Abstract
This study presents a systematic literature review to understand the applications, benefits, and challenges of digital assistants (DAs) in production and logistics tasks. Our conceptual framework covers three dimensions: information management, collaborative operations, and knowledge transfer. We evaluate human-DA collaborative tasks in the areas of product design, production, maintenance, quality management, and logistics. This allows us to expand upon different types of DAs, and reveal how they improve the speed and ease of production and logistic work, which was ignored in previous studies. Our results demonstrate that DAs improve the speed and ease of workers’ interaction with machines/information systems in searching, processing, and demonstrating. Extant studies describe DAs with different levels of autonomy in decision-making; however, most DAs perform tasks as instructed or with workers’ consent. Additionally, we observe that workers find it more intuitive to perform tasks and acquire knowledge when they receive multiple sensorial cues (e.g. auditory and visual cues). Consequently, future research can explore how DAs can be integrated with other technologies for robust multi-modal assistance such as eye tracking and augmented reality. This can provide customised DA support to workers with disabilities or conditions to facilitate more inclusive production and logistics.
1. Introduction
Researchers and practitioners have tried to design user-friendly human-computer interaction for decades. Finally, graphical user interfaces have been transformed into natural language user interfaces (Følstad and Brandtzæg Citation2017). Digital assistants (DAs), which are empowered by improved artificial intelligence (AI) systems, such as natural language processing, machine learning, and knowledge representation, can engage in conversations with humans, answer complex questions, perform tasks, provide recommendations, and make predictions (Oracle Citation2022). These are known as conversational agents, dialogue assistants, chatbots, intelligent personal assistants (IPA), virtual assistants (VA), softbots, or avatars (Maedche et al. Citation2019; Wellsandt, Hribernik, and Thoben Citation2021). Some examples are general aids such as Amazon’s Alexa, Apple’s Siri, and Google Assistant (Maedche et al. Citation2019), while Alibaba’s Xiaomi and JD’s JIMI are chatbots to improve customer service (Jiang, Qin, and Li Citation2022). In 2023, OpenAI released a large language model of a generative pre-trained transformer (GPT)-4 with the ChatGPT application (OpenAI), which demonstrates the potential (and limitations) in providing responses and solutions for a broad range of subjects, such as code generation, image generation, or text writing (Frederico Citation2023; OpenAI Citation2023a).
The production and logistics sectors are main drivers for employment and play critical roles in economic growth. The European Union for example employed more than 40.6 million people in its manufacturing, transportation, and storage sectors and generated a value addition of EUR 2509.3 billion (Eurostat Citation2022a; Citation2022b). However, production and logistics face challenges such as how to offer highly customised products and services, reduce production and delivery lead times and costs, and plan for uncertainty (Oracle Citation2021). DAs, in this regard, can be of great assistance. Juniper Research (Citation2023) predicted that chatbot use over two years could save around two and a half billion hours and eight billion dollars.
Owing to the digital transformation that inspired concepts such as Industry 4.0, human workers interact more frequently with smart materials, machines, equipment, and computers; process a higher volume of information; and manage more complex tasks (Kagermann, Wahlster, and Helbig Citation2013; Peruzzini, Grandi, and Pellicciari Citation2020; Romero et al. Citation2016). Enabling human workers to interact with cyber physical systems (CPS) through intelligent interfaces, such as DAs, is the key to supporting them in digitalised production and logistics environments, and to realise a human-system symbiosis in an Industry 5.0 context (Choi et al. Citation2022; Grosse et al. Citation2023). For example, DAs can enable human workers to chat with machines or information systems straightforwardly (Kassner et al. Citation2017), retrieve, process, and analyse shop floor information (Gärtler and Schmidt Citation2021), assist workers in completing tedious tasks, enable human-centric production control (Rabelo, Romero, and Zambiasi Citation2018), and coach workers to learn new tasks (Rooein et al. Citation2020).
Despite DAs being prevalent in our private and business lives, the literature has mainly focused on general applications of DAs in diverse industries, including banking, insurance, public transportation, retail, and customer relationship management (Agarwal, Agarwal, and Gupta Citation2022; Camilleri and Troise Citation2023; Quarteroni Citation2018; Silva et al. Citation2020). The aforementioned potentials of DAs in production and logistics have not yet been comprehensively discussed.
To contribute to closing this gap, this study aims to consolidate knowledge from the scientific literature and investigate how DAs can reduce human effort in production and logistics. They allow workers to be released from routine tasks, have additional control of the work process, and focus on creative and value-adding activities. We do this by performing a systematic literature review with the intention of (1) exploring and conceptualising the applications of DAs in supporting human workers in production and logistics tasks, (2) summarising the existing scientific evidence on the benefits and challenges of adopting DAs, and (3) proposing future research opportunities.
The remainder of this manuscript is structured as follows: Section 2 provides an overview of related literature reviews. Section 3 introduces the conceptual background of the study. Section 4 describes the research methodology used in the literature review, and Section 5 presents the literature review results in detail. Section 6 discusses the main findings of the literature review and syntheses regarding the application, benefits, and challenges of adopting DAs in production and logistics. Section 7 offers suggestions as to future research avenues, and Section 8 concludes the manuscript.
2. Overview of previous literature reviews
A few related literature reviews exist that discuss the use of different types of DAs in a production and/or logistics context. These related reviews are classified in Table , and they are briefly summarised in this section to highlight the novelty of our study.
Table 1. Summary of related literature reviews.
Von Wolff et al. (Citation2019) summarised applications of chatbots in digital workplaces and found that chatbots can be used to support information search tasks and standardise routine processes. Their review primarily focused on digital workplace design in the office instead of physical work (such as manual assembly or materials handling). Wellsandt, Hribernik, and Thoben (Citation2021) discussed the benefits of using DAs in production but did not discuss the potential of DAs in reducing human effort while performing tasks in detail. Pereira et al. (Citation2023) analysed physical and virtual assistance provided by VAs, their main services, and their limitations in an Industry 4.0 context. Rabelo, Zambiasi, and Romero (Citation2023) proposed the concept of softbots 4.0 and discussed their potential to support production with CPS. Colabianchi, Tedeschi, and Costantino (Citation2023) investigated manufacturing chatbots by clarifying their design elements and creating a taxonomy and development guidelines.
Table demonstrates that our present study differs from existing literature reviews by (a) applying a systematic literature search and selection methodology, (b) focusing on different types of DAs, (c) discussing DAs’ potential in reducing human effort in production and logistics tasks as well as the associated benefits and challenges, and (d) considering a larger literature sample than the earlier reviews.
3. Conceptual background
In this section we first introduce key concepts relevant to understanding how DAs can support production, and then logistics tasks (sub-sections 3.1 to 3.5). Furthermore, we combine these key elements in our conceptual framework (sub-section 3.6). This forms the basis of our discussion of the relevant literature in the subsequent sections.
3.1. Production and logistics areas
In this study, we consider four production and logistics areas, as stated in Pfohl (Citation2022 ) and Porter (Citation2011) : production (manufacturing), which transforms raw materials and semi-finished products into finished goods; maintenance, which involves functional checks, servicing, repairing, or replacing devices, equipment, and machines to ensure that all assets in industrial plants are in operational condition; quality management that ensures that the finished products meet the customers’ expectations; and logistics that handle demand, storage and distribution of goods. We consider these areas because they are value-creating processes of a typical manufacturing company characterised by the heavy reliance on the interactions between humans and technology (Neumann et al. Citation2021). In addition, we consider product design, including the design, testing, and possible redesign of product versions, because it is extremely interconnected with production. It ensures the manufactured products meet or exceed customer expectations in terms of quality and functionality (Borgianni et al. Citation2018).
3.2. Human-centricity
Humans are critical, both physically and cognitively, in every production and logistics area. They are endowed with domain knowledge, physical movement capacities, and work experience. In human-system interactions, the total system performance is determined by the symbiosis of the technical and social systems (Trist Citation1981). Owing to the ongoing digitalisation in production and logistics, human workers interact more often with the virtual world through advanced communication technologies and operate in complex and technology-driven systems. This leads to new challenges for them (Longo, Nicoletti, and Padovano Citation2017). In this context, terms such as ‘Operator 4.0’, ‘Human-in-the-loop’, and ‘Logistics Operator 4.0’ have been proposed, with the vision of supporting the physical and cognitive work of operators by new technological means (Cimini et al. Citation2020; Romero et al. Citation2016; Turner et al. Citation2021). Additionally, human-centricity, as one of the three pillars of Industry 5.0, highlights the central role of humans in the design of production and logistics systems (Grosse et al. Citation2023; Ivanov Citation2023). In this study, we consider the characteristics of workers from the perspectives of endowments and preferences based on the work of Baird and Maruping (Citation2021), who demonstrated that endowments occur when someone wants to free up resources for other pursuits or engage in activities that were previously unattainable to them. In addition, human workers may have preferences in production and logistics, such as learning how to perform assembly operations, monitoring and evaluating shopfloor issues, or simply retrieving query information from a machine or information system (Rabelo, Romero, and Zambiasi Citation2018).
