: Information and communication technology (ICT), Computing technology, Operations technology (OT), Sensor and data acquisition technology and Human-machine Interaction (HMI). Abbreviations: radio frequency identification (RFID), enterprise resource planning (ERP), manufacturing execution system (MES), automated guided vehicle (AGV), autonomous mobile robot (AMR), Computer-aided design (CAD), programmable logic controllers (PLC). Adapted from (Ghobakhloo Citation2020).
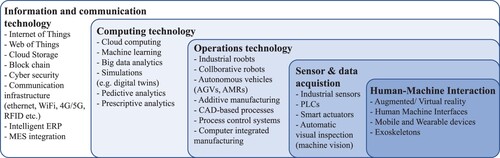
Table 1. Various industry 4.0 models and their type, focus, and view on competences.
The various reference architecture models show that the technical backbone of smart manufacturing is still under development. However, these ideas and smart manufacturing models still need to be communicated efficiently to the people within manufacturing, which proves challenging due to the abstraction level of the ideas, the broad interdisciplinary nature of the architectures, and the varying level of education in manufacturing.
The reference architecture models are currently not concerned with human factors, despite being crucial to their successful implementation (Sharpe et al. Citation2019). They merely adopt a technical focus (see Table ). Additionally, limited focus has been put on operationalising the architecture models, which often requires bespoke customisation (Nakagawa et al. Citation2021). For SMEs, a more grounded approach that specifies concrete focus areas may be more useful compared to complete technical reference architectures, which assume a higher digital maturity level than most SMEs possess (Mittal et al. Citation2018).
2.3. Smart manufacturing adoption in SMEs
Adoption of smart manufacturing solutions in SMEs is slow compared to large enterprises (Buer et al. Citation2020; Larsen, Magnusson, and Lassen Citation2022). Larger manufacturing companies tend to be more well-equipped for undertaking a digital transformation when it comes to financial resources, access to a global workforce, and overall business incentives to increase competitiveness (Horváth and Szabó Citation2019). SMEs do hold some advantages over large and multi-national enterprises when it comes to digitalisation, e.g. lower organisational complexity and fewer barriers to collaboration (Horváth and Szabó Citation2019). However, large-scale digital transformation initiatives are financially riskier for SMEs, and they often lack IT skills, which leads to a need for external support (Hansen and Hansen Citation2023; Moeuf et al. Citation2020). There is no 'one size fits all' solution. While reference architecture models outline a high-level structure of industry 4.0, few standardised solutions exist and the implementation following a reference architecture model still require highly specialised knowledge that is absent in SMEs. This indicates that SMEs should start with smaller steps towards their digital transformation (Buer et al. Citation2020). To better guide digitalisation efforts in SMEs, process models concerned with the operational adoption of smart technologies have recently emerged (Ghobakhloo Citation2020; Mittal et al. Citation2020). They recognise the importance of manager awareness and openness to industry 4.0 to initiate change. Mittal et al. (Citation2020) proposed and tested a framework for smart manufacturing adoption in SMEs from a technical point of view, focusing on data structure and connectivity capabilities. They analysed their case based on previously categorised 'toolboxes', which are bundles containing concrete focus areas where the SME was notably lacking, e.g. in data analytics. They found that successful steps towards smart manufacturing in SMEs really came down to the awareness of the managers (Mittal et al. Citation2020). Similarly, Ghobakhloo (Citation2020) unwrapped which mechanisms are crucial for manufacturers pursuing smart factory technology implementation. They conducted a structured literature review combined with expert input and used an interpretive structural modelling method to identify determinants for implementing smart manufacturing information and digital technologies (Ghobakhloo Citation2020). These smart technologies were divided into a layered overview as presented in Figure .
Ghobakhloo's model of determinants describes a step-wise process to implement smart technology (see Figure ). For example, a company that seeks to implement smart technology has to start with management perceiving the benefits of digitalisation, have financial and management support, and work on strategic roadmaps before employee qualification is undertaken and the technical implementation towards smart manufacturing can move forward. Thus, pinpointing that employee qualifications are a determinant for achieving smart manufacturing (Ghobakhloo Citation2020). As such, the path towards industry 4.0 combines people, organisation and technology in complex socio-technical systems (Liboni et al. Citation2019; Lundgren et al. Citation2022).
Figure 2. Ghobakhloo's model of determinants for adoption of smart technologies (Ghobakhloo Citation2020).
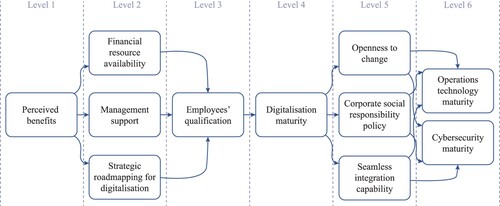
This line of thinking contrasts the technically abstract and holistic nature of the aforementioned reference architecture models, which practically ignore the human aspect of smart manufacturing and operate on a level unsuited for the reality of SMEs (Mittal et al. Citation2018). Additionally, such a standpoint is similar to the more human-centric approach to digital transformation, which we argue in favour of in this paper. Hence, a socio-technical process model is a solid analytical starting point for our research on how the approach to competence development in manufacturing SMEs influences their digital transformation, which may help explain the slow digital transformation in SMEs. For this, case studies on digital transformation have been called for Moeuf et al. (Citation2020) and Ghobakhloo and Ching (Citation2019).
Therefore, we investigate how a representative sample of SMEs within manufacturing matches the model of determinants for smart technology adoption and where they fall short. Identifying the areas where SMEs do not fit into the model may help explain why SMEs struggle to engage properly with digital transformation and thus contribute to theory related to digital transformation by expanding on the model of determinants. This was investigated empirically through a research program aimed towards SMEs to elevate their awareness of industry 4.0, as suggested by Buer et al. (Citation2020). Essential competencies have been identified, enabling technologies and decision aid models exist. However, it still appears hard for SMEs to transform. An in-depth study of multiple manufacturers can help explain why through a human-centred perspective.
3. Methodology
We applied a multiple case study approach to gain detailed insights into how manufacturing SMEs approach competence development and how this, in turn, influences their digital transformation. In doing so, we aim for results more practical in nature (Flyvbjerg Citation2006), and to provide more empirically founded research to understand human-centred approaches, which recently have been promoted (Grosse et al. Citation2023). Engaging directly with the cases combined with physical on-site company visits allows follow-up questions, which help describe real-life challenges that may not appear in surveys. By focusing on a broader selection, it is possible to compare similar issues from multiple sources. As such, this positions multiple case studies well in terms of developing, testing and refining theories (Ketokivi and Choi Citation2014; Voss, Johnson, and Godsell Citation2016).
We take our point of departure in the model of determinants by Ghobakhloo (Citation2020) and contrast our findings with the themes from the model. Therefore, we approach the phenomenon of digital transformation abductively to expand the existing model of determinants for digitalisation (i.e. Figure ) through a multiple case study of SMEs. Over the course of three years, we engaged multiple groups of SMEs in four engagements lasting three-four months each, providing them material on industry 4.0 and hands-on experience with smart manufacturing technologies to create a common language and inspire strategic thinking related to industry 4.0 through digital vision and maturity workshops.
3.1. Data selection
The focus was to gain insights into SMEs' digital transformation approaches. Hence, the central data selection criteria for the cases were based on the following:
Enterprises had to be small and medium-sized enterprises (SMEs) within manufacturing. SMEs comprise a large portion of the manufacturing industry, yet their digital transformation moves slowly. Thus, SMEs were chosen as our unit of analysis. The criterion is operationalised through the EU's definition of an SME: small = < 50 employees and
€ 10 million annual balance sheet; medium-sized = < 250 employees and
€ 50 million annual balance sheet (European Commission Citation2003).
Manufacturing companies interested in digital transformation. This ensured a fit to our unit of analysis while it increased the chances of engaged participation in the research. This criterion was operationalised as active participation in a large-scale research effort on digitalisation called Innovation Factory North (Møller et al. Citation2023)).
SMEs from a broad selection of manufacturing industries. This was to obtain a representative sample of manufacturing enterprises and gain a broader insight into actual focus areas or uncertainties. Including manufacturers from a diverse range of manufacturing industries was deliberate, as it enabled us to identify larger patterns representative of the current landscape within SMEs related to digital transformation. Additionally, working with a representative sample strengthens the transferability beyond just the sample.
All the cases chosen were from the Danish manufacturing sector, which allowed for comparable social and cultural context specificities through in-person engagements.
3.2. Data collection
The empirical data was collected through a learning process inspired by cognitive apprenticeship (Collins and Kapur Citation2014), which engaged managers and operational staff from a total of 30 SMEs. Cognitive apprenticeship roughly follows four dimensions:
Content: Domain knowledge about key concepts, procedures, and subject matter facts.
Methods: A prominent method is called scaffolding, which means that learning is structured and supported for the learner.
Sequencing: Variation in complexity and diversity of the tasks presented to the learner. This may be used along with the scaffolding method.
Sociology: Situated learning where the learner is taught or works in the context of realistic tasks and uses personal goals for intrinsic motivation e.g. within digitalisation.
The companies were interested in, and in the early stages of, digital transformation and multiple company representatives participated in structured learning activities aimed to raise their awareness of industry 4.0 (via industry 4.0 domain knowledge, i.e. content), where technologies listed in Figure were used as a reference. See Table for an overview of the SMEs and the company representatives who participated in this study.
