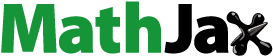
Abstract
This research examines the transformative potential of artificial intelligence (AI) in general and Generative AI (GAI) in particular in supply chain and operations management (SCOM). Through the lens of the resource-based view and based on key AI capabilities such as learning, perception, prediction, interaction, adaptation, and reasoning, we explore how AI and GAI can impact 13 distinct SCOM decision-making areas. These areas include but are not limited to demand forecasting, inventory management, supply chain design, and risk management. With its outcomes, this study provides a comprehensive understanding of AI and GAI's functionality and applications in the SCOM context, offering a practical framework for both practitioners and researchers. The proposed framework systematically identifies where and how AI and GAI can be applied in SCOM, focussing on decision-making enhancement, process optimisation, investment prioritisation, and skills development. Managers can use it as a guidance to evaluate their operational processes and identify areas where AI and GAI can deliver improved efficiency, accuracy, resilience, and overall effectiveness. The research underscores that AI and GAI, with their multifaceted capabilities and applications, open a revolutionary potential and substantial implications for future SCOM practices, innovations, and research.
1. Introduction
The accelerated advancement of Artificial Intelligence (AI), illustrated by the introduction of tools such as ChatGPT (OpenAI Citation2023a), GitHub Copilot (Github Citation2023), and DALL-E (OpenAI Citation2023b), has garnered a mix of excitement, intrigue, and apprehension (The White House Citation2022). These technologies belong to the realm of Generative AI (GAI), a branch of Machine Learning (ML) that can create new content, including text, images, music, or video, by learning patterns from existing data (Brynjolfsson, Li, and Raymond Citation2023). The remarkable strides in GAI can be attributed to four elements: increased computing power, pioneering model architecture, the potential for ‘pre-training’ using vast quantities of unlabelled data, and advancements in training techniques (Brynjolfsson, Li, and Raymond Citation2023). A model's performance heavily hinges on its scale, which is influenced by the amount of computing power utilised for training, the number of model parameters, and dataset size (Kaplan et al. Citation2020). Pre-training large language models (LLMs) involves significant resources, with thousands of GPUs working for weeks to months. For instance, a single training run for a GPT-3 model, with its 175 billion parameters trained on 300 billion tokens, is estimated to cost $5 million in computing alone (Brown et al. Citation2020). It's worth mentioning that the GPT-3 model, although substantial, is eclipsed by the undisclosed size and cost of GPT-4, which powers ChatGPT (OpenAI Citation2023c).
This progression of size and computational capacity has catalysed a significant increase in productivity. A study conducted by Harvard Business School (HBS) and the consulting firm Boston Consulting Group (BCG) revealed that individuals utilising ChatGPT-4 at BCG demonstrated superior performance across all measured dimensions compared to their peers (Dell'Acqua et al. Citation2023). The benefits of AI augmentation were evident across the skills spectrum, with consultants below the average threshold experiencing a remarkable 43% increase, and those above witnessing a 17% improvement in their scores, regardless of the performance metrics used. Likewise, Goldman Sachs' recent report suggests GAI could potentially uplift global GDP by 7%, an immense impact for a single technology (Financial Times Citation2023). The analysis of various use cases and the share of the workforce engaging in primarily cognitive tasks deems this projection plausible, though the ultimate productivity and growth effects of AI remain uncertain. For instance, a study by Noy and Zhang (Citation2023) illustrated that ChatGPT notably boosts worker productivity for midlevel professional writing tasks. Another study by Brynjolfsson, Li, and Raymond (Citation2023) demonstrated a 14% productivity rise for call centre operators using GAI, with the least experienced workers seeing gains over 30%. Interestingly, customer sentiment improved when interacting with operators aided by GAI, possibly contributing to reduced employee attrition.
According to Hulten's theorem, in competitive markets, the impact of a productivity increase in a specific sector on overall productivity and output equals the magnitude of the productivity surge multiplied by the size of the sector (Hulten Citation1978). Hence, as concluded by Baily, Brynjolfsson, and Korinek (Citation2023), if GAI enhances the productivity of cognitive workers by an average of 30% over a couple of decades and cognitive work contributes to about 60% of the economy's total value (as indicated by the wage bill for cognitive tasks), this translates into an 18% augmentation in aggregate productivity and output over the same period.
As disruptive technologies, AI and GAI will lead to a surge in productivity and revolutionise Supply Chain and Operations Management (SCOM) (Ivanov et al. Citation2021; Richey Jr et al. Citation2023; Sheffi Citation2023), extending its limits and irreversibly altering the job landscape. We are already witnessing these transformations. At the time of writing this paper, Walmart is harnessing GAI to automatically negotiate optimal prices with some vendors (Bloomberg Citation2023). Simultaneously, Maersk's Chief Technology and Information Officer has indicated the shipping giant's plan to integrate GAI substantially into its business operations (CNBC Citation2023). The adoption of GAI is not limited to retail and shipping industries. For instance, DHL is keen on harnessing ChatGPT, with a vision to automate processes and enhance efficiency in logistics, from warehouse operations to driver's cabins (DHL Citation2023). Meanwhile, Instacart, a leading grocery delivery service in the USA has collaborated with OpenAI to integrate ChatGPT to allow customers to efficiently shop, request recipes, and process orders for delivery (Instacart Citation2023). Additionally, the potential for GAI's application in supply chain communication and decision-making is further highlighted by recent insights from the Wall Street Journal (Young Citation2023), indicating a growing interest and experimentation in the field.
However, despite the enthusiasm of the early adopters, there is an observable gap in the theoretical preparedness of the SCOM discipline to accommodate this impending revolution (Hendriksen Citation2023). Some researchers have begun to explore the potential that digitalisation and AI offer in enhancing supply chain efficiency (Perano et al. Citation2023; Richey Jr et al. Citation2023). Other studies have shed light on the capabilities of AI systems in mitigating disruptions in the wake of the COVID-19 pandemic (Nayal et al. Citation2022). There are also promising attempts to analyse AI's potential in manufacturing and Industry 4.0 applications (Rai et al. Citation2021), and to understand its potential synergies with other disruptive technologies (Ivanov et al. Citation2021).
These perspectives provide valuable insight, but they tend to focus on AI's application without necessarily viewing the technology through the lens of its functional capabilities. As such, they may not fully capture the breadth and depth of disruption that AI tools can bring to SCOM. Therefore, in the face of such technological disruption, it becomes essential to reflect on the fundamental questions:
RQ1: How does GAI enhance the capabilities of traditional AI in the context of SCOM?
RQ2: What are the practical implications of AI and GAI capabilities for the future of Supply Chain and Operations Management?
By answering the stated research questions, our intention is to illuminate the multifaceted nature of AI, discern the unique contributions of GAI, and understand their practical implications within the diverse domains of Supply Chain Operations Management (SCOM). By systematically addressing these inquiries, we strive to establish the Capability-based Framework for analysing and implementing AI and GAI in SCOM. This framework is intended to serve as a crucial tool for both researchers and practitioners, enabling them to identify existing research gaps and devise appropriate methodologies. Our ultimate goal is to facilitate an effective dialogue between AI capabilities and SCOM areas, thereby fostering robust research trajectories and impactful applications within the field.
The remainder of this paper is organised as follows: We first introduce state-of-the-art AI and its capabilities, followed by an in-depth exploration of GAI. Subsequently, we present a framework centred on AI and GAI capabilities within the SCOM context. After that, a comprehensive discussion follows, encompassing managerial implications and avenues for future research. We conclude the paper by summarising our key findings and insights.
2. Background
As the dawn of AI transforms from potential into reality, it is crucial to understand and acknowledge the broad spectrum of capabilities this technology holds. In the quest to define AI's capabilities, it is indispensable to encompass the insights from the originators, visionaries, and practitioners who have been steering the evolution of this field. The AI pioneers, prominent scientists, philosophers of AI, leading tech companies, and consulting firms each carry a unique perspective shaped by their experiences and areas of expertise (see Appendix 1 for exact definitions). Alongside these perspectives, integrating the Resource-Based View (RBV) is crucial. RBV, a strategic framework focussing on internal resources for competitive advantage, highlights how AI's capabilities can be leveraged as unique, valuable, and inimitable resources within organisations, particularly in SCOM (Fan et al. Citation2022). Weaving together these multifaceted insights, this section aims to unravel and discuss not only the definitions and interpretations of AI's capabilities but also their strategic implications within the RBV framework. This approach seeks to establish a comprehensive and nuanced understanding of state-of-the-art AI, positioning it as a pivotal resource in the ever-evolving landscape of SCOM and setting the stage for future advancements in this dynamic field.
2.1. AI capabilities through the lens of the resource-Based view
The Resource-Based View (RBV) is a strategic framework that focuses on the internal resources of a firm to achieve a sustainable competitive advantage. Originating from Barney (Citation1991), the RBV posits that firms with valuable, rare, inimitable, and non-substitutable resources are more likely to maintain competitive superiority (Barney Citation1991). This perspective has been widely acknowledged and applied in various fields, including SCOM (Fan et al. Citation2022; Hitt, Xu, and Matz Carnes Citation2016; Ketchen Jr, Wowak, and Craighead Citation2014; Schroeder, Bates, and Junttila Citation2002).
Within the RBV framework, capabilities are considered a special type of resource. They are organisationally embedded, non-transferable, firm-specific resources that enhance the productivity of other resources within the firm (Makadok Citation2001). This perspective aligns well with the rapidly evolving field of AI, where capabilities developed through technological advancement become pivotal in achieving strategic goals. In the RBV context, the advent of AI as a pivotal digital technology fundamentally alters the landscape of strategic managerial resources. RBV underscores the importance of valuable, rare, inimitable, and non-substitutable resources in achieving competitive superiority. In this light, AI and GAI, in particular, challenge traditional notions within RBV, particularly concerning human cognitive capabilities, which have long been seen as a source of competitive advantage due to their unique and scarce nature (Helfat and Peteraf Citation2003; Kraaijenbrink, Spender, and Groen Citation2010; Kunc and Morecroft Citation2010). It is highlighted that the integration of AI in decision-making processes signifies a shift, suggesting that firms may need to diversify their managerial skills to harness AI's potential effectively (Krakowski, Luger, and Raisch Citation2023). The role of AI in RBV is twofold: it can either substitute or complement human cognitive capabilities. While its substitution may erode the traditional advantages attributed to human skills due to AI's low marginal reproduction costs and minimal imitation barriers (Brynjolfsson and McAfee Citation2014), its complementary role can create new strategic advantages by combining human expertise with AI's capabilities (Agrawal, Gans, and Goldfarb Citation2018). This duality underscores the need for a nuanced understanding of AI in organisational strategy and resource management (Krakowski, Luger, and Raisch Citation2023). As such, AI in general and GAI in particular serve not only as a technological tool but also as a driver for redefining and reconfiguring strategic resources within the RBV framework, especially in dynamic environments like SCOM.
Incorporating dynamic capabilities (Teece, Pisano, and Shuen Citation1997) into the RBV enhances our understanding of how organisations can leverage AI for strategic advantage. Dynamic capabilities, defined as the firm's ability to adapt, integrate, and reconfigure internal and external skills and resources, are crucial in environments marked by rapid technological change (Helfat and Peteraf Citation2003; Teece, Pisano, and Shuen Citation1997). In the context of RBV, these capabilities enable organisations to possess valuable resources like AI and effectively utilise them in alignment with evolving market conditions and operational challenges.
2.2. Defining the core AI capabilities
By analysing the definitions of AI, we could identify such core capabilities as Learning, Perception, Prediction, Interaction, Adaptation, and Reasoning (see Appendix 1). The capabilities are defined and further theoretically justified as follows:
Learning. The AI system learns from data to predict, analyse, and make decisions. The capability includes Supervised Learning (Hastie et al. Citation2009), Unsupervised Learning (Hastie et al. Citation2009), Semi-Supervised Learning (Berthelot et al. Citation2019), and Transfer Learning (Torrey and Shavlik Citation2010).
Perception. AI's capability to understand and interpret the world by mimicking human senses. The capability includes Computer Vision (Chai et al. Citation2021), Audio Processing (Purwins et al. Citation2019), and Natural Language Processing (Otter, Medina, and Kalita Citation2020).
Prediction. AI's ability to forecast future outcomes based on historical data and patterns (Wu, Zhang, and Zhou Citation2022). This capability extends beyond just numerical or categorical data, spanning text, images, audio, and more, and includes regression (Mitra, Saha, and Kumar Tiwari Citation2023), classification (Shahin et al. Citation2023), time series forecasting (Doganis, Aggelogiannaki, and Sarimveis Citation2008), and anomaly detection (Kim and Kim Citation2023).
Interaction. AI interacts and makes decisions in an environment, including interactions with humans. The capability includes Reinforcement Learning (Rolf et al. Citation2023) and Human-AI Interaction (Panagou, Neumann, and Fruggiero Citation2023).
Adaptation. AI's ability to adapt and improve over time based on new data and changing environments. The capability includes Continuous Learning (Li et al. Citation2023) and Evolutionary Algorithms (Xiao et al. Citation2014).
Reasoning. AI's ability to reason, plan and make decisions, which is crucial for complex tasks. The capability includes Symbolic Reasoning (Brooks Citation1991), Planning (Leo Kumar Citation2019), and Decision Making (McDonnell, Joshi, and Qiu Citation2005).
Table differentiates definitions of AI based on the capabilities. It is important to highlight that the overall taxonomy of AI capabilities has some overlaps with Samoili et al. (Citation2020). The definitions are aligned with Choi et al. (Citation2022) and Ivanov et al. (Citation2021) in order to fit better in SCOM context. It is essential to emphasise that these capabilities hardly exist in isolation; they often need to work together in an integrated manner to realise the full potential of AI in SCOM.
Table 1. Core AI Capabilities gleaned from the various definitions of AI.
2.3. GAI leads to new capabilities
Viewing through the lens of RBV, competitive advantage is traditionally linked to the possession of valuable, rare, inimitable, and non-substitutable resources such as GAI technologies (Krakowski, Luger, and Raisch Citation2023). However, the true competitive edge extends beyond merely owning these strategic resources to dynamically leveraging them (Helfat et al. Citation2023; Helfat and Peteraf Citation2003). This perspective necessitates continuously adapting AI and GAI capabilities to align with new market trends, seamlessly integrating them into organisational processes, and reconfiguring them to address emerging challenges in SCOM.
