Abstract
Digital Twins (DTs) hold significant promise in addressing the challenges faced by food supply chains (FSCs). This paper aims to provide critical insights into the potential for Digital Twins to meet the key challenges of the FSC and establish a comprehensive conceptual framework for their implementation. Following a systematic literature review (SLR), the study identified 81 peer-reviewed, high-quality papers published over the last decade (2012–2023). The typology-driven thematic analysis emphasises the emergent nature of DTs within FSCs, highlighting their key characteristics including monitoring, real-time simulation, and scenario analysis. The identified characteristics, applications, implementation drivers and barriers of Digital Twin form the basis for a novel conceptual framework for implementing DTs in FSCs. Leveraging insights from Innovation Adoption Theory and the Technology-Organization-Environment (TOE) framework, the study outlines a structured five step implementation process divided into three stages. Notably, technology assessment and performance evaluation emerge as two innovative steps necessary for the successful implementation of DTs specifically, not previously considered by the theory. The study identifies promising avenues for future research. These findings provide invaluable guidance to researchers and practitioners seeking to embrace the potential of Digital Twin within the food industry.
1. Introduction
The global food industry faces significant challenges around quality, waste, safety and security (Casino et al. Citation2021; Pylianidis, Osinga, and Athanasiadis Citation2021). In recent times, food safety scandals have endangered people’s health, bringing economic losses and incurring a crisis of consumer trust (Zhang et al. Citation2016). In addition, the dual challenges of short product shelf life and recent supply shortages make food supply chains (FSCs) distinctive and difficult to manage (La Scalia et al. Citation2016). The food supply chain has unique features compared with the general supply chain. Firstly, many food products are perishable, having a limited shelf life compared to durable goods and requiring cold chain management and timely transportation (Ahumada and Villalobos Citation2009). Secondly, to ensure food quality and safety food products often have specific storage and processing requirements, such as temperature, humidity, and handling (Salin Citation1998). Furthermore, food products often undergo more transformations (e.g. slaughtering, preservation, cooking, and packaging) compared to conventionally manufactured goods, increasing the complexity of the food supply chain (Manzini and Accorsi Citation2013). Thirdly, to ensure food safety food products require end-to-end traceability, which is critical for managing food recalls (Regattieri, Gamberi, and Manzini Citation2007; Tian et al. Citation2016). Recent technological advancements offer stakeholders such as farmers (e.g. Ghandar et al. Citation2021), food processors (e.g. Maheshwari et al. Citation2022), distributors (e.g. Onwude et al. Citation2022), and retailers (e.g. Burgos and Ivanov Citation2021) opportunities to identify bottlenecks, seek solutions and optimise their food supply chain operations to alleviate some of the FSC’s challenges (Alfian et al. Citation2020; Garaus and Treiblmaier Citation2021).
Industry 4.0 technologies, such as the Internet of Things (IoT), Blockchain, Artificial Intelligence, and Big Data Analytics (BDA), have been widely adopted (Davis et al. Citation2012; Zhao et al. Citation2019; Zhao et al. Citation2021a; Malik et al. Citation2021). This has included adopting these technologies in the food industry, such as in farming (e.g. Jans-Singh et al. Citation2020; Ghandar et al. Citation2021), logistics (e.g. Defraeye et al. Citation2019; Shoji et al. Citation2022b) and warehousing (e.g. Korth, Schwede, and Zajac Citation2018). One such technology is the Digital Twin (DT), which in a broad sense, is a digital replica of the physical environment capturing real-time information for decision-making (Janssen et al. Citation2017). Due to the development of Industry 4.0 enabling technologies, such as sensors, IoT, cloud computing, big data analytics, and augmented and virtual reality, Digital Twins have seen widespread adoption across a number of industry sectors (Ivanov and Dolgui Citation2021).
Digital Twins show a strong potential to solve some of the FSC’s fundamental issues. They can be used to build end-to-end FSC visibility and enhance FSC resilience (Melesse, Di Pasquale, and Riemma Citation2021). Digital Supply Chain Twins display the ‘physical supply chain based on actual transportation, inventory, demand, and capacity data’ (Cavalcante et al. Citation2019). Increasing food safety issues and incidents has led to an urgent need to visualise food networks (Hosseini, Ivanov, and Dolgui Citation2020). DTs not only help to visualise food supply chains but also offer stakeholders (such as food retailers and distributors) the ability to analyse risks and forecast FSC issues (Ivanov Citation2020).
One important characteristic of DTs is their ability to virtually duplicate the physical system and convey real-time information from the physical entity (Cai et al. Citation2017). This enables monitoring and real-time simulation (Melesse, Di Pasquale, and Riemma Citation2021) which can alleviate issues of information asymmetry within FSCs. Furthermore, DTs provide historical and real-time data from the physical entity, enabling scenario analysis to compare options and predict potential disruptions helping stakeholders, such as food manufacturers and distributors, to make informed decisions (Tao et al. Citation2018).
DTs are increasingly being used in a wide variety of industry contexts, including construction (e.g. Opoku et al. Citation2021), healthcare (e.g. Semeraro et al. Citation2021), industrial production/manufacturing (e.g. Tao et al. Citation2018), automotive (e.g. Davis et al. Citation2012) and aviation (e.g. Glaessgen and Stargel Citation2012). This wider adoption encourages implementation of DTs in the food industry, where the fundamental characteristics of DTs offer the opportunity of solving some of the FSC’s fundamental challenges. This is a new and rapidly evolving area and a comprehensive literature review discussing the opportunities for the adoption of Digital Twins in the FSC is still missing. This evident research gap leads to the following research questions:
RQ1. How can adopting Digital Twins address food supply chain challenges?
RQ2. What is the process for implementing Digital Twins in FSCs?
A Systematic Literature Review (SLR) is adopted to address the defined research questions. This study contributes by developing a novel conceptual framework for implementing DTs in the FSC and establishing avenues for future research.
The rest of the paper is structured as follows. Section 2 describes the systematic literature review process. Sections 3 and 4 discuss descriptive and thematic analysis findings, respectively. Section 5 develops a conceptual framework for the implementation of Digital Twins in the FSC by combining the findings from the thematic analysis and relevant innovation theories. Section 6 presents five future research directions. Section 7, concludes the paper, capturing contributions to theory and practice along with limitations of the study.
2. Systematic literature review
Following a systematic literature review, this study examines literature at the interface between Digital Twins and FSCs. Unlike a narrative literature review, an SLR is a transparent, evidence-based approach that adopts a rigorous, replicable scientific process (Tranfield, Denyer, and Smart Citation2003; Ghadge, Dani, and Kalawsky Citation2012). The adopted systematic literature review process follows three main steps, as shown in Figure .
Figure 1. Systematic literature review process (Adopted from: Tranfield, Denyer, and Smart Citation2003; Ghadge, Dani, and Kalawsky Citation2012).
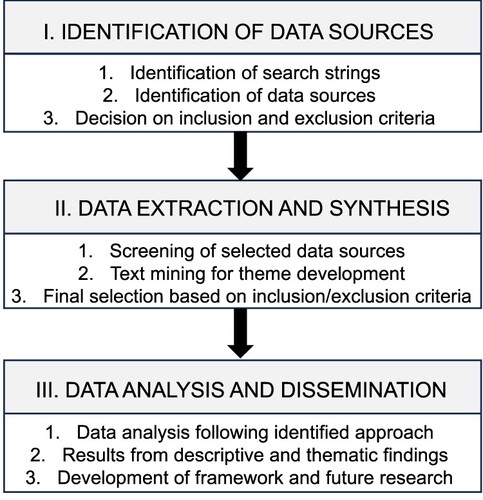
2.1. Identification of data sources
This study used four research databases: Web of Science, EBSCO, Scopus, and ABI/ProQuest to search for relevant papers. EBSCO and ABI/Inform provide literature specifically from the business and management field. In contrast, Scopus and Web of Science include literature from other relevant disciplines, including Engineering. Four databases were selected to capture a holistic, multidisciplinary picture of the research developments in the selected field of study. First, the relevant keywords associated with ‘Digital Twin(s)’ and ‘food supply chain’ were identified, as shown in Table 1. Then, the search strings were established using Boolean logic (Keyword combination with OR/AND), these were used in the identified databases to search for relevant literature.
Table 1. Keywords used for identification of data sources.
The screening process was divided into two stages. First, inclusion and exclusion criteria were introduced to screen the initial search results to identify the most relevant studies. Only full-text and peer-reviewed papers published in academic journals in English between 2012-2023, which were relevant to the topic and keywords of the study were selected. Other academic and non-academic sources, such as practitioner reports, textbooks, book chapters, conference papers, white papers, and HTML links, were excluded from the study. This exclusion of the ‘grey literature’ in SLR studies helps to secure a focus on quality publications (Ghadge et al. Citation2020). Later, full-text screening of papers was conducted using pre-defined relevance and quality criteria, namely methodology, contribution to theory, data analysis sample design and composition, and implications for practice. Details of the quality assessment criteria used are presented in Appendix I for more information.
2.2. Data extraction and synthesis
The four databases identified 5746 papers based on the search strings. After removing 2119 duplicates and applying the inclusion and exclusion criteria, 225 papers were retained. Following full-text screening using the quality assessment criteria, 79 papers were selected, including two additional papers identified through cross-referencing. Finally, 81 papers were approved for data synthesis and analysis. Figure presents the data screening process using the Preferred Reporting Items for Systematic Reviews and Meta Analysis (PRISMA) flow approach.
2.3. Data analysis and dissemination
Data analysis and dissemination followed descriptive and thematic analysis. The descriptive analysis provides a broad overview of the generic trends in the identified papers. In contrast, thematic analysis provides an in-depth analysis based on themes identified through text mining. The insights developed from these themes help to develop a conceptual framework and avenues for future research (Vu et al. Citation2023; Xu et al. Citation2023).
3. Descriptive analysis
This section provides a brief overview of the field following descriptive analysis. Digital Twin definitions and analysis of publication trends, geographical distribution and adopted research methods are presented.
3.1. Definition of digital twins
In recent years, scholars and practitioners have examined the applicability of Digital Twins in the supply chain. Existing definitions of Digital Twins from academic literature are captured in Appendix II. It is evident that there is a lack of consensus in defining Digital Twins. A key concern with most existing definitions is that they do not provide a comprehensive set of abilities, particularly the crucial role of the feedback loop. Thus, this study proposes the following inclusive definition:
A Digital Twin is a virtual representation of a physical entity (such as a part, product, process, or system) that is constantly updated using real-time data to generate dynamic insights and inform decisions which can then be fed back to the real world
3.2. Publication trends
Following the inclusion criteria, the selected papers were all published between 2012 and 2023, this captures the decade-long development of Digital Twins in the FSC. The reason for choosing 2012 as the starting year was that in 2012, NASA formally described the Digital Twin and predicted its potential in the aerospace industry. Since then, scholars and practitioners have examined the applicability of Digital Twins in the supply chain (e.g. Ivanov, Dolgui, and Sokolov Citation2019; Barykin et al. Citation2020). Figure shows the distribution of selected papers by publication year. Most papers in the literature pool have been published in the last four years (from 2019 to 2022), showcasing growing interest in this field. This increase may be attributed to the growing understanding of Digital Twins and their perceived benefits in different areas of the SC, along with the accelerated shift towards digital supply chains post-COVID-19.
3.3. Geographical distribution
The geographical distribution of the selected papers is presented in Figure . Fifty five percent of the selected studies were conducted in European countries. Figure indicates that China (20%), the UK (12%), Germany (11%), and Italy (10%) are the leading countries producing research on Industry 4.0 technology adoption, specifically Digital Twins, within the food industry. The term ‘international’ represents multi country studies and those where the study location is not specified.
3.4. Research methods
Figure summarises the distribution of research methods in the selected papers. Research methods are classified as either qualitative (e.g. Defraeye et al. Citation2021; Ivanov and Dolgui Citation2021; Mandolla et al. Citation2019; Semeraro et al. Citation2021), quantitative (e.g. Accorsi et al. Citation2022; Burgos and Ivanov Citation2021), or mixed methods (e.g. Badakhshan and Ball Citation2023a; Matzembacher et al. Citation2018; Zhao et al. Citation2022).