Table 2. Overview of sample enterprises used for our study.
The activities consisted of two physical workshops at the university and two on-site company visits at the company (scaffolding, sequencing); see Figure . Representatives from 4–6 companies were present in each workshop, where debate was encouraged between companies and based on their personal digitalisation challenges (sociology). The on-site company vists were conducted with two academic sparring partners for each company. The overall company engagement was applied to 10 groups of SMEs over a period of three years.
Figure 3. Overview of company engagement within a learning factory environment from Møller et al. (Citation2023).
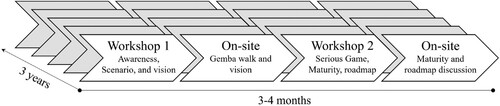
3.2.1. Data extraction
We consistently extracted statements from notes taken during the workshops by assigned note-takers. Thus, no voice recordings were made. However, the note-takers were instructed to type up responses as verbatim as possible. The workshops were conducted using a semi-structured approach. For example, the 360 Degree Maturity Assessment (360DMA), which involves structured learning through a dialogue between assessing and assessed teams, enhancing understanding of contextual factors and guiding context-specific improvement recommendations (Colli et al. Citation2019) that provided the structure for the maturity discussions in Workshop 1. Additionally, the on-site company visits at the SMEs' facilities acted as a follow-up on the workshops and were used as confirmation and triangulation of the information gathered during the workshops. See Table for an overview of the data collection. A more detailed description of the methods and approaches used in the workshops can be found in the supplementary material connected to this article, as recommended by Pratt, Kaplan, and Whittington (Citation2020) to ensure the trustworthiness of qualitative studies.
Table 3. Extent and type of data collection.
3.3. Data analysis
From the notes taken during the workshops, observations, and company visits, 414 unique statements related to the approaches to competence development and digitalisation were extracted and processed using QSR NVivo (NVivo Citation2022). The data analysis went through two stages: i) identification of statements which fit into the model of determinants in Figure referred to as the engagement cluster (EC); and ii) identification and analysis of the statements which did not fit the model of determinants referred to as the hindrance cluster (HC). We used the model of determinants in Figure as a theoretical framework so that statements, where the SMEs described some level of engagement with the model, were coded according to the model of determinants' 11 dependency themes (see Figure ).
3.3.1. Stage 1: engagement cluster
147 statements matched the model of determinants on some level of engagement with the model themes. This resulted in the engagement cluster (EC) in the first half of Table . Furthermore, the level of engagement was determined based on a non-statistical approach to reaching classifications of high, medium or low levels of engagement. The following logic was applied:
High levels of engagement: expressions of clear and dedicated activities, strategies or knowledge;
° Example from Employee Qualifications (EQ): 27. 'Dedicated education budget used on digitalisation courses for managers'
Medium levels of engagement: expressions of activities, strategies or knowledge lacking indication of either high or low levels of engagement.
° Example from Employee Qualifications (EQ): 3. 'Hired external consultants to help with ERP system but not how to involve employees'
Low levels of engagement: expressions of sparse and unspecific activities, strategies, or knowledge
° Example from Employee Qualifications (EQ): 27. ‘We currently rely on an unstructured peer-to-peer training process’
Table 4. Level of engagement and mismatch with model of determinants in the engagement cluster (EC) and the hindrance cluster (HC): Perceived Benefits (PB), Financial Resource Availability (FRA), Management Support (MS), Strategic Roadmapping for Digitalisation (SRD), Employee Qualification (EQ), Digital Maturity (DM), Corporate Social Responsibility (CSR), Seamless Integration Capability (SIC), Openness to Change (OC), Operations Technology Maturity (OTM), Cybersecurity Maturity (CM).
3.3.2. Stage 2: hindrance cluster
178 statements were found to relate to the 11 dependency themes, but with an emphasis on how the companies experienced uncertainties, challenges or hindrances related to these. This meant that no level of active engagement was expressed through these statements. These 178 statements were categorised as the hindrance cluster (HC) and were further analysed to identify explanatory patterns. Also included in the hindrance cluster were 88 statements which did not match any of the 11 themes. To make sense of the statements in the hindrance cluster, we applied the approach and terminology introduced by Gioia and Chittipeddi (Citation1991) and later updated by Gioia, Corley, and Hamilton (Citation2013).
The first step was to develop the first-order concepts from our sample. A single first-order concept consists of multiple related sample statements. Thus, the statements initially went through an open coding process when extracted from the workshop notes, and later, through axial coding, we connected related statements to tentative abstract codes that supported the development of first-order concepts. For example, 14 sample statements were grouped together to form the first-order concept 'manual data processes'.
The second step was to analyse the first-order concepts and truly encompass their meaning. This relied on an iterative process where the sample statements were revisited to ensure refinement. For example, first-order concepts that captured challenges regarding comprehending technology and industry 4.0 concepts, difficulties understanding how to develop strategies and perceive value, and knowledge differences between management and employees were joined under the second-order theme lack of understanding.
The third step involves distilling the first-order concepts and second-order themes into aggregate dimensions, which contain the essence of all the underlying codes and statements. For this, we relied on multiple rounds of coding, performed first independently by two of the authors, and then revisited and discussed among all to identify any differing interpretations and reach a consensus on how the data should be interpreted. For this step, we reviewed literature to inform us how the aggregated dimension could be interpreted. This meant that an aggregated dimension initially was coded as 'knowledge acquisition', but through revisiting the sample and reviewing the literature on prior knowledge (e.g. Dochy, de Rijdt, and Dyck Citation2002), the aggregated dimension was refined to a more abstract 'content knowledge' as this more accurately described the underlying issue of how the companies may overcome the challenges related to their lack of understanding of available technologies, data handling and integration challenges. By following this approach and thoroughly documenting the steps taken throughout the analysis in a data structure, we strive to reach theoretical saturation (Gioia, Corley, and Hamilton Citation2013). The resulting data structure representing the first-order concepts, second-order themes, and aggregate dimensions are presented in Section 4.2.
4. Findings
In the following, we present the findings from the analysis of the 414 unique statements relative to the state-of-the-art understanding of how companies adopt smart technologies, as suggested by Ghobakhloo (Citation2020). First, the results from the engagement cluster will be presented in each of the 11 dependency themes in Section 4.1. Then, the findings from the hindrance cluster and the Gioia methodology will be presented in Section 4.2. This analysis forms the basis for developing an updated model of determinants, which highlights areas where the companies need support in order to engage with the technology adoption and digital transformation in Section 4.3. Finally, we advocate that a supportive scaffold be made available for SMEs throughout the digitalisation process in Section 5.
4.1. Level of engagement with the model of determinants
Statements, where the SMEs described some level of engagement with the model of determinants, were coded according to the 11 dependency themes (see Table ). Of the 414 unique statements, 147 statements fit the engagement cluster as either expressions of low, medium, or high level of engagement with the 11 dependency themes. 267 statements were grouped in the hindrance cluster, as the statements either were a mismatch with the dependency themes, or the statements expressed a specific lack of activities related to the dependency themes.
4.1.1. Perceived benefits
Among the perceived benefits of digital transformation expressed by the companies were cost reduction, automatic data projection and data flow, leading to less employee stress. Additionally, increased data communication was understood as generating potential for quicker reactions to errors, while digital tools were expected to improve delivery and consistency of supply. Additive manufacturing was seen as an area of interest explored through external collaboration and company visits. Perceived benefits of smart technologies were also influenced by previous experiences with similar technology. Virtual reality was interesting too from a remote maintenance perspective. The SMEs often use vague terms related to specific technologies with few examples of actual value added.
8. ‘Great expectations that automation improves production, reduces cost price, increases competitiveness’; 4. 'Want to use digital tools to improve delivery and consistency of supply'; 13. ‘We see a potential to quicker react to production errors if our workstations could exchange data between them [currently tech-islands]’; 18. 'We are interested in the possibilities VR has within remote maintenance'
The companies expressed a lot of uncertainties as well, mainly a lack of understanding from both managers as well as the shop-floor workers. The main topics where they expressed lack of understanding were within data and what value it may bring. A general lack of understanding of Industry 4.0 and related technologies persists, which, in turn, makes it difficult to create strategies or to formulate a business case around digitalisation as there is no clear understanding of what value can be created.
4.1.2. Financial resource availability, management support, and strategic roadmapping for digitalisation:
Some but not all, express awareness that investments are needed for digitalisation. The statements generally mention that money is there or allocated for digitalisation projects. However, the projects are not clearly defined.
Overall, there is a tendency for management support within most of the companies, which was to be expected as members of the management agreed to participate in the research project about digitalisation.
29. ‘Our group supports our digitalisation efforts’; 16. ‘We think I4.0 should be a top-down process in the organisation’; 3. 'Willingness towards digitalisation from the board'
Only one company mentions the use of roadmaps and use of ‘lighthouses’ to communicate strategy to the employees. One other mentions a defined plan for an isolated IT project involving a new ERP system.
13. ‘We communicate our strategies through ‘lighthouses”’; 23. ‘New ERP project initiated with defined start/end date, team and processes to identify needs’
The areas where the SMEs express uncertainty are related to producing a proper business case before the allocation of financial means to support digitalisation efforts. Management support is strong, however, some still express a certain conservatism among management and a considerable knowledge gap from the top management down through the organisation. Strategic roadmapping for digitalisation is where the biggest uncertainty lies, where many express the lack of a digital strategy.