At its core, GAI models aim to understand and mimic the underlying distribution of a given dataset, enabling the generation of novel content that closely resembles the original data. These models, including Generative Adversarial Networks (GANs) (Goodfellow et al. Citation2014), Variational Autoencoders (VAEs) (Kingma and Welling Citation2019), and Transformer-based architectures (Vaswani et al. Citation2017) like GPT (OpenAI Citation2023a) and DALL-E (OpenAI Citation2023b), are revolutionising diverse aspects of AI, from Learning and Perception to Prediction, Interaction, Adaptation, and Reasoning. Understanding the algorithms and models that form the backbone of GAI offers a window into the future, providing us with the tools to envision how GAI can enhance existing AI capabilities. The models' ability to generate diverse and complex outputs has far-reaching implications, particularly when these capabilities are applied to various elements of supply chain and operations management. As we delve into the specific capabilities of AI and how GAI can augment them, we begin to see a future where AI's ability to learn, perceive, predict, interact, adapt, and reason is exponentially expanded, opening new possibilities for innovation, efficiency, and resilience in supply chain and operations management. Please refer to Appendix 2 for rigorous description and technical details.
2.3.1. Learning enhanced by GAI
GAI models, notably those developed from advanced machine learning frameworks such as GANs and VAEs, hinge on learning as a fundamental mechanism (OpenAI Citation2017). Their operating principle is to capture the essence of the training data and model its distribution to generate new, original content. This unique capability gives rise to exciting opportunities such as the creation of synthetic datasets, an invaluable asset when real data is limited, non-existent, or subject to privacy concerns.
The capacity to create synthetic data not only extends the breadth of information accessible for AI models to learn from but also potentially enhances the diversity of data. This, in turn, can reduce bias and improve the generalizability of AI systems. These generated datasets can reflect real-world complexities while preserving the privacy and anonymity necessary in many use cases (Hacker, Engel, and Mauer Citation2023).
Learning in GAI is further empowered by the application of Transformer models (Yu et al. Citation2022). These models are renowned for their attention mechanism, which allows them to assign varying weights of importance to different parts of the input. This means that the model can recognise and understand long-range dependencies in the data, significantly augmenting its learning ability. Transformers, for example, underpin the sophisticated language model GPT, enabling it to generate coherent, contextually relevant text or computer code (Jackson and Rolf Citation2023; Jackson, Saenz, and Ivanov Citation2023) over extended passages.
2.3.2. Perception enhanced by GAI
GAI contributes significantly to enhancing AI's Perception capability, as evident in various applications across diverse fields. In the realm of computer vision, generative models demonstrate a profound capability to fabricate entirely new images or manipulate existing ones (Han et al. Citation2022). These models can, for instance, increase resolution, remove noise, fill in missing parts, or even alter the style of images, creating an indispensable tool in many digital imaging and medical imaging tasks (Bi, Zhu, and Meng Citation2021).
In the sphere of natural language processing (NLP), Transformer models such as GPT have set a new benchmark in text generation. These models generate text so realistic that it is often indistinguishable from human-written text, spanning from simple sentences to full-length articles. Their ability to capture the complexity of language semantics and syntax showcases an exceptional capacity to perceive and comprehend complex patterns, akin to human language understanding (Floridi and Chiriatti Citation2020).
Extending this capability, generative models have shown remarkable success in code generation (Jackson, Saenz, and Ivanov Citation2023), a task that similarly involves perceiving and understanding complex patterns. Models like Codex (Xu et al. Citation2022) and Copilot (Github Citation2023), using the Transformer architecture, can produce functional code based on specific instructions or requirements. This advancement could revolutionise software development, making it more efficient and accessible.
2.3.3. Prediction enhanced by GAI
GAI holds significant potential to enhance AI's predictive abilities by creating realistic future scenarios based on historical data patterns. For instance, Lee, Cheon, and Hwang (Citation2021) illustrates the application of GAN architectures to generate time-series data. In the context of supply chain and operations management, these models can generate plausible projections of future data points, such as demand forecasts, inventory requirements, available capacity, or supplier performance assessments.
The generated scenarios could offer nuanced insights, facilitating comprehensive contingency planning. This fact can support strategic decision-making by modelling a range of possible outcomes, thereby illuminating potential opportunities and risks (Jo Citation2023). Consequently, it aids in building more robust and resilient supply chain systems and operational management structures that can withstand unexpected disruptions.
2.3.4. Interaction enhanced by GAI
GAI models, especially those that leverage the Transformer architecture like the GPT series, are revolutionising the interaction between humans and AI. These models generate contextually appropriate and human-like responses, making AI more conversational and, thus, more user-friendly (Jo Citation2023).
This capability finds a myriad of applications (Dwivedi et al. Citation2023). In customer service, for instance, AI can generate human-like responses to handle queries, significantly enhancing customer experience. Similarly, in the realm of virtual assistants, these models can produce more natural and contextually relevant responses, increasing user engagement and satisfaction. The human-AI interaction facilitated by GAI could profoundly impact various industries, creating new avenues for businesses to interact with customers and gather valuable insights.
2.3.5. Adaptation enhanced by GAI
Adaptation forms an integral part of GAI, especially in GANs. In these models, two networks – a generator and a discriminator – are involved in a continuous learning process from each other (Goodfellow et al. Citation2014). This learning dynamic creates an evolving learning environment within the model, enabling it to refine and improve its generation capability over time.
While this aspect mainly pertains to the model's internal learning dynamics, it emphasises the inherent adaptability of GAI models. This adaptability signifies their potential to respond to new data or changing environments over time, a critical requirement for many real-world applications.
2.3.6. Reasoning enhanced by GAI
GAI models, although primarily known for their content generation abilities, have shown potential to indirectly support tasks that require Reasoning (Epstein et al. Citation2023). For instance, they can generate simulations or scenarios that assist in the planning and decision-making processes (Vanhaelen, Lin, and Zhavoronkov Citation2020).
These models' ability to create a variety of complex scenarios infuses an additional layer of depth and realism into the reasoning process. For example, in supply chain and operations management, they can simulate various operational conditions or market dynamics, providing a more comprehensive basis for strategic decisions. As such, GAI can significantly contribute to enabling more robust and effective decision-making outcomes.
2.3.7. Unleashing artificial ‘Creativity’ with GAI
One of the most transformative new capabilities endowed by GAI is the ability to generate creative content, a faculty often reserved for the human intellect. Be it penning an engaging article, designing an image from a text description, or even composing harmonious melodies, these models have displayed a level of creativity previously unimagined in the realm of AI (Brynjolfsson, Li, and Raymond Citation2023). This development indicates a paradigm shift in the capabilities of AI models, blurring the lines between human creativity and machine learning (Jo Citation2023). We assign the name Artificial ‘Creativity’ to this newfound capability. It signifies the potential of AI systems to concoct original, useful, and often unexpected ideas or outputs that closely mirror the manifestations of human creativity (Brynjolfsson, Li, and Raymond Citation2023). These outputs could range from the creation of unique designs and solutions to the generation of innovative strategies in complex fields such as Supply Chain and Operations Management (SCOM). In the context of SCOM, this artificial creativity can be harnessed to simulate new market scenarios, develop alternative operational strategies, or predict unexpected demand trends. By using GAI to imagine diverse situations or solutions, businesses can use a creative toolbox for tackling strategic problems or operational bottlenecks. However, it's vital to note that this AI-enabled ‘Creativity’ is an extension of the model's training on vast datasets and its capacity to recombine learned elements in innovative ways (Dwivedi et al. Citation2023). It is essentially a data-driven process where the AI model leverages patterns and structures from the data it was trained on to generate unique and creative solutions. It doesn't involve personal experiences or subjective interpretations as human creativity often does. In this context, it's essential to remember that, despite its remarkable capabilities, GAI does not possess cognition in the way humans do. The concept of creativity when applied to AI, therefore, remains a functional term referring to the capacity of these models to generate novel and potentially useful outputs. We consciously abstain from delving into philosophical discussions regarding AI cognition and the essence of true creativity. In this paper, our focus lies in a practical orientation toward AI, emphasising its capabilities and the corresponding real-world applications. We aim to explore how GAI, with its unprecedented creative abilities, can be applied to transform various aspects of SCOM, paving the way for more robust, innovative, and efficient solutions in this domain.
3. Framework based on AI and GAI capabilities in SCOM
Supply chain engineering and management are burning topics in the industry (Dolgui and Proth Citation2010; Ivanov and Sokolov Citation2009). The rapid development and integration of AI and GAI into these industries necessitate a shift in the manner we comprehend and analyse these technological advancements (Brynjolfsson, Li, and Raymond Citation2023; Jo Citation2023). A new perspective, which emphasises the capabilities of these technologies rather than simply the underlying algorithms or paradigms, offers a more practical and instrumental viewpoint, especially for the field of SCOM (Choi, Wallace, and Wang Citation2018; Mithas et al. Citation2022).
AI and GAI, characterised by their capabilities, can be viewed as functional tools designed to fulfill certain roles or perform specific tasks. This perspective is especially suited to SCOM, where the emphasis lies on the functional aspects and the effects of technology on the operations and management of the supply chain (Kumar, Mookerjee, and Shubham Citation2018). Through the lens of capabilities, AI and GAI can be studied in terms of their functionality: Learning, Perception, Prediction, Interaction, Adaptation, Reasoning, and Creativity.
To build a framework that allows for the systematic analysis of AI and GAI applications in SCOM, we draw upon the seminal work of Ivanov et al. (Citation2021). This work outlines critical areas within SCOM in the context of Industry 4.0, including but not limited to Demand Forecasting, Distribution and Transportation Strategy, Inventory Management and Warehousing, Process Design, Production Planning and Control, Production Strategy, Quality Management, Revenue Management, Sales and Operations Planning, Scheduling and Routing, Sourcing Strategy, Supply Chain Design, and Supply Chain Risk Management. Since AI and GAI in particular form integral parts of the Industry 4.0 concept (Olsen and Tomlin Citation2020), we maintain that the areas identified by Ivanov et al. (Citation2021) retain their validity and applicability within the AI and GAI context. Figure provides a high-level view of the proposed framework and illustrates the core areas of SCOM as well as identified AI and GAI Capabilities.
Figure 1. High-level view of the proposed framework and its critical elements.
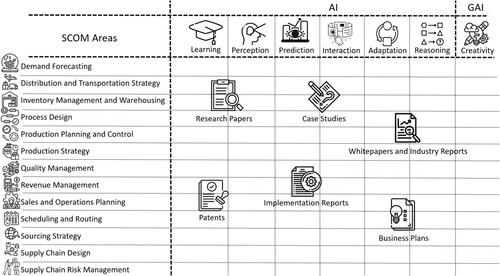
In this section, we illustrate how our proposed framework can be applied to dissect existing applications of AI and GAI in SCOM. Table provides a matrix that maps these applications against the capabilities and SCOM areas, presenting a snapshot of the current landscape of AI and GAI applications in SCOM. Nevertheless, we assert that our study does not aim for exhaustive coverage of the AI and GAI applications in SCOM. Instead, the matrix should serve as a guiding example, elucidating how the proposed framework can be utilised to systematically study and explore AI and GAI in SCOM.
Table 2. Analyzing AI and GAI Capabilities in SCOM areas using the proposed framework.
3.1. Forecasting
3.1.1. Demand forecasting
Demand Forecasting is one of the pivotal activities in supply chain and operations management, serving as the compass that guides inventory, production, and distribution decisions (Li and Li Citation2022). In recent years, the role of AI in refining this key process has become increasingly significant. AI algorithms are now widely used for demand forecasting, leveraging vast datasets to unravel intricate patterns and make predictions. With their ability to learn and adapt over time, these AI models increase forecasting accuracy and contribute to efficient and agile operations management, optimising the overall supply chain process (Jackson and Ivanov Citation2023; Mithas et al. Citation2022).
For example, Chien, Lin, and Lin (Citation2020) addresses demand uncertainty in semiconductor distribution. The Learning capability is embodied by the use of deep reinforcement learning. The model learns from data patterns to determine the best forecasting model for each product, echoing aspects of supervised learning as a reward feedback mechanism drives it. The Prediction capability is central to the system's function, as it forecasts future demand patterns based on historical data, possibly employing time series forecasting methods. The Interaction capability is evident in the system's use of reinforcement learning, enabling it to make decisions in its operational environment, which includes diverse supply chain entities. The system's ability to dynamically select forecasting models points to its Adaptation capability, as it can adjust its strategies based on evolving demand patterns, indicative of continuous learning. Lastly, the Reasoning capability underpins the system's capacity to choose the optimal demand forecast model for each product, which involves decision-making processes. The AI system reasons, plans, and makes strategic decisions to mitigate the adverse effects of demand uncertainty.
Kantasa-Ard et al. (Citation2021) presents a demand forecasting approach for complex supply chains using a Long Short-Term Memory (LSTM) model, with its hyperparameters tuned by a hybrid of genetic algorithms and scatter search. The study demonstrates the superior performance of the LSTM model in forecasting fluctuating demand, especially when compared to other supervised learning methods, thereby aiding in reducing distribution costs in a physical internet supply chain network. AI's capabilities are manifested in several ways. The Learning capability is demonstrated through the use of LSTM, a Supervised Learning method, which learns from historical demand data to make future predictions. The Prediction capability is central to the paper's theme as the LSTM model is utilised for demand forecasting, a form of time series forecasting. The Interaction capability could be inferred through the AI's engagement with the supply chain environment, making decisions that affect the system's operational efficiency, although this isn't directly addressed. Adaptation is evident in using a hybrid genetic algorithm and scatter search to optimise LSTM's hyperparameters, enabling the model to adjust and improve over time based on new data and different demand scenarios.
Bouquet et al. (Citation2023) explores AI-based predictive frameworks for solar energy management. The proposed solution implementats LSTM model epitomises AI's Learning capability, particularly in Supervised Learning. This capability is harnessed to analyse historical solar electricity generation data, enabling the model to accurately forecast future patterns. The study's focus on refining accuracy in solar electricity generation forecasts further underscores AI's Prediction capability, which is pivotal for enhancing grid reliability and efficiency in the context of Smart Grids. Additionally, the application of the LSTM model in a dynamic energy management environment suggests an implicit use of the Interaction capability, where AI's decisions directly influence operational efficiency. While not explicitly stated, elements of Adaptation and Reasoningare likely at play as the model adjusts to varying data inputs and complex grid scenarios, optimising solar energy management through strategic decision-making and continuous learning.
3.1.2. Production capacity forecasting
Indeed, AI-based forecasting is not limited to demand. Demand is only a part of the flows circulating within the supply chain. That is why forecasting data patterns from the supply side is pivotal. Forecasting production capacity planning is instrumental for end-to-end supply chain planning.
