Abstract
The increasing vessel size and automation level have shifted the productivity bottleneck of automated container terminals from the terminal side to the yard side. Operating an automated container terminal (ACT) yard with a big number of automated guided vehicles (AGV) is challenging due to the complexity and dynamics of the system, severely affecting the operational efficiency and energy use efficiency. In this paper, a hybrid multi-AGV scheduling algorithm is proposed to minimise the energy consumption and the total makespan of AGVs in an ACT yard. This framework first models the AGV scheduling process as a Markov decision process (MDP). Furthermore, a novel scheduling algorithm called MDAS is proposed based on multi-agent deep deterministic policy gradient (MADDPG) to facilitate online real-time scheduling decision-making. Finally, simulation experiments show that the proposed method can effectively enhance the operational efficiency and energy use performance of AGVs in ACT yards of various scales by comparing with benchmarking methods.
Disclosure statement
No potential conflict of interest was reported by the author(s).
Data availability statement
The authors confirm that the data supporting the findings of this study are available within the article and its supplementary materials.
Additional information
Funding
Notes on contributors
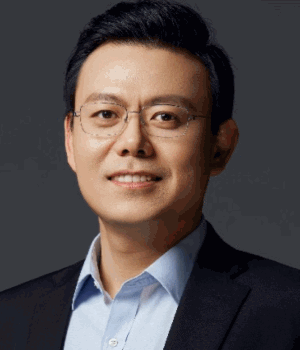
Lin Gong
Lin Gong received his B.S. degree and Ph.D. degree in Mechanical Engineering from Beijing Institute of Technology, Beijing, China. He was a visiting scholar with the University of Southern California, USA from 2013 to 2014. He is currently an Associate Professor and the Executive Deputy Dean of the School of Mechanical Engineering, Beijing Institute of Technology. His research interests include innovative design in complex systems, big data analysis, knowledge engineering, statistical optimisation, etc. He has published over 70 papers in domestic and international journals and conferences, where more than 30 papers were published on the journals or conferences indexed by SCI/EI. Lin Gong is also an expert committee member of Joint Innovation Center for Industrial Software and Industrial APP, a council member of Industrial Engineering and Management Research Society, Society of Management Science and Engineering of China, and a member of Industrial Engineer Qualification Expert Board of Chinese Mechanical Engineering Society, etc.
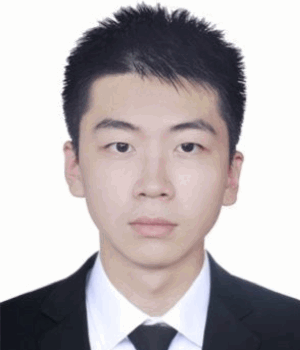
Zijie Huang
Zijie Huang received his B.Eng. degree in logistics engineering from University of Science and Technology Beijing. His main research interests are multi-agent systems and intelligent optimisation.
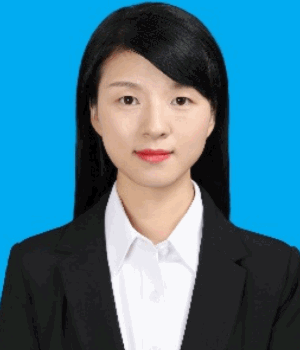
Xi Xiang
Xi Xiang received her Ph.D. degree in the Department of Industrial Engineering from Tsinghua University of China. She is currently an assistant professor in Beijing Institute of Technology. Her research interests include operations research on container terminal, theory and technology of operation optimisation or service system. Some of her research works are published in journals such as Omega, European Journal of Operational Research, and Transportation Research Part E.
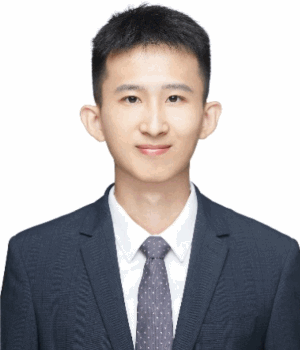
Xin Liu
Xin Liu received the B.Sc. degree in industrial engineering from the Beijing Institute of Technology, Beijing, China, in 2016 and Ph.D. degree in data science from the City University of Hong Kong. He is currently an Assistant Professor with the School of Mechanical Engineering, Beijing Institute of Technology, Beijing, China. His research focuses on machine learning, intelligent optimisation, and industrial systems.