4.1.3. Employee qualifications
Employee qualification is mentioned, but on a very basic level, e.g. ad hoc peer-to-peer training. Very few mention external courses and planned training, e.g. one mention of a separate management development track about interface standards.
25. ‘We have a separate management development track about interface standards and development processes’; 27. ‘We currently rely on an unstructured peer-to-peer training process’; 3. ‘Hired external consultants to help with ERP system but not how to involve employees’
The SMEs show a general understanding of which competencies they need (or have identified they need). Yet, few prioritise upskilling and training outside of peer-to-peer on the floor and management level, hence not moving in the direction of their established competence needs. The competencies they expressed a need for included: data-flow management, how to structure data (e.g. use of Power BI (business intelligence) and ERP data integration), data analysis, IT knowledge, 3D modelling, robot programming, programming above PLC level, and digital product configuration.
4.1.4. Digitalisation maturity
The SMEs mentioned topics showcasing engagement with the digitalisation maturity theme from the model of determinants. Mostly their engagement consists of IT systems such as ERP, Customer Relationship Management (CRM), Business Intelligence (BI), Warehouse Management System (WMS) or the use of digital dashboards and digital tools, e.g. the use of 3D drawings (Computer-Aided Design (CAD)) integrated into their production processes.
1. ‘We use Navision ERP system, which is integrated with our CAD program’ 30. ‘BI connected to ERP and used for performance measurement and decision-making’
However, this is the theme that the companies tend to struggle the most with, especially aspects surrounding data collection and exploitation. In many instances, data is collected but with no apparent use.
4.1.5. Corporate social responsibility, seamless integration capability, and openness to change
Very little was revealed about the Corporate social responsibility efforts of the SMEs. A few mention the wish to maintain current employees, striking a balance between OEE and surveillance and welcoming apprenticeships, which benefit both society and the SME.
8. ‘Wants to improve competitiveness, while maintaining employees’
Overall a very low level of engagement with corporate social responsibility was portrayed. In addition, the SMEs showed very little integration capability, meaning very little available knowledge about and technology for enabling horizontal and vertical integration. A few examples that could be fitted to the model of determinants include the active use of cloud-based services and remote monitoring of machines.
13. ‘In the process of migrating to Office 365 with cloud services’ 1. ‘Two of our machines can be monitored and controlled remotely’
Overall integration capability is an area where the SMEs struggle most based on the scope of responses and the portrayed low maturity level.
According to the companies, there was a general openness towards new initiatives and technology. Nearly half of the companies expressed that their workers displayed a willingness to learn, and some even regularly make digitalisation suggestions on their own initiative.
31. ‘Employees are generally positive towards new systems/initiatives’ 22. ‘We have the right culture to test out new initiatives’
4.1.6. Operations technology and cybersecurity maturity
The SMEs tend to focus more on operations technology such as robots and 3D printing compared to cybersecurity, which only one statement touched upon
13. ‘3D printing currently used for bespoke fixtures in production but not in products’ 30. ‘Milling and drilling department is most advanced with auto-feeding robots, semi-automated welding, three fully automatic robot cells.’ 13. ‘Our board is actively supporting value-adding technology, e.g. approved a big budget for cybersecurity’
Despite that many areas within smart manufacturing are not in place, a big group of companies mention their use of advanced operations technology, which indicates that SMEs prioritise physical hardware solutions. In contrast, most hindrances are mentioned within data associated with operations technology.
4.1.7. Sub-conclusion
Through the statements and on-site company visits, the SMEs show productive activities related to the determinants for smart technology implementation. Especially within management support, openness to change, operations technology maturity and, perhaps surprisingly, financial resource availability. However, they are aware of great challenges in perceived benefits, strategic roadmapping for digitalisation, employees' qualification, digitalisation maturity, corporate social responsibility policy, seamless integration capability and cybersecurity maturity. Statements describing challenges in these latter areas outweigh productive and active activities, and the majority fall outside the high, medium, and low level of engagement. This created the hindrance cluster, which is analysed in the next sections.
4.2. Foundational lack of content knowledge
The hindrance cluster was used to conduct an analysis following the Gioia methodology (Gioia, Corley, and Hamilton Citation2013) to make sense of the statements. The first aggregate dimension, content knowledge, (see Figure ) describes the lack of understanding of industry 4.0 and related technologies both from the management and shop-floor level. This included knowledge surrounding data and integration. The aggregated dimension describes areas where the companies need assistance in gaining deeper knowledge and understanding to advance their digital maturity, and thus describe what are important topics within digitalisation. This is explained in three second-order themes: lack of understanding, data handling, and integration challenges.
Figure 4. The data structure based on the hindrance cluster.
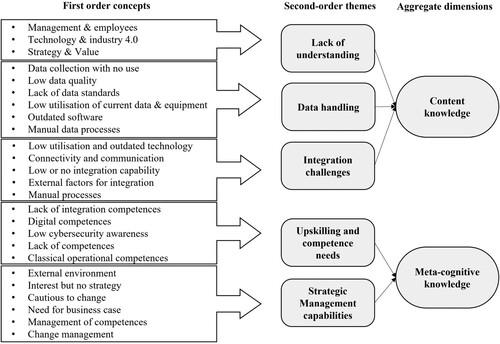
4.2.1. Lack of understanding
The first theme contributing to the aggregated dimension called content knowledge is centred around a lack of understanding of digital technologies and the overall concept of industry 4.0. This lack of understanding is present both from managers and shop-floor employees.
10. ‘Our digitalisation agenda is not understood generally by employees’; 14. ‘Management is interested in employee involvement because some have difficulties understanding the purpose of digitalisation’; 12. ‘We have overall goals but lack a plan and an understanding of why they are important’
The uncertainty surrounding industry 4.0 makes it difficult for the companies to understand what value a given technology may provide. Most of the sample companies also do not have any strategies or visions related to digitalisation as they lack the knowledge to base their direction on.
25. ‘We lack understanding of data analysis and what value it can bring’; 12. ‘It's difficult to define, which needs digitalisation should fulfil’; 10. ‘It is hard to plan a strategy when we don't know which technological solutions exists’
4.2.2. Data handling
The second theme under content knowledge relates to the companies' difficulties surrounding the use of data. Here there are a variety of obstacles for the companies related to low utilisation of the data that is currently being collected in their production processes. Many of the companies collect data in their current production processes, yet the majority do not use the collected data. The data quality is also currently a concern for the companies e.g. they experience discrepancies between actual available stock and what is listed in their ERP system. Many still rely on outdated and now unsupported ERP systems, which have been tailored to their needs but now presents an inflexible obstacle when looking towards newer digital solutions to be implemented alongside the ERP. Additionally, the companies currently rely on many manual processes, which they appear unable to overcome.
11. ‘We want to collect data but do not know for what’; 2. ‘We have historical data from our machines from the past 5 years but it has never been used’; 12. ‘We perceive our current ERP system as a barrier to pursue potential new solutions’; 27. ‘Warehouse data is there but often inaccurate, which causes a mismatch between the system and physical warehouse’; 15. ‘We have to manually handle an order 5 times before it leaves the factory’; 29. ‘Employees manually log order/product information. They manually write directly on the product, which is not ideal’; 20. ‘We need a better data flow. Currently a lot of files are sent manually’
4.2.3. Integration challenges
The third theme under knowledge acquisition contains challenges related to system integration and how SMEs struggle to establish effective connectivity between systems to enable such integration. The challenges are related to their available equipment, which either is not utilised fully or is outdated and inherent to their current processes, which makes integration difficult. This includes the many manual processes and tasks reliant on craftmanship, which makes it hard to achieve a meaningful integration.
31. ‘Servers collect data but are not connected/communicating’; 12. ‘Log data in multiple places due to lack of integration’; 8. ‘Currently have a very basic and classic setup with little connectivity. Working towards increased partner integration’ 11. ‘Our customers are unwilling to provide access/connectivity to our machines’; 26. ‘Our machines are not connected. Difficult when a lot of processes rely on craftmanship’; 31. ‘We currently print every drawing for every order’
Table 5. Supporting data used for each second order theme presented in the data structure.
4.3. Foundational lack of meta-cognitive knowledge
The second aggregate dimension captures the struggle for the SMEs to work on digital transformation strategies – that is, having clear plans for digitalisation including business needs, change management, competence requirements, and how to upskill or otherwise acquire the needed competencies. The dimension captures the need for competent management that positions the company advantageously for future digital changes. Meta-cognitive knowledge thus describes insights into how to learn and awareness of the processes involved. It consists of two themes: upskilling and competence needs and strategic management capabilities.
4.3.1. Upskilling and competence needs
The companies are aware that they need different competencies compared to what they currently have if they are to advance with digitalisation efforts. This especially materialises in competencies related to system integration and digital competencies related to data utilisation. Unsurprisingly, the SMEs are strong within classical operational competencies i.e. product-specific knowledge and masters of their own area, but lack experience with digital IT systems.
20. ‘We don't have the competencies for creating power BI apps or integration with customers ERP’; 8. ‘Our workers have very basic IT competencies and only know how to operate their specific machines’; 2. ‘We don't utilise the current equipment, which supports digitalisation optimally. It requires training in CAD-based software and knowledge of data utilisation’; 19. ‘Do not know the level of internal IT security’; 12. ‘We don't have the competencies for data-flow management’; 15. ‘On a journey from traditional craftmanship to digital modern factory’; 17. 'Our production is mainly practical and manual with a majority of unskilled labour'
4.3.2. Strategic management capabilities
The companies show a high interest in the general opportunities that industry 4.0 may provide, however, the vast majority do not have any strategy when it comes to digital technologies. The companies struggle with change management, e.g. when introducing new initiatives.