For example, Tremblet, Thevenin, and Dolgui (Citation2023) presents a comprehensive exploration of AI capabilities, particularly emphasising Learning, as it delves into machine learning models like decision trees and artificial neural networks to understand production dynamics. It underscores the Prediction capability by forecasting production capacity utilisation, ensuring that production plans align with real-world constraints. The paper illustrates a tool that aids production planners in estimating capacity consumption and highlights Reasoning capability, ensuring that decisions are made with regard to available resources. Furthermore, the paper's emphasis on models that swiftly adapt to the intricacies of a manufacturing environment, especially when computational time is limited, which highlights the Adaptation capability.
Another prominent research by Tremblet, Thevenin, and Dolgui (Citation2023) dives into the challenges manufacturers face when using lot-sizing models within advanced planning systems. The paper emphasises the Learning capability by investigating the integration of machine learning to refine capacity consumption approximations. This focus on machine learning showcases the paper's commitment to Prediction, aiming to produce more accurate and implementable production plans. The paper's focus on producing guaranteed feasible plans and its scalability underscores its Adaptation.
3.2. Distribution and transportation strategy
Huang et al. (Citation2023) deals with the optimisation of the shortest path interdiction problem, a significant challenge within the scope of Distribution and Transportation Strategy in SCOM. The core objective is to maximise the length of the shortest pathway a follower can traverse, considering a limited interdiction budget. In this context, strategic decisions influence the follower's choice of the shortest path. The research proposes an innovative solution utilising AI capabilities, specifically Learning, Prediction, Interaction, and Adaptation. The central strategy involves the deployment of a Reinforcement Learning (RL) framework, an AI technique under the Interaction capability. The RL model allows the system to interact with a dynamic environment, where the state and action spaces may be large or continuous. The use of RL underscores the value of AI's Learning and Interaction capabilities in the solution. It also touches on AI's Prediction capability as the model anticipates the follower's choice based on the leader's actions. Alongside the RL framework, a Pointer Network is used to manage variable output sizes, a component linked to the Learning capability of AI, particularly supervised learning. This innovative approach allows the model to learn sequences of varying lengths, helping it adapt to different problem sizes, and demonstrating AI's Adaptation capability. The study extensively tested the performance of the proposed RL model through computational experiments. The tests used instances generated from two distinct network topologies, grid networks and random graphs, representing different practical scenarios in physical distribution and transportation. This rigorous testing exemplifies the Reasoning capability of AI in action, with strategic planning, decision-making, and adaptation to varying scenarios.
3.3. Inventory management and warehousing
Kumar, Mookerjee, and Shubham (Citation2018) presents an innovative approach to addressing pervasive issues in supply chain management, focussing on inventory distortion. It proposes the application of No Code AI in the retail industry as a time and cost-efficient solution. AI capabilities are demonstrated in the following ways. At the heart of this study lies the Learning capability of the AI system. The proposed enables non-technical companies to construct machine learning models based on production quantity and inventory replenishment. This capability to learn from data and predict future patterns is essential in mitigating the prevalent problem of inventory distortion, consequently reducing substantial revenue losses. Prediction is another AI capability highlighted in this study. By building machine learning models using No Code AI, the AI system can forecast future inventory needs based on production quantity. This predictive power is central to tackling inventory distortion, offering valuable foresight to prevent stock-level issues and thereby increase sales.
Jackson, Saenz, and Ivanov (Citation2023) exemplifies an intriguing intersection of AI capabilities, namely Creativity and Interaction, within the realm of logistics simulation model development. Primarily, the novel application of Natural Language Processing (NLP) via the GPT-3 Codex model demonstrates the creative aspect of AI. This AI model, fine-tuned and integrated into a user-friendly interface, uses its creative prowess to generate Python code for simulating queuing and inventory control systems. By taking verbal descriptions as input, the system not only constructs functionally valid simulations but also exhibits an understanding of domain-specific vocabulary, illustrating a form of innovative problem-solving. Moreover, the Interaction capability becomes apparent as the GPT-3 Codex serves as an intelligent intermediary between human inputs and the resultant simulations. Its ability to interpret, translate, and execute domain-specific instructions from a human operator encapsulates the essence of effective Human-AI interaction. This leads to an optimised workflow, allowing experts to focus on high-level strategic aspects, thus integrating AI as a creative and interactive facilitator in simulation model development within logistics.
3.4. Process design
Kusiak (Citation2020) investigates the transformation of manufacturing processes, underscored by advancements in process technology, information technology, and data science. It highlights the role of Convolutional Neural Networks (CNNs) and GANs in developing predictive models for the manufacturing enterprise, following the digital twin concept. The Learning capability of AI is highlighted through the use of CNNs and GANs. These machine learning algorithms learn from the patterns inherent in manufacturing data to generate predictive models. In terms of Perception, the study indirectly hints at the use of CNNs, commonly employed in tasks related to Computer Vision. In a manufacturing context, this could involve tasks like anomaly detection or pattern recognition, which contribute to process efficiency and quality control. The Prediction capability is central to the paper's focus. Through machine learning algorithms, the AI system can forecast future scenarios based on past patterns and trends, thus aiding in strategic decision-making and risk mitigation. The Adaptation capability is indicated by the use of GANs, which inherently involve adaptation as part of their learning process. GANs consist of a generator and a discriminator network, which learn from each other continuously. This points to an inherent adaptive behaviour, allowing the models to refine and optimise their predictions over time based on new data and changing scenarios.
3.5. Production planning and control
Liu et al. (Citation2022) proposes a predictive approach for production progress (PP) in make-to-order manufacturing workshops is proposed, leveraging big data and the Industrial Internet of Things (IIoT) for production data acquisition. This approach demonstrates multiple AI capabilities. The Learning capability is at the heart of the paper's approach, with the use of CNN and LSTM, both of which learn from the massive historical and current order data to make accurate PP predictions. Moreover, the use of transfer learning, a form of supervised learning, is emphasised, allowing the CNN and LSTM models to utilise knowledge learned from previous tasks, thus improving computational efficiency. This method embodies the Adaptation capability, as the models are not trained from scratch each time but adapt based on past learning. The Perception capability is manifested in the CNN's extraction of features from the order data, effectively making sense of the various inputs. Finally, the Prediction capability is central, with the paper addressing the importance of accurate PP forecasting in the context of dynamic optimisation of the production process and ensuring on-time delivery of orders.
3.6. Production strategy
AI has also significantly impacted the production and manufacturing industries within the context of the Industry 4.0 paradigm, which promotes the use of smart devices and data-driven factories (Rai et al. Citation2021).
For example, Chen et al. (Citation2021) proposes a new perspective and harnesses the potential of AI in characterising and modelling strip breakage predictively. It leverages the capabilities of AI, specifically Learning and Prediction, to create an innovative model. In the learning phase, the system uses historical multivariate time-series data from the cold rolling process, extracted in a run-to-failure manner. It incorporates a sliding window strategy for data annotation. This process reflects supervised learning and semi-supervised learning, which is included in the Learning capability of AI. For the prediction phase, breakage-centric features are identified from physics-based approaches, empirical knowledge, and data-driven features. These features embody AI's Perception capability, which includes computer vision, audio processing, and natural language processing. The system applies these features to strip breakage modelling using Recurrent Neural Networks (RNNs). RNNs fall under AI's prediction capability, specialising in recognising underlying patterns in time-series data. It uses regression and time series forecasting, elements of the prediction capability, to model possible strip breakage. The interaction between the AI system and the data and the dynamic environment of manufacturing illustrates AI's Interaction and Adaptation capabilities. The paper discusses an experimental study using real-world data from a cold-rolled electrical steel strip manufacturer, showcasing the effectiveness of the proposed approach, thus highlighting the AI's Reasoning capability in understanding the problem, planning a solution, and making decisions.
3.7. Quality management
Shahin et al. (Citation2023) demonstrates the applications of AI capabilities, particularly Perception and Prediction, in the context of modern manufacturing settings underpinned by Industry 4.0 technologies. The Perception capability of AI is highlighted by the development and deployment of computer vision models designed to autonomously detect and classify damaged packages from their intact counterparts. This capability effectively emulates human visual inspection but performs the task with higher accuracy and speed, which is especially crucial in high-volume production environments. The Prediction capability is embodied in the AI model's ability to forecast potential waste in terms of resources and time and deteriorations in customer satisfaction by preventing damaged packages from proceeding to shipping operations. The YOLO v7 model is utilised here, having demonstrated high precision, accuracy, and recall values in the training and validation stages, thereby supporting the effectiveness of these AI capabilities.
3.8. Revenue management
Ferreira, Simchi-Levi, and Wang (Citation2018) presents a price-based network revenue management problem. The issue revolves around a retailer's objective to maximise revenue from multiple products with limited inventory over a finite selling season. The research addresses the common practice where the demand function contains unknown parameters, which must be learned from sales data. This circumstance employs the Learning and Prediction capabilities of AI.
3.9. Sales and operations planning
AI holds significant potential in optimising and revolutionising the sales and operations process Schlegel, Birkel, and Hartmann (Citation2021).
In the domain of sales and operations planning within SCOM, the ‘beer game’ exemplifies the need for effective supply chain coordination (Kimbrough, Wu, and Zhong Citation2002). The game emulates a decentralised multiagent network, where agents aim to minimise overall costs while working with limited information. Oroojlooyjadid et al. (Citation2022) introduces a novel approach utilising deep reinforcement learning (RL), an instance of AI's Interaction capability, to optimise decision-making in the game. The RL algorithm, once trained, performs in real-time and outperforms traditional strategies, particularly when other agents exhibit human-like, unpredictable behaviour, showcasing AI's Prediction capability. The results of applying this methodology are promising. When interacting with teammates who adhere to a base-stock policy, the deep RL algorithm generates near-optimal order quantities. Intriguingly, the algorithm outperforms the base-stock policy when other agents display more human-like, unpredictable ordering behaviour. These results stand consistent when tested with real-world datasets, emphasising the algorithm's Prediction capability in forecasting and adjusting to human behaviour. The research also demonstrates the AI's Adaptation ability, as it shows that the trained model can robustly accommodate changes in cost coefficients.
3.10. Scheduling and routing
The applications of machine learning are becoming increasingly common and diverse. Bai et al. (Citation2023) provides a comprehensive review of hybrid methods that use machine learning in conjunction with analytical strategies to address the Vehicle Routing Problem (VRP), an intensely studied combinatorial optimisation problem. Despite the disparate fields of relevant research and confusing terminologies, the paper underscores the significant potential of machine learning in enhancing VRP modelling and improving the performance of both online and offline VRP algorithms. The discussion concludes by highlighting the challenges and prospects in VRP research, suggesting a promising role for machine learning in the evolution of VRP solutions.
For example, Tyasnurita, Özcan, and John (Citation2017) explores the application of AI, specifically the capabilities of Learning, Prediction, and Adaptation, in solving the Open Vehicle Routing Problem (OVRP), a task notorious for its computational complexity. The task is achieved through a selection hyper-heuristic, a search method controlling a predetermined set of low-level heuristics. The aim of the study is to create an 'apprentice' hyper-heuristic that learns from an 'expert' hyper-heuristic responses to a training set of problem instances and then applies this learned knowledge to unseen problem instances. Learning capability is demonstrated through the use of a Time Delay Neural Network (TDNN). The TDNN learns from the data generated by the expert hyper-heuristic's decisions on which low-level heuristic to apply during the search process. The AI's Prediction ability is utilised when the TDNN, now trained, predicts which low-level heuristic to apply when confronted with unseen problem instances. This represents a typical application of classification tasks, where the AI predicts a certain class or category (in this case, the suitable low-level heuristic) based on past learning. Adaptation as an AI capability is also present in the study. The TDNN might adapt its selection of low-level heuristics based on the performance of previous decisions, incorporating continuous learning to optimise the solution.
3.11. Sourcing strategy
Van Hoek et al. (Citation2022) describes how Walmart used AI to improve negotiations with its suppliers, a previously inefficient task due to the sheer number of suppliers and the lack of personalisation in the agreements. The AI-powered software, Pactum AI, used a text-based chatbot to interact with suppliers, negotiate terms, and make decisions that benefitted both parties involved. Here, I will provide a breakdown of the AI capabilities displayed in the case as per the framework provided.
The Learning capability is evident in how Walmart employed an ML algorithm through a software product known as Pactum AI to automate negotiations with a multitude of tail-end suppliers. The AI system was trained using predefined scripts in a supervised learning environment, where internal buyers created scenarios for the algorithm to learn from. These scenarios later produced structured scripts that guided suppliers throughout the negotiation process. Interaction comes to the forefront when we consider the negotiation process itself. It's essentially a complex form of interaction where the AI-powered chatbot is engaging with human suppliers on behalf of Walmart. This AI-facilitated negotiation process proved to be not just efficient but also flexible and scalable, going beyond the capabilities of human agents. In this context, the AI system is displaying an advanced form of Human-AI Interaction, learning from each interaction and adjusting its responses according to the feedback received from the supplier. A degree of Creativity, inherent in GAI, is also instrumental in this application. The use of a chatbot in negotiations points to an ability to generate new, contextually appropriate content that mimics human interaction. This creative element can be observed in how the chatbot uses its training to create unique negotiation dialogues with each supplier. The chatbot's ability to adapt its language and negotiation strategies based on the supplier's responses is indicative of the creative potential of GAI.
Amazon Business (Citation2021) recently presented how AI is revolutionising procurement processes in businesses. The Learning and Prediction capabilities of AI are utilised to process large amounts of procurement data and deliver strategic insights. The Amazon Business Spend Visibility tool employs machine learning to analyse and learn from the organisation's buying patterns, which significantly reduces the need for human labour and provides accurate and useful insights for strategic planning. This application of AI supports quicker decision-making and allows professionals to redirect their time to other tasks. AI's Interaction capability is illustrated in how Amazon Business automates the competitive bidding for strategically sourced items and identifies cost-effective alternatives for routine supplies. This not only increases sourcing speed but also enables better pricing and procurement efficiencies. Furthermore, the concept of GAI and its Creative capabilities can be perceived in the context of Amazon Business's personalised buying experience. ML collects data from an individual's on-site behaviour and order history and generates curated search results and relevant recommendations.
3.12. Supply chain design
In the ever-evolving landscape of contemporary business, firms grapple with high competition and dynamic environmental conditions (Puche et al. Citation2016). This flux notably impacts Supply Chain Design, a complex critical area due to multiple intervening factors and their intricate interactions across the supply chain. Navigating such complexity to arrive at optimal configuration often becomes insurmountable (Inman and Blumenfeld Citation2014).
Priore et al. (Citation2019) brings to light the application of AI to simplify these complex scenarios and enable superior management of inventory flow. The study leverages an inductive learning algorithm, an illustration of Supervised Learning, to create a dynamic framework. This framework facilitates the establishment of the most suitable replenishment policies by adeptly adapting to environmental changes – a clear demonstration of AI's Learning and Adaptation capabilities. The utilisation of AI in Learning and Adaptation lends itself remarkably well to the three-echelon supply chain model presented in this paper, which is governed by seven variables – cost structure, demand variability, three lead times, and two partners' inventory policy.