3.3. Digital assistants
DAs are socio-technical systems that help humans perform tasks efficiently (Maedche et al. Citation2019). Early DAs could only have short conversations, and most of them did not easily learn from interactions (e.g. ELIZA, A.L.I.C.E.) (Wallace Citation2009; Weizenbaum Citation1966). With the advancement of AI, especially natural language processing and knowledge representation, DAs allow users to interact intuitively by using their natural language and provide assistance with daily activities (e.g. Apple’s Siri, Amazon’s Alexa, and IBM’s Watson) (Hoy Citation2018; IBM Citation2022). In production and logistics, DAs are included in the broader scope of Worker/Operator Assistance Systems, simplifying interactions between workers and complex machines and reinforcing workers’ physical and cognitive capabilities (Mark, Rauch, and Matt Citation2022; Roth, Moencks, and Bohné Citation2023). This study considers DAs that run on some information technology (laptop, touch panel, smartphone, smart speaker, etc.) and rely on a conversational, graphical, haptic, or multi-modal user interface. This is both for receiving input from and delivering output to users, to support them with information or knowledge to better perform tasks (Diederich et al. Citation2022; Maedche et al. Citation2019). We summarise the descriptions of various types of DAs based on the Operations Management and Information Systems literature in Table .
Table 2. Overview of different types of DAs.
3.4. Human-DA collaborative tasks
Depending on the characteristics of the DA, human characteristics, and related production and logistics areas, DAs can reduce the human effort involved in performing various tasks. Referring to the works of Adamopoulou and Moussiades (Citation2020), Maedche et al. (Citation2019), and Neumann et al. (Citation2021), we deductively categorise three macro-task types: information management (querying, retrieving, consulting, or analysing information), collaborative operations, (tasks in which DAs either instruct or assist human workers or assume the responsibility of completing parts of a joint task) and knowledge transfer (the involvement of DAs in reducing human effort for the acquisition of skills during real-life tasks). A more concrete classification of how DAs support humans while performing tasks across different production and logistics areas is discussed after an inductive refinement in the literature review (Section 5).
3.5. Benefits and challenges of using DAs
Inspired by the work of Pereira et al. (Citation2023) and Wellsandt, Hribernik, and Thoben (Citation2021), we summarise the benefits of using DAs in production and logistics from human and task perspectives. Given that DAs can guide humans in conducting tasks without memorising complex procedures, the cognitive workload of humans can be reduced. Workers can instruct DAs to perform certain tasks leaving them able to focus on abstract tasks instead, and they can also obtain assistance from them in developing skills and competencies. Given that DAs are capable of accessing multiple information systems and providing analytics for decision-making, their use can shorten task completion time and improve task efficiency. They can also support people ubiquitously, thereby increasing flexibility. A more concrete classification of benefits is discussed in Section 6.2.
Our review also aims to synthesise the challenges of using DAs in production and logistics from technological and implementational perspectives. They introduce complexities that can arise from intricate technical systems, for instance, accessing different user interfaces, interpreting structured, semi-structured, or unstructured data, or precise understanding of speech. Apart from technological challenges, companies may also face challenges that hinder the implementation of DAs, such as the integration of DAs into the existing systems as well as regulatory compliance. A more concrete classification of challenges is provided in Section 6.3.
3.6. Conceptual framework
We propose a framework summarising the conceptual background to guide the analysis of the literature sample (see Figure ). The framework consists of five main elements: (1) production and logistics areas, (2) human-centricity, (3) DAs, (4) human-DA collaborative tasks, and (5) benefits and challenges of adopting DAs. The framework is developed based on the socio-technical systems theory, which explains three key elements: humans that aim to achieve a specific goal; technology that is utilised by humans, in this case, the DAs; and tasks that are jointly accomplished by humans and technology to achieve the goal (Goodhue and Thompson Citation1995). The framework was inductively refined during the literature review (for better clarity, subsection numbers in Figure indicate where the respective part of the framework is discussed in further detail). Furthermore, the framework demonstrates DA-related benefits and challenges in production and logistics based on the results of the literature review (Section 6).
Figure 1. Conceptual framework of human-DA interaction in production and logistics.
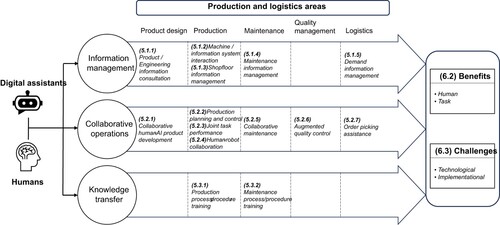
4. Systematic literature review methodology
To develop a literature sample for this research, we adopted a four-step approach: (I) identify groups of keywords, (II) search scholarly databases, (III) screen the literature, and (IV) complement the literature sample using a snowball search (Denyer and Tranfield Citation2009; Gough, Oliver, and Thomas Citation2017). First, we identified two groups of keywords based on related reviews (Section 2) and the conceptual framework (Section 3). Table lists the identified keywords. Group A keywords are related to DAs. Group B keywords are related to production and logistics, including product design, production, maintenance, quality management, and logistics.
Table 3. Groups of keywords used in the literature search.
Second, we searched for keywords in the Scopus and Web of Science databases, which are leading databases with comprehensive research coverage in the fields of science, technology, social sciences, arts, and humanities (Birkle et al. Citation2020; Burnham Citation2006). We used the Boolean operator ‘AND’ to combine the keywords in Group A with those in Group B and generated the final list of keywords for the search query. Studies published until the end of November 2023 and featuring at least one of the keywords in the article title, abstract, or list of keywords were retrieved for further analysis. This was with the proviso that the articles were written in English and published in peer-reviewed conference proceedings or scientific journals. The initial literature sample consisted of 7,337 papers, with 5,740 from Scopus and 1,597 from Web of Science. After eliminating duplicates, 5,913 papers were consolidated in the initial sample.
Third, we screened the titles, abstracts, keywords, and publication sources of the papers to select those that presented research on DAs and their applications in production and logistics. Studies that (1) did not examine a DA according to our definition or (2) did not focus on any of the production or logistics areas defined in Figure were excluded. In the screening process, all authors were involved to avoid selection bias, and any mismatches were discussed and reviewed by the authors. The screening process was recorded in an Excel spreadsheet and the selected papers were marked and categorised according to their application areas. At this stage, 172 papers were considered suitable for further analysis. Next, the screened papers were downloaded and exported to the Citavi Reference Management Software for full-text reading and annotation. Studies that did not satisfy the above-described selection criteria were excluded from further analysis. Consequently, the sample consisted of 66 papers.
Finally, a snowball search using the reference lists of the sampled papers or citations of the sampled papers was conducted to identify additional papers (Wohlin Citation2014). As a result, three more papers were identified, thus resulting in a total of 69 papers in the final literature sample. A summary of the literature selection process and results is shown in Figure .
Figure 2. Literature search and selection protocol.
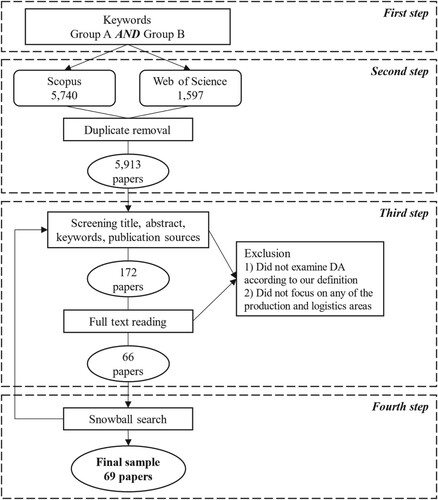
After selecting the final literature sample, we carried out descriptive analyses to show how research on DAs in production and logistics had developed over time, as well as the methods adopted in this stream of research (see Figure A1 and Table A1 in the Appendix).
5. Results
This section reviews the literature sample according to the framework developed in Section 3.6. We focus on highlighting the importance of DAs in reducing human effort while performing three types of tasks (information management, collaborative operations, and knowledge transfer) in production and logistics areas (product design, production, maintenance, quality management, and logistics). To structure the results, we also provide a more specific classification of tasks that inductively emerged during the analysis of the literature sample. An overview and categorisation of the 69 sampled papers are provided in Table A2 in the Appendix.