The most used research approaches are qualitative, namely case studies (20%), literature reviews, and conceptual papers. The selected articles also include studies employing mixed method (28%) and quantitative analysis methods (24%). Literature review papers accounted for 18.7% of the selected papers, these papers focus either on analysing previous literature to acquire knowledge of FSC challenges or applications of Digital Twins within the supply chain, no paper to date provides a conceptual framework for DT implementation in the FSC.
The mixed methods-based studies employ a combination of methods including case studies and various quantitative analysis methods such as mathematical modelling, simulation, and statistical analysis.
3.5. Distribution of journals
The 81 selected papers appear in 27 separate journals. These journals cover a range of different fields, namely operations research and management science, food, and computer science/engineering. Most research discussing how Digital Twins interface with FSCs appears in the operations research and management science field.
The journal publishing the most articles is the International Journal of Production Research (15), a leading journal publishing technological innovation in production and operations, at the forefront of driving this research. In addition, studies investigating how Digital Twins interface with the FSC also appear in journals such as Computers in Industry (4) and Computers and Industrial Engineering (4). Research focusing on food industry applications of Digital Twins also appears in leading food-led journals such as Food Control (8), Journal of Food Engineering (5), and British Food Journal (2). A complete list of journals is provided in Appendix III.
3.6. Technology integration in DTs
Table 2 summarises the Industry 4.0 technologies reported in the selected articles. The Internet of Things (IoT) appears most often (60 times), indicating the importance of this supporting technology for developing Digital Twins. Other relevant technologies, such as cloud computing and wireless networks, are also discussed, these technologies facilitate data storage and transfer. Data analysis is an important component of a Digital Twin, relevant technologies such as Artificial Intelligence (including BDA, Machine Learning and Simulation) appear commonly in the selected articles (35 times), enabling decision-making and providing feedback to the physical environment.
Table 2. Summary of technology occurrences.
4. Thematic analysis
This study adopted a text-mining approach (Queiroz and Fosso Wamba Citation2021; Ghadge et al. Citation2023) for identifying and developing themes from the selected literature. Cluster analysis of the text of the selected papers was conducted using VOS viewer to explore the frequency of occurrence of keywords, establishing potential themes for the thematic analysis. Appendix IV highlights the protocol adopted following Queiroz and Fosso Wamba (Citation2021) to determine the frequency and ensure the reliability of the keywords identified for each cluster. Figure shows a cluster diagram showcasing the key themes emanating from text mining. Identified clusters are circled with dotted lines for clarity.
Cluster one and two are overlapping clusters showcasing characteristics (e.g. monitoring, optimisation, simulation) and applications (such as farming and manufacturing) of Digital Twins. Cluster three showcases relevant emerging technologies (e.g. Internet of Things, Blockchain, Cloud Computing, Artificial Intelligence) needed to build a Digital Twin. Another linking cluster (four) showcases the key drivers and barriers of adopting technologies for DT realisation. Cluster five collates the food supply chain and associated issues/challenges (e.g. food safety, quality, and traceability). Figure highlights the five finalised themes, namely: DT characteristics, FSC challenges, DT applications, and DT implementation barriers and drivers, these themes were used to conduct the thematic analysis.
The food industry faces several challenges, such as food safety, food waste, and lack of traceability. By synthesising the literature, the characteristics of Digital Twins are mapped to provide potential solutions to these challenges. DTs are applied in specific stages of the FSC, such as farming, logistics, and retail processes. This study provides examples of industrial cases to present evidence of the benefits of DTs for FSCs. Analysis of our identified literature pool, using these themes provides an answer to RQ1. Identifying drivers and barriers to implementing DTs guides the development of a framework for adopting DTs in FSCs, thus answering RQ2.
4.1. FSC challenges
The following sections discuss the challenges faced by FSCs identified from the selected literature, these include complexity and uncertainty, food safety and security, food losses and waste, information asymmetry and traceability.
4.1.1. FSC complexity and uncertainty
The FSC is vast and complex, involving multiple direct stakeholders such as farmers, manufacturers, processors, and consumers, but also including other participants such as non-government organisations, international agricultural agencies, governments, and concerned institutions (Yadav et al. Citation2022b). Each stakeholder in the FSC faces unique challenges. Farmer specific challenges include inadequate financial and human resources, poor quality of fertilisers and pesticides, lack of information sharing, and inadequate infrastructure facilities such as refrigerated warehousing (Gardas et al. Citation2019; Zhao et al. Citation2021b; Yadav et al. Citation2022b). Whereas, intermediaries such as processors, distributors, and retailers face complexities such as warehouse management, handling the highly perishable nature of produce, lack of information on consumer prices, and inconsistency in the supply and demand for agricultural produce (Piramuthu, Farahani, and Grunow Citation2013). There is a strong relationship between complexity and uncertainty in the FSC; as the complexity of the FSC increases, so does the level of uncertainty associated with it (Simangunsong, Hendry, and Stevenson Citation2012).
Uncertainty is another significant challenge that occurs at different stages of the FSC. Uncertainty occurs because food businesses and supply chains are fragmented, resulting in poor control over food storage and transport operations (Defraeye et al. Citation2021; Accorsi et al. Citation2022). Furthermore, climate conditions and consumer purchasing behaviour also shape FSC geography and uncertainty (Gokarn and Kuthambalayan Citation2017; Sreedevi and Saranga Citation2017).
In the upstream FSC, uncertainty is caused by seasonal factors, weather conditions, soil quality, and interregional disparities in climate and capital availability (Ghandar et al. Citation2021; Accorsi et al. Citation2022). Simultaneously, the downstream FSC is subjected to heterogeneous and volatile financial and economic fluctuations (Borodin et al. Citation2016). The selected papers demonstrate that the uncertainties responsible for operational interruptions to supply and the supply of degraded quality food products can be attributed to environmental (e.g. Gokarn and Kuthambalayan Citation2017; Onwude et al. Citation2022), transportation (e.g. Pan et al. Citation2021) and operational (Defraeye et al. Citation2021) uncertainties. These uncertainties result in reduced production and marketing opportunities for farmers and, subsequently, food shortages and consumer safety incidents.
4.1.2. Food safety and security
Safe food is defined as food that will not cause sickness or harm when it is prepared, served, or eaten (Matzembacher et al. Citation2018; Behnke and Janssen Citation2020). Most stakeholders, for example, food manufacturers (e.g. Pylianidis, Osinga, and Athanasiadis Citation2021), processors (e.g. Maheshwari et al. Citation2022), distributors (e.g. Accorsi et al. Citation2022), and retailers (e.g. Burgos and Ivanov Citation2021) in the food industry consider food safety to be a central issue (Alfian et al. Citation2020). Food safety is threatened by uncertainties in distribution, such as adverse weather conditions along the route and unscheduled shipping delays (Accorsi et al. Citation2022). Whereas improper post-harvest handling impacts the security of the supply of food as it ultimately results in poor-quality food that is unacceptable to consumers (Shoji et al. Citation2022a). Lastly, food fraud is a growing concern in the FSC, involving origin misrepresentation or substitution, mislabelling, and wrong variety declaration (Yadav et al. Citation2022b). Food contamination, which can introduce dangerous substances or microbes into the FSC, leading to foodborne illnesses that can be life-threatening is another critical factor that can compromise food safety and poses a significant risk to public health globally (Aung and Chang Citation2014). The World Health Organisation (WHO) has identified foodborne disease outbreaks caused by natural, accidental, and deliberate food contamination as major threats to global public health (WHO Citation2007; Aung and Chang Citation2014). In addition, inappropriate storage conditions, particularly temperature abuse during transportation, in food service, or by the consumer, can cause foodborne illnesses (Shankar, Gupta, and Pathak Citation2018; Gallo et al. Citation2021). However, tracking food contamination or damage (physical or due to variation in temperature conditions) during transportation is often hampered by a lack of information (Aung and Chang Citation2014).
4.1.3. Food losses and waste
Food waste is another substantial issue that accounts for one-third of total food production (Dilkes-Hoffman et al. Citation2018; Defraeye et al. Citation2019). Inefficiencies in handling, storage, and lack of infrastructure for processing food, transportation, and cold storage are among the main causes of food waste (Oliveira, Lago, and Dal’ Magro Citation2021). In addition, food production consumes a large amount of energy and resources, food waste represents the waste of these natural resources, including the waste of land, water, and energy (Nakandala, Lau, and Zhao Citation2017; Zhao et al. Citation2021b). Due to this complexity, food stakeholders are unable to assess the total quantity of lost products accurately (Shoji et al. Citation2022b). Food waste or losses happen at various FSC stages, directly and negatively impacting economic development and environmental sustainability (Ringsberg Citation2014; Singh et al. Citation2019).
4.1.4. Information asymmetry
The food industry encounters information asymmetry issues and inadequate information management, which make the FSC inefficient and difficult to manage (Yadav et al. Citation2022b). Two types of information asymmetry are relevant to food safety and security, moral hazard and adverse selection (Astill et al. Citation2019; Sun and Wang Citation2019). Information asymmetry identified between different stages of the FSC directly hinders information sharing, and makes optimal decision-making for stakeholders difficult (Ringsberg Citation2014), causing food safety incidents and scandals. To date, limited literature has focused on problems of asymmetric information related to food attributes (e.g. Piramuthu, Farahani, and Grunow Citation2013). Thus, information sharing and transparency in the FSC are critical to alleviate information asymmetry, improve supply chain management, reduce recall expenses, and expand sales of differentiated products (Ringsberg Citation2014; Behnke and Janssen Citation2020).
4.1.5. FSC traceability
The lack of traceability of food products is another critical challenge for the FSC. Traceability is the ability to track food through all stages of the SC for safety and quality control, traced upward or downward whenever necessary (Bosona and Gebresenbet Citation2013; Behnke and Janssen Citation2020; Zhou et al. Citation2022). The lack of transparent and accessible information in the FSC can also result in inefficiencies and waste, as products are passed from one stage to another with little information about their origin (Vilas-Boas, Rodrigues, and Alberti Citation2022). It is difficult to achieve efficiency in the FSC when multiple blind spots exist (Chen Citation2017). Despite information on food labels, the real-time condition of products during logistics is often unknown, leading to spoilage and reducing consumer confidence (Behnke and Janssen Citation2020).
Traceability across the FSC positively benefits FSCs in terms of food safety (Óskarsdóttir and Oddsson Citation2019; Qian et al. Citation2020), cost (Bosona and Gebresenbet Citation2013), and sustainability (Ringsberg Citation2014). Thus, if food companies cooperate across the FSC, the cost and time of tracking and querying food information can be significantly reduced, which can also improve the flexibility and resilience of the supply chain in responding to disruptions (Verdouw et al. Citation2016; Yadav et al. Citation2022b). Effective collaboration and coordination among stakeholders is critical for ensuring traceability within FSCs. However, independent and heterogeneous data sources generate a massive volume of information, most of which is useless and irrelevant (Parreño-Marchante et al. Citation2014).
4.2. Characteristics of digital twins
The following section discusses the various characteristics of Digital Twins, such as monitoring, real-time simulation, scenario analysis, data and technology integration, and visibility.
4.2.1. Monitoring
Monitoring is highlighted in recent studies as an important characteristic of Digital Twins (Franceschi et al. Citation2022; Kong et al. Citation2021). Digital Twins have been used to monitor production processes, reduce the risk of system failure, and optimise the utilisation of material and human resources in various industries, including FSCs (Rožanec et al. Citation2022). In addition, a DT offers an integrated suite of technologies which provide real-time tracking of food products. For example, an important component technology of Digital Twins, IoT can obtain real-time data across food supply chains to help managers make informed decisions (Pan et al. Citation2021).
Some organisations have applied DTs to determine how their physical systems operate and to keep track of, or monitor, performance in real-time (Battini et al. Citation2022; Maheshwari et al. Citation2022). For example, Digital Twins relying on real-time monitoring and virtual operations, such as maintenance, have been used to predict the remaining life of products (Kittipanya-ngam and Tan Citation2020; Semeraro et al. Citation2021). In addition, Digital Twins can proactively mitigate risks and disruptions through timely feedback and decision-making (Nguyen et al. Citation2022).