3.13. Supply chain risk management
Supply chain risk management involves strategies aimed at identifying, assessing, mitigating, and monitoring unexpected events that could negatively impact any part of a supply chain. Due to the need for rapid and adaptive decision-making based on vast and complex data sources, supply chain risk management is a promising application area for AI (Baryannis et al. Citation2019).
For example, Wong et al. (Citation2022) emphasises the role of AI, particularly its Learning, Prediction, and Reasoning capabilities, in enhancing the efficiency and agility of supply chain risk management, especially for small to medium-sized enterprises. These enterprises face an ever-changing and volatile business environment, and traditional models struggle to react dynamically to these challenges. AI offers a unique solution, enabling supply chains to not only adapt to these fluctuations but also make informed decisions that could significantly reduce potential costs and resource expenditures. The researchers used AI to examine its impact on supply chain risk management, providing a unique perspective rooted in the resource-based view. They employed a multi-faceted approach that included partial least squares-based structural equation modelling and artificial neural network, showcasing AI's Learning capability in deriving insights from complex datasets. The AI's Predictive capability was exhibited in modelling various potential scenarios that would otherwise remain unanswered by traditional infrastructures. By forecasting different outcomes based on historical patterns and data, AI proved invaluable in guiding decision-making processes under high levels of demand uncertainties, thus helping to mitigate supply chain risks.
4. Discussion
This section provides a discussion regarding the future role and potential of AI and GAI in SCOM and attempts to find the potential interactions with other cutting-edge technologies. Besides, this section sheds light on the managerial implications and outlines promising directions for future research.
4.1. Summary of our findings
Our exploration surfaced AI as a multifaceted technology equipped with capabilities spanning Learning, Perception, Prediction, Interaction, Adaptation, and Reasoning. Moreover, we found GAI to possess the transformative potential to enhance these existing AI capabilities and introduce a new one, namely Creativity. This fact essentially reconfigures the boundaries of AI applications within SCOM.
Our findings resonate with the propositions presented in a recent study by Wamba et al. (Citation2023). Their research states that the perceived benefits of GAI in SCOM intensify after implementation. Besides, the authors suggest that the challenges associated with GAI diminish following its adoption. These findings align with our observation of GAI's transformative potential in enhancing AI capabilities. Additionally, Wamba et al. (Citation2023) highlight that early adopters of GAI in SCO are more inclined to recognise its positive trajectories and potential compared to non-adopters. This fact underscores the importance of early engagement with emerging technologies to fully grasp and harness their transformative capabilities in the SCOM domain. Similarly, one of the remarkable aspects of our findings is the synergistic relationship between AI and the core elements of Industry 4.0 (Dolgui et al. Citation2019). The fourth industrial revolution, characterised by the fusion of digital, biological, and physical worlds, hinges heavily on AI capabilities (Schwab Citation2017). As a result, AI is playing an instrumental role in driving this transformation, creating interconnected, efficient, and intelligent systems (Ivanov and Dolgui Citation2020). Coupled with other cutting-edge technologies such as IoT (Luo, Thevenin, and Dolgui Citation2022), 5G (Dolgui and Ivanov Citation2022), Metaverse (Dolgui and Ivanov Citation2023), Blockchain (Dolgui et al. Citation2020), Big Data (Jahani, Jain, and Ivanov Citation2023) and Cloud technologies, AI is pivotal in powering the digital transformation encapsulated by Industry 4.0 (Ivanov, Dolgui, and Sokolov Citation2022).
For instance, the IoT, with its network of interconnected devices, leverages AI to glean insights from vast amounts of data, thus enhancing decision-making and operational efficiency (Luo, Thevenin, and Dolgui Citation2022). Similarly, the advent of 5G technology significantly boosts AI's ability to interact with and control remote systems, enhancing its capabilities in Interaction and Adaptation (Dolgui and Ivanov Citation2022). Additionally, Metaverse and AI collectively hold the promise of creating entirely new, immersive, and interactive digital experiences (Dolgui and Ivanov Citation2023). Blockchain (Dolgui et al. Citation2020), with its emphasis on transparency and security, complements AI in areas such as supply chain risk management and quality control. Lastly, Cloud and especially Edge technologies serve as a platform to amplify AI's potential, providing the computational power necessary to handle the intensive tasks that AI undertakes (Ivanov, Dolgui, and Sokolov Citation2022).
It is also essential to recognise the transformative power of Intelligent Digital Twins (iDTs) in the context of supply chain resilience (Ivanov Citation2023). iDT is a promising concept of a human-AI system that digitally replicates physical supply chains. Integrating iDTs in SCOM offers a more holistic, adaptive, and proactive approach to managing supply chain disruptions and uncertainties as we transition into an era where digital transformation is paramount. As Industry 5.0 (Ivanov Citation2023) is approaching, the role of AI and specifically GAI, will undoubtedly become even more profound. Industry 5.0 is expected to further enhance the human-machine collaboration that AI and GAI enable, creating even more personalised, efficient, and adaptive systems. Therefore, in our study, we are not merely considering AI and GAI in isolation but as part of a broader technological ecosystem that is fundamentally altering how industries operate. This perspective paints a more holistic picture of the transformative potential of AI and GAI in SCOM.
4.2. Theoretical implications
In the theoretical implications of our study, the RBV provides a strategic lens through which to understand the burgeoning role of AI and GAI in SCOM. RBV posits that the possession of valuable, rare, inimitable, and non-substitutable resources is pivotal for achieving competitive superiority (Helfat and Peteraf Citation2003). In this context, AI, and more specifically GAI, emerge as critical resources, redefining traditional concepts of competitive advantage within the RBV framework.
Such AI capabilities as Learning, Perception, Prediction, Interaction, Adaptation, Reasoning, and Creativity, when viewed through the RBV lens, are not merely technological assets but strategic resources that enhance organisational productivity and innovation. The advent of GAI technologies, such as GANs, VAEs, and Transformer-based architectures, further extends these capabilities, introducing elements like Creativity into the AI repertoire. This evolution suggests a necessary shift in organisational strategies, where firms must dynamically leverage these AI and GAI capabilities to stay competitive. The integration of these advanced technologies necessitates a continuous adaptation to market trends, effective incorporation into existing processes, and a readiness to reconfigure them to meet new challenges in SCOM (Krakowski, Luger, and Raisch Citation2023).
Furthermore, our analysis aligns with the argument presented by Helfat et al. (Citation2023) and Krakowski, Luger, and Raisch (Citation2023), highlighting the need for a nuanced understanding of AI in organisational strategy and resource management. As AI technologies evolve, they not only serve as a technological tool but also as a catalyst for redefining and reconfiguring strategic resources within organisations. This theoretical implication points towards an emerging paradigm in which AI and GAI are not just components of technological infrastructure but are central to the strategic resource base of firms, significantly impacting their capacity for innovation, efficiency, and resilience in SCOM.
4.3. Managerial implications
Our study, especially the development and application of the framework linking AI and GAI capabilities to SCOM areas, holds several implications for supply chain and operations management practitioners. Here are some of the key takeaways:
Identifying Potential Applications: The proposed framework systematically identifies where and how AI and GAI can be applied in SCOM. Managers can use it as a guide to evaluate their operational processes and identify areas where AI and GAI can deliver improved efficiency, accuracy, and overall effectiveness.
Decision-Making Enhancement: The AI and GAI capabilities, particularly Prediction and Reasoning, can greatly augment decision-making processes (Choi, Wallace, and Wang Citation2018). AI's ability to analyse large volumes of data can facilitate more informed, data-driven decisions (Rai et al. Citation2021), while GAI's creative capability can introduce novel solutions and strategies that were not previously considered.
Process Optimization: AI and GAI can be instrumental in refining SCOM processes. For instance, demand forecasting can be significantly enhanced using machine learning, while production planning can be optimised using AI-driven prediction models. Additionally, GAI can generate innovative approaches for inventory management (Jackson, Saenz, and Ivanov Citation2023), sourcing strategies (Bloomberg Citation2023), and other critical SCOM areas (CNBC Citation2023).
Investment Prioritization: Understanding the functional capabilities of AI and GAI can help managers make more informed decisions about where to invest resources. By identifying which capabilities align most closely with their operational needs, managers can prioritise investments in AI technology that offer the greatest potential benefits (Brynjolfsson, Li, and Raymond Citation2023).
Skill Development: As AI and GAI become more embedded in SCOM, there will be a growing need for skills in managing and working with these technologies (Sheffi Citation2023). Organizations will need to invest in training and development to equip their workforce with the necessary skills to effectively utilise AI and GAI tools.
Our study underscores the transformative potential of AI and GAI in SCOM. The proposed framework serves as a critical tool for managers, helping them navigate the complex landscape of AI, identify promising applications, make informed decisions, optimise processes, manage risks, prioritise investments, and build necessary skills within their teams. The integration of AI and GAI in SCOM promises to usher in a new era of innovation, efficiency, and resilience in the field.
4.4. Limitations and future research
Although our study provides valuable insights and a useful tool for SCOM practitioners, it bears several limitations that warrant discussion and serve as a launchpad for future research directions. First, the current study is not an exhaustive literature review but a demonstration of how our proposed framework can be applied to analyse existing AI and GAI applications in SCOM. We focussed on illuminating the functional capabilities of AI and GAI and aligning them within the SCOM realm through a few pertinent examples. While this approach enables us to highlight the transformative potential of AI and GAI in SCOM, it does not encapsulate the full breadth of their applications as depicted in the broader literature. Future research would benefit significantly from employing our proposed framework as a lens for a more comprehensive literature review. Such an endeavour would not only provide a more detailed snapshot of the current landscape of AI and GAI applications in SCOM but also serve to validate and refine the framework.
Second, our framework, while robust and informed by the pioneering work of Ivanov et al. (Citation2021), may benefit from expansion and refinement. The SCOM domain is complex and multifaceted, and while the framework captures a broad range of areas within SCOM, a more granular categorisation could provide deeper insights into specific operational and managerial challenges (Choi, Wallace, and Wang Citation2018). Future research could explore expanding the framework to incorporate more nuanced SCOM categories, thus providing a more detailed mapping of AI and GAI capabilities and their applications.
Finally, our study has primarily considered AI and GAI within the context of SCOM. However, as core components of the broader Industry 4.0 landscape, the roles and impacts of AI and GAI are significantly broader (Dolgui et al. Citation2019). Future research should explore the implications of AI and GAI in driving the transition from Industry 4.0 to Industry 5.0, where human-machine collaboration and the convergence of physical, digital, and biological systems are anticipated to be even more pronounced (Ivanov Citation2023). Such research would deepen our understanding of the role of AI and GAI in this transformative period and the ways in which they can shape the future of industries beyond SCOM.
5. Conclusion
In conclusion, our investigation into the nature of AI, as seen through the lens of RBV, reveals that defining AI transcends a singular dimension. This complexity arises from diverse perspectives encompassing its multifaceted applications and capabilities (Barney Citation1991; Helfat et al. Citation2023). Adopting an instrumental view (Bostrom Citation2016), we focus on AI's functional capabilities – Learning, Perception, Prediction, Interaction, Adaptation, and Reasoning – as crucial elements that drive its application in SCOM.
Further, our analysis of GAI underscores its transformative enhancement of AI's capabilities, particularly through the introduction of Creativity. Pioneering technologies like GANs, VAEs, and transformer-based models expand the boundaries of what AI can achieve, demonstrating GAI's unique ability to generate novel, data-mimicking content.
Finally, applying our framework to integrating AI and GAI within SCOM, we leverage these technologies as functional tools, aligning their diverse capabilities with key SCOM processes. This approach, informed by Ivanov et al. (Citation2021), bridges AI and GAI capabilities with critical SCOM areas, illustrating the significant role AI and GAI play in the context of Industry 4.0. In essence, our exploration, grounded in RBV, showcases how AI's capabilities, enhanced by GAI, become strategic resources that redefine and enrich SCOM practices.
Acknowledgements
The first author of this paper wants to dedicate this work to the cherished memory of his Father and Grandfather. Their unwavering support, motivation, and encouragement have been the cornerstone of his perseverance and success. They continue to inspire beyond their time on this earth.
We would also like to express our gratitude to the two anonymous reviewers and the editors of this manuscript. Their insightful and critical comments were instrumental in enhancing the quality of this work.
Disclosure statement
No potential conflict of interest was reported by the author(s).
Data availability statement
The authors confirm that the data supporting the findings of this study are available.
Additional information
Notes on contributors
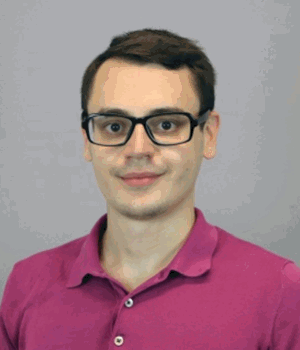
Ilya Jackson
Dr. Ilya Jackson is a Postdoctoral Associate at MIT Center for Transportation & Logistics. He earned his PhD in Civil Engineering and Transportation from the Transport and Telecommunication Institute, where he spent one year as an Assistant Professor shortly after that. The main ideas of his PhD thesis have been summarised in the paper ‘Neuroevolutionary approach to metamodel-based optimisation in production and logistics’, which received the Young Researcher Award in 2020. Dr. Ilya Jackson currently focuses on Machine Learning and AI for Supply Chain Management.
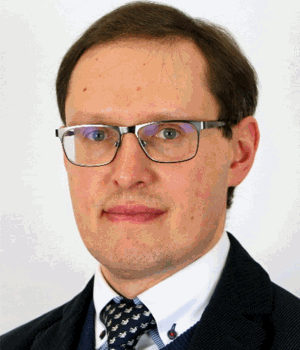
Dmitry Ivanov
Dmitry Ivanov is a professor of supply chain and operations management at Berlin School of Economics and Law. He serves at the school as an Academic director of M.A. Global Supply Chain and Operations Management and B.Sc. International Sustainability Management as well as a Deputy Director of Institute for Logistics. His publication list includes around 400 publications, including over 150 papers in international academic journals and leading textbooks Global Supply Chain and Operations Management and Introduction to Supply Chain Resilience. His main research interests and results span resilience, viability and ripple effect in supply chains, risk analytics, and digital twins. Author of the Viable Supply Chain Model and founder of the ripple effect research in supply chains. Recipient of IISE Transactions Best Paper Award 2021, Best Paper and Most Cited Paper Awards of IJPR (2018,2019, 2020, 2021, 2022), OMEGA Best Paper Award 2022, Clarivate Highly Cited Researcher Award (2021, 2022, 2023). He co-edits IJISM and is an associate editor of the IJPR and OMEGA. He is Chairman of IFAC CC 5 ‘Cyber-Physical Manufacturing Enterprise’.
