Abstract
Anomaly detection is a frequently discussed topic in manufacturing. However, the issues of anomaly detection are typically attributed to the manufacturing process or equipment itself. In practice, the sensor responsible for collecting data and monitoring values may fail, leading to a biased detection result – false alarm. In such cases, replacing the sensor is necessary instead of performing equipment maintenance. This study proposes an effective framework embedded with autoencoder-based control limits that can dynamically distinguish sensor anomaly from process anomaly in real-time. We conduct a simulation numerical study and an empirical study of semiconductor assembling manufacturers to validate the proposed framework. The results show that the proposed model outperforms other benchmark methods and can successfully identify sensor failures, even under conditions of (1) large variations in process values or sensor values and (2) heteroscedasticity effect. This is particularly beneficial in various practical applications where sensors are used for numerical measurements and support equipment maintenance.
Disclosure statement
No potential conflict of interest was reported by the author(s).
Data availability statement
The authors confirm that the data supporting the findings of this study are available within the article [and/or] its supplementary materials.
Notes
1 Note that, for different equipment types, equipment nature and collected sensor data show diverse alarm patterns, and thus, the definitions of alarms could be diverse. Based on our definitions of anomalies, particularly for some rotating equipment or machine tools, the effect of machine/part degradation (e.g. bearing wear) is attributed to process anomaly. For example, the vibration signals of bearing wear process show an increasing variation over time (Lee et al. Citation2019).
Additional information
Funding
Notes on contributors
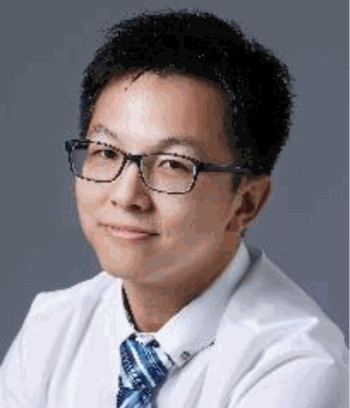
Chia-Yen Lee
Chia-Yen Lee received a PhD degree from the Department of Industrial and Systems Engineering, Texas A&M University, USA, in 2012. He is currently a professor at Dept. of Information Management, National Taiwan University, and the associate editor of IEEE Transactions on Semiconductor Manufacturing. He was the director at the Institute of Manufacturing Information and Systems, National Cheng Kung University (NCKU), Taiwan, and the associate editor of IEEE Transactions on Automation Science and Engineering, IEEE Transactions on Semiconductor Manufacturing. His research interests include manufacturing data science, intelligent manufacturing systems, productivity and efficiency analysis, and multi-criteria decision analysis. His research works appear in European Journal of Operational Research, IEEE Transactions on Engineering Management, Annals of Operations Research, IEEE Transactions on Automation Science and Engineering, Journal of Environmental Management, etc. Lee received the Best Practice Paper Award from the 17th Asia Pacific Industrial Engineering and Management Systems Conference (APIEMS2016), the 2016 Outstanding Young Industrial Engineer Award from Chinese Institute of Industrial Engineers (CIIE), 2017 Ta-You Wu Memorial Award of Distinguished Young Scholars from the Ministry of Science and Technology (MOST), Taiwan, the 2018 Kwoh-Ting Li Technology & Literature Lectureships Award of Distinguished Young Scholars form NCKU-Delta Electronics, and the 2019 Feng-Zhang Lu Memorial Medal from Chinese Management Association. He received the 2021 Outstanding Research Award, MOST, Taiwan, and the 2023 Industrial Engineering Award, CIIE.
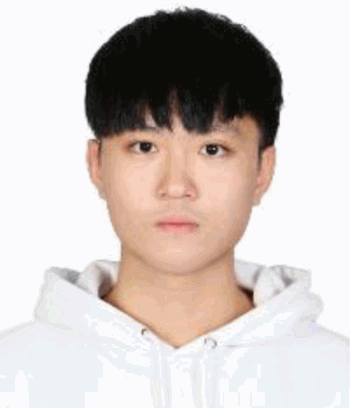
Kai Chang
Kai Chang received the M.B.A. degree from the Department of Information Management, National Taiwan University, Taipei, Taiwan, in 2022. His research interests include data science, machine learning, statistical process control and prognostics & health management.
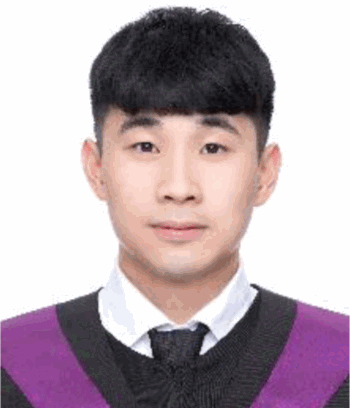
Chien Ho
Chien Ho received the B.A. degree from the Department of Economics, National Taiwan University, Taipei, Taiwan, in 2023. His research interests include data science, machine learning, and econometrics.