5.1. Information management
5.1.1. Product/Engineering information consultation [Product design]
Using DAs can accelerate collaborative engineering design and enhance customer experience. Choi, Hamanaka, and Matsui (Citation2017) developed a chatbot for a product manual. They demonstrated that users perceived the interaction with the chatbot as more helpful than paper-based instructions. In addition, because users can visualise product-function-related information via a chatbot interface, they can better understand the functions of the product. Trappey et al. (Citation2022) developed a natural language and virtual reality (VR)-enabled engineering consultation chatbot. Their proposed system presents product structures in a VR environment and enables customers to quickly ask questions and receive answers via chatbots. The authors tested the proposed system for an interactive design process and demonstrated that the chatbot could answer users’ questions with high accuracy (over 91%) irrespective of the kind of questions. However, the authors found that the developed chatbot sometimes failed to give correct answers owing to an incorrect judgement of the user’s intent or subjectivity of the query. Their study revealed that integrating chatbots with VR may help users obtain answers easily while viewing and reconfiguring a product’s structure in an immersive environment, thus facilitating vivid design reviews of the product.
5.1.2. Machine/information system interaction [Production]
Compared to various human-machine interfaces such as visual displays, physical interaction, and haptics, DAs help humans interact with machines more intuitively and naturally. For example, workers can communicate with machines by using one-to-one or public messages through text-based chatbots, where machines are interconnected and represented by their social network profiles via a digital twin (DT) (Kassner et al. Citation2017). Using avatars on the machine controller side may better assist humans in paying attention to unusual events and returning to normal situations. They can be developed with various facial and body characteristics for different information representation scenarios, such as maintenance notifications, warning and alarm messages. The question of whether they can increase safety and long-term user acceptance should be further investigated (Ziegeler and Zuehlke Citation2005).
In addition to interactions with machines, DAs also facilitate interactions with information systems and help workers monitor operational statuses. For example, Mantravadi, Jansson, and Møller (Citation2020) attempted to accommodate an AI-enabled chatbot to MES for information retrieval. They demonstrated that using an AI-based chatbot for manufacturing order management is quicker than a regular database lookup. Chatbots can also be connected to robot process automation (RPA) to enable users to obtain manufacturing-related information, generate queries, and schedule tasks anytime and anywhere (Do and Jeong Citation2022). Hüsson, Holland, and Sánchez (Citation2020) demonstrated that using an IPA to explain graphic and tabular information was less effective for long text speech input and information searches from an enterprise resource planning (ERP) system; therefore, IPA was recognised as an addition to traditional input methods (keyboard, mouse). Similarly, Gärtler and Schmidt (Citation2021) compared the effectiveness of a VA with GUI-based solutions (Excel, Power BI) for information extraction and demonstrated that, although the VA can help solve problems with different complexity levels, users are more comfortable in using the VA to solve simple problems and are reluctant to use the same to reach complex goals. To facilitate evaluating the benefits of DAs, Bousdekis et al. (Citation2022) proposed a framework for assessing AI-based DAs from the perspective of the trustworthiness of AI, the usability of DAs, the cognitive workload of human users, and overall business benefits for the company.
5.1.3. Shop floor information management [Production]
In shopfloor management, it is important to keep track of the equipment and item status. In this regard, DAs enable information retrieval, monitoring, and processing of information and facilitate shopfloor control. For example, Afanasev et al. (Citation2019) and Loh et al. (Citation2023) demonstrated the potential of using a dialogue assistant to automate the access and retrieval of production data, and in particular, constantly monitor equipment parameters and notify workers when an anomaly occurs. Reis et al. (Citation2022) used a VA to retrieve information from a database and alert workers based on predefined rules. Their results demonstrated that workers were comfortable using the VA for monitoring production and work shift performance and that the VA was reliable in recognising user requirements even though users had to speak a foreign language during the test. Jwo, Lin, and Lee (Citation2021) designed an interactive shop floor information dashboard and embedded a VA into a mobile API to help workers communicate and control the dashboard through vocal commands. Their results demonstrated that the VA is more flexible and easier to learn than a traditional user interface where the keyboard and mouse are used for dashboard monitoring and control. In addition, it can actively contact the related staff when the real-time data and historical data stored in the dashboard cannot answer the users’ questions. Penica et al. (Citation2023) combined a chatbot with smart glasses to allow workers hands-free access to real-time data and instructions. Their test results revealed that the chatbot can facilitate data exchange among machines and that it has a good level of dialogue interpretation.
Additionally, softbots can be used to analyse operational performance and help managers in decision-making. A softbot developed by Abner et al. (Citation2020), for example, supports three types of behavioural modes during communication with end users (reactive, planned, and proactive) and four types of business analytics (description, diagnostics, prediction, and prescription). The authors tested the system in reactive mode in real-time and demonstrated that it can work as an additional module of an MES to analyse shop floor information, identify current operational excellence maturity models, and help managers in decision-making. Furthermore, the authors argued that training is essential for managers to understand the terminology used by the softbot.
In addition to interacting with machines or information systems, DAs can be used to interact with item-level information for product tracking and tracing in a factory. For example, using Amazon Echo 5 as a voice-based DA can enable workers to interact with the DT of the product, helping them to keep track of a product’s status and identify its location. However, the use of this type of assistant poses challenges related to low transcription accuracy of speech and factory noise (Wellsandt, Foosherian, and Thoben Citation2020).
5.1.4. Maintenance information management [Maintenance]
Lean maintenance requires the workforce to focus their attention on their primary task, rather than maintenance data documentation. Compared with paper-based maintenance report generation, using a DA can streamline the process, shorten maintenance task completion time, and generate a higher-quality report. However, technicians may experience increased time pressure when interacting with the conversational agent because they are multitasking and have to maintain the conversation flow (Kernan Freire et al. Citation2022).
5.1.5. Demand information management [Logistics]
In logistics, understanding the market demand is important for companies to plan their operational strategies for market fulfilment. In this respect, DAs enable companies to connect better with customers and improve their logistics services. Hsiao and Chang (Citation2019) found that embedding a DA into the existing information systems for queries and evaluating customer interaction may add value to logistics services. Companies need to improve their capabilities for organisational, scientific and technological innovation as well as logistics services to successfully adopt DAs. Murciego et al. (Citation2020) proposed an order-processing system targeting older people living in rural areas. They employed an IPA to collect customer demand information and used the collected data to optimise delivery routing. The results demonstrate that the use of an IPA provides new channels for older people to access demand ordering services and that the logistics company can utilise the order and customer location information to optimise the routings and minimise delivery costs. Angelov and Lazarova (Citation2019) presented a distributed chatbot system for order queries in supply chains. The system comprises several services, including a chatbot, natural language processing, and supply chain services, and it can analyse the user’s query, and provide information about queried orders and supplies. Kern et al. (Citation2006) described an approach in which softbots can provide information to supply chain managers anytime and anywhere. Their approach was enabled by a decentralised overlay network, thus allowing the assistants to dynamically communicate with each other in the network, exchange information, and negotiate tasks.
5.2. Collaborative operations
5.2.1. Collaborative human-AI product development [Product design]
Moving beyond current scenarios in which humans generate design ideas within a team, AI-enabled DAs can facilitate human-DA collaborative design. In Makokha’s (Citation2022) work, the assistant observes the human activity and provides relevant suggestions via text or voice. The author trained language models, based on a publicly available database (Ubuntu) and transcripts of professional designers. Although the models did not perform as expected, the author found that using designer transcripts as a training set provided better conversation quality (Makokha Citation2022).
5.2.2. Production planning and control [Production]
CPS necessitate the intensive collaboration of workers with smart machines. DAs can assist in these tedious activities and human-centred production planning and control. Rabelo, Romero, and Zambiasi (Citation2018) demonstrated that workers could delegate automatic execution tasks, management of the shop floor process, notifications and summary reports to DAs. Rabelo et al. (Citation2019) then leveraged multiple softbots to enable symbiotic collaboration among workers, CPS, and information systems. Their proof-of-concept study demonstrated that softbots can collaborate to access the MES, query the ERP system regarding the scheduling of orders, confirm with workers, coordinate orders, trigger alarms, address errors, and adapt communication language automatically to the text input. Rabelo et al. (Citation2021) followed up with a softbot prototype which they found could help users interact with the workstation DT, actuate physical workstations, and proactively perform predefined tasks and communicate results to the user via chatting. Similarly, Li and Yang (Citation2021) developed a VA for sales order generation, material checks, production order generation, and shop floor equipment state monitoring, which reduced repetitive operations and improved productivity. Additionally, they highlighted that owing to ambient noise, the VA needs to filter the noise, which can be time-consuming and may lower efficiency.
Schwartz et al. (Citation2016) described a hybrid scenario in which a team composed of robots, virtual characters, and softbots work collaboratively. A worker here issues task commands via a dialogue engine to the blackboard accessed by robots that then execute the tasks. Softbots aggregate data produced by team members and update databases. The virtual character serves as a natural interface that communicates with the worker. Longo, Nicoletti, and Padovano (Citation2022) presented a knowledge-management platform that supports workers with multiple front-end applications (mobile and wearable devices, AR, and XR) and an AI-enabled DA. In this case, the DA serves as the main interaction tool for workers, which continuously screens the back-end knowledge platform and interacts with workers using a question-answer form, thus providing meaningful knowledge quickly and effectively.