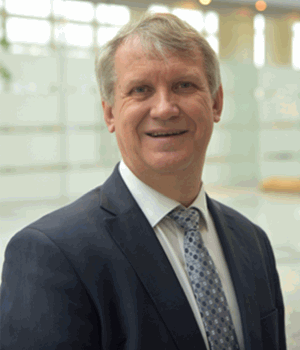
Alexandre Dolgui
Alexandre Dolgui is an IISE Fellow, Distinguished Professor and the Head of Automation, Production and Computer Sciences Department at the IMT Atlantique, Nantes, France. His research and teaching activities focuse on manufacturing line design, production planning and supply chain optimisation. He is the co-author of 5 books, the co-editor of 32 books or conference proceedings, the author of over 310 refereed papers in international journals. He is the Editor-in-Chief of the International Journal of Production Research, an Area Editor of Computers & Industrial Engineering. Member of Board for 27 international journals, including INternational Journal of Production Economics. Former Associate Editor for IEEE Transactions in Industrial Informatics and Omega. He is an Active Fellow of the European Academy for Industrial Management, Member of the Board of the International Foundation for Production Research, former Chair (Vice-Chair now) of IFAC TC 5.2 Manufacturing Modelling for Management and Control, Member of IFIP WG 5.7 Advances in Production Management Systems, and IEEE System Council Analytics and Risk Technical Committee, he has been Scientific Chair of many leading scientific conferences and received several international awards for his research.
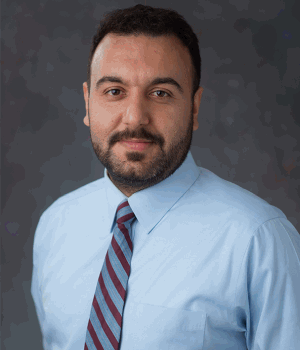
Jafar Namdar
Jafar Namdar is a postdoctoral associate at Massachusetts Institute of Technology (MIT), having earned his Ph.D. in Business Analytics from the Tippie College of Business, University of Iowa. His research focuses on applying business analytics techniques, including Applied Econometrics, Machine Learning (ML), Simulation, and Optimization techniques to address business problems, focussing on supply chain risk management, supply chain digitalisation, policy uncertainty, and political instability. For this purpose, he employs data science tools like web scraping and text analytics to leverage unstructured and distributed data sources, including news, web, conference earning calls, and social media platforms. His work has received interest from professionals and obtained financial support from the corporate and public sectors. Furthermore, he has been invited to participate as a guest speaker at the MIT Industry Liaison Programme (ILP) and several schools to give presentations on supply chain risk management.
References
- Accenture. 2019. “What is Artificial Intelligence?” Accessed July 23, 2023. https://www.accenture.com/us-en/insights/artificial-intelligence-summary-index.
- Agrawal, Ajay, Joshua Gans, and Avi Goldfarb. 2018. Prediction Machines: the Simple Economics of Artificial Intelligence. Boston, MA: Harvard Business Press.
- Agrawal, Ajay, Joshua Gans, and Avi Goldfarb. 2019. The Economics of Artificial Intelligence: An Agenda. Chicago, IL: University of Chicago Press.
- Alammar, Jay. 2018. “The Illustrated Transformer. GitHub Blog, Online.” Available from: https://jalammar.github.io/illustrated-transformer Retrieved 09.09.2023.
- Amazon. 2023. “What is Artificial Intelligence? Machine Learning and Deep Learning.” Accessed July 23, 2023. https://aws.amazon.com/machine-learning/what-is-ai/.
- Amazon Business. 2021. “How AI and Analytics are Transforming Procurement.” Accessed July 23, 2023. https://business.amazon.com/en/discover-more/blog/how-ai-and-ml-are-transforming-procurement.
- Bai, Ruibin, Xinan Chen, Zhi-Long Chen, Tianxiang Cui, Shuhui Gong, Wentao He, and Xiaoping Jiang. 2023. “Analytics and Machine Learning in Vehicle Routing Research.” International Journal of Production Research 61 (1): 4–30. https://doi.org/10.1080/00207543.2021.2013566.
- Baily, Martin Neil, Erik Brynjolfsson, and Anton Korinek. 2023. “Machines of Mind: The Case for An AI-powered Productivity Boom. Brookings.” Accessed July 23, 2023. https://www.brookings.edu/articles/machines-of-mind-the-case-for-an-ai-powered-productivity-boom/.
- Barney, Jay. 1991. “Firm Resources and Sustained Competitive Advantage.” Journal of Management 17 (1): 99–120. https://doi.org/10.1177/014920639101700108.
- Baryannis, George, Sahar Validi, Samir Dani, and Grigoris Antoniou. 2019. “Supply Chain Risk Management and Artificial Intelligence: State of the Art and Future Research Directions.” International Journal of Production Research 57 (7): 2179–2202. https://doi.org/10.1080/00207543.2018.1530476.
- Bengio, Yoshua, Réjean Ducharme, Pascal Vincent, and Christian Jauvin. 2003. “A Neural Probabilistic Language Model.” Journal of Machine Learning Research 3:1137–1155.
- Berthelot, David, Nicholas Carlini, Ian Goodfellow, Nicolas Papernot, Avital Oliver, and Colin A. Raffel. 2019. “Mixmatch: A Holistic Approach to Semi-Supervised Learning.” In Advances in Neural Information Processing Systems. Vol. 32. Red Hook, NY: Curran Associates, Inc.
- Bi, Jiarui, Zengliang Zhu, and Qinglong Meng. 2021. “Transformer in Computer Vision.” In 2021 IEEE International Conference on Computer Science, Electronic Information Engineering and Intelligent Control Technology (CEI), 178–188. Fuzhou, China: IEEE.
- Bishop, Christopher M., and Nasser M. Nasrabadi. 2006. Pattern Recognition and Machine Learning. Vol. 4. New York: Springer.
- Bloomberg. 2023. “Walmart Is Using AI to Negotiate the Best Price With Some Vendors.” Accessed July 23, 2023. https://www.bloomberg.com/news/articles/2023-04-26/walmart-uses-pactum-ai-tools-to-handle-vendor-negotiations#xj4y7vzkg.
- Bostrom, Nick. 2016. “The Control Problem. Excerpts from Superintelligence: Paths, Dangers, Strategies.” In Science Fiction and Philosophy: From Time Travel to Superintelligence, 308–330. Oxford: Oxford University Press.
- Bouquet, Pierre, Ilya Jackson, Mostafa Nick, and Amin Kaboli. 2023. “AI-based Forecasting for Optimised Solar Energy Management and Smart Grid Efficiency.” International Journal of Production Research 1–22. https://doi.org/10.1080/00207543.2023.2269565.
- Brooks, Rodney A. 1991. “Intelligence Without Representation.” Artificial Intelligence 47 (1-3): 139–159. https://doi.org/10.1016/0004-3702(91)90053-M.
- Brown, Tom, Benjamin Mann, Nick Ryder, Melanie Subbiah, Jared D. Kaplan, Prafulla Dhariwal, and Arvind Neelakantan. 2020. “Language Models are Few-shot Learners.” Advances in Neural Information Processing Systems 33:1877–1901.
- Brynjolfsson, Erik, Danielle Li, and Lindsey R. Raymond. 2023. Generative AI at Work. Technical Report. National Bureau of Economic Research.
- Brynjolfsson, Erik, and Andrew McAfee. 2014. The Second Machine Age: Work, Progress, and Prosperity in a Time of Brilliant Technologies. New York: WW Norton & Company.
- Chai, Junyi, Hao Zeng, Anming Li, and Eric W. T. Ngai. 2021. “Deep Learning in Computer Vision: A Critical Review of Emerging Techniques and Application Scenarios.” Machine Learning with Applications 6:100134. https://doi.org/10.1016/j.mlwa.2021.100134.
- Chen, Zheyuan, Ying Liu, Agustin Valera-Medina, Fiona Robinson, and Michael Packianather. 2021. “Multi-faceted Modelling for Strip Breakage in Cold Rolling Using Machine Learning.” International Journal of Production Research 59 (21): 6347–6360. https://doi.org/10.1080/00207543.2020.1812753.
- Chien, Chen-Fu, Yun-Siang Lin, and Sheng-Kai Lin. 2020. “Deep Reinforcement Learning for Selecting Demand Forecast Models to Empower Industry 3.5 and An Empirical Study for a Semiconductor Component Distributor.” International Journal of Production Research 58 (9): 2784–2804. https://doi.org/10.1080/00207543.2020.1733125.
- Choi, Tsan-Ming, Subodha Kumar, Xiaohang Yue, and Hau-Ling Chan. 2022. “Disruptive Technologies and Operations Management in the Industry 4.0 Era and Beyond.” Production and Operations Management 31 (1): 9–31. https://doi.org/10.1111/poms.13622.
- Choi, Tsan-Ming, Stein W. Wallace, and Yulan Wang. 2018. “Big Data Analytics in Operations Management.” Production and Operations Management 27 (10): 1868–1883. https://doi.org/10.1111/poms.12838.
- CNBC. 2023. “A.I. Could ‘Remove All Human Touchpoints’ in Supply Chains. Here's What that Means.” Accessed July 23, 2023. https://www.cnbc.com/2023/06/19/supply-chains-how-ai-could-remove-all-human-touchpoints.html.
- Dell'Acqua, Fabrizio, Edward McFowland, Ethan R. Mollick, Hila Lifshitz-Assaf, Katherine Kellogg, Saran Rajendran, Lisa Krayer, François Candelon, and Karim R. Lakhani. 2023. “Navigating the Jagged Technological Frontier: Field Experimental Evidence of the Effects of AI on Knowledge Worker Productivity and Quality.” Harvard Business School Technology & Operations Mgt. Unit Working Paper (24-013).
- DHL. 2023. “ChatGPT and the Like: Artificial Intelligence in Logistics.” Accessed November 9, 2023. https://dhl-freight-connections.com/en/trends/chatgpt-and-the-like-artificial-intelligence-in-logistics/.
- Doersch, Carl. 2016. “Tutorial on Variational Autoencoders.” Preprint arXiv:1606.05908.
- Doganis, Philip, Eleni Aggelogiannaki, and Haralambos Sarimveis. 2008. “A Combined Model Predictive Control and Time Series Forecasting Framework for Production-inventory Systems.” International Journal of Production Research 46 (24): 6841–6853. https://doi.org/10.1080/00207540701523058.
- Dolgui, Alexandre, and Dmitry Ivanov. 2022. “5G in Digital Supply Chain and Operations Management: Fostering Flexibility, End-to-end Connectivity and Real-time Visibility Through Internet-of-everything.” International Journal of Production Research 60 (2): 442–451. https://doi.org/10.1080/00207543.2021.2002969.
- Dolgui, Alexandre, and Dmitry Ivanov. 2023. “Metaverse Supply Chain and Operations Management.” International Journal of Production Research 61 (23): 8179–8191. https://doi.org/10.1080/00207543.2023.2240900.
- Dolgui, Alexandre, Dmitry Ivanov, Semyon Potryasaev, Boris Sokolov, Marina Ivanova, and Frank Werner. 2020. “Blockchain-oriented Dynamic Modelling of Smart Contract Design and Execution in the Supply Chain.” International Journal of Production Research 58 (7): 2184–2199. https://doi.org/10.1080/00207543.2019.1627439.
- Dolgui, Alexandre, Dmitry Ivanov, Suresh P. Sethi, and Boris Sokolov. 2019. “Scheduling in Production, Supply Chain and Industry 4.0 Systems by Optimal Control: Fundamentals, State-of-the-art and Applications.” International Journal of Production Research 57 (2): 411–432. https://doi.org/10.1080/00207543.2018.1442948.
- Dolgui, Alexandre, and Jean-Marie Proth. 2010. Supply Chain Engineering: Useful Methods and Techniques. Vol. 539. New York: Springer.
- Dwivedi, Yogesh K., Nir Kshetri, Laurie Hughes, Emma Louise Slade, Anand Jeyaraj, Arpan Kumar Kar, and Abdullah M. Baabdullah. 2023. “‘So what If ChatGPT Wrote it?’ Multidisciplinary Perspectives on Opportunities, Challenges and Implications of Generative Conversational AI for Research, Practice and Policy.” International Journal of Information Management 71:102642. https://doi.org/10.1016/j.ijinfomgt.2023.102642.
- Epstein, Ziv, Aaron Hertzmann, Investigators of Human Creativity, Memo Akten, Hany Farid, Jessica Fjeld, and Morgan R. Frank. 2023. “Art and the Science of Generative AI.” Science (New York, N.Y.) 380 (6650): 1110–1111. https://doi.org/10.1126/science.adh4451.
- European Commission. 2018a. “Coordinated Plan on Artificial Intelligence.” Accessed July 23, 2023. https://digital-strategy.ec.europa.eu/en/library/coordinated-plan-artificial-intelligence.
- European Commission. 2018b. “EC JRC Flagship Report on AI: Artificial Intelligence. A European Perspective, 2018.” Accessed July 23, 2023. https://publications.jrc.ec.europa.eu/repository/.
- Fan, Di, Andy C. L. Yeung, Christopher S. Tang, Chris K. Y. Lo, and Yi Zhou. 2022. “Global Operations and Supply-Chain Management Under the Political Economy.”
- Ferreira, Kris Johnson, David Simchi-Levi, and He Wang. 2018. “Online Network Revenue Management Using Thompson Sampling.” Operations Research 66 (6): 1586–1602. https://doi.org/10.1287/opre.2018.1755.
- Financial Times. 2017. “The Mind in The Machine: Demis Hassabis on Artificial Intelligence.” Accessed July 23, 2023. https://www.ft.com/content/048f418c-2487-11e7-a34a-538b4cb30025.
- Financial Times. 2023. “Surrender Your Desk Job to the AI Productivity Miracle, Says Goldman Sachs.” Accessed July 23, 2023. https://www.ft.com/content/50b15701-855a-4788-9a4b-5a0a9ee10561.
- Floridi, Luciano, and Massimo Chiriatti. 2020. “GPT-3: Its Nature, Scope, Limits, and Consequences.” Minds and Machines 30 (4): 681–694. https://doi.org/10.1007/s11023-020-09548-1.
- Fogel, D. B. 1995. “Review of Computational Intelligence: Imitating Life.” Proceedings of the IEEE 83 (11): 1588. https://doi.org/10.1109/JPROC.1995.481636.