4.2.2. Real-Time simulation
Real-time simulation of the physical environment in a computer model is a key characteristic of Digital Twins (Tao et al. Citation2018). Real-time simulation involves using simulation as a digital model of a system to make accurate decisions based on the current state of the physical entity (Barricelli, Casiraghi, and Fogli Citation2019; Lugaresi and Matta Citation2021). Specifically, the physical and digital environments must be synchronised and connected using a well-defined network of sensors and actuators (Ait-Alla et al. Citation2021). Using real-time data from the physical entity, a Digital Twin can simulate different actions and decisions in advance and reflect the most suitable course of action in the physical system (Perno, Hvam, and Haug Citation2022). For example, Ocado uses a Digital Twin to replicate their current grocery fulfilment centres in real-time, simulating the effects of potential layout changes through virtual replicas. This allows Ocado to make precise and economical decisions without interfering with facility operations in the real world (Tozanli and Saénz Citation2022). In addition, feedback from the virtual product can improve the physical product's real-world state (Ghandar et al. Citation2021).
Digital Twins utilise real-time information to drive traceability and visibility, facilitating dynamic operations management (Kamble et al. Citation2022; Wu et al. Citation2022b). Digital Twins create high-fidelity virtual models for each physical entity to simulate their states and behaviours with abilities to evaluate and optimise processes (Semeraro et al. Citation2021).
4.2.3. Scenario analysis
Digital Twins are used to predict the behaviour of physical entities, by conducting scenario analysis they can enable decision-making or the assessment of the impact of disruptions in the SC (Liu et al. Citation2021; Rabah et al. Citation2018; Ivanov Citation2023). In other words, Digital Twins can describe real-world behaviour and predict and propose potential solutions based on scenario analysis (Tao et al. Citation2018; Greif, Stein, and Flath Citation2020). As a result, decision-makers can create vivid simulation scenarios, effectively apply simulation tests to prototypes, and accurately predict the future performance of physical products (Badakhshan and Ball Citation2023a) or investigate the suitability of various inventory policies in each scenario (Badakhshan and Ball Citation2023b).
Digital Twins have been applied in the logistics sector to improve operational efficiency and achieve effective planning and control of logistics operations (Wong et al. Citation2021). Decision-makers can understand both the current status of the logistics system, and analyse different alternative scenarios (Coelho, Relvas, and Barbosa-Póvoa Citation2021). Virtual experiments for different scenarios widen the application of Digital Twins from evaluating food quality in the existing FSC to predicting the impacts of potential changes (Defraeye et al. Citation2021). Scenario analysis allows FSC practitioners to evaluate different scenarios and select the best solution, thus helping to improve their operational efficiency (Accorsi et al. Citation2022; Shoji et al. Citation2022b). The cold chain warehousing and logistics company, Lineage Logistics, has applied Digital Twins to optimise their design processes prior to expensive investment in the construction of a physical warehouse. Digital Twins are used to simulate scenarios, such as demand characteristics, facility location, and consumer preferences, helping the company determine a suitable warehouse design (Tozanli and Saénz Citation2022).
4.2.4. Data and technology integration
Digital Twins are characterised by their ability to integrate different technologies (Franceschi et al. Citation2022; Ivanov and Dolgui Citation2021; Rožanec et al. Citation2022; Tozanli and Saénz Citation2022) and data from heterogeneous sources (Pylianidis, Osinga, and Athanasiadis Citation2021; Perno, Hvam, and Haug Citation2022; Zhang et al. Citation2022). For example, DTs have integrated machine learning and simulation to determine the inventory replenishment policies that minimise the impact of disruptions on SC performance (Badakhshan and Ball Citation2023a). Furthermore, DTs can integrate data from multiple sources such as Internet of Things, Blockchain, AI, and supplier collaboration portals (Ivanov Citation2023) to accurately simulate and assess product design decisions (Semeraro et al. Citation2021) or optimise warehouse layout design and operational processes (Maheshwari et al. Citation2023). If the data and technology integration features of Digital Twins are adopted in the FSC, stakeholders could integrate information from multiple sources to help them make informed decisions (Defraeye et al. Citation2019).
4.2.4. Visibility
Visibility of heterogeneous information sources is important to improve traceability and identify potential food safety or contamination issues at different stages of the FSC. One important characteristic of Digital Twins is the ability to achieve visibility of products across the FSC (Ivanov Citation2023; Tozanli and Saénz Citation2022), as they can store and provide data on food products’ history, from farm to fork (Liu et al. Citation2021). Moreover, Digital Twins facilitate real-time communication and improve information transparency between FSC stakeholders such as food suppliers, manufacturers, producers, distributors, and retailers, which is crucial for effective supply chain management (Mandolla et al. Citation2019; Leung, Lee, and Ouyang Citation2022).
Finally, Digital Twins can be employed to improve the supply chain network’s intelligence, transparency, resilience, and capacity during supply chain disruptions (Burgos and Ivanov Citation2021; Ivanov and Dolgui Citation2021). By applying DT’s visibility functionality, food stakeholders, such as food processors (e.g. Maheshwari et al. Citation2022) and distributors (e.g. Onwude et al. Citation2022; Shoji et al. Citation2022b), can track products to assure food safety, and improve decision-making efficiency.
4.3. Applications of digital twins
The applications of DTs are presented, divided into the multiple stages of the FSC.
4.3.1. Food farming and processing
The application of Digital Twins in farming supports decision-making and response to environmental uncertainties. For example, Ghandar et al. (Citation2021) developed a virtual model of an aquaponics system for urban farming based on a Digital Twin. Likewise, Onwude et al. (Citation2022) used a Digital Twin to quantify the impact of pre and post-harvest variability of orange fruit harvest quantity to reduce food losses, improve resource conservation and food security, and increase supply chain sustainability. The simulation and prediction capabilities of DTs have been used to allow farmers to forecast and optimise farming scenarios, such as the onset of mastitis due to intensive milking (Tagliavini, Defraeye, and Carmeliet Citation2019). A farmer can simulate and evaluate multiple milking scenarios. The application of Digital Twins for disease prevention in livestock farming remains under-exploited, despite the demonstrated potential for early detection of diseases like swine fever (Kamilaris, Kartakoullis, and Prenafeta-Boldú Citation2017). This has the potential to help stakeholders such as farmers (e.g. Pylianidis, Osinga, and Athanasiadis Citation2021), food manufacturers, and processors (e.g. Maheshwari et al. Citation2022) to take proactive measures to prevent both the spread and occurrence of disease. However, adopting Digital Twins in farming may encounter barriers, such as environmental uncertainties, high costs, and a lack of skilled employees.
Digital Twins are also widely used in performance improvement analysis in food processing companies (Maheshwari et al. Citation2022). For example, recently, Mars Wrigley and Accenture trialled a Digital Twin to replicate the flow of chocolate and other ingredients through the production process, identifying bottlenecks and inefficiencies, helping streamline manufacturing, reducing waste, and improving product quality (Accenture Citation2022).
4.3.2. Food logistics
In food logistics Digital Twins comprising a virtual representation of a food product, have allowed companies to track the product’s journey through the supply chain, monitoring the temperature and humidity from production to consumption. This enables them to identify potential bottlenecks or inefficiencies in the logistics process and to maintain their quality and safety. Furthermore, DTs can simulate the physiological behaviour of the fruit and evaluate the different cold chain scenarios, including shortening the shipment time, adjusting the set delivery air temperature during transport, implementing cooling at retail stores, and controlling humidity levels (Defraeye et al. Citation2019; Shoji et al. Citation2022a). For example, Shoji et al. (Citation2022a) used the sensor data from the food transportation process to feed a Digital Twin which calculated the temperature of fruits, including surface and core temperature, and linked the thermal information to a fruit quality decay model which predicted changes in fruit quality. Defraeye et al. (Citation2019), designed a Digital Twin based on the temperature and quality data, to simulate the cooling process in the real-time and improve refrigeration and logistics, reducing food losses. Similarly, Onwude et al. (Citation2022) used a DT to monitor and control the post-harvest life of imported fruits from packhouses to retail stores.
More operationally, the port of Rotterdam has been working with IBM to create a Digital Twin that helps the port test scenarios and understand how to improve operational efficiency (Boyles Citation2019). Finally, DTs also have the potential to help logistics players manage container fleets more efficiently (DHL Citation2019).
4.3.3. Food packaging and retail
There has been little research which discusses how food and retail companies can adopt Digital Twins for supply and demand management. However, Burgos and Ivanov (Citation2021) developed a Digital Twin of a retail SC based on real-life pandemic scenarios and proposed several practical implementation guidelines to improve food SC resilience. Accorsi et al. (Citation2022) proposed a novel Digital Twin framework, deploying scenario analysis to simulate food packaging conditions under environmental stresses throughout the FSC. Tetra Pak, a pioneer in food packaging, is employing a Digital Twin strategy, utilising the remote monitoring capabilities of DTs with cloud-connected machines to precisely predict equipment maintenance (Microsoft Citation2017).
4.4. Drivers of digital twin implementation
This section presents key drivers for the implementation of Digital Twins, broadly categorised into internal and external motivations. External motivations emanate from outside an organisation, such as competitive pressures (cost, productivity, and quality) and wider technology support. Internal drivers come from inside the organisation and include improving operational efficiency and enhancing the visibility and transparency of FSCs.
4.4.1. Improving operational efficiency
Digital Twins are expected to enable supply chains to respond quickly to uncertainties and optimise supply chain operations to improve decision-making efficiency through real-time communication and feedback between the cyber and physical systems (Kamble et al. Citation2022; Tozanli and Saénz Citation2022; Tao et al. Citation2018; Lim, Zheng, and Chen Citation2020).
By applying different simulation models and combining them with various technologies, DTs can enhance an existing system’s availability, allocation efficiency, technical efficiency, worker efficiency, and utilisation rate (Maheshwari et al. Citation2022). Digital Twins are a key technology for implementing reconfigurable SC networks and improving operational efficiency (Ivanov et al. Citation2019; Park et al. Citation2021). In addition, Badakhshan and Ball (Citation2023a) developed a Digital Twin of a warehouse management system to enhance the efficiency of in-house operations. Furthermore, Digital Twins increase the communication between cyber and physical systems, helping identify potential bottlenecks, seek solutions, and eventually achieve higher efficiency in the FSC (Onwude et al. Citation2022; Pylianidis, Osinga, and Athanasiadis Citation2021).
4.4.2. Enhancing FSC visibility and transparency
An increasing number of consumers demand to know the origin of the food they buy (Bosona and Gebresenbet Citation2013; Gallo et al. Citation2021; Ringsberg Citation2014). Due to the complexity of the FSC and the constantly changing market conditions (Fritz and Schiefer Citation2009), it is difficult for consumers to get sufficient information and for stakeholders to have enough information to make the right decisions. Insufficient transparency and visibility can also result in quality and safety issues in the FSC.
Achieving visibility and transparency is a key motivation for implementing Digital Twins. Digital Twins provide food manufacturers (e.g. Maheshwari et al. Citation2022), distributors (e.g. Accorsi et al. Citation2022), and retailers with end-to-end visibility of food conditions (Burgos and Ivanov Citation2021). They can be used to identify potential safety risks and bottlenecks, providing managers with actionable insights, and allowing stakeholders to simulate different scenarios to find better solutions. Digital Twins can identify problems in real-time by recording and storing information such as shipment condition (temperature, humidity, warehouse transportation) data (Defraeye et al. Citation2019; Alfian et al. Citation2020). Improving food safety is another driver for implementing Digital Twins in the FSC. For agricultural products, such as meat, where food safety is critical (Fang et al. Citation2017), DTs have the potential to promote food safety by tracing, tracking, and controlling the important quality factors.
4.4.3. Competitive pressures (cost, productivity, and quality)
An external driver of implementing Digital Twins, ever-increasing business competition puts pressure on companies to reduce costs and improve productivity (Battini et al. Citation2022; Gebhardt et al. Citation2022; Mandolla et al. Citation2019; Semeraro et al. Citation2021). In response to this external pressure, the role of Digital Twins is to reduce cost and improve productivity and quality internally, this includes quality defect detection, fault prediction, reducing testing-related costs, simulating scenarios, improving quality and reducing risks and cost losses of making improper decisions. For example, the architecture of Digital Twins is driven by the goal of cost reduction and was found to be associated with the execution of predictive maintenance or fault prediction (Tao et al. Citation2018; Greif, Stein, and Flath Citation2020; Rožanec et al. Citation2022). Furthermore, a key function of a Digital Twin is finding solutions to improve product quality through multiple simulations and predictions based on different scenarios (Pan et al. Citation2021).