5.2.3. Joint task performance [Production]
DAs can assist workers in performing tasks by providing instructions either directly or in combination with other technologies (e.g. object detection, machine vision). For example, Zimmer et al. (Citation2020) demonstrated the potential of chatbots to assist production ramp-up processes. They argued that the chatbot may here guide workers to perform better by familiarising them with the equipment and processes compared to relying solely on their own knowledge and equipment manuals. Li and Wang (Citation2021) developed an audio-visual humanoid VA that uses a camera and sensory system worn on the human forearm to detect workers’ assembly actions, a facial tracking system to detect the worker’s facial expression, and a natural language processing system to predict intention. Their proposed VA successfully communicated with co-workers to complete collaborative assembly tasks based on its own judgement of the current assembly situation. Recent studies by Chen et al. (Citation2021) and Chiu et al. (Citation2021) demonstrated the potential of using multi-modal chatbots that combine speech with object detection to classify user intentions to assist users with multi-step assembly tasks. Their test results demonstrate that multi-modal input can capture the user’s requirements better and thus provide more accurate assistance to the user than speech information alone. Similarly, Talacio et al. (Citation2021) introduced machine vision techniques as part of their developed IPA to augment the capabilities of speech recognition with image recognition and guide workers in assembly execution. They compared the developed IPA with conventional printed manuals and found that their use helps decrease assembly time and increases worker comfort, particularly beneficial with complex and time-consuming procedures. Behrendt and Strohmeier (Citation2021) proposed a process modelling methodology that can efficiently and accurately synthesise DAs and workers in task information and progress.
5.2.4. Human-Robot collaboration [Production]
DAs can serve as a natural interface that communicates between workers and robots in the production environment. Li et al. (Citation2021), for example, built a VA that adopts generic conversational strategies. They revealed that the prediction accuracy of the VA depends on ambient noise, workers’ voice volume, sentence length, and physical distance between the worker and the VA. Using a GPT neural network as the backbone, Li, Zhang, et al. (Citation2022) and Li et al. (Citation2023) constructed a dialogue corpus that accurately supported task-related and small talk dialogue in assembly, internal logistics delivery, and robot positioning and relocation. Li, Hansen, et al. (Citation2022) used a VA that assisted workers in completing internal logistics tasks by communicating with them to obtain task-related information, including the object, recipient, and destination. The VA then identified and instructed a suitably skilled robot to perform the task. Although this study was successful, Li, Chrysostomou, and Yang (Citation2023) found the DA had some interpretation latency and inaccuracy that could lead to safety issues in multi-robot working scenarios (e.g. the recent deadly incident where an industrial robot failed to differentiate between a human and a boxFootnote1). Ye, You, and Du (Citation2023) fine-tuned ChatGPT into a worker’s robot assistant when controlling a robot arm, demonstrating that this increased the assembly task performance and enhanced the trust of humans. This was owing to its naturalness in communication and its capabilities in memorising previous decisions. However, the authors noted that in case of miscommunication, the robot assistant may become too assertive.
5.2.5. Collaborative maintenance [Maintenance]
In maintenance, DAs can assist workers in searching for maintenance-related information and provide instructions. In an early study, Nyrkko et al. (Citation2007) illustrated a dialogue system to support three types of dialogues: (1) metacommunication (greeting, goodbye), (2) providing information and feedback (inform, agree/disagree, partial information), (3) and asking questions (ask what, answer yes, or no). Ade et al. (Citation2020) later developed an AI-enabled chatbot for maintenance procedural consultations. Their study demonstrated that the chatbot could effectively help workers navigate the procedural steps linearly, return to the previous step, and repeat the step by identifying the context based on a previous conversation. They concluded that the chatbot could serve as a tool for safety and performance improvement, owing to its ability to navigate a large number of procedural steps and its effective engagement with the worker. Wellsandta et al. (Citation2020) developed concepts for using DAs to support manufacturing maintenance. They collected the requirements of maintenance stakeholders (i.e. maintenance coordinator, maintenance technician, and machine operator), and identified function modules of DAs (i.e. process monitoring, task execution, reporting, problem-solving, and maintenance planning). They emphasised that solving interoperability issues and convincing stakeholders to trust DAs remains a challenge for the implementation. Wellsandt et al. (Citation2021) proposed a novel approach to integrate human knowledge in the design of DAs for supporting predictive maintenance. They identified five stages of human intervention for predictive maintenance, including sense, detect, predict, decide, and act. They demonstrated that DAs can provide data required by the technician, configure the data visualisation based on the communication with the technician, recommend maintenance solutions, and offer instructions to guide inspection or repair. Wellsandt, Klein, et al. (Citation2022) argued that to integrate DAs successfully in the company, the benefits and limitations of their use should be clearly communicated to managers, the company-level data should be free from biases, errors, inaccuracies and mistakes, and also that the employees should be trained to adapt human-AI collaborative working scenarios. Aceta, Fernández, and Soroa (Citation2022) and Aceta et al. (Citation2022) developed a semantics-based task-oriented dialogue system to assist workers in maintenance tasks using natural language. Their usability test demonstrated that users could obtain accurate information from the system and complete a high share of the maintenance dialogue in a short time. Moreover, they perceived lower cognitive demands and higher security when interacting with the dialogue system.
DAs can be combined with other technologies to complete maintenance tasks efficiently. For instance, Abate et al. (Citation2008) demonstrated the possibility of using an avatar to guide workers towards collaborative maintenance. They proposed a framework consisting of a behavioural engine for retrieving human biometric data and creating a virtual model, a visualisation engine for managing all visual components, and an avatar for assisting workers in failure search and repair. They argued that the proposed avatar was more effective than a conventional screen-based interface because it provided the maximum possible level of adaptivity to users’ needs and assisted workers proactively. Zambiasi et al. (Citation2022) demonstrated that participants found merit in combining a DA with AR for task guidance, as it reduced their efforts so that they could concentrate on conducting tasks correctly. Serras et al. (Citation2020a) and Serras et al. (Citation2020b) augmented kinesthetic–bionic interactive technologies using data collected from device sensors to enhance domain knowledge creation. The results of their usability study demonstrated that novices found the system intuitive to use and that they felt more confident under multi-modal guidance. More importantly, the system was perceived as highly efficient for users with cognitive impairments because the cognitive workload is lower if such a system is used. Fleiner et al. (Citation2021) argued that conversational user interfaces are not sufficiently robust for maintenance guidance because of the ambient noise that interferes with voice recognition. Therefore, they proposed a set of user-defined gestural inputs (hand and head) as a complement to text- and voice-based communication. Their usability study revealed that gestures can be used as an alternative in noisy environments and that the participants preferred gestures for simple actions, whereas speech was more suitable for abstract actions.
5.2.6. Augmented quality control [Quality management]
DAs augment workers’ capabilities for quality control by simplifying the retrieval and processing of quality data. Bousdekis et al. (Citation2021) investigated a scenario in which quality data were collected and processed using prescriptive analytics such that defects and corresponding root causes could be quickly detected and mitigating actions could be defined. In this case, the DA provides descriptive, predictive, and prescriptive information to workers, thus enabling them to easily manage quality issues and make decisions based on the given analytics results. Wellsandt, Foosherian, et al. (Citation2022) summarised how DAs can augment workers by interacting with data analytics for quality testing. Users can retrieve existing information (eventually with customised parameters) and instruct the DA to add quality reports for further data learning, or the DA can proactively remind the user and provide relevant suggestions. The DA ensures that workers spend less time preparing, processing, and understanding data, and their cognitive capabilities are augmented.
5.2.7. Order picking assistance [Logistics]
DAs can provide remote instructions for order pickers. For example, Wang et al. (Citation2020) investigated the effect of avatars with different body representations (whole body, hand and arm, and hand only) on the quality of remote instructive order picking tasks in terms of efficiency, performance, workload, usability, and performance. The results illustrated that all three types of avatars transferred the instructions accurately; the avatar with a body representation outperformed the other types of avatars in terms of perceived workload by the participants and the time taken to respond to the instructions.
5.3. Knowledge transfer
5.3.1. Production process/procedure training [Production]
In a production environment, DAs assist workers in learning new tasks. Tanaka et al. (Citation2003) developed an assembly training system based on bidirectional verbal and nonverbal communication between humans and avatars. Verbal data from humans were collected and processed via a language processing system. Position sensors and data gloves were used to detect the pointing actions of the humans. Based on these data, the avatar can understand the user’s intention, recognise the object that the user is referring to, and give instructions on how a specific assembly operation should be performed. Kernan Freire et al. (Citation2023b) demonstrated the potential of using a DA to support transferring tacit knowledge from experienced workers to novices. They argued that using a DA can automatically prompt experienced workers to begin a reflection session by providing contextual information, generating visualising graphs and communicating in a natural way. Although usually, large language models-enabled DAs can interpret workers’ questions accurately, their use also induces ethical concerns that are related to privacy and knowledge security, as well as the validation of the knowledge bias collected by the DA (Kernan Freire, Foosherian, et al. Citation2023; Kernan Freire et al. Citation2023a).