- Gharakhanian, Al. 2023. “Generative Adversarial Networks – Hot Topic in Machine Learning.” Accessed September 9, 2023. https://www.kdnuggets.com/2017/01/generative-adversarial-networks-hot-topic-machine-learning.html.
- Github. 2023. “Your AI Pair Programmer.” Accessed July 23, 2023. https://github.com/features/copilot.
- Goertzel, B. 2016. “The AGI Revolution: An Inside View of the Rise of Artificial General Intelligence. New York: Humanity+ Press.
- Goodfellow, Ian, Jean Pouget-Abadie, Mehdi Mirza, Bing Xu, David Warde-Farley, Sherjil Ozair, Aaron Courville, and Yoshua Bengio. 2014. “Generative Adversarial Nets.” Advances in Neural Information Processing Systems 27: 1–15.
- Google. 2023. “What is Artificial Intelligence (AI)?” Accessed July 23, 2023. https://cloud.google.com/learn/what-is-artificial-intelligence.”
- Graves, Alex. 2013. “Generating Sequences with Recurrent Neural Networks.” Preprint arXiv:1308.0850.
- Hacker, Philipp, Andreas Engel, and Marco Mauer. 2023. “Regulating ChatGPT and Other Large Generative AI Models.” In Proceedings of the 2023 ACM Conference on Fairness, Accountability, and Transparency, 1112–1123. Chicago: Association for Computing Machinery.
- Han, Kai, Yunhe Wang, Hanting Chen, Xinghao Chen, Jianyuan Guo, Zhenhua Liu, and Yehui Tang. 2022. “A Survey on Vision Transformer.” IEEE Transactions on Pattern Analysis and Machine Intelligence 45 (1): 87–110. https://doi.org/10.1109/TPAMI.2022.3152247.
- Hastie, Trevor, Robert Tibshirani, Jerome Friedman, Trevor Hastie, Robert Tibshirani, and Jerome Friedman. 2009. “Overview of Supervised Learning.” In The Elements of Statistical Learning: Data Mining, Inference, and Prediction, 9–41. New York: Springer.
- Hastie, Trevor, Robert Tibshirani, Jerome Friedman, Trevor Hastie, Robert Tibshirani, and Jerome Friedman. 2009. “Unsupervised Learning.” In The Elements of Statistical Learning: Data Mining, Inference, and Prediction, 485–585. New York: Springer.
- Helfat, Constance E., Aseem Kaul, David J. Ketchen Jr, Jay B. Barney, Olivier Chatain, and Harbir Singh. 2023. “Renewing the Resource-based View: New Contexts, New Concepts, and New Methods.” Strategic Management Journal 44 (6): 1357–1390. https://doi.org/10.1002/smj.v44.6.
- Helfat, Constance E., and Margaret A. Peteraf. 2003. “The Dynamic Resource-based View: Capability Lifecycles.” Strategic Management Journal 24 (10): 997–1010. https://doi.org/10.1002/smj.v24:10.
- Hendriksen, Christian. 2023. “Artificial Intelligence for Supply Chain Management: Disruptive Innovation Or Innovative Disruption.” Journal of Supply Chain Management 59 (3): 65–76. https://doi.org/10.1111/jscm.v59.3.
- Hitt, Michael A., Kai Xu, and Christina Matz Carnes. 2016. “Resource Based Theory in Operations Management Research.” Journal of Operations Management 41 (1): 77–94. https://doi.org/10.1016/j.jom.2015.11.002.
- Holland, John H. 1975. “Adaptation in Natural and Artificial Systems: An Introductory Analysis with Applications to Biology, Control, and Artificial Intelligence. Ann Arbor, Michigan: University of Michigan Press.
- Huang, Dian, Zhaofang Mao, Kan Fang, and Lin Chen. 2023. “Solving the Shortest Path Interdiction Problem Via Reinforcement Learning.” International Journal of Production Research 61 (1): 31–48. https://doi.org/10.1080/00207543.2021.2002962.
- Hulten, Charles R. 1978. “Growth Accounting with Intermediate Inputs.” The Review of Economic Studies 45 (3): 511–518. https://doi.org/10.2307/2297252.
- Inman, Robert R., and Dennis E. Blumenfeld. 2014. “Product Complexity and Supply Chain Design.” International Journal of Production Research 52 (7): 1956–1969. https://doi.org/10.1080/00207543.2013.787495.
- Instacart. 2023. “Introducing the Instacart Plugin for ChatGPT.” Accessed November 9, 2023. https://www.instacart.com/company/updates/instacart-chatgpt/.
- Ivanov, Dmitry. 2023. “The Industry 5.0 Framework: Viability-based Integration of the Resilience, Sustainability, and Human-centricity Perspectives.” International Journal of Production Research 61 (5): 1683–1695. https://doi.org/10.1080/00207543.2022.2118892.
- Ivanov, Dmitry. 2023. “Intelligent Digital Twin (iDT) for Supply Chain Stress-testing, Resilience, and Viability.” International Journal of Production Economics 263:108938. https://doi.org/10.1016/j.ijpe.2023.108938.
- Ivanov, Dmitry, and Alexandre Dolgui. 2020. “Viability of Intertwined Supply Networks: Extending the Supply Chain Resilience Angles Towards Survivability. A Position Paper Motivated by COVID-19 Outbreak.” International Journal of Production Research 58 (10): 2904–2915. https://doi.org/10.1080/00207543.2020.1750727.
- Ivanov, Dmitry, Alexandre Dolgui, and Boris Sokolov. 2022. “Cloud Supply Chain: Integrating Industry 4.0 and Digital Platforms in the ‘Supply Chain-as-a-Service’.” Transportation Research Part E: Logistics and Transportation Review 160:102676. https://doi.org/10.1016/j.tre.2022.102676.
- Ivanov, Dmitry, and Boris Sokolov. 2009. “Adaptive Supply Chain Management. Berlin, Germany: Springer Science & Business Media.
- Ivanov, Dmitry, Christopher S. Tang, Alexandre Dolgui, Daria Battini, and Ajay Das. 2021. “Researchers' Perspectives on Industry 4.0: Multi-disciplinary Analysis and Opportunities for Operations Management.” International Journal of Production Research 59 (7): 2055–2078. https://doi.org/10.1080/00207543.2020.1798035.
- Jackson, Ilya, and Dmitry Ivanov. 2023. “A Beautiful Shock? Exploring the Impact of Pandemic Shocks on the Accuracy of AI Forecasting in the Beauty Care Industry.” Transportation Research Part E: Logistics and Transportation Review 180:103360. https://doi.org/10.1016/j.tre.2023.103360.
- Jackson, Ilya, and Benjamin Rolf. 2023. “Do Natural Language Processing Models Understand Simulations? Application of GPT-3 to Translate Simulation Source Code to English.” IFAC-PapersOnLine 56 (2): 221–226. https://doi.org/10.1016/j.ifacol.2023.10.1572.
- Jackson, Ilya, Maria Jesus Saenz, and Dmitry Ivanov. 2023. “From Natural Language to Simulations: Applying AI to Automate Simulation Modelling of Logistics Systems.” International Journal of Production Research 1–24. https://doi.org/10.1080/00207543.2023.2276811.
- Jahani, Hamed, Richa Jain, and Dmitry Ivanov. 2023. “Data Science and Big Data Analytics: A Systematic Review of Methodologies Used in the Supply Chain and Logistics Research.” Annals of Operations Research 1–58.
- Jo, A. 2023. “The Promise and Peril of Generative AI.” Nature 614 (1): 214–216.
- Kantasa-Ard, Anirut, Maroua Nouiri, Abdelghani Bekrar, Abdessamad Ait el Cadi, and Yves Sallez. 2021. “Machine Learning for Demand Forecasting in the Physical Internet: a Case Study of Agricultural Products in Thailand.” International Journal of Production Research 59 (24): 7491–7515. https://doi.org/10.1080/00207543.2020.1844332.
- Kaplan, Jared, Sam McCandlish, Tom Henighan, Tom B. Brown, Benjamin Chess, Rewon Child, Scott Gray, et al. 2020. “Scaling Laws for Neural Language Models.” Preprint arXiv:2001.08361.
- Ketchen Jr, David J., Kaitlin D. Wowak, and Christopher W. Craighead. 2014. “Resource Gaps and Resource Orchestration Shortfalls in Supply Chain Management: The Case of Product Recalls.” Journal of Supply Chain Management 50 (3): 6–15. https://doi.org/10.1111/jscm.2014.50.issue-3.
- Kim, Hyojoong, and Heeyoung Kim. 2023. “Deep Embedding Kernel Mixture Networks for Conditional Anomaly Detection in High-dimensional Data.” International Journal of Production Research 61 (4): 1101–1113. https://doi.org/10.1080/00207543.2022.2027040.
- Kimbrough, Steven O., Dong-Jun Wu, and Fang Zhong. 2002. “Computers Play the Beer Game: Can Artificial Agents Manage Supply Chains.” Decision Support Systems 33 (3): 323–333. https://doi.org/10.1016/S0167-9236(02)00019-2.
- Kingma, Diederik P., and Max Welling. 2019. “An Introduction to Variational Autoencoders.” In Foundations and Trends in Machine Learning, Vol. 12, 307–392. Maryland: Now Publishers.
- Kraaijenbrink, Jeroen, J.-C. Spender, and Aard J. Groen. 2010. “The Resource-based View: A Review and Assessment of Its Critiques.” Journal of Management 36 (1): 349–372. https://doi.org/10.1177/0149206309350775.
- Krakowski, Sebastian, Johannes Luger, and Sebastian Raisch. 2023. “Artificial Intelligence and the Changing Sources of Competitive Advantage.” Strategic Management Journal 44 (6): 1425–1452. https://doi.org/10.1002/smj.v44.6.
- Kullback, Solomon, and Richard A. Leibler. 1951. “On Information and Sufficiency.” The Annals of Mathematical Statistics 22 (1): 79–86. https://doi.org/10.1214/aoms/1177729694.
- Kumar, Subodha, Vijay Mookerjee, and Abhinav Shubham. 2018. “Research in Operations Management and Information Systems Interface.” Production and Operations Management 27 (11): 1893–1905. https://doi.org/10.1111/poms.12961.
- Kunc, Martin H., and John D. W. Morecroft. 2010. “Managerial Decision Making and Firm Performance Under a Resource-based Paradigm.” Strategic Management Journal 31 (11): 1164–1182. https://doi.org/10.1002/smj.v31:11.
- Kusiak, Andrew. 2020. “Convolutional and Generative Adversarial Neural Networks in Manufacturing.” International Journal of Production Research 58 (5): 1594–1604. https://doi.org/10.1080/00207543.2019.1662133.
- LeCun, Yann, Yoshua Bengio, and Geoffrey Hinton. 2015. “Deep Learning.” Nature 521 (7553): 436–444. https://doi.org/10.1038/nature14539.
- Lee, Chang-Ki, Yu-Jeong Cheon, and Wook-Yeon Hwang. 2021. “Studies on the GAN-based Anomaly Detection Methods for the Time Series Data.” IEEE Access 9:73201–73215. https://doi.org/10.1109/ACCESS.2021.3078553.
- Leo Kumar, S. P. 2019. “Knowledge-based Expert System in Manufacturing Planning: State-of-the-art Review.” International Journal of Production Research 57 (15-16): 4766–4790. https://doi.org/10.1080/00207543.2018.1424372.
- Lex Fridman Podcast. 2022. “Andrej Karpathy: Tesla AI, Self-Driving, Optimus, Aliens, and AGI.” Accessed July 23, 2023. hhttps://www.youtube.com/watch?v=cdiD-9MMpb0.
- Li, Jian, Linyuan Jin, Zhiyuan Wang, Qinghai Peng, Yueai Wang, Jia Luo, and Jiawei Zhou. 2023. “Towards Precision Medicine Based on a Continuous Deep Learning Optimization and Ensemble Approach.” NPJ Digital Medicine 6 (1): 18. https://doi.org/10.1038/s41746-023-00759-1.
- Li, Meng, and Tao Li. 2022. “AI Automation and Retailer Regret in Supply Chains.” Production and Operations Management 31 (1): 83–97. https://doi.org/10.1111/poms.13498.
- Liu, Changchun, Haihua Zhu, Dunbing Tang, Qingwei Nie, Shipei Li, Yi Zhang, and Xuan Liu. 2022. “A Transfer Learning CNN-LSTM Network-based Production Progress Prediction Approach in IIoT-enabled Manufacturing.” International Journal of Production Research 61:4045–4068. https://doi.org/10.1080/00207543.2022.2056860.
- Luo, Dan, Simon Thevenin, and Alexandre Dolgui. 2022. “A State-of-the-art on Production Planning in Industry 4.0.” International Journal of Production Research 61:6602–6632. https://doi.org/10.1080/00207543.2022.2122622.
- Makadok, Richard. 2001. “Toward a Synthesis of the Resource-based and Dynamic-capability Views of Rent Creation.” Strategic Management Journal 22 (5): 387–401. https://doi.org/10.1002/smj.v22:5.
- McCarthy, John. 1959. “Programs with Common Sense.” In Proceedings of the Teddington Conference on the Mechanization of Thought Processes, 75–91. London: Her Majesty's Stationary Office.
- McDonnell, P, S Joshi, and R. G. Qiu. 2005. “A Learning Approach to Enhancing Machine Reconfiguration Decision-making Games in a Heterarchical Manufacturing Environment.” International Journal of Production Research 43 (20): 4321–4334. https://doi.org/10.1080/00207540500142431.
- McKinsey. 2023. “What is AI?” Accessed July 23, 2023. https://www.mckinsey.com/featured-insights/mckinsey-explainers/what-is-ai.
- Minsky, M. 1968. Semantic Information Processing. Cambridge, MA: MIT Press.
- Mithas, Sunil, Zhi-Long Chen, Terence J. V. Saldanha, and Alysson De Oliveira Silveira. 2022. “How Will Artificial Intelligence and Industry 4.0 Emerging Technologies Transform Operations Management?.” Production and Operations Management 31 (12): 4475–4487. https://doi.org/10.1111/poms.13864.
- Mitra, Rony, Priyam Saha, and Manoj Kumar Tiwari. 2023. “Sales Forecasting of a Food and Beverage Company Using Deep Clustering Frameworks.” International Journal of Production Research 1–13.
- Nayal, Kirti, Rakesh Raut, Pragati Priyadarshinee, Balkrishna Eknath Narkhede, Yigit Kazancoglu, and Vaibhav Narwane. 2022. “Exploring the Role of Artificial Intelligence in Managing Agricultural Supply Chain Risk to Counter the Impacts of the COVID-19 Pandemic.” The International Journal of Logistics Management 33 (3): 744–772. https://doi.org/10.1108/IJLM-12-2020-0493.
