ABSTRACT
The drilling quality of carbon fibre reinforced polymer (CFRP) components is a key factor affecting the service life of the components, while energy saving and emission reduction in industrial production are crucial. In this study, drilling experiments were conducted on T300 plywood using a 55° coated tungsten steel drill bit, and CNN-LSTM neural network models were used to construct mapping relationships between process parameters (spindle speed, feed rate, and fibre lay-up sequence) and delamination factor and machine energy consumption. A new method of predicting the delamination factor by process parameters is proposed, and explored the optimal process parameter combinations that reduce the energy consumption of machine tools and minimise the delamination factor at the same time. The research results show that within the parameter settings, a spindle speed of 7000 r/min, a feed rate of 40 mm/min, and a lay-up sequence of [0°, 0°, −45°, 90°]6s ensure both low power consumption in the drilling process and the highest possible hole quality. This paper clearly demonstrates the feasibility of achieving low-power, high-quality drilling of CFRP through parameter optimisation, providing guidance to the manufacturing industry to improve the quality of CFRP hole-making while easing the pressure on carbon emissions.
Disclosure statement
No potential conflict of interest was reported by the author(s).
Data availability statement
Full data are available on request from the authors.
Additional information
Funding
Notes on contributors
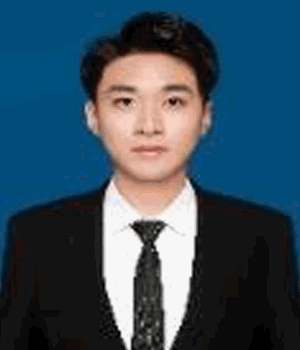
Huang Shunhu
Huang Shunhu is a master's student. His current research interests include green manufacturing and manufacturing system energy efficiency. He has authored or co-authored over several academic papers in Journal of Mechanical Engineering, and other international journals and conference proceedings.
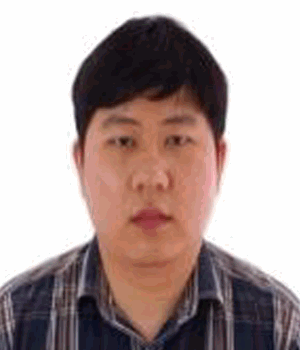
Ma Feng
Ma Feng received the Ph.D. degree in mechanical engineering from Wuhan University of Science & Technology, China, in 2019. His current research interests include green manufacturing and manufacturing system energy efficiency. He has authored or co-authored over10 academic papers in Journal of Cleaner Production, International Journal of Production Research and other international journals and conference proceedings.
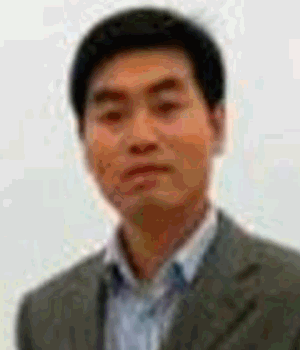
Gong Qingshan
Gong Qingshan received the Ph.D. degree in mechanical engineering from Wuhan University of Science & Technology, China, in 2019. And currently working at Hubei Automotive University. His current research interests include green manufacturing and Remanufacturing. He has published over 20 scientific papers in international journals and conference proceedings.
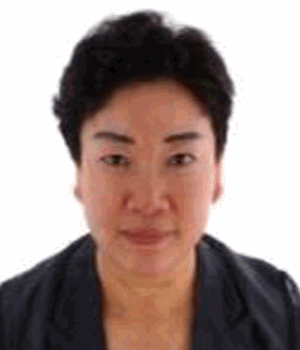
Zhang Hua
Zhang Hua received the Ph.D. degree in mechanical engineering from Chongqing University, China, in 2000, and is currently the Dean of Green Manufacturing Engineering Research Institute, Wuhan University of Science and Technology, the vice-director of the Green Manufacturing Technical Committee (SAC/TC146/SC2) of Standardization Administration of China, and the member of Sino-UK LCMC. Her current research interests include green manufacturing and sustainable manufacturing. Moreover, she presided over more than 30 national scientific research projects and published over 100 scientific papers in international journals and conference proceedings.