19. ‘Technology roadmap is in place but lack a clear IT strategy and roadmap’; 26. ‘We have management support for digitalisation initiatives but lack a concrete vision and strategy’ 2. ‘There's no training strategy in place’; 1. ‘We are interested, but have no concrete plan for digitalisation’; 3. ‘We have a general direction but no concrete digitalisation plan. We need more knowledge’ 17. ‘We can't send our employees through training courses/education as it would halt the production’ 25. ‘We lack the structure and resources to drive a digitalisation process’
There are also decisions surrounding external factors that managers need to navigate, e.g. when to rely on building internal competencies or buy externally. The labour market also affects decision-making around digitalisation as some companies experience that much-needed courses are unavailable or certain competence profiles are hard to find. One mentions a hard time keeping their employees after they have gained new skills as they seek new job opportunities elsewhere. Creating a business case to justify investments in digital technology is generally perceived as a challenge, yet a necessity.
30. ‘If the business case is clear, the money is there’; 11. ‘Difficult to create a business case around our solutions’
4.4. Implications for the model of determinants
Based on the company statements, the table of engagement (Table ) and the data structure in Figure ), we interpret the areas where companies face the biggest hindrances. These are mapped in Figure . The highlighted hindrances are identified through both the distribution (high, medium, low) and the content of the statements. We identify what can be considered a level 0, focusing on their lack of understanding of industry 4.0 concepts, low data handling and integration capability (content knowledge), and strategic management capabilities together with structured upskilling and competence development (meta-cognitive knowledge). With no support, the companies will struggle to transform effectively, as described in the model of determinants shown in Figure . The highlighted themes show that SMEs need support in vital steps throughout the entire journey towards smart manufacturing technology.
Figure 5. New model of determinants, where observed hindrances are highlighted.
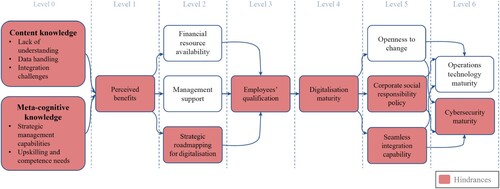
5. Discussion
Firstly, the findings of our study tie into a growing body of research that identifies the main barriers to industry 4.0 adoption (Masood and Sonntag Citation2020; Møller Citation2019; Stentoft et al. Citation2021), and literature, which emphasises greater focus on the importance of the humans involved alongside digital technologies (Kaasinen et al. Citation2020; Kadir and Broberg Citation2021; Rangraz and Pareto Citation2021; Xu et al. Citation2021). The SMEs in our sample generally experience that their employees show a high willingness to learn, and at the same time the organisation is not clear on what they want to achieve, nor have they prepared their workers for the change initiatives, e.g. by presenting a clear vision and roadmap for planned activities and targets to re-design their work systems considering human factors, as suggested by Kadir and Broberg (Citation2021). Awareness and guidance among management are needed for companies to elevate the support from the shop-floor. Additionally, openness towards industry 4.0 (Cugno, Castagnoli, and Büchi Citation2021) and employee involvement (Tortorella et al. Citation2021) heavily influences how well enterprises manage to adopt industry 4.0 technologies. To exploit this openness to industry 4.0, it requires both a level of awareness towards available technologies and on how to transform that into perceived value in the organisation – including attention to competencies and employee qualification. This falls well in line with previous results and models describing digital transformation (Cugno, Castagnoli, and Büchi Citation2021; Ghobakhloo Citation2020; Mittal et al. Citation2020). However, it appears that it is not possible for small companies to do effectively without external support (Hansen and Hansen Citation2023; Moeuf et al. Citation2020).
In many ways, digital transformation can be viewed as a learning process. Prior knowledge has previously been identified as the biggest influencing factor on learning outcome (Dochy, de Rijdt, and Dyck Citation2002). Dochy et al. summarise key aspects that make up the concept of prior knowledge. Here, content knowledge and meta-cognitive knowledge are the two main pillars, where content knowledge relates to domain specific knowledge, where meta-cognitive knowledge is more reflective and deals with the understanding of the learning processes related to tasks, the self, and strategic processes, according to Dochy, de Rijdt, and Dyck (Citation2002), who further conclude that, while prior knowledge is a key factor to reap the benefits, it also highly depends on the facilitation of learning (Ibid.). Aligning and facilitating a path for the companies may therefore profoundly affect their digital maturity. If companies do not manage to expand on relevant prior knowledge, one risks getting caught in knowledge inertia. Knowledge inertia locks companies into a finite set of processes and knowledge defined by past experience (Liao, Fei, and Liu Citation2008). If no new knowledge is introduced to break their existing knowledge inertia, companies will continue on the path determined by knowledge and skills untenable to adopting smart technologies.
This is revealed in the interaction between the content knowledge dimension, i.e. knowledge about specific smart technologies and their related processes, and the meta-cognitive knowledge dimension, in which companies express serious uncertainty about how best to move forward and strategically plan their digitalisation direction. Especially, how to identify the right competencies, how to obtain new knowledge and develop competencies, while being confident in the business case. Evidence of the SMEs' weak knowledge foundation of industry 4.0 appears to send ripples through the ideal path defined by Ghobakhloo (Citation2020), which we highlight in Figure as observed hindrances (i.e. evidence of knowledge inertia). Thus, the addition of a ‘Level 0’ to the model of determinants provides a better starting point and knowledge foundation for companies to engage with the dependency themes than what is currently observed. This leads to our first proposition:
Proposition 1
Challenges towards digitalisation experienced by SMEs can be mitigated by an increased focus on both their understanding of content knowledge and meta-cognitive knowledge related to industry 4.0. Content knowledge relates heavily to the general conceptual understanding of industry 4.0, and specifically, value creation through data exploitation methods and integration capabilities. Meta-cognitive knowledge encompasses how to work strategically with digital technologies and how to target competence development early to increase digitalisation capability.
Secondly, our findings suggest a big deficiency in strategic management, beyond attention directed towards industry 4.0, which aligns with barriers identified by Kadir and Broberg (Citation2021); Møller (Citation2019); Stentoft et al. (Citation2021), and Lassen et al. (Citation2023). An example of strategic competencies can be found within Saabye, Kristensen, and Wæhrens (Citation2022). They approached industry 4.0 adoption from an action learning perspective and focused on developing a learning to learn capability in an organisation. They emphasised the role of management and broad involvement of people in the organisation beyond cost reduction (digitalisation) and towards organisational capabilities (digital transformation). Knowledge about industry 4.0 has to be present, and managers should adopt a role as learning facilitators instead of solely relying on acquiring best practice knowledge about industry 4.0, while fostering a supportive learning environment that encourages questions and helps create a psychologically safe environment (Saabye, Kristensen, and Wæhrens Citation2022). Likewise, Ghobakhloo and Fathi (Citation2020) reported a significant reduction in defect rates after introducing focused management practices in an SME. They emphasised the importance of managers supporting organisational changes, especially concerning IT knowledge, in-house and external training related to smart technology to reduce uncertainty, and increase participation in developing the right solutions for the SME (Ghobakhloo and Fathi Citation2020). Our updated model of determinants depicted in Figure offers a scaffold for companies to strategically approach industry 4.0, and in a human-centric manner, by focusing on the enablers of acquiring smart manufacturing technology – an operational direction and scaffold absent from the technical reference architecture models from Table . This leads to our second proposition:
Proposition 2
A lack of foundational prior knowledge leads to knowledge inertia, which explains lack of progress regarding digitalisation observed in manufacturing SMEs.
Lastly, on ways to combat knowledge inertia, Liao, Fei, and Liu (Citation2008) suggest that companies should actively encourage new methods and ideas, which have been shown to form through governance development and support programs i.e. interactive research (Ellström et al. Citation2020) or engaged scholarship (Van De Ven and Johnson Citation2006).
Over the course of three years, we engaged multiple representatives from each SME in four engagements lasting three-four months each, providing them material on industry 4.0 and hands-on experience with smart manufacturing technologies to create a common language and inspire strategic thinking related to industry 4.0 through digital vision and maturity workshops. Despite receiving such initial help, the SMEs struggled to independently focus on the smart technology dependency themes and would benefit from close support and facilitation suitable to their current situation, as shown by Saabye, Kristensen, and Wæhrens (Citation2022), especially in the areas highlighted in the model of determinants, supported by our aggregate dimensions and extant literature (see Figure ). They have yet to reach the event horizon (i.e. the point of no return), where they leave knowledge inertia behind and become self-sufficient enough to identify value-adding technology, manage competence development and pursue digital transformation towards industry 4.0. Competence development highly relies on the management and their awareness about which competencies are relevant and that managers understand the importance of providing the right environment to learn (Saabye, Kristensen, and Wæhrens Citation2022). Thus, managers also need knowledge and competencies about how to lead a digital transformation (Hecklau et al. Citation2016; Shet and Pereira Citation2021), e.g. competencies within project leadership, design thinking, collaborative mindset, and data analytics, inter alia (Shet and Pereira Citation2021). Finding a suitable path to increased digitalisation for SMEs may then be realised through competent facilitation, sparring, and a focus on maturing the prior knowledge in level 0. This leads to our third proposition:
Proposition 3
Technology investment alone is not enough to succeed with digitalisation. A supportive scaffold to help with the facilitation of digitalisation initiatives is needed to escape the knowledge inertia present in SMEs and help guide strategies for competence development in parallel with operational technology implementation.