- Ng, Andrew. 2023. ““AI For Everyone. DeepLearning.AI.” Accessed July 23, 2023. https://www.coursera.org/learn/ai-for-everyone.
- Noy, Shakked, and Whitney Zhang. 2023. “Experimental Evidence on the Productivity Effects of Generative Artificial Intelligence.” Science (New York, N.Y.) 381 (6654): 187–192. https://doi.org/10.1126/science.adh2586.
- OECD. 2019. “Recommendation of the Council on Artificial Intelligence.” Accessed July 23, 2023. https://legalinstruments.oecd.org/en/instruments/OECD-LEGAL-0449.
- Olsen, Tava Lennon, and Brian Tomlin. 2020. “Industry 4.0: Opportunities and Challenges for Operations Management.” Manufacturing & Service Operations Management 22 (1): 113–122. https://doi.org/10.1287/msom.2019.0796.
- OpenAI. 2017. “Generative Models.” Accessed July 23, 2023. https://openai.com/research/generative-models.
- OpenAI. 2023. “DALL·E 2 is An AI System that Can Create Realistic Images and Art From a Description in Natural Language.” Accessed July 23, 2023. https://openai.com/dall-e-2.
- OpenAI. 2023a. ““ChatGPT – Release Notes.” Accessed July 23, 2023. https://help.openai.com/en/articles/6825453-chatgpt-release-notes.
- OpenAI. 2023c. “GPT-4 Technical Report.”
- Oroojlooyjadid, Afshin, MohammadReza Nazari, Lawrence V. Snyder, and Martin Takáč. 2022. “A Deep Q-network for the Beer Game: Deep Reinforcement Learning for Inventory Optimization.” Manufacturing & Service Operations Management 24 (1): 285–304. https://doi.org/10.1287/msom.2020.0939.
- Otter, Daniel W., Julian R. Medina, and Jugal K. Kalita. 2020. “A Survey of the Usages of Deep Learning for Natural Language Processing.” IEEE Transactions on Neural Networks and Learning Systems 32 (2): 604–624. https://doi.org/10.1109/TNNLS.5962385.
- Panagou, Sotirios, W. Patrick Neumann, and Fabio Fruggiero. 2023. “A Scoping Review of Human Robot Interaction Research Towards Industry 5.0 Human-centric Workplaces.” International Journal of Production Research 1–17.
- Perano, Mirko, Antonello Cammarano, Vincenzo Varriale, Claudio Del Regno, Francesca Michelino, and Mauro Caputo. 2023. “Embracing Supply Chain Digitalization and Unphysicalization to Enhance Supply Chain Performance: a Conceptual Framework.” International Journal of Physical Distribution & Logistics Management.
- Petersen, Philipp, and Felix Voigtlaender. 2020. “Equivalence of Approximation by Convolutional Neural Networks and Fully-connected Networks.” Proceedings of the American Mathematical Society 148 (4): 1567–1581. https://doi.org/10.1090/proc/2020-148-04.
- Priore, Paolo, Borja Ponte, Rafael Rosillo, and David de la Fuente. 2019. “Applying Machine Learning to the Dynamic Selection of Replenishment Policies in Fast-changing Supply Chain Environments.” International Journal of Production Research 57 (11): 3663–3677. https://doi.org/10.1080/00207543.2018.1552369.
- Puche, Julio, Borja Ponte, José Costas, Raúl Pino, and David De la Fuente. 2016. “Systemic Approach to Supply Chain Management Through the Viable System Model and the Theory of Constraints.” Production Planning & Control 27 (5): 421–430. https://doi.org/10.1080/09537287.2015.1132349.
- Purwins, Hendrik, Bo Li, Tuomas Virtanen, Jan Schlüter, Shuo-Yiin Chang, and Tara Sainath. 2019. “Deep Learning for Audio Signal Processing.” IEEE Journal of Selected Topics in Signal Processing 13 (2): 206–219. https://doi.org/10.1109/JSTSP.4200690.
- Rai, Rahul, Manoj Kumar Tiwari, Dmitry Ivanov, and Alexandre Dolgui. 2021. “Machine Learning in Manufacturing and Industry 4.0 Applications.” International Journal of Production Research 59 (16): 4773–4778. https://doi.org/10.1080/00207543.2021.1956675.
- Richey Jr, Robert Glenn, Soumyadeb Chowdhury, Beth Davis-Sramek, Mihalis Giannakis, and Yogesh K. Dwivedi. 2023. “Artificial Intelligence in Logistics and Supply Chain Management: A Primer and Roadmap for Research.” Journal of Business Logistics 44 (4): 532–549. https://doi.org/10.1111/jbl.v44.4.
- Rocca, Joseph. 2023. “Understanding Variational Autoencoders (VAEs).” Accessed September 9, 2023. https://towardsdatascience.com/understanding-variational-autoencoders-vaes-f70510919f73.
- Rolf, Benjamin, Ilya Jackson, Marcel Müller, Sebastian Lang, Tobias Reggelin, and Dmitry Ivanov. 2023. “A Review on Reinforcement Learning Algorithms and Applications in Supply Chain Management.” International Journal of Production Research 61 (20): 7151–7179. https://doi.org/10.1080/00207543.2022.2140221.
- Rush, Alexander. 2018. “The Annotated Transformer.” In Proceedings of Workshop for NLP Open Source Software (NLP-OSS), 52–60. Melbourne, Australia: Association for Computational Linguistics.
- Samoili, Sofia, Montserrat Lopez Cobo, Emilia Gómez, Giuditta De Prato, Fernando Martínez-Plumed, and Blagoj Delipetrev. 2020. “AI Watch. Defining Artificial Intelligence. Towards an operational definition and taxonomy of artificial intelligence.”
- Samuel, A. L. 1959. “Some Studies in Machine Learning Using the Game of Checkers.” IBM Journal of Research and Development 3 (3): 210–229. https://doi.org/10.1147/rd.33.0210.
- Schlegel, Alexander, Hendrik Sebastian Birkel, and Evi Hartmann. 2021. “Enabling Integrated Business Planning Through Big Data Analytics: a Case Study on Sales and Operations Planning.” International Journal of Physical Distribution & Logistics Management 51 (6): 607–633. https://doi.org/10.1108/IJPDLM-05-2019-0156.
- Schroeder, Roger G., Kimberly A. Bates, and Mikko A. Junttila. 2002. “A Resource-based View of Manufacturing Strategy and the Relationship to Manufacturing Performance.” Strategic Management Journal 23 (2): 105–117. https://doi.org/10.1002/smj.v23:2.
- Schwab, Klaus. 2017. The Fourth Industrial Revolution. New York: Currency.
- Shahin, Mohammad, F. Frank Chen, Ali Hosseinzadeh, Hamed Bouzary, and Awni Shahin. 2023. “Waste Reduction Via Image Classification Algorithms: Beyond the Human Eye with An AI-based Vision.” International Journal of Production Research 1–19. https://doi.org/10.1080/00207543.2023.2225652.
- Sheffi, Yossi. 2023. The Magic Conveyor Belt: Supply Chains, AI, and the Future of Work. Cambridge: MIT CTL Media.
- Teece, David J., Gary Pisano, and Amy Shuen. 1997. “Dynamic Capabilities and Strategic Management.” Strategic Management Journal 18 (7): 509–533. https://doi.org/10.1002/(ISSN)1097-0266.
- Tesla. 2023. “AI and Robotics.” Accessed July 23, 2023. https://www.tesla.com/AI.
- Torrey, Lisa, and Jude Shavlik. 2010. “Transfer Learning.” In Handbook of Research on Machine Learning Applications and Trends: Algorithms, Methods, and Techniques, 242–264. Hershey, Pennsylvania: IGI Global.
- Tremblet, David, Simon Thevenin, and Alexandre Dolgui. 2023. “Learning the Capacity Consumption of Lot-Sizing Models from Production Schedules.” Working Paper, Submitted to Operations Research.
- Tremblet, David, Simon Thevenin, and Alexandre Dolgui. 2023. “Makespan Estimation in a Flexible Job-shop Scheduling Environment Using Machine Learning.” International Journal of Production Research 1–17. https://doi.org/10.1080/00207543.2023.2245918.
- Tyasnurita, Raras, Ender Özcan, and Robert John. 2017. “Learning Heuristic Selection Using A Time Delay Neural Network for Open Vehicle Routing.” In 2017 IEEE Congress on Evolutionary Computation (CEC), 1474–1481. Donostia: IEEE.
- U.S. Department of Defense. 2018. “Summary of the 2018 Department of Defense Artificial Intelligence Strategy. Harnessing AI to Advance Our Security and Prosperity.” Accessed July 23, 2023. https://media.defense.gov/2019/Feb/12/2002088963/-1/-1/1/SUMMARY-OF-DOD-AI-STRATEGY.PDF.
- Vanhaelen, Quentin, Yen-Chu Lin, and Alex Zhavoronkov. 2020. “The Advent of Generative Chemistry.” ACS Medicinal Chemistry Letters 11 (8): 1496–1505. https://doi.org/10.1021/acsmedchemlett.0c00088.
- Van Hoek, Remko, Michael DeWitt, Mary Lacity, and Travis Johnson. 2022. “How Walmart Automated Supplier Negotiations.” Harvard Business Review.
- Vaswani, Ashish, Noam Shazeer, Niki Parmar, Jakob Uszkoreit, Llion Jones, Aidan N. Gomez, Łukasz Kaiser, and Illia Polosukhin. 2017. “Attention is All You Need.” In Advances in Neural Information Processing Systems. Cambridge, MA: The MIT Press.
- Wamba, Samuel Fosso, Maciel M. Queiroz, Charbel Jose Chiappetta Jabbour, and Chunming Victor Shi. 2023. “Are Both Generative AI and ChatGPT Game Changers for 21st-Century Operations and Supply Chain Excellence.” International Journal of Production Economics 265:109015. https://doi.org/10.1016/j.ijpe.2023.109015.
- Webster, Graham, Rogier Creemers, Paul Triolo, and Elsa Kania. 2017. “Full Translation: China's ‘new Generation Artificial Intelligence Development Plan’(2017).” New America 1.
- The White House. 2022. “The Impact of Artificial Intelligence on the Future of Workforces in the European Union and the United States of America.” Accessed July 23, 2023. https://www.whitehouse.gov/cea/written-materials/2022/12/05/the-impact-of-artificial-intelligence/.
- Wong, Lai-Wan, Garry Wei-Han Tan, Keng-Boon Ooi, Binshan Lin, and Yogesh K. Dwivedi. 2022. “Artificial Intelligence-driven Risk Management for Enhancing Supply Chain Agility: A Deep-learning-based Dual-stage PLS-SEM-ANN Analysis.” International Journal of Production Research 1–21. https://doi.org/10.1080/00207543.2022.2063089.
- World Economic Forum. 2017. “Impact of The Fourth Industrial Revolution on Supply Chains.” Accessed July 23, 2023. https://www3.weforum.org/docs/WEF_Impact_of_the_Fourth_Industrial_Revolution_on_Supply_Chains_.pdf.
- Wu, Jing, Zhaocheng Zhang, and Sean X. Zhou. 2022. “Credit Rating Prediction Through Supply Chains: A Machine Learning Approach.” Production and Operations Management 31 (4): 1613–1629. https://doi.org/10.1111/poms.13634.
- Xiao, Jian-hua, Bing-lian Liu, Yu-fang Huang, and Zhen Cheng. 2014. “An Adaptive Quantum Swarm Evolutionary Algorithm for Partner Selection in Virtual Enterprise.” International Journal of Production Research 52 (6): 1607–1621. https://doi.org/10.1080/00207543.2013.841329.
- Xu, Frank F., Uri Alon, Graham Neubig, and Vincent Josua Hellendoorn. 2022. “A Systematic Evaluation of Large Language Models of Code.” In Proceedings of the 6th ACM SIGPLAN International Symposium on Machine Programming, 1–10. New York: Association for Computing Machinery.
- Xu, Kelvin, Jimmy Ba, Ryan Kiros, Kyunghyun Cho, Aaron Courville, Ruslan Salakhudinov, Rich Zemel, and Yoshua Bengio. 2015. “Show, Attend and Tell: Neural Image Caption Generation with Visual Attention.” In International Conference on Machine Learning, 2048–2057. France: PMLR.
- Young, Liz. 2023. “The Wall Street Journal. Chatbots Are Stepping Toward Supply Chains.” Accessed July 23, 2023. https://www.wsj.com/articles/chatbots-are-stepping-toward-supply-chains-5661039a.
- Yu, Zhongzhi, Yonggan Fu, Sicheng Li, Chaojian Li, and Yingyan Lin. 2022. “Mia-former: Efficient and Robust Vision Transformers via Multi-Grained Input-adaptation.” In Proceedings of the AAAI Conference on Artificial Intelligence, Vol. 36, 8962–8970. Washington: Walter E. Washington Convention Center.
Appendices
Appendix 1.
Extracting AI Capabilities from its Definition
A.1. Perspective of AI researchers
McCarthy (Citation1959), one of the founding fathers of the field of AI, emphasised that Reasoning capabilities are key to AI. Namely, ‘A programme has common sense if it automatically deduces for itself a sufficiently wide class of immediate consequences of anything it is told and what it already knows.’. Another pioneer of AI, Minsky, 1969 supposed that AI should possess all the cognitive capabilities attributed to humans. Namely, ‘AI is the science of making machines do things that would require intelligence if done by men.’ (Minsky Citation1968). On the other hand, Samuel (Citation1959) provide a more instrumentalist definition of AI that highlights Learning as a key capability. Namely, ‘AI is the field of study that gives computers the ability to learn by using sampled data without being explicitly programmed.’. Other prominent researchers highlight adaptability as the most critical capability of AI systems. For example, Holland (Citation1975), a pioneer in evolutionary computations, proposed an idea of a pattern recognition device based on the artificial adaptive system. Another pioneer in evolutionary computations, Fogel (Citation1995) defined AI as ‘Any system that generates adaptive behaviour to meet goals in a range of environments can be said to be intelligent.’