4.4.4. Wider technology support
Industry 4.0 technologies, such as IoT, cloud computing, and virtual reality systems, enable the remote sensing and monitoring of supply chain systems, allowing effective control of devices and synchronisation between the virtual and the real worlds (Kamble et al. Citation2022; Kuo et al. Citation2021). This has become a key external motivation for implementing Digital Twins. Industry 4.0 has contributed to the rise of new technologies for data acquisition, storage, and communication, revealing knowledge of a products status at any time (Tao et al. Citation2018; Lugaresi and Matta Citation2021). The collaborative use of Industry 4.0 technologies has resulted in the development of Digital Twins (Liu et al. Citation2021; Kong et al. Citation2021; Li et al. Citation2021). For example, Blockchain, a support tool of DTs can ensure that transactions between stakeholders are secure as well as transparent (Mandolla et al. Citation2019).
4.5. Barriers to digital twin implementation
This study categorised the barriers to Digital Twin implementation as data security and storage, data processing, lack of collaboration, lack of system synchronisation and integration, and high implementation cost.
4.5.1. Data security and storage issues
Data is the bridge which enables interaction between virtual and physical systems in Digital Twins (Kong et al. Citation2021). Data security issues involve risks associated with data acquisition, exchange, storage, and processing (Perno, Hvam, and Haug Citation2022). FSC companies intend to develop Digital Twins to convey food product data in real-time, this data is generated by multiple stakeholders and from multiple data sources (Ghandar et al. Citation2021; Maheshwari et al. Citation2022; Tozanli and Saénz Citation2022). Thus, data storage and security are critical barriers to the implementation of Digital Twins.
Perno, Hvam, and Haug (Citation2022) highlighted the criticality of data security and the difficulty in ensuring it. Data privacy and security issues within Digital Twins will often be seen in supply chains operating in heterogeneous environments with multiple applications and users. Keeping the data, models, and related information secure will be a key challenge in the successful implementation of DTs in the supply chain context (Tao et al. Citation2018; Kamble et al. Citation2022).
4.5.2. Data processing issues
Data processing issues are directly related to limitations in the hardware and software resources that guarantee an efficient flow of data between physical and digital systems (Tao et al. Citation2019a; Kong et al. Citation2021). These issues have the potential to limit the effectiveness of DTs. One of the key characteristics of DTs is their ability to represent and monitor the status of their physical twin in real time. It is critical to provide sufficient hardware and software infrastructure to link the multiple FSC data sources and therefore support the Digital Twins data processing capabilities. Zhao et al. (Citation2021a) highlighted the issue of ensuring an efficient stream of data from the physical assets to their DT and the difficulty of working with big data to ensure real-time communication.
4.5.3. Lack of collaboration
Digital Twins integrate multiple technologies to serve stakeholders (Li et al. Citation2021). Ensuring effective collaboration between these different stakeholders is often challenging (Tao et al. Citation2019b; Mandolla et al. Citation2019; Jiang et al. Citation2021). FSC stakeholders (such as farmers, processors, manufacturers, and retailers) have different interests, and finding the collaboration mechanism to bring them together is a barrier to the implementation of Digital Twins in the food industry. During the exploitation of DTs, much time and resources are needed to build collaboration between stakeholders (Li et al. Citation2021; Lugaresi and Matta Citation2021).
4.5.4. Lack of system synchronisation and integration
Modelling Digital Twins encounters issues with synchronisation between the physical objects and the entities in the virtual environment (Maheshwari et al. Citation2022). This requires effective and reliable sensing technologies to capture information about the physical objects and fast communication between sensors and Digital Twins (Kuo et al. Citation2021). Interpretation of physical properties of the entities in virtual space (Semeraro et al. Citation2021), conflicts between detection and resolving methods (Li et al. Citation2021), and problems associated with automatic updating of the Digital Twin model on a real-time basis (Lugaresi and Matta Citation2021) are all associated with synchronisation.
This barrier includes integrating new systems or platforms into the existing system (Perno, Hvam, and Haug Citation2022). Establishing a new Digital Twin platform to replace legacy systems is difficult (Maheshwari et al. Citation2022). Similarly, developing an integrated Digital Twin platform that connects separate existing systems is equally difficult. Heterogenous data formats, data quality, and collection frequency across various information systems result in inconsistencies (Franceschi et al. Citation2022; Kong et al. Citation2021). In addition, system integration issues impact the transition from old and outdated legacy equipment or systems to a new state. Perno, Hvam, and Haug (Citation2022) argued that the need to integrate any new asset or make operational changes to the existing infrastructure, is a major challenge which needs significant investment in terms of time and effort.
4.5.5. High implementation cost
One of the biggest challenges for organisations in adopting Digital Twins is the high investment cost (Ivanov Citation2020; Manos and Manikas Citation2010). This includes high research and development costs, technical training costs, and implementation consultant costs, all of these are likely to delay the adoption of a Digital Twin (Lim, Zheng, and Chen Citation2020; Kamble et al. Citation2022; Rožanec et al. Citation2022).
Large companies such as Maersk and IBM are anticipated to be ‘early adopters’. However, small and medium-sized firms may hesitate to be involved in such projects. Investing in DTs is expensive and challenging for small and medium-sized enterprises (Perno, Hvam, and Haug Citation2022; Maheshwari et al. Citation2023) as the firm’s return on investment and the benefits of integrating DT technologies are not guaranteed.
5. Framework for the implementation of digital twins within the FSC
Following the thematic analysis, it is evident that the characteristics of DTs provide the potential to overcome many of the challenges and issues faced by the FSC, such as food traceability, uncertainty, and information asymmetry. A Digital Twin is the integration of a number of technologies, such as the combination of sensory data acquisition, big data analytics, IoT, AI, and other supporting technologies (Ivanov Citation2023; Tozanli and Saénz Citation2022). Digital Twins virtually duplicates the physical system and convey real-time data and information from the physical entity (Cai et al. Citation2017), which helps alleviate information asymmetry issues in the FSC. Figure showcases the relationships between FSC challenges and the characteristics of Digital Twins, as evidenced by the analysis.
Digital Twins have the potential to help alleviate some of the FSC’s complexity and uncertainty issues. Digital Twins assists FSC stakeholders (farmers, food manufacturers, food logistics, and distributors) to identify their current challenges or risks through scenario analysis (Accorsi et al. Citation2022; Pylianidis, Osinga, and Athanasiadis Citation2021; Shoji et al. Citation2022b). This characteristic further helps to develop contingencies to deal with complexity and make informed decisions based on historical and real-time data. Furthermore, Digital Twins can reduce uncertainty by integrating data across different food supply chain stages, providing much-needed visibility to manage complex, distributed FSC networks. Real-time simulation and data shared across Digital Twins provides the agility to address uncertain events such as weather disruptions (Burgos and Ivanov Citation2021; Defraeye et al. Citation2019).
Digital Twins can enhance food safety and security through characteristics such as real-time simulation, monitoring, scenario analysis, and visibility. For example, DTs can employ IoT to improve visibility and transparency in food manufacturing and operations processes. Additionally, tracking and monitoring the flow of food using IoT can help identify contamination sources during recalls or disease outbreaks (Alfian et al. Citation2020; Aung and Chang Citation2014; Piramuthu, Farahani, and Grunow Citation2013). Digital Twins can alleviate information asymmetry to some extent in the FSC by providing integration and visibility across different data and information sources (Yadav et al. Citation2022a). Digital Twins carry an integrative ability to consolidate fragmented data from growers, farmers, processors, distributors, and retailers into a unified virtual model, replacing siloed information with end-to-end visibility and transparency. This relationship between FSC challenges and the characteristics of DTs provides the answer to RQ1, exploring the potential of DTs to address the challenges of the FSC.
Answering the second research question involves developing a detailed process for implementing DTs in the FSC. Observations made through the thematic analysis combined with Innovation Adoption Theory and the Technology-Organization-Environment (TOE) framework are used to develop a conceptual framework for the implementation of DTs in the FSC, as shown in Figure . This framework follows the Innovation Adoption Process and proposes three main implementation stages, namely, pre-adoption, adoption, and post-adoption. These three implementation stages are further broken down into five steps: initiation, technology assessment, adoption decision, implementation, and performance evaluation. The adoption of innovation in organisations can be considered a step-wise process spanning from decision-making to implementation within an organisation (Hameed, Counsell, and Swift Citation2012; Zhu et al. Citation2006). The following section explains each stage and the associated steps needed for successfully implementing DTs in the FSC.
5.1. Pre-Adoption stage
The TOE framework, initially proposed and later adapted in IT adoption research, provides a useful analytical framework for studying the pre-adoption decisions of different types of IT innovation (Baker Citation2012; Orji et al. Citation2020). The TOE framework explains the factors that influence the implementation of technological innovations from technological, organisational, and environmental dimensions (Pichlak Citation2016). In the pre-adoption stage, the first step is initiation, where food companies determine if they have a demand or need; such as identifying a FSC challenge that they may be facing, and proposing a suitable plan to address this challenge (Damanpour and Schneider Citation2006; Hameed, Counsell, and Swift Citation2012; Kirkman Citation2012).
From the results of our thematic findings, the characteristics of DTs reveal the potential benefits, and usefulness of the technology. These include monitoring, real-time simulation, and scenario analysis. The thematic analysis also highlights a limited number of successful pilot applications of DTs driven by innovation attributes such as complexity and compatibility. Wider technology support is a critical driver of the implementation of DTs. Data synchronisation between different technologies and system integration supports the implementation of DTs helping to overcome the barriers to the implementation of technology innovation identified in this study.
Similarly, there are specific attributes that can influence the technology adoption process from an organisational perspective. Organisational drivers such as improving operational efficiency and enhancing visibility and transparency are identified in this study. Implementation barriers, such as data security and storage and data processing, can be regarded as characteristics of an organisation. These attributes impact different stages of technology or innovation implementation. Lack of collaboration among different companies and stakeholders is another organisational characteristic and a limitation for the large-scale implementation of technology.
Environmental characteristics include attributes such as markets, uncertainty, and resource availability (Pichlak Citation2016). FSC challenges such as FSC complexity and uncertainty, food safety, food waste, and information asymmetry between stakeholders are considered external environmental barriers to implementing Digital Twins. Competitive pressure in terms of cost, productivity, and quality are environmental drivers of the implementation of DTs.
5.2. Adoption stage
The adoption stage follows Innovation Adoption Theory which guides organisations in adopting information technology (IT) within their businesses and includes the technology assessment, adoption decision and implementation stages (Vu, Ghadge, and Bourlakis Citation2023; Xu et al. Citation2023). The technology assessment literature has emphasised the importance and necessity of technology assessment (Banta Citation2009; Huff et al. Citation2014; Tran and Daim Citation2008). Hellström (Citation2003) proposed a general framework for ‘innovation’ within technology assessment. Compared with other technologies, Digital Twins are an integration of technologies (Glaessgen and Stargel Citation2012; Tao et al. Citation2019b) and thus need an iterative process to implement successfully. Before implementing Digital Twins, the company assesses whether its current information technology is sufficient and determines if currently adopted information technologies can be combined with the Digital Twins applications. This crucial stage is currently not included in the existing Innovation Adoption Theory adoption stages.
The adoption decision stage is further subdivided into decision-making and resource allocation. Specifically, once organisations make the decision to adopt a technology based on the technology innovation plan (considering technical, financial, and strategy aspects), the next stage is to determine the resource allocation for technology adoption. The implementation stage involves understanding stakeholders’ acceptance of the innovation. If stakeholders approve the technology innovation, then the company will extend the technology adoption from the pilot stage to large-scale application.