Such support could arrive from consortia to help provide detailed documentation on technical reference architectures while making training material easily accessible and up to date for companies (Nakagawa et al. Citation2021). Ellström et al. (Citation2020) report promising results from a type of consortium that acts as an intermediary knowledge creation centre and a broker between practitioners and researchers.
Ten years on from the initial conceptualisation of industry 4.0, the importance of human-centred solutions and employee involvement are becoming evident, e.g. as shown in Kaasinen et al. (Citation2020) and Rangraz and Pareto (Citation2021), and in the thinking around industry 5.0 (Breque, Nul, and Petridis Citation2021; Xu et al. Citation2021). SMEs have significant hurdles when it comes to digitalisation, yet great opportunities as their culture and smaller size often afford greater employee involvement and collaboration (Horváth and Szabó Citation2019). If they can overcome their technical hurdles and keep their inclusive culture, they are on a path towards industry 5.0 – a human-centric, sustainable, and resilient production.
5.1. Research perspectives
Future research directions should include in-depth case studies of SMEs who have managed to overcome the challenges outlined in this paper to obtain a deeper knowledge of the proposed dimensions, approaches, and barriers (Flyvbjerg Citation2006). We call for more research on how to effectively create content knowledge and meta-cognitive knowledge to support competencies for smart manufacturing both on the management and shop-floor levels. Additionally, exemplary cases could be used to verify propositions and the effect of providing a proper knowledge foundation on digitalisation progress. While we found that the main barriers for SMEs were other ones than commonly known barriers such as financial and managerial support, openness to change, and operations technology maturity, different patterns may emerge in other cultural contexts and economies compared to Denmark. This study was made possible through the Innovation Factory North research program, which was an opportunity for SMEs with limited human and financial resources to get started with digital transformation while providing valuable insights for research. More research is needed to determine the most effective setups combining industry and academia – not only to raise awareness of digital transformation but also to learn more about which human and organisational factors both hinder and enable digital transformation.
6. Conclusion
The industry 4.0 paradigm still captures the attention of manufacturing and small- and medium-sized enterprises (SMEs) are eager to get on board. However, it appears difficult for them to successfully perform a digital transformation in line with the industry 4.0 vision.
Our study, based on a sample of 30 SMEs, reveals that important determinants for digitalisation are overlooked, often leading back to an experienced lack of knowledge of opportunities and competencies. SMEs struggle across all levels of the original model of determinants for smart technology adoption. We suggest that a level 0 determinant stage exists that should serve to mature the companies and allow them to engage effectively with the levels identified by Ghobakhloo (Citation2020). Level 0 should target content knowledge related to general industry 4.0 concepts, opportunities through specific data handling methods, and integration between digital systems. Additionally, level 0 should target meta-cognitive knowledge related to strategic management of digital technologies and reflections on how to identify and operationalise competence development strategically moving forwards.
This ties into what is known about prior knowledge (Dochy, de Rijdt, and Dyck Citation2002) and knowledge inertia (Liao, Fei, and Liu Citation2008): SMEs need to expand and leverage existing knowledge to advance digitalisation while actively acquiring new knowledge to avoid hindering their own innovation. By expanding Ghobakhloo's (2020) model, our study highlights the crucial role of a determinant level 0 in addressing the knowledge gap for SMEs aiming to adopt industry 4.0 production systems.
The addition of a level 0 in the model of determinants forces researchers to consider how a suitable knowledge foundation can be fostered in manufacturing enterprises, emphasising the human-centred aspects equally (if not higher) than technology-driven aspects. This corresponds with the findings from Estensoro et al. (Citation2022), who emphasise that resources have to be present as pre-conditions towards industry 4.0. Additionally, Mittal et al. (Citation2018) investigated existing maturity and readiness assessment models for industry 4.0 through a structured literature review and found that such models were often more suitable for larger multinational enterprises. The models start on a level already too advanced for SMEs and there is a need for a level 0, which corresponds more closely to the reality of SMEs (Mittal et al. Citation2018). In this paper, we have specified which knowledge areas are especially important to act as industry 4.0 pre-conditions (i.e. level 0) for SMEs based on empirical evidence of 30 SMEs. However, where Mittal et al. (Citation2020) find the need for SMEs to develop awareness of smart manufacturing after having identified manufacturing data, we argue that awareness needs to be created earlier in the process as our sample of SMEs expressed great uncertainty in both data utilisation and integration capabilities, making it difficult for them to make informed decisions surrounding production data. Additionally, our results back up the recommendation by Ellström et al. (Citation2020); Estensoro et al. (Citation2022), and Saabye, Kristensen, and Wæhrens (Citation2022) for companies to collaborate with policymakers, and experts (or managers) as facilitators through different development stages and close prolonged engagements.
This study makes four key contributions, which materialise in three propositions and an expanded model of determinants for smart technology adoption. In summation, the propositions state that:
SMEs need a knowledge starting point before tackling later stages towards digitalisation: this includes content knowledge (what) and meta-cognitive knowledge (how).
A lack of foundational prior knowledge leads to knowledge inertia, which explains lack of progress regarding digitalisation observed in manufacturing SMEs.
Technology investments are not enough for digitalisation. Competence development strategies should be developed in parallel with operational technology implementation, preferably through structured facilitation (e.g. a scaffolding approach.
Practically, managers in manufacturing enterprises can utilise the model of determinants as a process guide for structuring their digitalisation efforts, ensuring that crucial aspects like competence development are not overlooked. Our findings underline the importance of closer collaboration between manufacturing SMEs and supportive digitalisation programs, which can facilitate knowledge creation. This necessitates proactive involvement from policymakers in initiating and incentivising such engagements.
Supplemental Material
Download PDF (208.9 KB)Disclosure statement
No potential conflict of interest was reported by the author(s).
Disclosure and data availability statement
The authors declare no potential conflicts of interest. The data supporting this study's findings are available from the corresponding author, Andreas Kornmaaler Hansen, upon reasonable request.
Correction Statement
This article has been corrected with minor changes. These changes do not impact the academic content of the article.
Additional information
Funding
Notes on contributors
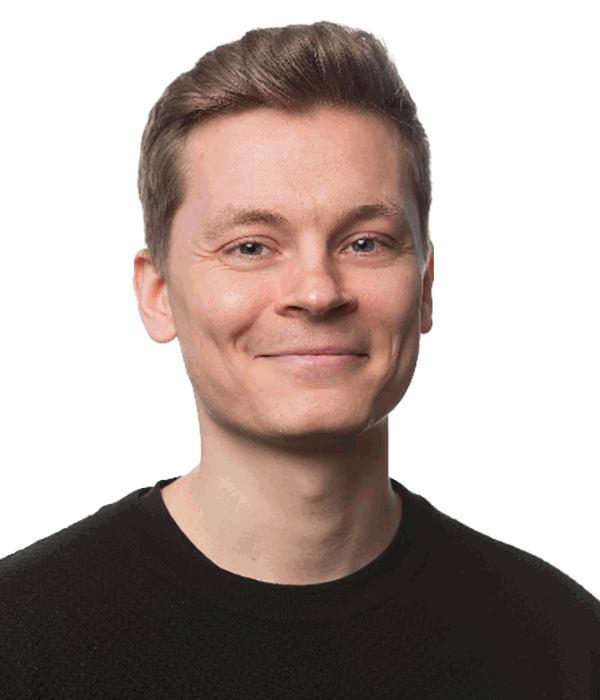
Andreas Kornmaaler Hansen
Andreas Kornmaaler Hansen is a PhD fellow at the University of Aalborg, Denmark, where he received both his BSc. and MSc. in engineering psychology in 2017 and 2019 respectively. He focuses mainly on small and medium-sized companies. His research interests include human-centric digital transformation of manufacturing companies, competence development, Industry 4.0/5.0, and human-robot interaction.
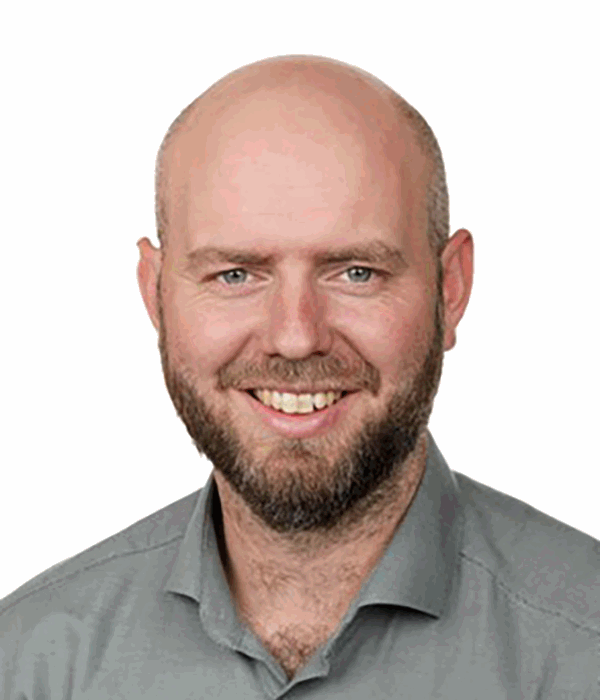
Lasse Christiansen
Dr. Lasse Christiansen is a Postdoc at Aalborg University, Denmark, Department of Materials and Production and a Docent at the University College of Northern Denmark. Lasse performs research within the areas of digital transformation and upskilling with a focus on enterprises, the workforce, and digital technologies. He has also published several academic books on such topics. He received his PhD in material science and uses his technological background to collaborate with companies and manufacturing professionals in his research.