Among modern AI researchers, the definition of AI also ranges from more specific and instrumental to more philosophical and abstract. For example, Yann LeCun, Yoshua Bengio, and Geoffrey Hinton highlight Learning as a key capability and define it as ‘a set of methods that allows a machine to be fed with raw data and to automatically discover the representations needed for detection or classification’ (LeCun, Bengio, and Hinton Citation2015). However, they also emphasise that in the future, ‘a major progress in AI will come about through systems that combine representation learning with complex reasoning’ (LeCun, Bengio, and Hinton Citation2015). Ng (Citation2023) understands AI as ‘a huge set of tools for making computers behave intelligently and in an automated fashion.’ Demis Hassabis views AI as ‘a process that converts unstructured information into useful and actionable knowledge.’ (Financial Times Citation2017). Andrej Karpathy, in his recent interview, highlighted Interaction as a critical capability by defining AI as ‘an automated human-like system that we can interact with in a digital or physical realm.’ (Lex Fridman Podcast Citation2022). Goertzel (Citation2016) defines intelligence as ‘the ability to detect patterns in the world and in the agent itself.’, which primarily focuses on the Learning capability since pattern recognition is a subset of machine learning (Bishop and Nasrabadi Citation2006). Shedding light on the economics of AI, Agrawal, Gans, and Goldfarb (Citation2019) highlight predictive capabilities as the core in the business and economics context. For example, one of the provided definitions states that ‘AI is a prediction technology, predictions are inputs to decision making, and economics provides a perfect framework for understanding the trade-offs underlying any decision.’ (Agrawal, Gans, and Goldfarb Citation2018). The team of AI researchers, including Ruslan Salakhutdinov and Yoshua Bengio pointed out that one of the most important challenges in AI is ‘to mimic the remarkable human ability to compress huge amounts of salient visual information into descriptive language.’. Besides, the authors highlight that the AI systems ‘must be powerful enough to solve the computer vision challenges of determining which objects are in an image, but they must also be capable of capturing and expressing their relationships in a natural language.’ (Xu et al. Citation2015), which emphasises Perception capability, especially visual and natural language processing.
A.2. Perspective of international organisations and national agencies
As the field of AI advances, different national agencies have formulated their own unique perspectives and interpretations of what constitutes AI. These various visions underscore the broad nature of AI and its distinct applications and capabilities. For example, the U.S. Department of Defense provides a multifaceted definition of AI by defining it as ‘the ability of machines to perform tasks that normally require human intelligence – for example, recognising patterns, learning from experience, drawing conclusions, making predictions, or taking action – whether digitally or as the smart software behind autonomous physical systems.’, which highlights such capabilities as Learning, Perception and Prediction (U.S. Department of Defense Citation2018). The European Commission understands AI as ‘systems that display intelligent behaviour by analysing their environment and taking action to achieve specific goals’ (European Commission Citation2018a). The definition is further detailed in European AI Strategy and the Flagship report on AI. The letter postulates that AI is ‘a generic term that refers to any machine or algorithm that is capable of observing its environment, learning, and based on the knowledge and experience gained, taking intelligent action or proposing decisions.’, which puts forward such capabilities as Learning, Perception, and Interaction (European Commission Citation2018b). Even though the Chinese AI National Strategy doesn't provide any definition but instead focuses on the technological, economic, and geopolitical implications, the documents mainly focus on such capabilities as Reasoning, Learning, Interaction, and Adaptability (Webster et al. Citation2017). The High-Level Expert Group (HLEG) on AI came up with the most detailed, verbose, and nuanced definition that encompasses such AI capabilities as Reasoning, Learning, Perception, Interaction, and Adaptation. According to HLEG, AI is ‘a software or hardware system that, given a complex goal, acts in the physical or digital dimension by perceiving their environment through data acquisition, interpreting the collected structured or unstructured data, reasoning on the knowledge, or processing the information, derived from this data and deciding the best actions to take to achieve the given goal. AI systems can either use symbolic rules or learn a numeric model, and they can also adapt their behaviour by analysing how the environment is affected by their previous actions.’ (Samoili et al. Citation2020).
International Organisations also have quite different viewpoints. For example, the Organisation for Economic Co-operation and Development (OECD) defines AI as ‘a machine-based system that can, for a given set of human-defined objectives, make predictions, recommendations, or decisions influencing real or virtual environments.’, which highlights predictive capabilities along with the Reasoning that is essential for decisions and recommendations (OECD Citation2019). World Economic Forum, on the other hand, defines AI very broadly and vaguely as ‘a collective term for machines that replicate the cognitive abilities of human beings.’ (World Economic Forum Citation2017).
A.3. Perspective of Industry
Industry and business definitions of AI can often be best understood by referencing leading technology companies and consulting firms. Given this paper's primary focus on Supply Chain and Operations Management (SCOM), the perspectives and visions of these industry leaders are particularly significant.
According to McKinsey (Citation2023), AI can be defined as ‘algorithms that are trained on data and can detect patterns and learn how to make predictions and recommendations by processing data and experiences, rather than by receiving explicit programming instruction. The algorithms also adapt in response to new data and experiences to improve their efficacy over time.’. This definition is very multifaced and points out such capabilities as Learning, Prediction, Reasoning (necessary for recommendations), Perception, and Adaptation. According to Accenture (Citation2019), AI is ‘a constellation of many different technologies working together to enable machines to sense, comprehend, act, and learn with human-like levels of intelligence.’, which emphasises such capabilities as Perception, Learning, and Interaction.
Amazon (Citation2023), in their definition of AI, especially highlights the Learning capability by defining AI as ‘the field of computer science dedicated to solving cognitive problems commonly associated with human intelligence, such as learning, problem-solving, and pattern recognition.’. Tesla (Citation2023), on the other hand, aims to achieve a general solution for fully self-driving vehicles and bi-pedal robotics and focuses on such technological advancements as ‘vision and planning, supported by efficient use of inference hardware.’, which pays special attention to Perception and Reasoning. Google (Citation2023), in its definition, also emphasises Perception and Reasoning as well as Learning by defining AI as ‘a set of technologies that enable computers to perform a variety of advanced functions, including the ability to see, understand and translate spoken and written language, analyse data, and make recommendations.
Appendix 2.
Algorithms behind GAI
GAI is a class of AI models that are capable of generating new content that resembles the data they were trained on. In essence, GAI models, including GANs, VAEs (OpenAI Citation2017), and Transformers (Vaswani et al. Citation2017), explained in the subsequent subsections, have made significant contributions to various areas of AI, enhancing existing capabilities and paving the way for new applications. The attention mechanism in Transformers, in particular, has led to breakthroughs in handling long-range dependencies in data, leading to more sophisticated generative capabilities.
A.4. Generative adversarial networks
The idea behind GANs is that there are two neural networks, a generator G, and a discriminator D, that are set up in a sort of competition. G takes random noise as input and generates a sample data output. Initially, this generated data won't resemble the desired output at all, but over time the generator learns to produce more accurate results. At the same time, D takes as input a data sample and outputs the probability of that sample coming from the real dataset (as opposed to the generator). The discriminator is also trained over time, improving its ability to tell the difference between real and fake data. The training process involves both networks trying to outsmart each other, hence the term ‘adversarial’. The generator tries to produce data that the discriminator can't distinguish from real data, and the discriminator tries to get better at telling the difference. Through this process, the generator learns to produce very realistic data (Goodfellow et al. Citation2014).
According to its inventors, Goodfellow et al. (Citation2014), the adversarial modelling framework can be explained more formally by considering both G and D as fully connected artificial neural networks (Petersen and Voigtlaender Citation2020). To learn the generator's distribution over data x, we define a prior on input noise variables
, then represent a mapping to data space as
, where G has parameters
and can be considered a differentiable function. Discriminator
outputs a single scalar.
represents the probability that x came from the data rather than
. D is trained to maximise the probability of assigning the correct label to both training examples and samples from G. G is simultaneously trained to minimise
(See Figure ). In other words, D and G play the following two-player minimax game with value function
:
(A1)
(A1)
Figure A1. Generative Adversarial Framework. Adapted from Gharakhanian (Citation2023).
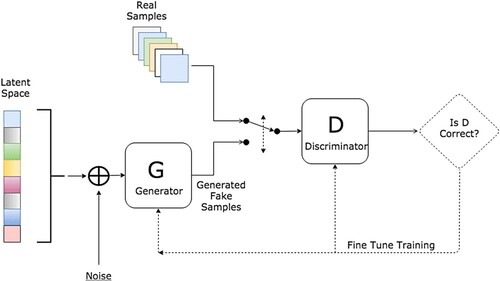
GANs offer a transformative approach to the core capabilities of AI in the context of SCOM. Firstly, GANs inherently demonstrate the Learning capability, as the generator refines its outputs based on feedback from the discriminator. This iterative feedback loop also showcases the Adaptation capability, as the generator continually adjusts to produce more realistic data. The Prediction capability is evident as GANs can generate new, synthetic data instances that can be used to predict or simulate various scenarios in SCOM. In turn, the adversarial nature of GANs is an instance of the Interaction capability.
A.5. Variational autoencoders
VAEs are an alternative approach to generative modelling based on the concept of a latent variable (Doersch Citation2016). VAEs have rapidly become one of the favoured methodologies for unsupervised learning involving complex distributions. The allure of VAEs is derived from their foundation on common function approximators, such as neural networks, and their compatibility with training through stochastic gradient descent (Doersch Citation2016).
The model assumes that there exists some hidden variable (z), which generates an observation (x). It is only possible to observe (x), but we would like to infer the characteristics of (z) by computing as:
(A2)
(A2) However, computing
is practically challenging and involves dealing with an intractable distribution:
(A3)
(A3) Therefore, the strategy behind VAEs is to approximate
by a tracktable distribution
. The distribution
is selected and parametrised such that it is similar to
, which is achieved by minimising Kullback–Leibler (KL) divergence (Kullback and Leibler Citation1951) as
, which is equivalent to maximising
] (Kingma and Welling Citation2019). Please, refer to Doersch (Citation2016) and Kingma and Welling (Citation2019) for complete derivation and more detailed explanations. In this setting,
can be used to infer the latent variables used to generate an observation. The idea could be implemented in a neural network architecture where the encoder model learns a mapping from x to z and the decoder learns the opposite mapping from z to x (See Figure ). The learning is performed through the minimisation of the following loss function:
(A4)
(A4) VAEs bring forth an approach that can significantly enhance the core capabilities of AI in the context of SCOM. The Learning capability is at the heart of VAEs, as they employ neural networks to learn complex distributions in an unsupervised fashion. This learning is not simply about reproducing data but also involves understanding the underlying latent variables, which is crucial to the Perception capability. The latent space representation in VAEs showcases the Adaptation capability, as it captures the essence of data and can be adjusted to generate variations of the original data.
Figure A2. Variational Autoencoder visualised. Adapted from Rocca (Citation2023).
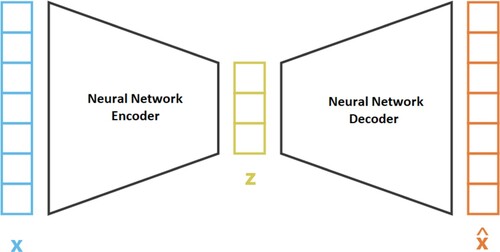
A.6. Large language models and transformers
From a formal standpoint, the learning objective is simplified to estimating the distribution from corpora, given a set of training examples , where each example represents a sequence of symbols with variable length
. Since both natural and programming languages are ordered sequentially, the joint probabilities over symbols (or tokens) can be factorised as the product of conditional probabilities:
(A5)
(A5) This assumption allows one to perform tractable sampling and estimation of
and other conditional probabilities of the form
(Bengio et al. Citation2003).
In the recent five years, there have been significant improvements in the performance of language models based on conditional probabilities of the form (Equation (EquationA5(A5)
(A5) )). The most notable advances can be attributed to self-attention architectures like the Transformer (Vaswani et al. Citation2017). Encoder-decoder structure constitutes a core behind the Transformer's architecture. The role of the encoder is to map an input sequence of symbol representations
to a sequence of representations
. The decoder produces an output
given
as an input. This process is autoregressive in the sense that the previously generated symbols are used as additional input (Graves Citation2013). Both encoder and decoder use stacked self-attention mechanisms as well as fully connected layers.
The encoder is composed of a series of N identical layers, each containing two sublayers. The first sublayer incorporates a multi-head self-attention mechanism, while the second sublayer features a position-wise, fully connected feed-forward network, reminiscent of multilayer perceptron architectures. To enable residual connections, all sub-layers and embedding layers produce outputs of the same dimension, . Similarly, the decoder is structured with a stack of N identical layers. A self-attention function is designed to map a query and its associated key-value pairs to an output, with the query, keys, values, and output all represented as vectors. The output is calculated as a weighted sum, where the weights corresponding to the respective values are determined using a feed-forward artificial neural network. The scaled dot-product attention serves as a crucial element within the self-attention mechanism. To enhance computational efficiency, the attention function is executed on a collection of queries simultaneously. Inputs consisting of queries and keys with dimension
, as well as values with dimension
, are consolidated into matrices Q, K, and V. This approach not only streamlines the process but also allows for more effective handling of complex language modelling tasks within the Transformer architecture.
(A6)
(A6) The Q, K, and V matrices are linearly projected h times to
, and
dimensions, respectively. After that, the self-attention function is performed on each of these projections, resulting in
-dimensional output vector. As a result, multi-head attention allows the model to access information from different representation subspaces at different positions.
(A7)
(A7) where
with parameter matrices
,
,
and
. Besides the sub-layers, each of the layers in both encoder and decoder contains a fully connected feed-forward network that can be represented as a composite function
.
is equipped with Rectified Linear Unit (ReLU) activation function and includes two linear transformations.
(A8)
(A8) The Transformer takes advantage of the learned embeddings to convert the input and output tokens to vectors of the dimension
, which is basically the embedding size. Besides, the regular learned linear transformation and
activation function convert the decoder output to estimated probabilities of the next token to appear in the sequence. Figure illustrates the matrix operations behind the Transformer architecture.
Figure A3. The matrix operations behind the Transformer. The illustration is adapted from Alammar (Citation2018) and Rush (Citation2018).
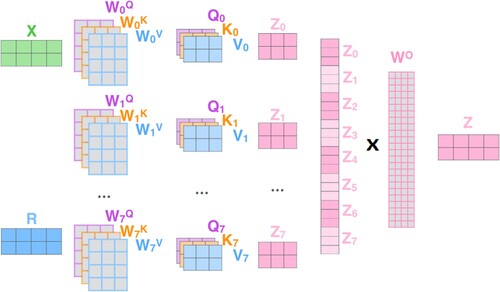
The self-attention mechanism, central for Large language models, emphasises the Perception capability, comprehending context and relationships within sequences. This mechanism, combined with the autoregressive nature of Transformers, amplifies the Prediction capability, as models predict subsequent tokens given prior context. The Transformer's multi-head attention, which processes different parts of an input concurrently, demonstrates Adaptation, allowing the model to adjust its focus dynamically. Lastly, Transformers have the potential to enhance the Reasoning capability when integrated with decision-making tools, enabling context-rich decisions in SCOM scenarios based on textual insights.