5.3. Post-Adoption stage
An illustration of the effectiveness and suitability of a specific technology in the context of wider technology implementation is necessary (Love and Irani Citation2004). Thus, a step named performance evaluation is included in the post-adoption stage. Currently, there are few studies or industrial implementation cases of Digital Twins within supply chains, and most of the literature discusses a small number of benefits of Digital Twins, such as cost, quality, efficiency or sustainability (Defraeye et al. Citation2019, Citation2021; Tao et al. Citation2019a; Ivanov and Dolgui Citation2021; Kamble et al. Citation2022). Thus, performance evaluation of adopted Digital Twins within FSCs is important to understand both whether DTs meet the companies’ business requirements and the wider benefits. The performance evaluation stage also allows stakeholders to compare the performance of DTs implemented in FSCs with conventional FSCs.
In summary, the conceptual framework for the implementation of DTs is suitable for food companies who wish to explore implementing DTs to overcome the challenges of conventional FSCs. The structured implementation process is divided into three stages, encompassing five critical steps. During the pre-adoption stage, companies can ascertain their business and operational conditions from technological, organisational, and environmental perspectives. FSC practitioners can evaluate the operational performance of DTs in their company using the suggested performance evaluation metrics. This framework for DT implementation is expected to bring several benefits to FSCs, including increased traceability, improved efficiency, cost reduction, and better sustainability performance. These benefits collectively contribute to the increased competitiveness of FSCs.
6. Future research directions
Based on the analysis presented in this study, we make five recommendations for future research directions.
6.1. Need for digital twin evaluation metrics in FSCs
Digital Twins are a potential solution for improving the performance of FSCs (Ivanov and Dolgui Citation2021; Shoji et al. Citation2022a; Singh et al. Citation2023). However, the performance criteria for evaluating the effectiveness of Digital Twins are yet to be developed. Digital Twins have been demonstrated to optimise supply chain systems and improve operational efficiency (Ghandar et al. Citation2021; Badakhshan and Ball Citation2023a; Zheng et al. Citation2022). However, there is limited discussion of the specific influence and benefit of Digital Twins on industry performance. There is, therefore, a need to develop performance evaluation metrics for Digital Twins. Future research should develop specific performance evaluation metrics for Digital Twins to enable the quantification of their impact on the performance of food supply chains.
6.2. Lack of clarity of technology relevance and integration capabilities
The extant literature has highlighted that the technologies which are combined to make Digital Twins, such as IoT, cloud computing, and BDA, have been demonstrated to help improve real-time data collection and transfer and solve the challenges faced at different stages of the FSC (Defraeye et al. Citation2019; Alfian et al. Citation2020; Lim, Zheng, and Chen Citation2020). However, the devices used at different FSC stages currently differ. For example, considering IoT, the sensors that are used on a farm to collect real-world data on plants (Ghandar et al. Citation2021), are likely to be different from the sensors used for capturing transportation data. Compatibility and integration issues associated with these technologies need to be addressed as a lack of standardisation and scalability of these technologies will cause a significant barrier to the wider implementation of DTs (Kuo et al. Citation2021; Wu et al. Citation2022a).
These technologies are also currently assessed independently, their performance and compatibility, when integrated as part of a DT, still needs to be explored. For example, Dolgui and Ivanov (Citation2023) have conceptualised the supply chain metaverse as supply chains enabled by DTs with additional properties and behaviours. There needs to be clarity as to how different technologies and data sources should be integrated to create a reliable and efficient supply chain. There is therefore a need to develop a decision support system for choosing relevant technologies for sector-specific applications of DTs.
6.3. Need for SC inter-level applications in FSCs
The concept of a Digital Twin focuses on developing a digital replica of a single system or asset within a firm, such as a manufacturing plant or a specific piece of equipment (Lugaresi and Matta Citation2021; Rožanec et al. Citation2022). However, as Digital Twins evolve, there is a growing need to extend their application across different stages of the FSC. To date, researchers and scholars have focused on discussing the application of Digital Twins in specific FSC stages, such as farming (Ghandar et al. Citation2021), food transportation (Shoji et al. Citation2022b), or food retailing (Burgos and Ivanov Citation2021). The shift from implementing Digital Twins in a single food firm to an SC inter-level application is currently missing (Battini et al. Citation2022; Chabanet et al. Citation2023; Lim, Zheng, and Chen Citation2020).
While there has been some effort to develop Digital Twins for wider supply chain systems, there is a significant research gap in this area. One of the key challenges in extending the application to the wider supply chain is the lack of standardisation in data collection and information sharing across supply chain stakeholders (Perno, Hvam, and Haug Citation2022; Semeraro et al. Citation2021; Tozanli and Saénz Citation2022). This results in data silos and limits the ability to create an integrated system-wide Digital Twin or network of Digital Twins that can span across the supply chain network.
6.4. Need for user acceptance analysis of digital twins
To date, most studies have focussed on the technical challenges of developing Digital Twins. However, research on the user acceptance of this technology in the supply chain or FSC context is needed. The study by Kuo et al. (Citation2021) provides an example of how Digital Twins might be enhanced from the user perspective. However, without understanding users’ potential resistance to these technologies, the success of the implementation of Digital Twins may be limited. Empirical studies attempting to understand stakeholders’ attitudes towards technology use can be further leveraged to accelerate the adoption of Digital Twins. Future research should also explore the attributes influencing user acceptance (Kuo et al. Citation2021; Pichlak Citation2016) and identify strategies to address user challenges to increase the speed of adoption of Digital Twins.
6.5. Achieving digital transformation driven by digital twins in FSCs
While it is true that Digital Twins have the potential to transform the FSC (Pylianidis, Osinga, and Athanasiadis Citation2021; Accorsi et al. Citation2022; Shoji et al. Citation2022a), it is important to evaluate how well they can support wider digital transformation. Digital transformation involves integrating different technologies and capabilities to improve production, operations, or services (Kamilaris, Fonts, and Prenafeta-Boldύ Citation2019; Bhandal et al. Citation2022). Digital Twins are just one of the many digital technologies enabling wider digital transformation. However, pilot adoptions of Digital Twins in various stages of the FSC (Hang, Ullah, and Kim Citation2020; Kuo et al. Citation2021; Maheshwari et al. Citation2022) are providing strong support to the potential achievement of digital transformation within FSCs. Thus, there is a need for research which explores other supporting technologies, such as cloud computing and virtual reality, that can be integrated with a Digital Twin to achieve digital transformation within the FSC.
7. Conclusion and contribution
7.1. Conclusion
This study aimed to answer two research questions following a systematic literature review. To address RQ1: How can adopting Digital Twins address food supply chain challenges? Five themes were identified from the selected literature and assessed using thematic analysis. FSC challenges, characteristics of DTs, applications, implementation drivers and barriers were used to derive comprehensive insights. The challenges of the FSC were established: complexity and uncertainty, food safety, food waste, information asymmetry, and traceability. The characteristics of Digital Twins, including monitoring, real-time simulation, scenario analysis, data and technology integration, and visibility, were identified. The combination of complementary characteristics of DTs was identified as a key enabler for addressing FSC challenges. Furthermore, the study identified a number of current applications of Digital Twins in the food industry. These real-world examples help validate the need and opportunity for adopting Digital Twins in the FSC. A comprehensive understanding of the relationship between FSC challenges and the characteristics of DTs, along with applications of DTs, answers RQ1.
To answer RQ2: What is the process for implementing Digital Twins in FSCs? The study explored the drivers, barriers, and examples of successful adoption of DTs in the FSC. Specifically, internal drivers such as improving operational efficiency and enhancing visibility and transparency and external drivers including wider technology support and competitive pressures (cost, production, and quality) are expected to drive organisations towards implementing Digital Twins. Similarly, barriers were identified which hinder the implementation of Digital Twins, including data security and storage, data processing, lack of collaboration, synchronisation and system integration, and high implementation costs. These insights are brought together to develop a theory-driven conceptual framework for implementing Digital Twins in FSCs. This framework proposed three main implementation stages: pre-adoption, adoption, and post-adoption. The TOE framework provides technological, organisational, and environmental characteristics critical for the pre-adoption stage. Technology assessment and performance evaluation were established as two innovative steps to implementing DTs successfully. The presentation of this conceptual framework for implementing DTs in FSCs (Figure ) provides the answer to RQ2. Finally, detailed synthesis of the findings of the thematic analysis has allowed us to propose five avenues for future research.
7.2. Contribution to theory
The study makes the following contributions to theory. This review enriches existing knowledge at the interface between Digital Twins and FSCs. To the best of our knowledge, it is the first systematic literature review study to explore the potential of Digital Twins in the food supply chain context. Our SLR provides researchers with insights into the attributes, characteristics and applications of DTs at different stages of the supply chain, complementing the research conducted by Ivanov (Citation2023), which conceptualised a Digital Twin framework for supply chain and operations management.
We have employed Innovation Adoption Theory and the TOE framework to develop a novel conceptual framework for implementing Digital Twins in the FSC. This novel conceptual framework divides the implementation process into three main stages: pre-adoption, adoption, and post-adoption. In the pre-adoption stage, the TOE framework provides three dimensions for analysing the potential factors of pre-adoption. Building on Innovation Adoption Theory our framework extends the original three-step IT adoption process into five steps, with technology assessment added to the adoption process and performance evaluation metrics added to the post-adoption stage.
Our proposed conceptual framework for the implementation of Digital Twins within the FSC should be tested and validated by researchers in the future. More importantly, our five proposed research directions offer a sound foundation for researchers to explore further development and evaluation of Digital Twins in the supply chain.
7.3. Contribution to practice
This systematic literature review offers insights which enable food industry managers and practitioners to understand the characteristics and applications of DTs in the FSC context. The insights generated by this review can assist managers in assessing the current status of technology adoption in their organisations and determine if there is a requirement to implement Digital Twins in their operations. It is unlikely that Digital Twins will be able to alleviate all the challenges of the FSC and companies are likely to have already invested in other IT systems (such as EDI, ERP, Blockchain or IoT). Considering the inherent complexity and uncertainty of the food industry, managers need to think about their existing information technology and consider how Digital Twin-based solutions can be integrated with these existing technologies.
Our novel conceptual framework provides detailed guidelines for implementing Digital Twins in the food industry. The framework is divided into three main stages, in the pre-adoption stage the framework enables practitioners to assess the key factors from technological, organisational, and environmental viewpoints. In the post-adoption stage the performance evaluation metrics will assist practitioners in determining if the implementation of DTs will benefit their businesses. Following full validation, this framework will provide an invaluable guide for policymakers and governments to better understand the benefits of Digital Twins in the food industry and help formulate future policies and regulations for encouraging wider adoption of this disruptive technology in other supply chains. Overall, this review has highlighted the importance of establishing a standard roadmap for adopting DTs in supply chains.
7.4. Limitations of the study
This study has the following limitations. First, the study is based on 81 papers from a range of research areas. The inclusion of papers was limited to literature discussing Digital Twins within the FSC context. Only papers discussing DTs and FSCs published between 2012-2023 were selected. Thus, valuable literature on FSCs published earlier or later may have been neglected. Furthermore, this study only selected peer-reviewed journal papers and excluded other ‘grey literature’, thus potentially missing useful insights. Secondly, although an SLR provides a rigorous process, the mechanical method of extracting relevant data may have limited the findings. The selection of keywords and the screening process can be subjective and biased as it depends on the interpretation of the research team. Meanwhile, the potential for human error is unavoidable during the search, screening, and data extraction process, which may lead to erroneous review results. The use of text mining/data mining tools would have helped with establishing themes and validating other findings. Finally, as the application of Digital Twins in the FSC is a relatively new field of research, and there is limited empirical evidence to support their effectiveness in a real-world setting. This makes it difficult to draw generic conclusions from the available literature.
Acknowledgement
The authors are grateful to four anonymous reviewers and Associate Editor for their constructive comments and suggestions to improve the contents of this paper.
Disclosure statement
No potential conflict of interest was reported by the author(s).
Data availability statement
The authors confirm that the secondary data used for this study is available within the article and its supplementary materials. Derived data supporting the findings of this study are available from the corresponding author on reasonable request.