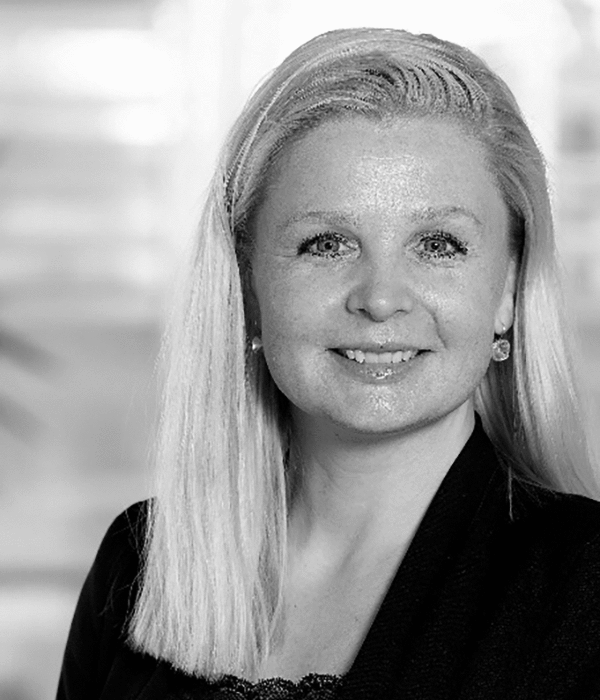
Astrid Heidemann Lassen
Dr. Astrid Heidemann Lassen is Associate Professor in Operations and Innovation Management at Aalborg University, Denmark, Department of Materials and Production. Astrid is Head of Section in Production and Vice Head of Department. Since 2015 she has been Honorary Visiting Professor at the Unit for Innovation and Entrepreneurship at University of Gothenburg, Sweden. Astrid is the author/co-author of 100+ international journal and conference articles on the topics of digital transformation, innovation management, and knowledge intensive entrepreneurship. She has also published several academic books on such topics. Her research focus is in particular on the process of developing organizational capabilities for innovation and digital transformation in the context of both established companies and knowledge intensive entrepreneurial ventures.
Notes
1 The authors note that we use the term industry 4.0 and smart manufacturing interchangeably throughout the article. In our view, the meaning of the two terms generally refers to the same topic and may help reach a broader audience by doing so.
References
- Ananiadou, Katerina, and Magdalean Claro. 2009. 21st Century Skills and Competences for New Millennium Learners in OECD Countries. Technical Report 41. OECD.
- Antonino, Pablo Oliveira, Frank Schnicke, Zai Zhang, and Thomas Kuhn. 2019. “Blueprints for Architecture Drivers and Architecture Solutions for Industry 4.0 Shopfloor Applications.” In Proceedings of the 13th European Conference on Software Architecture – Volume 2, ECSA '19, 261–268, New York, NY, USA: Association for Computing Machinery. https://doi.org/10.1145/3344948.3344971.
- Belinski, Ricardo, Adriana M M Peixe, Guilherme F Frederico, and Jose Arturo Garza-reyes. 2020. “Organizational Learning and Industry 4. 0 : Findings From a Systematic Literature Review and Research Agenda.” Benchmarking: An International Journal 27 (8): 2435–2457. https://doi.org/10.1108/BIJ-04-2020-0158.
- Breque, M, L. D.e Nul, and A Petridis. 2021. Industry 5.0: Towards a Sustainable, Human-centric and Resilient European Industry. Luxembourg, LU: European Commission, Directorate-General for Research and Innovation.
- Buer, Sven-Vegard, Jo Wessel Strandhagen, Marco Semini, and Jan Ola Strandhagen. 2020. “The Digitalization of Manufacturing: Investigating the Impact of Production Environment and Company Size.” Journal of Manufacturing Technology Management 32 (3): 621–645. Accessed 2023-03-02. https://doi.org/10.1108/JMTM-05-2019-0174/full/html.
- Capestro, Mauro, and Steffen Kinkel. 2020. “Industry 4.0 and Knowledge Management: A Review of Empirical Studies.” In Knowledge Management and Industry 4.0: New Paradigms for Value Creation, edited by Marco Bettiol, Eleonora Di Maria, and Stefano Micelli, Knowledge Management and Organizational Learning, 19–52. Cham: Springer International Publishing. Accessed 2022-07-04. https://doi.org/10.1007/978-3-030-43589-9_2.
- Cirillo, Valeria, Lucrezia Fanti, Andrea Mina, and Andrea Ricci. 2023. “The Adoption of Digital Technologies: Investment, Skills, Work Organisation.” Structural Change and Economic Dynamics66:89–105. Accessed 2023-08-02. https://linkinghub.elsevier.com/retrieve/pii/S0954349X23000577. https://doi.org/10.1016/j.strueco.2023.04.011.
- Colli, M., U. Berger, M. Bockholt, O. Madsen, C. Møller, and B. Vejrum Wæhrens. 2019. “A Maturity Assessment Approach for Conceiving Context-specific Roadmaps in the Industry 4.0 Era.” Annual Reviews in Control 48:165–177. https://www.sciencedirect.com/science/article/pii/S1367578819300409. https://doi.org/10.1016/j.arcontrol.2019.06.001.
- Collins, Allan, and Manu Kapur. 2014. “Cognitive Apprenticeship.” In The Cambridge Handbook of the Learning Sciences, edited by R. Keith Sawyer, 2nd ed., Cambridge Handbooks in Psychology, 109–127. Cambridge University Press.
- Cugno, Monica, Rebecca Castagnoli, and Giacomo Büchi. 2021. “Openness to Industry 4.0 and Performance: The Impact of Barriers and Incentives.” Technological Forecasting and Social Change168:120756. https://doi.org/10.1016/j.techfore.2021.120756.
- Dochy, Filip, Catherine de Rijdt, and Walter Dyck. 2002. “Cognitive Prerequisites and Learning: How Far Have We Progressed Since Bloom? Implications for Educational Practice and Teaching.” Active Learning in Higher Education 3 (3): 265–284. https://doi.org/10.1177/1469787402003003006.
- Ellström, Per-Erik, Mattias Elg, Andreas Wallo, Martina Berglund, and Henrik Kock. 2020. “Interactive Research: Concepts, Contributions and Challenges.” Journal of Manufacturing Technology Management 31 (8): 1517–1537. https://doi.org/10.1108/JMTM-09-2018-0304.
- Estensoro, Miren, Miren Larrea, Julian M. Müller, and Eduardo Sisti. 2022. “A Resource-based View on SMEs Regarding the Transition to More Sophisticated Stages of Industry 4.0.” European Management Journal40 (5): 778–792. Accessed 2023-03-02. https://linkinghub.elsevier.com/retrieve/pii/S0263237321001444. https://doi.org/10.1016/j.emj.2021.10.001.
- European Commission, 2003. “Commission Recommendation of 6 May 2003 concerning the definition of micro, small and medium-sized enterprises.” Accessed 2023-04-12.
- Fareri, Silvia, Gualtiero Fantoni, Filippo Chiarello, Elena Coli, and Anna Binda. 2020. “Estimating Industry 4.0 Impact on Job Profiles and Skills Using Text Mining.” Computers in Industry 118:103222. https://doi.org/10.1016/j.compind.2020.103222.
- Flores, Emmanuel, Xun Xu, and Yuqian Lu. 2020. “Human Capital 4.0: a Workforce Competence Typology for Industry 4.0.” Journal of Manufacturing Technology Management 31 (4): 687–703. https://doi.org/10.1108/JMTM-08-2019-0309.
- Flyvbjerg, Bent. 2006. “Five Misunderstandings About Case-study Research.” Qualitative Inquiry 12 (2): 219–245. https://doi.org/10.1177/1077800405284363.
- Ghobakhloo, Morteza. 2020. “Determinants of Information and Digital Technology Implementation for Smart Manufacturing.” International Journal of Production Research 58 (8): 2384–2405. https://doi.org/10.1080/00207543.2019.1630775.
- Ghobakhloo, Morteza, and Ng Tan Ching. 2019. “Adoption of Digital Technologies of Smart Manufacturing in SMEs.” Journal of Industrial Information Integration 16:100107. Accessed 2023-08-07. https://www.sciencedirect.com/science/article/pii/S2452414X19300020. https://doi.org/10.1016/j.jii.2019.100107.
- Ghobakhloo, Morteza, and Masood Fathi. 2020. “Corporate Survival in Industry 4.0 Era: the Enabling Role of Lean-digitized Manufacturing.” Journal of Manufacturing Technology Management 31 (1): 1–30. https://doi.org/10.1108/JMTM-11-2018-0417.
- Gioia, Dennis A, and Kumar Chittipeddi. 1991. “Sensemaking and Sensegiving in Strategic Change Initiation.” Strategic Management Journal 12 (6): 433–448. https://doi.org/10.1002/smj.v12:6.
