Abstract
Nowadays industrial robots have been increasingly widely deployed across various sectors and assembly tasks are seen as one of the dominant application fields. The assembly tasks, as the critical process in manufacturing, are still challenging for the robot because of the complex contact state between the robot and the environment (i.e. assembly components). In the assembly task, the robot should switch its controller from a non-contact mode to a contact-rich mode when the contact condition changes, where compliant behaviour is necessary for robustness to uncertain contact and safety of physical interaction. This paper proposes a deep reinforcement learning (DRL) method to achieve such compliance using variable stiffness compliant control. Concretely, a Cartesian compliant controller is built on a virtual dynamics model with a variable non-diagonal stiffness matrix to derive desired motion that reacts with the external force/torque. Upon that, a deep deterministic policy gradient (DDPG)-based agent is deployed to fine-tune such a non-diagonal stiffness matrix. After error-and-trial learning, robots can handle changes in contact state in assembly tasks without any pause and switching its controller mode, thus increasing task efficiency and compliance. The simulation and experiments show that our method allows robots to complete assembly tasks safely and efficiently under noisy observation.
SUSTAINABLE DEVELOPMENT GOALS:
Disclosure statement
No potential conflict of interest was reported by the author(s).
Data availability statement
Data presented in this study are available on request from the corresponding author.
Notes
Additional information
Funding
Notes on contributors
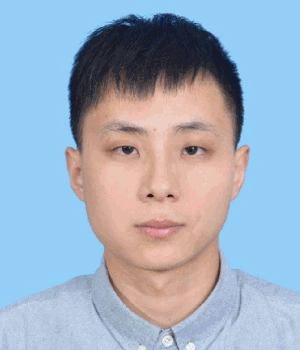
Zhenrui Ji
Zhenrui Ji received the B.S. degree in information engineering from Wuhan University of Technology, Wuhan, China, in 2018. Since 2020, he has been studying as a student with successive postgraduate and doctoral programs at Wuhan University of Technology. His research interests include robot learning and human–robot collaborative manufacturing.
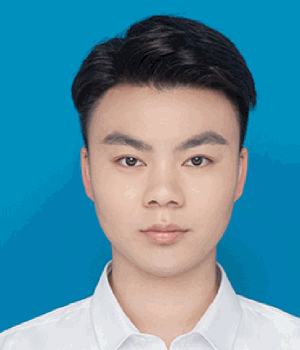
Gang Liu
Gang Liu received the M.E. degree in information and communication engineering from the Wuhan University of Technology, Wuhan, China, in 2023. His research interests include deep reinforcement learning and robotic compliance assembly.
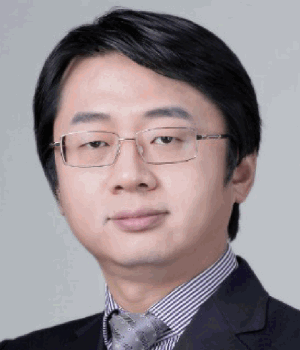
Wenjun Xu
Wenjun Xu received the B.E. degree in information engineering and the M.E. and Ph.D. degrees in communication and information system from the Wuhan University of Technology, Wuhan, China, in 2005, 2007, and 2010, respectively. He was a Visiting Researcher with Cardiff University, Cardiff, UK, from 2008 to 2010. He is currently a Professor and the Deputy Dean of the School of Information Engineering, Wuhan University of Technology. His research interests include smart collaborative manufacturing, digital twin, industrial artificial intelligence, human–robot collaboration, and industrial Internet. He received the Outstanding Young Manufacturing Engineer Award, Society of Manufacturing Engineers (SME), in 2018.
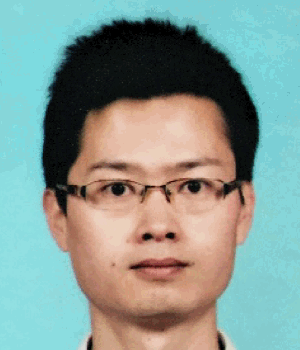
Bitao Yao
Bitao Yao received the Ph.D. degree in mechanical engineering from Wuhan University of Technology, Wuhan, China, in 2017. From 2015 to 2017, he was a guest Ph.D. student with the Department of Production Engineering, KTH Royal Institute of Technology, Stockholm, Sweden. He is currently an Associate Professor at the School of Mechanical and Electronic Engineering, Wuhan University of Technology, Wuhan, China. His research interests include human–robot collaboration and smart manufacturing.
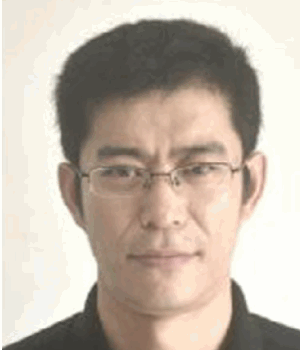
Xuedong Liu
Xuedong Liu received the Ph.D. degree in control science and engineering from the Institute of Image Recognition & Artificial Intelligence, Huazhong University of Science and Technology, Wuhan, China, in 2009. Since 2003, he has been working at the School of Information Engineering, Wuhan University of Technology, Wuhan, China, where he is now an Associate Professor. His main research interests are machine learning, digital signal processing, and video compression.
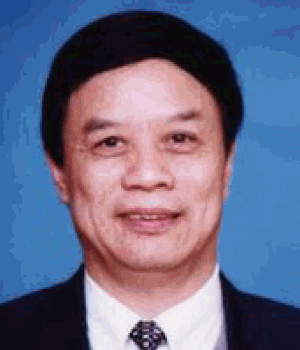
Zude Zhou
Zude Zhou is currently the Chief Professor with the School of Mechanical and Electronic Engineering, Wuhan University of Technology, Wuhan, China. His research interests include digital manufacturing, advanced manufacturing technology, digital monitoring and fault diagnosis, numerical control systems, networked control systems, etc.