Researchers have also focused on the design of avatars by integrating AR/VR for effective training. Pace et al. (Citation2019) compared the effectiveness of a human avatar and an abstract metaphor in assembly guidance, but could not identify a significant difference between the two interfaces in terms of the user’s perception of efficiency, learnability, or satisfaction. Moreover, a large humanoid avatar may cause difficulties for the participants in visualising the real objects owing to the narrow field-of-view of the AR wearable device, thereby increasing occlusion problems with overlapping virtual objects. Lampen, Liersch, and Lehwald (Citation2020) provided insights into the possibility of using an AR avatar for implicit imitation learning in manual assembly. Their results demonstrated that workers could learn ergonomic motions by imitating an avatar without decreasing the assembly performance. Furthermore, Brade et al. (Citation2020) evaluated the effect of different avatar representations (hands only, full body) on perceived presence and acceptance during manual assembly training in a VR environment. Their results illustrated that participants perceived a high ‘sense of physical space’ and ‘engagement’ during the task when an animated avatar appeared; however, there was no significant difference in the impact of different avatars on task performance. They concluded that the details of animated avatars could be reduced to focus on the body parts that are important in fulfilling the given tasks.
In addition to using avatars for training assistance, Rooein et al. (Citation2020) demonstrated how a chatbot can be used as a navigator for tailored learning for workers. Their chatbot especially filled the gap between novice workers’ knowledge of the factory’s business processes and the complex notions of business process descriptions. Casillo et al. (Citation2020) and Clarizia et al. (Citation2021) also developed a chatbot for novice training based on text information processing and elaboration of user needs. Their results exhibited improved learning paths, although the dialogue capabilities of the chatbot could be further improved. Longo, Nicoletti, and Padovano (Citation2017) introduced a DA to enable the interaction and assistance of workers by providing knowledge about components, machines, tasks, procedures, and processes via vocal exchange. They found that workers assisted by the DA had better task performance and that the system led to a stronger learning effect.
5.3.2. Maintenance process/procedure training [Maintenance]
DAs can transform multi-modal data into easily understandable information to support maintenance staff in learning complex tasks. In contrast to the existing AR-based mentoring systems, Zhu et al. (Citation2014) combined a VA with AR and pose-tracking to guide users during maintenance tasks using multi-modal cues. In the developed system, the VA processes visual, audio, and location data to interpret context information and provide precise technical assistance to the user. As a result, novices can perform complex maintenance tasks without any technical manuals or instructor support; simultaneously, the burden on the instructor is reduced as the novices’ task performance need not be monitored. Barbosa et al. (Citation2018) demonstrated that VAs and DT can be combined to automatically detect equipment errors, compare them with historical data, and provide maintenance suggestions to technical staff. When compared with traditional maintenance routines, their proposed method enables the online monitoring of equipment, facilitates the visualisation of equipment and its components, and makes it easier for maintenance staff to learn how to conduct maintenance by receiving instructions from the VA. Recently, Zambiasi et al. (Citation2023) demonstrated the potential of using a softbot as an intelligent tutor in training workers in an immersed industrial metaverse environment. In particular, their proposed system can adapt itself to support both normal workers and workers with hearing or colour-blind disabilities in three maintenance training scenarios (reactive, planning, proactive), leading to more inclusive workplaces.
6. Discussion
6.1. Different application maturity levels of DAs
The applications of DAs in the production and logistics literature are summarised in Figure , which demonstrates that production and maintenance are the most popular areas. The literature investigates the use of DAs in reducing human effort while performing different types of tasks in this area, summing up to 59 papers. Additionally, they are the only two areas in which the literature highlights how DAs can help workers gain knowledge and learn new skills.
Figure 3. Application of DAs across different production and logistics areas.
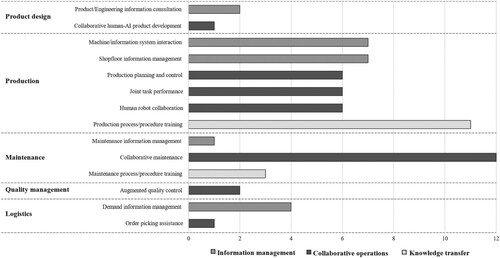
Furthermore, certain studies have focused on product design (3 papers, 4.3%), quality management (2 papers, 2.9%), or logistics (5 papers, 7.2%). With respect to product design, the literature indicates the substantial potential of DAs as an effective tool to build communication channels between designers and customers and help designers generate new ideas collaboratively. In terms of quality management, the literature has focused on using DAs to collect and analyse quality-related data and remind workers about quality issues. Regarding logistics, existing literature uses DAs to facilitate demand queries from the customer’s end to improve logistics service.
6.2. Benefits of using DAs
This study summarises the benefits of using DAs from two perspectives: humans and tasks. Regarding benefits for humans, some works demonstrated that DAs can reduce the cognitive workload of humans from different perspectives. First, DAs can streamline the shopfloor information by actively collecting data and thus decrease the information overload for shopfloor management (Abner et al. Citation2020; Aceta et al. Citation2022; Aceta, Fernández, and Soroa Citation2022; Kern et al. Citation2006; Longo, Nicoletti, and Padovano Citation2022). Second, when technicians are being assisted by DAs for collaborative maintenance, they do not need to memorise complex procedures but can feel more confident and perceive less mental workload in performing the tasks (Ade et al. Citation2020; Serras et al. Citation2020a; Zambiasi et al. Citation2022). Third, as humans differ in terms of skills and personality, DAs can provide customised assistance to enhance humans’ decision-making capabilities in production planning and control (see e.g. Abner et al. (Citation2020); Longo, Nicoletti, and Padovano (Citation2022)), quality control (Bousdekis et al. Citation2021; Wellsandt, Foosherian, et al. Citation2022), and demand management (Hsiao and Chang Citation2019; Kern et al. Citation2006). Moreover, using DAs can reduce the physical workload of humans. For instance, humans can delegate DAs in actuating physical workstations or robots to realise hands-free operation (see e.g. Li et al. (Citation2021); Rabelo et al. (Citation2021)). From a psychosocial point of view, our results indicate that owing to the easy-to-learn and -use character of DAs (see e.g. Murciego et al. (Citation2020); Trappey et al. (Citation2022)), as well as the human-like conversation generated by DAs (Ade et al. Citation2020; Jwo, Lin, and Lee Citation2021; Li, Zhang, et al. Citation2022), people enjoy using DAs and engage more in working on the actual tasks (see e.g. Talacio et al. (Citation2021); Gärtler and Schmidt (Citation2021)). In addition, as DAs are endowed with knowledge and a strong digital structure, humans can get assistance from them in retrieving missing information and be guided by them for unfamiliar tasks. Consequently, DAs are useful for training, particularly for novices (see e.g. Behrendt and Strohmeier (Citation2021); Rooein et al. (Citation2020)).
Regarding benefits for tasks, our results demonstrated that DAs are powerful in interacting with machines, robots, and information systems (e.g. ERP and MES), which facilitates access to information from multiple sources, decreases lead times for information search, and improves task flexibility and efficiency (see e.g. Jwo, Lin, and Lee (Citation2021); Kassner et al. (Citation2017); Li, Hansen, et al. [Citation2022]). The benefits of using DAs that emerged from our literature analysis are summarised in Table (note that ‘ID’ refers to the paper identification code as exhibited in the third column of Table A2 in the Appendix).
Table 4. Benefits of adopting DAs in production and logistics.
6.3. Challenges of using DAs
This study summarised the challenges of using DAs from both technological and implementational perspectives. Owing to the emerging nature of implementing DAs in production and logistics, some technical aspects of DAs are still not mature enough to guarantee smooth interactions between DAs and humans, such as the reliability of speech-to-text (STT) processing (Gärtler and Schmidt Citation2021; Wellsandt, Foosherian, and Thoben Citation2020) and accuracy of text modelling and handling of more complex interactions (Abner et al. Citation2020). In production and logistics, human workers often perform tasks subject to time pressure, and the response speed of DAs therefore needs to be sufficiently fast to maintain low latency during interactions (Barbosa et al. Citation2018; Bousdekis et al. Citation2021; Hsiao and Chang Citation2019). As a reliable STT is based on a large amount of audio data and its transcription, STT model training is inevitably time-consuming and there is a lack of adaptability when using DAs in different application scenarios. Moreover, although companies such as Google and Amazon provide STT solutions, the training data for these solutions are not sufficiently transparent, thus raising ethical concerns (Bousdekis et al. Citation2021; Wellsandta et al. Citation2020). Moreover, substantial effort is required to translate the analytical results into easy-to-understand information for human workers (Abner et al. Citation2020; Bousdekis et al. Citation2021).