- Gioia, Dennis A, Kevin G Corley, and Aimee L Hamilton. 2013. “Seeking Qualitative Rigor in Inductive Research: Notes on the Gioia Methodology.” Organizational Research Methods 16 (1): 15–31. https://doi.org/10.1177/1094428112452151.
- Gorgels, Stefan, Priem Maximilian, Blagoeva Tsvetelina, Martinelle Agnèss, and Milanesi Giulio, European Commission. 2022. Annual report on European SMEs 2021/2022 : SMEs and environmental sustainability. Publications Office of the European Union.
- Grosse, Eric H., Fabio Sgarbossa, Cecilia Berlin, and W. Patrick Neumann. 2023. “Human-centric Production and Logistics System Design and Management: Transitioning From Industry 4.0 to Industry 5.0.” International Journal of Production Research 61 (22): 7749–7759. Publisher: Taylor & Francis _eprint: Accessed 2023-10-19. https://doi.org/10.1080/00207543.2023.2246783.
- Hankel, Martin, and Bosch Rexroth. 2015. The reference architectural model industrie 4.0 (RAMI 4.0). Technical Report 1. ZVEI. Accessed 2023-06-06.
- Hansen, Svend Aage, and Poul Kyvsgaard Hansen. 2023. “Differences Between Small and Medium Sized Companies When Realizing Smart Production – Experiences from Northwest Smart Production Program in Denmark.” In The Future of Smart Production for SMEs: A Methodological and Practical Approach Towards Digitalization in SMEs, edited by Ole Madsen, Ulrich Berger, Charles Møller, Astrid Heidemann Lassen, Brian Vejrum Waehrens, and Casper Schou, 101–111. Cham: Springer International Publishing. Accessed 2023-10-19. https://doi.org/10.1007/978-3-031-15428-7_9.
- Hecklau, Fabian, Mila Galeitzke, Sebastian Flachs, and Holger Kohl. 2016. “Holistic Approach for Human Resource Management in Industry 4.0.” Procedia CIRP 54:1–6. http://dx.doi.org/10.1016/j.procir.2016.05.102.
- Horváth, Dóra, and Roland Zs. Szabó. 2019. “Driving Forces and Barriers of Industry 4.0: Do Multinational and Small and Medium-sized Companies Have Equal Opportunities?” Technological Forecasting and Social Change 146: 119–132. https://www.sciencedirect.com/science/article/pii/S0040162518315737.
- IBM, 2017. “Industry 4.0 reference architecture.” Accessed 2023-05-04. https://www.ibm.com/cloud/architecture/architectures/industry-40/reference-architecture.
- IVCI, 2018. IVRA Next: Strategic implementation framework of industrial value chain for connected industries. Technical Report. Industrial Value Chain Initiative. Accessed 2023-05-03. https://iv-i.org/wp-content/uploads/2018/04/IVRA-Next_en.pdf.
- Jerman, Andrej, Mirjana Pejić Bach, and Andrej Bertoncelj. 2018. “A Bibliometric and Topic Analysis on Future Competences At Smart Factories.” Machines 6 (3): 1–13. https://doi.org/10.3390/machines6030041.
- Kaasinen, Eija, Franziska Schmalfuß, Cemalettin Özturk, Susanna Aromaa, Menouer Boubekeur, Juhani Heilala, and Päivi Heikkilä, et al. 2020. “Empowering and engaging industrial workers with Operator 4.0 solutions.” Computers and Industrial Engineering 139 (January 2019). Publisher: Elsevier.
- Kadir, Bzhwen A., and Ole Broberg. 2021. “Human-centered Design of Work Systems in the Transition to Industry 4.0.” Applied Ergonomics 92:103334. https://www.sciencedirect.com/science/article/pii/S0003687020302829. https://doi.org/10.1016/j.apergo.2020.103334.
- Kagermann, Henning, Wolfgang Wahlster, Johannes Helbig, and Ariane Hellinger. 2013. Recommendations for Implementing the Strategic Initiative Industrie 4.0: Final Report of the Industrie 4.0 Working Group. Germany: Acatech-National Academy of Science and Engineering.
- Kamble, Sachin S., Angappa Gunasekaran, and Rohit Sharma. 2018. “Analysis of the Driving and Dependence Power of Barriers to Adopt Industry 4.0 in Indian Manufacturing Industry.” Computers in Industry 101:107–119. https://www.sciencedirect.com/science/article/pii/S0166361518301453. https://doi.org/10.1016/j.compind.2018.06.004.
- Kassner, Laura, Christoph Gröger, Jan Königsberger, Eva Hoos, Cornelia Kiefer, Christian Weber, Stefan Silcher, and Bernhard Mitschang. 2017. “The Stuttgart IT Architecture for Manufacturing.” In Enterprise Information Systems, edited by Slimane Hammoudi, Leszek A. Maciaszek, Michele M. Missikoff, Olivier Camp, and José Cordeiro, Cham, 53–80. Springer International Publishing.
- Ketokivi, Mikko, and Thomas Choi. 2014. “Renaissance of Case Research As a Scientific Method.” Journal of Operations Management 32 (5): 232–240. https://doi.org/10.1016/j.jom.2014.03.004.
- Kipper, Liane Mahlmann, Sandra Iepsen, Ana Julia Dal Forno, Rejane Frozza, Leonardo Furstenau, Jéssica Agnes, and Danielli Cossul. 2021. “Scientific Mapping to Identify Competencies Required by Industry 4.0.” Technology in Society 64:101454. https://doi.org/10.1016/j.techsoc.2020.101454.
- Kusiak, Andrew. 2018. “Smart Manufacturing.” International Journal of Production Research 56 (1-2): 508–517. https://doi.org/10.1080/00207543.2017.1351644.
- Larsen, Maria Stoettrup Schioenning, Mats Magnusson, and Astrid Heidemann Lassen. 2022. “Industry 4.0 Holds a Great Potential for Manufacturers, So Why haven't They Started?” In Towards Sustainable Customization: Bridging Smart Products and Manufacturing Systems, edited by Ann-Louise Andersen, Rasmus Andersen, Thomas Ditlev Brunoe, Maria Stoettrup Schioenning Larsen, Kjeld Nielsen, Alessia Napoleone, and Stefan Kjeldgaard, Cham, 721–729. Springer International Publishing.
- Lassen, Astrid Heidemann, Andreas Kornmaaler Hansen, Daniel Grud Hellerup Sørensen, and Maria Stoettrup Schioenning Larsen. 2023. “Labour 4.0: How is the Workforce Prepared for the Future of Manufacturing Industries?” In The Future of Smart Production for SMEs: A Methodological and Practical Approach Towards Digitalization in SMEs, edited by Ole Madsen, Ulrich Berger, Charles Møller, Astrid Heidemann Lassen, Brian Vejrum Waehrens, and Casper Schou, 391–403. Cham: Springer International Publishing. https://doi.org/10.1007/978-3-031-15428-7_36.
- Lassen, Astrid Heidemann, and Brian Vejrum Waehrens. 2021. “Labour 4.0: Developing Competences for Smart Production.” Journal of Global Operations and Strategic Sourcing 14 (4): 659–679. https://doi.org/10.1108/JGOSS-11-2019-0064.
- Lee, Jay, Behrad Bagheri, and Hung-An Kao. 2015. “A Cyber-Physical Systems Architecture for Industry 4.0-based Manufacturing Systems.” Manufacturing Letters 3:18–23. https://www.sciencedirect.com/science/article/pii/S221384631400025X. https://doi.org/10.1016/j.mfglet.2014.12.001.
- Liao, Shu-hsien, Wu-Chen Fei, and Chih-Tang Liu. 2008. “Relationships Between Knowledge Inertia, Organizational Learning and Organization Innovation.” Technovation 28 (4): 183–195. https://www.sciencedirect.com/science/article/pii/S0166497207001502. https://doi.org/10.1016/j.technovation.2007.11.005.
- Liboni, Lara Bartocci, Luciana Oranges Cezarino, Charbel José Chiappetta Jabbour, Bruno Garcia Oliveira, and Nelson Oliveira Stefanelli. 2019. “Smart Industry and the Pathways to HRM 4.0: Implications for SCM.” Supply Chain Management: An International Journal 24 (1): 124–146. https://doi.org/10.1108/SCM-03-2018-0150.
- Lin, Shi-Wan, Eric Simmon, Daniel Young, Bradford Miller, Jasques Durand, Graham Bleakly, Amine Chigani, et al. 2022. The industrial internet reference architecture: An industry IoT consortium foundational document (Version 1.10). Technical Report V1.10. Industry IoT Consortium (IIC).
- Lundgren, Camilla, Cecilia Berlin, Anders Skoogh, and Anders Källström. 2022. “How industrial maintenance managers perceive socio-technical changes in leadership in the Industry 4.0 context.” International Journal of Production Research 1–20. Accessed 2023-01-05. https://doi.org/10.1080/00207543.2022.2101031.
- Mahmood, Kashif, Tauno Otto, Jesper H Kristensen, Astrid Heidemann Lassen, Thomas D Brunoe, Casper Schou, Lasse Christiansen, and Esben Skov Laursen. 2021. “Analysis of Industry 4.0 Capabilities: A Perspective of Educational Institutions and Needs of Industry.” In Towards Sustainable Customization: Bridging Smart Products and Manufacturing Systems, 887–894. Springer.