Additional information
Notes on contributors
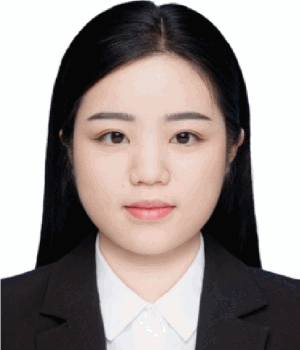
Ying Huang
Ying Huang is a PhD researcher at the Centre of Logistics, Procurement, and Supply Chain Management, Cranfield University, UK. Ying obtained her Master of Science in Logistics and Supply Chain Management with distinction at Cranfield University, UK, in 2020. She is actively engaged in advancing research in the implementation of Digital Twins within the food supply chain. Her research interests include food supply chain management and digital transformation (e.g. Big Data, the Internet of Things, Cloud Computing, and Digital Twins).
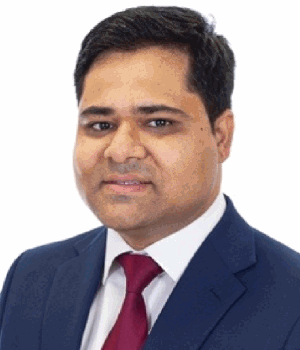
Abhijeet Ghadge
Dr Abhijeet Ghadge is an Associate Professor/Reader of Supply Chain Management at Cranfield School of Management, UK. He holds PhD in Operations and Supply Chain Management from Loughborough University, UK, and MTech in Industrial Engineering and Management from Indian Institute of Technology, India. He has several years of industrial, academic and consulting experience working with a wide range of UK, European and Asian organisations. Dr Ghadge has published over 80 research papers, including 50 journal articles in leading operations, logistics and supply chain management journals. He follows a practice-driven approach to problems across the broad domains of Digital and Resilient Supply Chains.
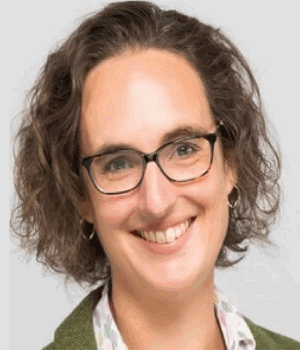
Nicky Yates
Dr Nicky Yates is a Senior Lecturer within the Cranfield Centre for Logistics, Procurement and Supply Chain Management and Director of Doctoral Programmes for Cranfield School of Management. An Engineer by training, she spent the first six years of her working life in the food industry for Unilever and, subsequently Birds Eye as a Research Engineer. She has a wide general interest in many aspects of modelling the supply chain and her teaching and research are focused in these areas, with a particular interest in the management of resilient supply chains and those which handle perishable products such as food and blood. Her research has been published in a number of internationally renowned journals, and she has carried out projects in many sectors from healthcare to construction.
References
- Accenture. 2022. “Accenture Collaborates with Mars to Develop Factory of the Future Using AI, Cloud, Edge, and Digital Twins.” Accenture Newsroom. https://newsroom.accenture.com/news/accenture-collaborates-with-mars-to-develop-factory-of-the-future-using-ai-cloud-edge-and-digital-twins.htm.
- *Accorsi, R., M. Bortolini, M. Gamberi, B. Guidani, R. Manzini, and M. Ronzoni. 2022. “Simulating Product-Packaging Conditions Under Environmental Stresses in a Food Supply Chain Cyber-Physical Twin.” Journal of Food Engineering 320: 110930.
- Ahumada, O., and J. R. Villalobos. 2009. “Application of Planning Models in the Agri-Food Supply Chain: A Review.” European Journal of Operational Research 196 (1): 1–20.
- *Ait-Alla, A., M. Kreutz, D. Rippel, M. Lütjen, and M. Freitag. 2021. “Simulated-based Methodology for the Interface Configuration of Cyber-Physical Production Systems.” International Journal of Production Research 59 (17): 5388–5403.
- Alam, K. M., and A. El Saddik. 2017. “C2PS: A Digital Twin Architecture Reference Model for the Cloud-Based Cyber-Physical Systems.” IEEE Access 5: 2050–2062.
- *Alfian, G., M. Syafrudin, U. Farooq, M. R. Ma'arif, M. A. Syaekhoni, N. L. Fitriyani, … J. Rhee. 2020. “Improving Efficiency of RFID-Based Traceability System for Perishable Food by Utilizing IoT Sensors and Machine Learning Model.” Food Control 110: 107016.
- Asimov, R. M., S. V. Chernoshey, I. Kruse, and V. S. Osipovich. 2018. Digital twin in the Analysis of a Big Data.
- Astill, J., R. A. Dara, M. Campbell, J. M. Farber, E. D. Fraser, S. Sharif, and R. Y. Yada. 2019. “Transparency in Food Supply Chains: A Review of Enabling Technology Solutions.” Trends in Food Science & Technology 91: 240–247.
- *Aung, M. M., and Y. S. Chang. 2014. “Traceability in a Food Supply Chain: Safety and Quality Perspectives.” Food Control 39 (1): 172–184.
- *Badakhshan, E., and P. Ball. 2023a. “Applying Digital Twins for Inventory and Cash Management in Supply Chains Under Physical and Financial Disruptions.” International Journal of Production Research 61(15): 5094–5116.
- *Badakhshan, E., and P. Ball. 2023b. “Deploying Hybrid Modelling to Support the Development of a Digital Twin for Supply Chain Master Planning Under Disruptions.” International Journal of Production Research, 1–32.
- Baker, J. 2012. “The Technology-Organization-Environment.” In Information Systems Theory: Explaining and Predicting our Digital Society, 231–245. Hamburg, Germany: University of Hamburg,
- Banta, D. 2009. “What is Technology Assessment?” International Journal of Technology Assessment in Health Care 25 (S1): 7–9.
- *Barricelli, B. R., E. Casiraghi, and D. Fogli. 2019. “A Survey on Digital Twin: Definitions, Characteristics, Applications, and Design Implications.” IEEE Access 7: 167653–167671.
- Barykin, S. Y., A. A. Bochkarev, O. V. Kalinina, and V. K. Yadykin. 2020. “Concept for a Supply Chain Digital Twin.” International Journal of Mathematical, Engineering and Management Sciences 5 (6): 1498.
- *Battini, D., N. Berti, S. Finco, M. Guidolin, M. Reggiani, and L. Tagliapietra. 2022. “WEM-Platform: A Real-Time Platform for Full-Body Ergonomic Assessment and Feedback in Manufacturing and Logistics Systems.” Computers & Industrial Engineering 164: 107881.
- *Behnke, K., and M. F. W. H. A. Janssen. 2020. “Boundary Conditions for Traceability in Food Supply Chains Using Blockchain Technology.” International Journal of Information Management 52: 101969.
- *Bhandal, R., R. Meriton, R. E. Kavanagh, and A. Brown. 2022. “The Application of Digital Twin Technology in Operations and Supply Chain Management: A Bibliometric Review.” Supply Chain Management: An International Journal 27 (2): 182–206.
- Borodin, V., J. Bourtembourg, F. Hnaien, and N. Labadie. 2016. “Handling Uncertainty in Agricultural Supply Chain Management: A State of the Art.” European Journal of Operational Research 254 (2): 348–359.
- *Bosona, T., and G. Gebresenbet. 2013. “Food Traceability as an Integral Part of Logistics Management in Food and Agricultural Supply Chain.” Food Control 33 (1): 32–48.
- Boyles, R. 2019. “How the Port of Rotterdam Is using IBM Digital Twin Technology to Transform Itself from the Biggest to the Smartest.” IBM Blog. https://www.ibm.com/blog/iot-digital-twin-rotterdam/.
- *Burgos, D., and D. Ivanov. 2021. “Food Retail Supply Chain Resilience and the COVID-19 Pandemic: A Digital Twin-Based Impact Analysis and Improvement Directions.” Transportation Research Part E: Logistics and Transportation Review 152: 102412.
- Cai, Y., B. Starly, P. Cohen, and Y. S. Lee. 2017. “Sensor Data and Information Fusion to Construct Digital-Twins Virtual Machine Tools for Cyber-Physical Manufacturing.” Procedia Manufacturing 10: 1031–1042.
- *Casino, F., V. Kanakaris, T. K. Dasaklis, S. Moschuris, S. Stachtiaris, M. Pagoni, and N. P. Rachaniotis. 2021. “Blockchain-based Food Supply Chain Traceability: A Case Study in the Dairy Sector.” International Journal of Production Research 59 (19): 5758–5770.
- *Cavalcante, I. M., E. M. Frazzon, F. A. Forcellini, and D. Ivanov. 2019. “A Supervised Machine Learning Approach to Data-Driven Simulation of Resilient Supplier Selection in Digital Manufacturing.” International Journal of Information Management 49: 86–97.
- *Chabanet, S., H. Bril El-Haouzi, M. Morin, J. Gaudreault, and P. Thomas. 2023. “Toward Digital Twins for Sawmill Production Planning and Control: Benefits, Opportunities, and Challenges.” International Journal of Production Research 61(7): 2190–2213.
- *Chen, R. Y. 2017. “An Intelligent Value Stream-Based Approach to Collaboration of Food Traceability Cyber Physical System by Fog Computing.” Food Control 71: 124–136.
- *Coelho, F., S. Relvas, and A. P. Barbosa-Póvoa. 2021. “Simulation-based Decision Support Tool for in-House Logistics: The Basis for a Digital Twin.” Computers & Industrial Engineering 153: 107094.
- Damanpour, F., and M. Schneider. 2006. “Phases of the Adoption of Innovation in Organizations: Effects of Environment, Organization and Top Managers.” British Journal of Management 17 (3): 215–236.
- Davis, J., T. Edgar, J. Porter, J. Bernaden, and M. Sarli. 2012. “Smart Manufacturing, Manufacturing Intelligence and Demand-Dynamic Performance.” Computers and Chemical Engineering 47: 145–156.
- *Defraeye, T., C. Shrivastava, T. Berry, P. Verboven, D. Onwude, S. Schudel, and R. M. Rossi. 2021. “Digital Twins are Coming: Will we Need Them in Supply Chains of Fresh Horticultural Produce?” Trends in Food Science & Technology 109: 245–258.
- *Defraeye, T., G. Tagliavini, W. Wu, K. Prawiranto, S. Schudel, M. A. Kerisima, … A. Bühlmann. 2019. “Digital Twins Probe Into Food Cooling and Biochemical Quality Changes for Reducing Losses in Refrigerated Supply Chains.” Resources, Conservation and Recycling 149: 778–794.
- DHL. 2019. “Digital Twins in Logistics.” DHL. https://www.dhl.com/content/dam/dhl/global/core/documents/pdf/glo-core-digital-twins-in-logistics.pdf.
- Dilkes-Hoffman, L. S., J. L. Lane, T. Grant, S. Pratt, P. A. Lant, and B. Laycock. 2018. “Environmental Impact of Biodegradable Food Packaging When Considering Food Waste.” Journal of Cleaner Production 180: 325–334.
- Dolgui, A., and D. Ivanov. 2023. “Metaverse Supply Chain and Operations Management.” International Journal of Production Research 61(23): 8179–8191.
- Fang, Z., Y. Zhao, R. D. Warner, and S. K. Johnson. 2017. “Active and Intelligent Packaging in Meat Industry.” Trends in Food Science and Technology 61 (2): 60–71.
- *Franceschi, P., S. Mutti, K. Ottogalli, D. Rosquete, D. Borro, and N. Pedrocchi. 2022. “A Framework for Cyber-Physical Production System Management and Digital Twin Feedback Monitoring for Fast Failure Recovery.” International Journal of Computer Integrated Manufacturing 35 (6): 619–632.
- Fritz, M., and G. Schiefer. 2009. “Tracking, Tracing, and Business Process Interests in Food Commodities: A Multi-Level Decision Complexity.” International Journal of Production Economics 117 (2): 317–329.
- *Gallo, A., R. Accorsi, A. Goh, H. Hsiao, and R. Manzini. 2021. “A Traceability-Support System to Control Safety and Sustainability Indicators in Food Distribution.” Food Control 124 (October 2020): 107866.
- Garaus, M., and H. Treiblmaier. 2021. “The Influence of Blockchain-Based Food Traceability on Retailer Choice: The Mediating Role of Trust.” Food Control 129 (February): 108082.