Adoption of DAs in production and logistics also presents implementation-related challenges. Several researchers have pointed out that noise in industrial environments reduces the performance, particularly of speech-based DAs (Bousdekis et al. Citation2021; Ghofrani and Reichelt Citation2019; Li et al. Citation2021; Li and Yang Citation2021; Serras et al. Citation2020a; Citation2020b; Ziegeler and Zuehlke Citation2005). In addition, testing DA prototypes in real-world settings is essential to validate their effectiveness (Bousdekis et al. Citation2022; Kassner et al. Citation2017; Kern et al. Citation2006; Kernan Freire et al. Citation2022; Makokha Citation2022; Mantravadi, Jansson, and Møller Citation2020; Rabelo, Romero, and Zambiasi Citation2018; Rabelo, Zambiasi, and Romero Citation2019) as well as cost-performance issues, especially for small- and medium-sized companies (Wellsandt, Klein, et al. Citation2022; Wellsandta et al. Citation2020). Moreover, the adoption of DAs must consider data security requirements and their compatibility with legal frameworks, which include – but are not limited to – data ownership, data processing scope, and user consent management (Afanasev et al. Citation2019; Bousdekis et al. Citation2021; Kernan Freire et al. Citation2023a; Mantravadi, Jansson, and Møller Citation2020; Wellsandt, Foosherian, and Thoben Citation2020). The challenges of using DAs are summarised in Table (note that ‘ID’ refers to the paper identification code as exhibited in the third column of Table A2 in the Appendix).
Table 5. Challenges of adopting DAs in production and logistics.
7. Future research opportunities
7.1. Using augmented analytics to support human workers
We indicated that DAs can be embedded in management systems (e.g. ERP, MES) or other interfaces (e.g. API, dashboard) for information search and demonstration, which enables human workers to communicate with machines quickly and straightforwardly. However, our literature review identified only a few studies that describe the analytical potential of DAs and that mention the difficulties of translating analytical results into easy-to-understand information for humans (Abner et al. Citation2020; Bousdekis et al. Citation2021; Wellsandt, Foosherian, et al. Citation2022). We believe that DAs are more powerful when they are combined with other digital technologies, for instance, CPS, IoT, Cloud, RPA, or DT, such that real-time analytics regarding the status of materials or equipment can be constantly visualised and interpreted by humans. For example, empirical studies could investigate the usage of DAs to explain the real-time analytics and predictive analytics data on the shop floor, specifically concerning shop floor workers’ understanding of the data and resulting consequences.
Proposition 1. Future studies should explore the synergetic use of DAs, CPS, IoT, and DT in providing transparent, understandable, and predictive analytics and validate their benefits in the real-world.
Proposition 2. Future studies should identify how DAs can augment the decision-making capabilities of different target groups by providing customised analytics, as well as identifying the most suitable DA approach for specific production and logistics tasks (i.e. investigating the best possible DA-Task-Fit).
Proposition 3. To augment workers effectively, worker-related characteristics, such as learning or individual experience should be considered in the design and implementation of DAs.
7.2. Hybrid decision-making and teamwork in production and logistics
Our results reveal that DAs have different levels of automation for supporting decision-making, and in most cases, they perform actions as instructed by workers or generate recommended options to implement a task with the consent of workers. Only a few studies have shown the full autonomy of DAs in decision-making. This finding aligns with that of Endsley (Citation2017), who argued that the autonomy level depends on system reliability. As DAs in production and logistics still face challenges related to understanding worker intentions, they require human intervention and are not completely autonomous. Given that humans and DAs have individual rights and responsibilities in completing joint tasks, and task performance is a cooperative effort between human workers and the surrounding system (Abate et al. Citation2008), additional research is needed to clarify the distribution of tasks between workers and DAs.
Proposition 4. Future research should identify suitable thresholds of automation levels of DAs in the decision-making process and their association with task characteristics.
Proposition 5. Hybrid human-DAs teamwork should be evaluated considering workers’ intentions, trust levels, comfort, satisfaction, and cognitive workload to achieve human-DA symbiosis in production and logistics tasks. Furthermore, a long-term evaluation is necessary.
7.3. Multi-modal worker assistance
Our results indicated that DAs can be combined with tracking technologies (e.g. motion tracking, object tracking, facial tracking, and eye tracking) to predict workers’ intentions beyond performing a task and providing assistance (Chen et al. Citation2021; Talacio et al. Citation2021). However, the use of DAs and tracking technologies to assist workers is still in its initial phase and is only applied to a few tasks, such as assembly and maintenance guidance. Given that the quality of communication between workers and DAs is often influenced by ambient noise, multi-modal inputs enable more robust assistance in task completion.
Proposition 6. Future research needs to explore further areas where multi-modal assistance can be applied, such as production ramp-ups, material handling, and order picking.
Proposition 7. Future research should investigate how to generate domain knowledge to refine the usage scenarios of DAs, discover to what extent workers can rely on the input given by DAs, when human expert intervention is necessary, and ascertain who is responsible for the losses due to task operational errors.
7.4. DAs as facilitators for an inclusive workforce in production and logistics
Many countries are facing demographic changes in terms of age, gender, and cultural background combined with workforce shortages. Our results demonstrated that DAs can provide task assistance, automate information processing, and potentially interpret and communicate in different languages (Reis et al. Citation2022). It is therefore essential to explore the potential of DAs in supporting older workers and reconciling cultural background issues. Moreover, labour market participation remains a challenge for people with disabilities (Eurofound Citation2021) who often face difficulties in securing jobs in production and logistics (Mark et al. Citation2019). An inspiring example of using a DA to support people with disabilities is ‘Be My Eyes’. Here ChatGPT-4 acts as the ‘eyes’ of visually impaired people transforming images into text, so that real-world information can be obtained immediately (OpenAI Citation2023b). We encourage future research to develop concepts and use case scenarios for the inclusion of people with disabilities in production and logistics.
Proposition 8. Future studies should explore opportunities for leveraging DAs in production and logistics to achieve an inclusive workforce that involves older workers, people with varying cultural backgrounds, and those with disabilities.
7.5. Methodological insights
Approximately 60% of the studies reviewed by us developed concepts and qualitatively demonstrated the use of DAs. The remainder used quantitative or mixed methods to assess the usefulness of DAs, only a few of which were conducted in the field (see the summary of methods adopted in the literature sample in Table A1 in the Appendix). As field studies usually provide higher practical validity, we encourage future studies to test the practical feasibility and benefits of DAs. Our review also demonstrates that only a few studies combine performance measures (e.g. task success rate using DAs) with established questionnaires, such as the system usability scale (SUS), subjective assessment of speech system interfaces (SASSI), and NASA Task Load Index (NASA-TLX), while a larger number of studies used simple self-developed questionnaires. DAs in production and logistics normally rely on a conversational, graphical, or multi-modal user interface. Additionally, user satisfaction and experience are crucial for the adoption of DAs. Future studies may thus consider using multiple questionnaires to obtain a more comprehensive analysis of user experience when interacting with DAs. Moreover, our review demonstrates that most extant studies focus on production and maintenance, while fewer works investigate product development, quality management, and logistics despite their obvious relevance for the company. We thus call for more empirical studies to explore the potentials of DAs in these areas. Furthermore, although we did not identify studies in our literature sample that investigate DAs from an Operations Research perspective, we see fruitful opportunities in this field. DAs can be used to support decision-making in production and logistics, which is ultimately connected to solving different planning problems (e.g. assignment or routing problems in intralogistics). Interesting questions include which of these problems should be solved by the DA and which by a central IT system, and what methods are best suited to a quick decision-making process.
Proposition 9. More empirical research, for example, surveys, experiments, qualitative interviews, and case studies, is required to better understand workers’ acceptance and usage of DAs, as well as their resulting actions. In addition, researchers should develop planning models and solution procedures that enable DAs to provide quick decision support. Moreover, research should not only focus on production and maintenance, but extend to other areas such as product development, quality management, and logistics.
8. Conclusion
We presented a systematic literature review based on a sample of 69 papers and summarised existing scientific evidence on the applications, benefits, and challenges of using DAs in production and logistics. Based on a conceptual framework, we categorised three main tasks, namely information management, collaborative operations, and knowledge transfer. We performed a detailed analysis of how DAs support human workers in performing tasks across the areas of product design, production, maintenance, quality management, and logistics. We observed that research on DAs mainly concentrated on production and maintenance, and that only a few works investigated product design, quality management, and logistics. We discussed the potentials of DAs from the perspective of augmented analytics, hybrid team decision-making, and multi-modal assistance. Additionally, we highlighted the role of DAs in facilitating inclusive production and logistics. From a methodological perspective, we found that existing studies frequently used conceptual or qualitative methods to develop concepts and assess the benefits of DAs; however, additional field studies and comprehensive measurements of DAs are also essential in this line of research.