- Masood, Tariq, and Paul Sonntag. 2020. “Industry 4.0: Adoption Challenges and Benefits for SMEs.” Computers in Industry121:103261. Accessed 2023-03-02. https://linkinghub.elsevier.com/retrieve/pii/S0166361520304954. https://doi.org/10.1016/j.compind.2020.103261.
- Matt, Dominik T., Guido Orzes, Erwin Rauch, and Patrick Dallasega. 2020. “Urban Production – A Socially Sustainable Factory Concept to Overcome Shortcomings of Qualified Workers in Smart SMEs.” Computers & Industrial Engineering139:105384. Accessed 2023-03-02. https://linkinghub.elsevier.com/retrieve/pii/S0360835218304157. https://doi.org/10.1016/j.cie.2018.08.035.
- Mittal, Sameer, Muztoba Ahmad Khan, Jayant Kishor Purohit, Karan Menon, David Romero, and Thorsten Wuest. 2020. “A Smart Manufacturing Adoption Framework for SMEs.” International Journal of Production Research 58 (5): 1555–1573. https://doi.org/10.1080/00207543.2019.1661540.
- Mittal, Sameer, Muztoba Ahmad Khan, David Romero, and Thorsten Wuest. 2018. “A Critical Review of Smart Manufacturing & Industry 4.0 Maturity Models: Implications for Small and Medium-sized Enterprises (SMEs).” Journal of Manufacturing Systems 49:194–214. Accessed 2023-05-03. https://www.sciencedirect.com/science/article/pii/S0278612518301341. https://doi.org/10.1016/j.jmsy.2018.10.005.
- Moeuf, Alexandre, Samir Lamouri, Robert Pellerin, Simon Tamayo-Giraldo, Estefania Tobon-Valencia, and Romain Eburdy. 2020. “Identification of Critical Success Factors, Risks and Opportunities of Industry 4.0 in SMEs.” International Journal of Production Research 58 (5): 1384–1400. Publisher: Taylor & Francis. https://doi.org/10.1080/00207543.2019.1636323.
- Moldovan, Liviu. 2019. “State-of-the-art Analysis on the Knowledge and Skills Gaps on the Topic of Industry 4.0 and the Requirements for Work-based Learning.” Procedia Manufacturing 32:294–301. 12th International Conference Interdisciplinarity in Engineering, INTER-ENG 2018, 4–5 October 2018, Tirgu Mures, Romania, https://www.sciencedirect.com/science/article/pii/S2351978919302525. https://doi.org/10.1016/j.promfg.2019.02.217.
- Møller, Julian M. 2019. “Assessing the Barriers to Industry 4.0 Implementation From a Workers' Perspective.” IFAC-PapersOnLine 52 (13): 2189–2194. Accessed 2023-03-02. https://linkinghub.elsevier.com/retrieve/pii/S2405896319315149. https://doi.org/10.1016/j.ifacol.2019.11.530.
- Møller, Charles, Andreas Kornmaaler Hansen, Dan Palade, Daniel Grud Hellerup Sørensen, Emil Blixt Hansen, Jonas Nygaard Uhrenholt, and Maria Stoettrup Schioenning Larsen. 2023. “Innovation Factory North: An Approach to Make Small and Medium Sized Manufacturing Companies Smarter.” In The Future of Smart Production for SMEs: A Methodological and Practical Approach Towards Digitalization in SMEs, edited by Ole Madsen, Ulrich Berger, Charles Møller, Astrid Heidemann Lassen, Brian Vejrum Waehrens, and Casper Schou, 113–126. Cham: Springer International Publishing. https://doi.org/10.1007/978-3-031-15428-7_10.
- Motyl, Barbara, Gabriele Baronio, Stefano Uberti, Domenico Speranza, and Stefano Filippi. 2017. “How Will Change the Future Engineers' Skills in the Industry 4.0 Framework? A Questionnaire Survey.” Procedia Manufacturing 11:1501–1509. https://doi.org/10.1016/j.promfg.2017.07.282.
- Nakagawa, Elisa Yumi, Pablo Oliveira Antonino, Frank Schnicke, Rafael Capilla, Thomas Kuhn, and Peter Liggesmeyer. 2021. “Industry 4.0 Reference Architectures: State of the Art and Future Trends.” Computers & Industrial Engineering 156:107241. https://www.sciencedirect.com/science/article/pii/S0360835221001455. https://doi.org/10.1016/j.cie.2021.107241.
- Neumann, W. Patrick, Sven Winkelhaus, Eric H. Grosse, and Christoph H. Glock. 2021. “Industry 4.0 and the Human Factor – A Systems Framework and Analysis Methodology for Successful Development.” International Journal of Production Economics 233:107992. https://doi.org/10.1016/j.ijpe.2020.107992.
- NVivo, 2022. Accessed 2022-11-11. https://www.qsrinternational.com/nvivo-qualitative-data-analysis-software/home.
- Pivoto, Diego G. S., Luiz F. F. de Almeida, Rodrigo da Rosa Righi, Joel J. P. C. Rodrigues, Alexandre Baratella Lugli, and Antonio M. Alberti. 2021. “Cyber-physical Systems Architectures for Industrial Internet of Things Applications in Industry 4.0: A Literature Review.” Journal of Manufacturing Systems 58 (PA): 176–192. https://doi.org/10.1016/j.jmsy.2020.11.017.
- Pratt, Michael G., Sarah Kaplan, and Richard Whittington. 2020. “Editorial Essay: The Tumult Over Transparency: Decoupling Transparency From Replication in Establishing Trustworthy Qualitative Research.” Administrative Science Quarterly 65 (1): 1–19. https://doi.org/10.1177/0001839219887663.
- Rangraz, Masood, and Lena Pareto. 2021. “Workplace Work-integrated Learning: Supporting Industry 4.0 Transformation for Small Manufacturing Plants by Reskilling Staff.” International Journal of Lifelong Education 40 (1): 5–22. https://doi.org/10.1080/02601370.2020.1867249.
- Resman, Matevž, Miha Pipan, Marco Šimic, and Niko Herakovič. 2019. “A New Architecture Model for Smart Manufacturing: A Performance Analysis and Comparison with the RAMI 4.0 Reference Model.” Adv. Prod. Eng. Manag 14 (2): 153–165.
- Saabye, Henrik, Thomas Borup Kristensen, and Brian Vejrum Wæhrens. 2022. “Developing a Learning-to-learn Capability: Insights on Conditions for Industry 4.0 Adoption.” International Journal of Operations & Production Management42 (13): 25–53. https://doi.org/10.1108/IJOPM-07-2021-0428.
- Sharpe, Richard, Katherine van Lopik, Aaron Neal, Paul Goodall, Paul P. Conway, and Andrew A. West. 2019. “An Industrial Evaluation of An Industry 4.0 Reference Architecture Demonstrating the Need for the Inclusion of Security and Human Components.” Computers in Industry 108:37–44. https://www.sciencedirect.com/science/article/pii/S0166361518304950. https://doi.org/10.1016/j.compind.2019.02.007.
- Shet, Sateesh V., and Vijay Pereira. 2021. “Proposed Managerial Competencies for Industry 4.0 – Implications for Social Sustainability.” Technological Forecasting and Social Change 173:121080. https://doi.org/10.1016/j.techfore.2021.121080.
- Stentoft, Jan, Kent Adsbøll Wickstrøm, Kristian Philipsen, and Anders Haug. 2021. “Drivers and Barriers for Industry 4.0 Readiness and Practice: Empirical Evidence From Small and Medium-sized Manufacturers.” Production Planning & Control 32 (10): 811–828. Accessed 2023-03-02. https://doi.org/10.1080/09537287.2020.1768318.
- Szász, Levente, Krisztina Demeter, Béla-Gergely Rácz, and Dávid Losonci. 2020. “Industry 4.0: a Review and Analysis of Contingency and Performance Effects.” Journal of Manufacturing Technology Management 32 (3): 667–694. Publisher: Emerald Publishing Limited, Accessed 2023-08-08. https://doi.org/10.1108/JMTM-10-2019-0371.
- Tortorella, Guilherme, Rogério Miorando, Rodrigo Caiado, Daniel Nascimento, and Alberto Portioli Staudacher. 2021. “The Mediating Effect of Employees' Involvement on the Relationship Between Industry 4.0 and Operational Performance Improvement.” Total Quality Management & Business Excellence 32 (1-2): 119–133. Accessed 2023-03-02. https://doi.org/10.1080/14783363.2018.1532789.
- Van De Ven, Andrew H., and Paul E. Johnson. 2006. “Knowledge for Theory and Practice.” Academy of Management Review 31 (4): 802–821. Accessed 2023-01-05. https://doi.org/10.5465/amr.2006.22527385.
- Voss, Chris, Mark Johnson, and Jan Godsell. 2016. “Case Research.” In Research Methods for Operations Management, 165–197. Cham: Springer International Publishing.
- Xu, Xun, Yuqian Lu, Birgit Vogel-Heuser, and Lihui Wang. 2021. “Industry 4.0 and Industry 5.0 – Inception, Conception and Perception.” Journal of Manufacturing Systems 61:530–535. https://doi.org/10.1016/j.jmsy.2021.10.006.
- Xu, Li Da, Eric L. Xu, and Ling Li. 2018. “Industry 4.0: State of the Art and Future Trends.” International Journal of Production Research 56 (8): 2941–2962. https://doi.org/10.1080/00207543.2018.1444806.