- Gardas, B. B., R. D. Raut, N. Cheikhrouhou, and B. E. Narkhede. 2019. “A Hybrid Decision Support System for Analyzing Challenges of the Agricultural Supply Chain.” Sustainable Production and Consumption 18: 19–32.
- *Gebhardt, M., M. Kopyto, H. Birkel, and E. Hartmann. 2022. “Industry 4.0 Technologies as Enablers of Collaboration in Circular Supply Chains: A Systematic Literature Review.” International Journal of Production Research 60 (23): 6967–6995.
- Ghadge, A., M. Bourlakis, S. Kamble, and S. Seuring. 2023. “Blockchain Implementation in Pharmaceutical Supply Chains: A Review and Conceptual Framework.” International Journal of Production Research 61 (9): 6633–6651.
- Ghadge, A., S. Dani, and R. Kalawsky. 2012. “Supply Chain Risk Management: Present and Future Scope.” The International Journal of Logistics Management 23 (3): 313–339.
- Ghadge, A., H. Wurtmann, and S. Seuring. 2020. “Managing Climate Change Risks in Global Supply Chains: A Review and Research Agenda.” International Journal of Production Research 58 (1): 44–64.
- *Ghandar, A., A. Ahmed, S. Zulfiqar, Z. Hua, M. Hanai, and G. Theodoropoulos. 2021. “A Decision Support System for Urban Agriculture Using Digital Twin: A Case Study with Aquaponics.” IEEE Access 9: 35691–35708.
- Glaessgen, E., and D. Stargel. 2012. “The Digital Twin Paradigm for Future NASA and US Air Force Vehicles.” 53rd AIAA/ASME/ASCE/AHS/ASC structures, structural dynamics and materials conference 20th AIAA/ASME/AHS adaptive structures conference 14th AIAA (p. 1818).
- Gokarn, S., and T. S. Kuthambalayan. 2017. “Analysis of Challenges Inhibiting the Reduction of Waste in Food Supply Chain.” Journal of Cleaner Production 168: 595–604.
- *Greif, T., N. Stein, and C. M. Flath. 2020. “Peeking Into the Void: Digital Twins for Construction Site Logistics.” Computers in Industry 121: 103264.
- Hameed, M. A., S. Counsell, and S. Swift. 2012. “A Conceptual Model for the Process of IT Innovation Adoption in Organizations.” Journal of Engineering and Technology Management 29 (3): 358–390.
- *Hang, L., I. Ullah, and D. H. Kim. 2020. “A Secure Fish Farm Platform Based on Blockchain for Agriculture Data Integrity.” Computers and Electronics in Agriculture 170: 105251.
- Hellström, T. 2003. “Systemic Innovation and Risk: Technology Assessment and the Challenge of Responsible Innovation.” Technology in Society 25 (3): 369–384.
- Huff, S. L., M. C. Munro, and B. S. L. Huff. 2014. “Information Technology Assessment and Adoption: A Field Study.” MIS Quarterly 9 (4): 327–340.
- Hosseini, S., D. Ivanov, and A. Dolgui. 2020. “Ripple Effect Modelling of Supplier Disruption: Integrated Markov Chain and Dynamic Bayesian Network Approach.” International Journal of Production Research 58 (11): 3284–3303.
- *Ivanov, D. 2020. “Predicting the Impacts of Epidemic Outbreaks on Global Supply Chains: A Simulation-Based Analysis on the Coronavirus Outbreak (COVID-19/SARS-CoV-2) Case.” Transportation Research Part E: Logistics and Transportation Review 136: 101922.
- *Ivanov, D. 2023. “Conceptualisation of a 7-Element Digital Twin Framework in Supply Chain and Operations Management.” International Journal of Production Research 1-13: 0020–7543.
- *Ivanov, D., and A. Dolgui. 2021. “A Digital Supply Chain Twin for Managing the Disruption Risks and Resilience in the era of Industry 4.0.” Production Planning and Control 32 (9): 775–788.
- *Ivanov, D., A. Dolgui, and B. Sokolov. 2019. “The Impact of Digital Technology and Industry 4.0 on the Ripple Effect and Supply Chain Risk Analytics.” International Journal of Production Research 57 (3): 829–846.
- Jans-Singh, M., K. Leeming, R. Choudhary, and M. Girolami. 2020. “Digital Twin of an Urban-Integrated Hydroponic Farm.” Data-Centric Engineering, 1: e20.
- Janssen, S. J., C. H. Porter, A. D. Moore, I. N. Athanasiadis, I. Foster, J. W. Jones, and J. M. Antle. 2017. “Towards a new Generation of Agricultural System Data, Models and Knowledge Products: Information and Communication Technology.” Agricultural Systems 155: 200–212.
- *Jiang, Y., X. Liu, K. Kang, Z. Wang, R. Y. Zhong, and G. Q. Huang. 2021. “Blockchain-enabled Cyber-Physical Smart Modular Integrated Construction.” Computers in Industry 133: 103553.
- *Kamble, S. S., A. Gunasekaran, H. Parekh, V. Mani, A. Belhadi, and R. Sharma. 2022. “Digital Twin for Sustainable Manufacturing Supply Chains: Current Trends, Future Perspectives, and an Implementation Framework.” Technological Forecasting and Social Change 176: 121448.
- *Kamilaris, A., A. Fonts, and F. X. Prenafeta-Boldύ. 2019. “The Rise of Blockchain Technology in Agriculture and Food Supply Chains.” Trends in Food Science & Technology 91: 640–652.
- Kamilaris, A., A. Kartakoullis, and F. X. Prenafeta-Boldú. 2017. “A Review on the Practice of big Data Analysis in Agriculture.” Computers and Electronics in Agriculture 143: 23–37.
- Kirkman, D. M. 2012. “Social Enterprises: An Multi-Level Framework of the Innovation Adoption Process.” Innovation 14 (1): 143–155.
- *Kittipanya-ngam, P., and K. H. Tan. 2020. “A Framework for Food Supply Chain Digitalization: Lessons from Thailand.” Production Planning and Control 31 (2-3): 158–172.
- *Kong, T., T. Hu, T. Zhou, and Y. Ye. 2021. “Data Construction Method for the Applications of Workshop Digital Twin System.” Journal of Manufacturing Systems 58: 323–328.
- Korth, B., C. Schwede, and M. Zajac. 2018, December. “Simulation-ready Digital Twin for Realtime Management of Logistics Systems.” In 2018 IEEE International Conference on big Data (Big Data), 4194–4201. Seattle, WA, USA: IEEE.
- *Kuo, Y. H., F. Pilati, T. Qu, and G. Q. Huang. 2021. “Digital Twin-Enabled Smart Industrial Systems: Recent Developments and Future Perspectives.” International Journal of Computer Integrated Manufacturing 34 (7-8): 685–689.
- La Scalia, G., L. Settanni, R. Micale, and M. Enea. 2016. “Predictive Shelf Life Model Based on RF Technology for Improving the Management of Food Supply Chain: A Case Study.” International Journal of RF Technologies 7 (1): 31–42.
- *Leung, E. K. H., C. K. H. Lee, and Z. Ouyang. 2022. “From Traditional Warehouses to Physical Internet Hubs: A Digital Twin-Based Inbound Synchronization Framework for PI-Order Management.” International Journal of Production Economics 244: 108353.
- *Li, M., Z. Li, X. Huang, and T. Qu. 2021. “Blockchain-based Digital Twin Sharing Platform for Reconfigurable Socialized Manufacturing Resource Integration.” International Journal of Production Economics 240: 108223.
- *Lim, K. Y. H., P. Zheng, and C. H. Chen. 2020. “A State-of-the-art Survey of Digital Twin: Techniques, Engineering Product Lifecycle Management and Business Innovation Perspectives.” Journal of Intelligent Manufacturing 31: 1313–1337.
- *Liu, J., X. Cao, H. Zhou, L. Li, X. Liu, P. Zhao, and J. Dong. 2021. “A Digital Twin-Driven Approach Towards Traceability and Dynamic Control for Processing Quality.” Advanced Engineering Informatics 50: 101395.
- Love, P. E. D., and Z. Irani. 2004. “An Exploratory Study of Information Technology Evaluation and Benefits Management Practices of SMEs in the Construction Industry.” Information and Management 42 (1): 227–242.
- *Lugaresi, G., and A. Matta. 2021. “Automated Manufacturing System Discovery and Digital Twin Generation.” Journal of Manufacturing Systems 59: 51–66.
- *Maheshwari, P., S. Kamble, A. Belhadi, V. Mani, and A. Pundir. 2022. “Digital Twin Implementation for Performance Improvement in Process Industries- A Case Study of Food Processing Company.” International Journal of Production Research, 61 (23): 8343–8365.
- *Maheshwari, P., S. Kamble, S. Kumar, A. Belhadi, and S. Gupta. 2023. “Digital Twin-Based Warehouse Management System: A Theoretical Toolbox for Future Research and Applications.” The International Journal of Logistics Management 0957–4093.
- Malik, P. K., R. Sharma, R. Singh, A. Gehlot, S. C. Satapathy, W. S. Alnumay, D. Pelusi, U. Ghosh, and J. Nayak. 2021. “Industrial Internet of Things and Its Applications in Industry 4.0: State of The Art.” Computer Communications 166: 125–139.
- *Mandolla, C., A. M. Petruzzelli, G. Percoco, and A. Urbinati. 2019. “Building a Digital Twin for Additive Manufacturing Through the Exploitation of Blockchain: A Case Analysis of the Aircraft Industry.” Computers in Industry 109: 134–152.
- Manos, B., and I. Manikas. 2010. “Traceability in the Greek Fresh Produce Sector: Drivers and Constraints.” British Food Journal 112 (6): 640–652.
- Manzini, R., and R. Accorsi. 2013. “The new Conceptual Framework for Food Supply Chain Assessment.” Journal of Food Engineering 115 (2): 251–263.
- *Matzembacher, D. E., I. do Carmo Stangherlin, L. A. Slongo, and R. Cataldi. 2018. “An Integration of Traceability Elements and Their Impact in Consumer’s Trust.” Food Control 92: 420–429.
- Melesse, T. Y., V. Di Pasquale, and S. Riemma. 2021. “Digital Twin Models in Industrial Operations: State-of-the-art and Future Research Directions.” IET Collaborative Intelligent Manufacturing 3 (1): 37–47.
- Microsoft. 2017. “The Promise of a Digital Twin Strategy.” https://info.microsoft.com/The-promise-of-a-digital-twin-strategyBest-practices-for-designers-Registration-ForminBody.html.
- Nakandala, D., H. Lau, and L. Zhao. 2017. “Development of a Hybrid Fresh Food Supply Chain Risk Assessment Model.” International Journal of Production Research 55 (14): 4180–4195.
- *Nguyen, T., Q. H. Duong, T. Van Nguyen, Y. Zhu, and L. Zhou. 2022. “Knowledge Mapping of Digital Twin and Physical Internet in Supply Chain Management: A Systematic Literature Review.” International Journal of Production Economics 244: 108381.
- Oliveira, M. M. de, A. Lago, and G. P. Dal’ Magro. 2021. “Food Loss and Waste in the Context of the Circular Economy: A Systematic Review.” Journal of Cleaner Production 294: 126284.
- *Onwude, D., F. Bahrami, C. Shrivastava, T. Berry, P. Cronje, J. North, N. Kirsten, et al. 2022. “Physics-driven Digital Twins to Quantify the Impact of pre- and Postharvest Variability on the end Quality Evolution of Orange Fruit.” Resources, Conservation and Recycling 186: 106585.
- Opoku, D. G. J., S. Perera, R. Osei-Kyei, and M. Rashidi. 2021. “Digital Twin Application in the Construction Industry: A Literature Review.” Journal of Building Engineering 40 (May): 102726.
- Orji, I. J., S. Kusi-Sarpong, S. Huang, and D. Vazquez-Brust. 2020. “Evaluating the Factors that Influence Blockchain Adoption in the Freight Logistics Industry.” Transportation Research Part E: Logistics and Transportation Review 141(April).
- *Óskarsdóttir, K., and G. V. Oddsson. 2019. “Towards a Decision Support Framework for Technologies Used in Cold Supply Chain Traceability.” Journal of Food Engineering 240: 153–159.