This study offers support to researchers and managers who are identifying the starting points in this emerging research field, by providing a comprehensive analysis of the various tasks that DAs can perform to help human workers in production and logistics. The objective of this study was to ensure that DAs improve the connection between workers, machines, and information systems, and effectively process information to support decision-making. Furthermore, we demonstrated that DAs could provide appropriate assistance in collaborative operations. Additionally, if they are jointly used with tracking technologies (e.g. motion tracking and eye tracking) and assistive technologies (e.g. AR and VR), they can provide robust assistance to workers. Managers can utilise the results of this study to further explore the possibilities of using DAs to assist workers in their daily tasks. These include combining DA and DT in shop floor management, using a DA and a scanner for order picking, and using a DA along with AR/VR for task guidance and training. Moreover, practitioners can use DAs to engage diverse workforces (e.g. older workers, people with different cultural backgrounds, or people with disabilities) in production and logistics. A few commercially available DAs already exist that can support production and logistics managers, such as SAP Conversational AI, Oracle Digital Assistants, and ChatGPT Enterprise. We expect new start-ups to enter this highly innovative area in the near future.
Some of the limitations of this study are: first, we may have missed some keywords during the database search and omitted a fraction of the literature not included in the Scopus and Web of Science databases. However, we conducted a snowball search as a complementary step to limit potential oversight. Second, subjectivity bias may have occurred during the literature sample selection. To address this shortcoming, we cross-checked the literature that presented DAs related to the framework among the authors.
Acknowledgements
The authors are grateful to the editor and anonymous reviewers for their constructive comments on an earlier version of this manuscript, which helped to improve the paper substantially.
Disclosure statement
No potential conflict of interest was reported by the author(s).
Data availability statement
Data is available on request from the authors.
Additional information
Notes on contributors
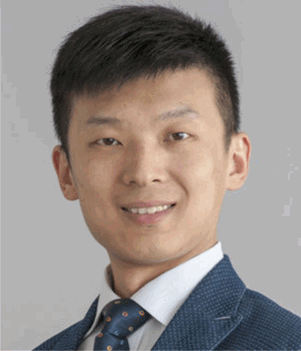
Ting Zheng
Ting Zheng is a postdoc and research associate at the Institute of Production and Supply Chain Management of Technical University of Darmstadt, Germany, since 2021. His research interests primarily relate to Industry 4.0, Eye tracking technology, Behavioural operations management, and Human-technology interaction. His works appeared in Computers & Industrial Engineering and the International Journal of Production Research, among others.
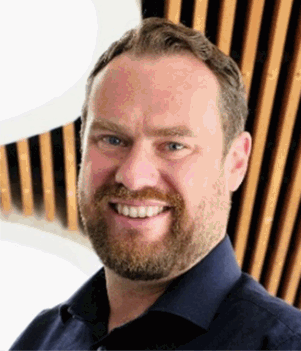
Eric H. Grosse
Eric H. Grosse is a Junior Professor and the Head of the Chair of Business Management and Digital Transformation in Operations Management at the Faculty of Human and Business Sciences at Saarland University, Germany. His research interests include sustainability in logistics and operations management with a focus on human-centric digital transformation. He is an area editor of Operations Management Research and co-edited several special issues in international journals. He is co-director of the Center for Digital Transformation (CeDiT) at Saarland University and serves as a co-chair of IFAC TC 5.2 ‘Management and Control in Manufacturing and Logistics’.
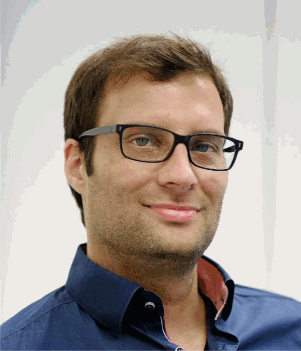
Stefan Morana
Stefan Morana is a Full Professor and the Head of the Chair of Business Management and Digital Transformation and Information Systems. His research focuses on human-centred design of interactive systems for digital transformation from the perspective of individuals, companies, and society. Thereby, the research focuses on affective, behavioural, and cognitive effects of the interaction between humans and interactive systems in different contexts. In various projects, he and his team bring their expertise and methodological knowledge on human-centred design and systematic evaluation (in laboratory and field studies) of interactive systems.
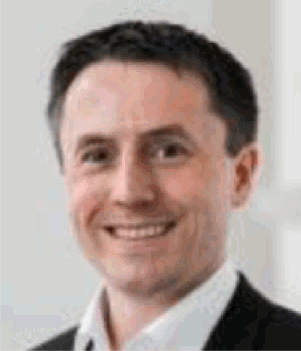
Christoph H. Glock
Christoph H. Glock is a Professor of Production and Supply Chain Management at Technical University of Darmstadt, Germany. His research interests include inventory management, supply chain management, warehousing, sustainable production, and human factors in logistics and inventory systems. His work has appeared in various international journals, such as IISE Transactions, Decision Sciences, OR Spectrum, the Journal of Business Logistics or the European Journal of Operational Research.
Notes
References
- Abate, Andrea F., Vincenzo Loia, Michele Nappi, Stefano Ricciardi, and Enrico Boccola. 2008. “ASSYST: Avatar baSed SYStem MainTenance." 2008 IEEE Radar Conference, Rome, Italy, pp. 1–6. https://doi.org/10.1109/RADAR.2008.4720962
- Abner, Brunno, Ricardo J. Rabelo, Saulo P. Zambiasi, and David Romero. 2020. “Production Management as-a-Service: A Softbot Approach.” In Advances in Production Management Systems. Towards Smart and Digital Manufacturing. Vol. 592, edited by Bojan Lalic, Vidosav Majstorovic, Ugljesa Marjanovic, Gregor von Cieminski, and David Romero, 19–30. IFIP Advances in Information and Communication Technology. Cham: Springer International Publishing.
- Aceta, Cristina, Patricia Casla, Izaskun Fernandez, and Aitor Soroa. 2022. “KIDE4Assistant: An Ontology-Driven Dialogue System Adaptation for Assistance in Maintenance Procedures.” In FOMI 2022: Formal Ontologies Meet Industry, edited by Emilio M. Sanfilippo, Mohamed-Hedi Karray, Dimitrios Kyritsis, and Arkopaul Sarkar, 1–8, Tarbes: CEUR Workshop Proceedings.
- Aceta, Cristina, Izaskun Fernández, and Aitor Soroa. 2022. “KIDE4I: A Generic Semantics-Based Task-Oriented Dialogue System for Human-Machine Interaction in Industry 5.0.” Applied Sciences 12 (3): 1192. https://doi.org/10.3390/app12031192.
- Adamopoulou, Eleni, and Lefteris Moussiades. 2020. “Chatbots: History, technology, and applications.” Machine Learning with Applications 2: 100006. https://doi.org/10.1016/j.mlwa.2020.100006.
- Ade, Nilesh, Noor Quddus, Trent Parker, and S. Camille Peres. 2020. “ProBot – A Procedure Chatbot for Digital Procedural Adherence.” In Proceedings of the 2020 HFES 64th International Annual Meeting. Vol. 64, 224–228. Sage CA: Los Angeles, CA: SAGE Publications. https://doi.org/10.1177/1071181320641054
- Afanasev, Maxim Y., Yuri V. Fedosov, Yuri S. Andreev, Anastasiya A. Krylova, Sergey A. Shorokhov, Kseniia V. Zimenko, and Mikhail V. Kolesnikov. 2019. “A Concept for Integration of Voice Assistant and Modular Cyber-Physical Production System.” In 2019 IEEE 17th International Conference on Industrial Informatics (INDIN), 27–32. Helsinki: IEEE. https://doi.org/10.1109/INDIN41052.2019.8972015
- Agarwal, Shubhangi, Bhawna Agarwal, and Ruchika Gupta. 2022. “Chatbots and Virtual Assistants: A Bibliometric Analysis.” Library Hi Tech 40 (4): 1013–1030. https://doi.org/10.1108/LHT-09-2021-0330 .
- Angelov, Stefan, and Milena Lazarova. 2019. “E-commerce Distributed Chatbot System.” In Proceedings of the 9th Balkan Conference on Informatics, edited by George Eleftherakis, Milena Lazarova, Adelina Aleksieva-Petrova, and Antoniya Tasheva, 1–8. New York, NY, USA: ACM.
- Baird, Aaron, and Likoebe M. Maruping. 2021. “The Next Generation of Research on IS Use: A Theoretical Framework of Delegation to and from Agentic IS Artifacts.” MIS Quarterly 45 (1): 315–341. https://doi.org/10.25300/MISQ/2021/15882.
- Barbosa, André S., Felipe P. Silva, Lucas Rafael dos Santos Crestani, and Rodrigo B. Otto. 2018. �����Virtual Assistant to Real Time Training on Industrial Environment.” In Advances in Transdisciplinary Engineering // Transdisciplinary Engineering Methods for Social Innovation of Industry 4.0. Vol. 7, edited by Margherita Peruzzini, Marcello Pellicciari, Cees Bil, Josip Stjepandić, and Nel Wognum, 33–42. Amsterdam: IOP.