- *Pan, Y. H., T. Qu, N. Q. Wu, M. Khalgui, and G. Q. Huang. 2021. “Digital Twin Based Real-Time Production Logistics Synchronization System in a Multi-Level Computing Architecture.” Journal of Manufacturing Systems 58: 246–260.
- *Park, K. T., Y. H. Son, and S. D. Noh. 2021. “The Architectural Framework of a Cyber Physical Logistics System for Digital-Twin-Based Supply Chain Control.” International Journal of Production Research 59 (19): 5721–5742.
- *Parreño-Marchante, A., A. Alvarez-Melcon, M. Trebar, and P. Filippin. 2014. “Advanced Traceability System in Aquaculture Supply Chain.” Journal of Food Engineering 122: 99–109.
- *Perno, M., L. Hvam, and A. Haug. 2022. “Implementation of Digital Twins in the Process Industry: A Systematic Literature Review of Enablers and Barriers.” Computers in Industry 134: 103558.
- Pichlak, M. 2016. “The Innovation Adoption Process: A Multidimensional Approach.” Journal of Management & Organization 22 (4): 476–494.
- *Piramuthu, S., P. Farahani, and M. Grunow. 2013. “RFID-generated Traceability for Contaminated Product Recall in Perishable Food Supply Networks.” European Journal of Operational Research 225 (2): 253–262.
- *Pylianidis, C., S. Osinga, and I. N. Athanasiadis. 2021. “Introducing Digital Twins to Agriculture.” Computers and Electronics in Agriculture 184: 105942.
- Qian, J., L. Ruiz-Garcia, B. Fan, J. I. Robla Villalba, U. McCarthy, B. Zhang, Q. Yu, and W. Wu. 2020. “Food Traceability System from Governmental, Corporate, and Consumer Perspectives in the European Union and China: A Comparative Review.” Trends in Food Science and Technology 99 (January): 402–412.
- Queiroz, M. M., and S. Fosso Wamba. 2021. “A Structured Literature Review on the Interplay Between Emerging Technologies and COVID-19–Insights and Directions to Operations Fields.” Annals of Operations Research, 1–27.
- Rabah, S., A. Assila, E. Khouri, F. Maier, F. Ababsa, V. Bourny, P. Maier, and F. Mérienne. 2018. “Towards Improving the Future of Manufacturing Through Digital Twin and Augmented Reality Technologies.” Procedia Manufacturing 17: 460–467.
- *Regattieri, A., M. Gamberi, and R. Manzini. 2007. “Traceability of Food Products: General Framework and Experimental Evidence.” Journal of Food Engineering 81 (2): 347–356.
- *Ringsberg, H. 2014. “Perspectives on Food Traceability: A Systematic Literature Review.” Supply Chain Management: An International Journal 19 (5/6): 558–576.
- *Rožanec, J. M., J. Lu, J. Rupnik, M. Škrjanc, D. Mladenić, B. Fortuna, X. Zheng, and D. Kiritsis. 2022. “Actionable Cognitive Twins for Decision Making in Manufacturing.” International Journal of Production Research 60 (2): 452–478.
- Salin, V. 1998. “Information Technology in Agri-Food Supply Chains.” The International Food and Agribusiness Management Review 1 (3): 329–334.
- *Semeraro, C., M. Lezoche, H. Panetto, and M. Dassisti. 2021. “Digital Twin Paradigm: A Systematic Literature Review.” Computers in Industry 130: 103469.
- *Shankar, R., R. Gupta, and D. K. Pathak. 2018. “Modeling Critical Success Factors of Traceability for Food Logistics System.” Transportation Research Part E: Logistics and Transportation Review 119: 205–222.
- *Shoji, K., S. Schudel, D. Onwude, C. Shrivastava, and T. Defraeye. 2022a. “Mapping the Postharvest Life of Imported Fruits from Packhouse to Retail Stores Using Physics-Based Digital Twins.” Resources, Conservation and Recycling 176: 105914.
- *Shoji, K., S. Schudel, C. Shrivastava, D. Onwude, and T. Defraeye. 2022b. “Optimizing the Postharvest Supply Chain of Imported Fresh Produce with Physics-Based Digital Twins.” Journal of Food Engineering 329: 111077.
- Simangunsong, E., L. C. Hendry, and M. Stevenson. 2012. “Supply-chain Uncertainty: A Review and Theoretical Foundation for Future Research.” International Journal of Production Research 50 (16): 4493–4523.
- Singh, R. K., S. Luthra, S. K. Mangla, and S. Uniyal. 2019. “Applications of Information and Communication Technology for Sustainable Growth of SMEs in India Food Industry.” Resources, Conservation and Recycling 147: 10–18.
- *Singh, G., R. Rajesh, Y. Daultani, and S. Chandra. 2023. “Resilience and Sustainability Enhancements in Food Supply Chains Using Digital Twin Technology: A Grey Causal Modelling (GCM) Approach.” Computers & Industrial Engineering 179: 109172.
- Sreedevi, R., and H. Saranga. 2017. “Uncertainty and Supply Chain Risk: The Moderating Role of Supply Chain Flexibility in Risk Mitigation.” International Journal of Production Economics 193 (July 2015): 332–342.
- *Sun, S., and X. Wang. 2019. “Promoting Traceability for Food Supply Chain with Certification.” Journal of Cleaner Production 217: 658–665.
- Tagliavini, G., T. Defraeye, and J. Carmeliet. 2019. “Multiphysics Modeling of Convective Cooling of non-Spherical, Multi-Material Fruit to Unveil Its Quality Evolution Throughout the Cold Chain.” Food and Bioproducts Processing 117: 310–320.
- Tao, F., J. Cheng, Q. Qi, M. Zhang, H. Zhang, and F. Sui. 2018. “Digital Twin-Driven Product Design, Manufacturing and Service with Big Data.” International Journal of Advanced Manufacturing Technology 94 (9–12): 3563–3576.
- *Tao, F., F. Sui, A. Liu, Q. Qi, M. Zhang, B. Song, … A. Y. Nee. 2019a. “Digital Twin-Driven Product Design Framework.” International Journal of Production Research 57 (12): 3935–3953.
- *Tao, F., H. Zhang, A. Liu, and A. Y. C. Nee. 2019b. “Digital Twin in Industry: State-of-the-Art.” IEEE Transactions on Industrial Informatics 15 (4): 2405–2415.
- Tian, F. 2016, June. “An Agri-Food Supply Chain Traceability System for China Based on RFID & Blockchain Technology.” 2016 13th international conference on service systems and service management (ICSSSM) (pp. 1–6). IEEE.
- *Tozanli, Ö, and M. J. Saénz. 2022. “Unlocking the Potential of Digital Twins in Supply Chains.” MIT Sloan Management Review 63 (4): 1–4.
- Tran, T. A., and T. Daim. 2008. “A Taxonomic Review of Methods and Tools Applied in Technology Assessment.” Technological Forecasting and Social Change 75 (9): 1396–1405.
- Tranfield, D., D. Denyer, and P. Smart. 2003. “Towards a Methodology for Developing Evidence-Informed Management Knowledge by Means of Systematic Review.” British Journal of Management 14 (3): 207–222.
- Van der Valk, H., G. Strobel, S. Winkelmann, J. Hunker, and M. Tomczyk. 2022. “Supply Chains in the Era of Digital Twins-A Review.” Procedia Computer Science 204: 156–163.
- Verdouw, C. N., J. Wolfert, A. J. M. Beulens, and A. Rialland. 2016. “Virtualization of Food Supply Chains with the Internet of Things.” Journal of Food Engineering 176: 128–136.
- *Vilas-Boas, J. L., J. J. P. C. Rodrigues, and A. M. Alberti. 2022. “Convergence of Distributed Ledger Technologies with Digital Twins, IoT, and AI for Fresh Food Logistics: Challenges and Opportunities.” Journal of Industrial Information Integration 100393.
- Vu, N., A. Ghadge, and M. Bourlakis. 2023. “Blockchain Adoption in Food Supply Chains: A Review and Implementation Framework.” Production Planning and Control 34 (6): 506–523.
- *Wong, E. Y. C., D. Y. Mo, and S. So. 2021. “Closed-loop Digital Twin System for Air Cargo Load Planning Operations.” International Journal of Computer Integrated Manufacturing 34 (7–8): 801–813.
- World Health Organization. 2007. “Food safety and Foodborne Illness.” Available at: https://www.who.int/news-room/fact-sheets/detail/food-safety.
- *Wu, W., L. Shen, Z. Zhao, M. Li, and G. Q. Huang. 2022a. “Industrial IoT and Long Short-Term Memory Network-Enabled Genetic Indoor-Tracking for Factory Logistics.” IEEE Transactions on Industrial Informatics 18 (11): 7537–7548.
- *Wu, W., Z. Zhao, L. Shen, X. T. R. Kong, D. Guo, R. Y. Zhong, and G. Q. Huang. 2022b. “Just Trolley: Implementation of Industrial IoT and Digital Twin-Enabled Spatial-Temporal Traceability and Visibility for Finished Goods Logistics.” Advanced Engineering Informatics 52: 101571.
- Xu, L., F. Jia, X. Lin, and L. Chen. 2023. “The Role of Technology in Supply Chain Decarbonisation: Towards an Integrated Conceptual Framework.” Supply Chain Management: An International Journal 28 (4): 803–824.
- Yadav, V. S., A. R. Singh, A. Gunasekaran, R. D. Raut, and B. E. Narkhede. 2022a. “A Systematic Literature Review of the Agro-Food Supply Chain: Challenges, Network Design, and Performance Measurement Perspectives.” Sustainable Production and Consumption 29: 685–704.
- Zhu, K., K. L. Kraemer, and S. Xu. 2006. “The Process of Innovation Assimilation by Firms in Different Countries: A Technology Diffusion Perspective on e-Business.” Management Science 52 (10): 1557–1576.
- *Yadav, V. S., A. R. Singh, R. D. Raut, S. K. Mangla, S. Luthra, and A. Kumar. 2022b. “Exploring the Application of Industry 4.0 Technologies in the Agricultural Food Supply Chain: A Systematic Literature Review.” Computers and Industrial Engineering 169: 108304.
- *Zhang, Z., Z. Guan, Y. Gong, D. Luo, and L. Yue. 2022. “Improved Multi-Fidelity Simulation-Based Optimisation: Application in a Digital Twin Shop Floor.” International Journal of Production Research 60 (3): 1016–1035.
- Zhang, L., Y. Xu, P. Oosterveer, and A. P. J. Mol. 2016. “Consumer Trust in Different Food Provisioning Schemes: Evidence from Beijing, China.” Journal of Cleaner Production 134: 269–279.
- Zhao, G., S. Liu, C. Lopez, H. Lu, S. Elgueta, H. Chen, and B. M. Boshkoska. 2019. “Blockchain Technology in Agri-Food Value Chain Management: A Synthesis of Applications, Challenges and Future Research Directions.” Computers in Industry 109: 83–99.
- *Zhao, Z., L. Shen, C. Yang, W. Wu, M. Zhang, and G. Q. Huang. 2021a. “IoT and Digital Twin Enabled Smart Tracking for Safety Management.” Computers & Operations Research 128: 105183.
- Zhao, X., P. Wang, and R. Pal. 2021b. “The Effects of Agro-Food Supply Chain Integration on Product Quality and Financial Performance: Evidence from Chinese Agro-Food Processing Business.” International Journal of Production Economics 231 (December 2019): 107832.
- *Zhao, Z., M. Zhang, J. Chen, T. Qu, and G. Q. Huang. 2022. “Digital Twin-Enabled Dynamic Spatial-Temporal Knowledge Graph for Production Logistics Resource Allocation.” Computers and Industrial Engineering 171.
- *Zheng, X., J. Lu, and D. Kiritsis. 2022. “The Emergence of Cognitive Digital Twin: Vision, Challenges and Opportunities.” International Journal of Production Research 60 (24): 7610–7632.
- Zhou, X., M. Pullman, and Z. Xu. 2022. “The Impact of Food Supply Chain Traceability on Sustainability Performance.” Operations Management Research 15 (1-2): 93–115.