- Behrendt, Wernher, and Felix Strohmeier. 2021. “Orchestration and Situation Awareness in an Assistance System for Assembly Tasks.” In Smart Technologies for Precision Assembly. Vol. 620, edited by Svetan Ratchev, 332–343. IFIP Advances in Information and Communication Technology. Cham: Springer International Publishing.
- Birkle, Caroline, David A. Pendlebury, Joshua Schnell, and Jonathan Adams. 2020. “Web of Science as a Data Source for Research on Scientific and Scholarly Activity.” Quantitative Science Studies 1 (1): 363–376. https://doi.org/10.1162/qss_a_00018.
- Borgianni, Y., E. Rauch, L. Maccioni, and B. G. Mark. 2018. User Experience Analysis in Industry 4.0 – the Use of Biometric Devices in Engineering Design and Manufacturing: IEEE IEEM2018: 16-19 Dec., Bangkok, Thailand. Piscataway, NJ: IEEE. http://ieeexplore.ieee.org/servlet/opac?punumber=8589962.
- Bousdekis, Alexandros, Gregoris Mentzas, Dimitris Apostolou, and Stefan Wellsandt. 2022. “Evaluation of AI-Based Digital Assistants in Smart Manufacturing.” In Advances in Production Management Systems. Smart Manufacturing and Logistics Systems: Turning Ideas Into Action. Vol. 664, edited by Duck Y. Kim, Gregor von Cieminski, and David Romero, 503–510. IFIP Advances in Information and Communication Technology. Cham: Springer Nature Switzerland.
- Bousdekis, Alexandros, Stefan Wellsandt, Enrica Bosani, Katerina Lepenioti, Dimitris Apostolou, Karl Hribernik, and Gregoris Mentzas. 2021. “Human-AI Collaboration in Quality Control with Augmented Manufacturing Analytics.” In Advances in Production Management Systems. Artificial Intelligence for Sustainable and Resilient Production Systems. Vol. 633, edited by Alexandre Dolgui, Alain Bernard, David Lemoine, Gregor von Cieminski, and David Romero, 303–310. IFIP Advances in Information and Communication Technology. Cham: Springer International Publishing.
- Brade, Jennifer, Alexander Kögel, Christian Fuchs, and Philipp Klimant. 2020. “Impact of First Person Avatar Representation in Assembly Simulations on Perceived Presence and Acceptance.” In Proceedings of the 15th International Joint Conference on Computer Vision, Imaging and Computer Graphics Theory and Applications, 17–24. SCITEPRESS – Science and Technology Publications. https://doi.org/10.5220/0008878700170024
- Burnham, Judy F. 2006. “Scopus Database: A Review.” 1–8.
- Camilleri, Mark A., and Ciro Troise. 2023. “Live Support by Chatbots with Artificial Intelligence: A Future Research Agenda.” Service Business 17 (1): 61–80. https://doi.org/10.1007/s11628-022-00513-9.
- Casillo, Mario, Francesco Colace, Loretta Fabbri, Marco Lombardi, Alessandra Romano, and Domenico Santaniello. 2020. “Chatbot in Industry 4.0: An Approach for Training New Employees.” In 2020 IEEE International Conference on Teaching, Assessment, and Learning for Engineering (TALE), 371–376. Takamatsu: IEEE. https://doi.org/10.1109/TALE48869.2020.9368339
- Chen, Tzu-Yu, Yu-Ching Chiu, Nanyi Bi, and Richard T.-H. Tsai. 2021. “Multi-Modal Chatbot in Intelligent Manufacturing.” IEEE Access 9: 82118–82129. https://doi.org/10.1109/ACCESS.2021.3083518.
- Chiu, Yu-Ching, Bo-Hao Chang, Tzu-Yu Chen, Cheng-Fu Yang, Nanyi Bi, Richard T.-H. Tsai, Hung-yi Lee, and Jane Y. Hsu. 2021. “Multi-modal User Intent Classification Under the Scenario of Smart Factory.” In The Thrity-Fifth AAAI Conference 2021. Vol. 35, 15771–15772. Palo Alto, CA: AAAI Press. https://doi.org/10.1609/aaai.v35i18.17882
- Choi, Hanjong, Takeshi Hamanaka, and Kanae Matsui. 2017. “Design and Implementation of Interactive Product Manual System Using Chatbot and Sensed Data.” In IEEE 6th Global Conference. http://ieeexplore.ieee.org/servlet/opac?punumber=8187602.
- Choi, Tsan-Ming, Subodha Kumar, Xiaohang Yue, and Hau-Ling Chan. 2022. “Disruptive Technologies and Operations Management in the Industry 4.0 Era and Beyond.” Production and Operations Management 31 (1): 9–31. https://doi.org/10.1111/poms.13622 .
- Cimini, Chiara, Alexandra Lagorio, David Romero, Sergio Cavalieri, and Johan Stahre. 2020. “Smart Logistics and The Logistics Operator 4.0.” IFAC-PapersOnLine 53 (2): 10615–10620. https://doi.org/10.1016/j.ifacol.2020.12.2818.
- Clarizia, Fabio, Massimo de Santo, Marco Lombardi, and Domenico Santaniello. 2021. “E-Learning and Industry 4.0: A Chatbot for Training Employees.” In Proceedings of Fifth International Congress on Information and Communication Technology. Vol. 1184, edited by Xin-She Yang, Simon Sherratt, Nilanjan Dey, and Amit Joshi, 445–453. Advances in Intelligent Systems and Computing. Singapore: Springer Singapore.
- Colabianchi, Silvia, Andrea Tedeschi, and Francesco Costantino. 2023. “Human-Technology Integration with Industrial Conversational Agents: A Conceptual Architecture and a Taxonomy for Manufacturing.” Journal of Industrial Information Integration 35: 100510. https://doi.org/10.1016/j.jii.2023.100510.
- Denyer, David, and David Tranfield. 2009. “Producing a Systematic Review.” In The Sage Handbook of Organizational Research Methods, edited by D. Buchanan, and A. Bryman, 671–689. Sage Publications Ltd.
- Diederich, Stephan, Alfred B. Brendel, Stefan Morana, and Lutz Kolbe. 2022. “On the Design of and Interaction with Conversational Agents: An Organizing and Assessing Review of Human-Computer Interaction Research.” JAIS(Journal of the Association for Information Systems) 23 (1): 96–138. https://doi.org/10.17705/1jais.00724
- Do, Sanghoon, and Jongpil Jeong. 2022. “Design and Implementation of RPA Based ChatMES System Architecture for Smart Manufacturing.” WSEAS Transactions on Computer Research 10: 88–92. https://doi.org/10.37394/232018.2022.10.11.
- Endsley, Mica R. 2017. “From Here to Autonomy.” Human Factors: The Journal of the Human Factors and Ergonomics Society 59 (1): 5–27. https://doi.org/10.1177/0018720816681350.
- Eurofound. 2021. “Disability and Labour Market Integration: Policy Trends and Support in EU Member States.”
- Eurostat. 2022a. “Manufacturing Statistics – NACE Rev. 2.” Accessed November 10, 2022. https://ec.europa.eu/eurostat/statistics-explained/index.php?title=Manufacturing_statistics_-_NACE_Rev._2#Structural_profile.
- Eurostat. 2022b. “Transportation and Storage Statistics – NACE Rev. 2.” Accessed February 2, 2023. https://ec.europa.eu/eurostat/statistics-explained/index.php?title=Transportation_and_storage_statistics_-_NACE_Rev._2#Structural_profile.
- Fleiner, Christian, Till Riedel, Michael Beigl, and Marcel Ruoff. 2021. “Ensuring a Robust Multimodal Conversational User Interface During Maintenance Work.” In Mensch und Computer 2021, edited by Stefan Schneegass, Bastian Pfleging, and Dagmar Kern, 79–91. New York, NY, USA: ACM.
- Følstad, Asbjørn, and Petter B. Brandtzæg. 2017. “Chatbots and the new World of HCI.” interactions 24 (4): 38–42. https://doi.org/10.1145/3085558 .
- Frederico, Guilherme F. 2023. “ChatGPT in Supply Chains: Initial Evidence of Applications and Potential Research Agenda.” Logistics 7 (2): 26. https://doi.org/10.3390/logistics7020026.
- Gärtler, Marco, and Benedikt Schmidt. 2021. “Practical Challenges of Virtual Assistants and Voice Interfaces in Industrial Applications.” In Proceedings of the 54th Hawaii International Conference on System Sciences, edited by Tung Bui. Proceedings of the Annual Hawaii International Conference on System Sciences: Hawaii International Conference on System Sciences, 4063-4072, Kauai, Hawaii. https://doi.org/10.24251/HICSS.2021.494.
- Ghofrani, Javad, and Dirk Reichelt. 2019. “Using Voice Assistants as HMI for Robots in Smart Production Systems.” In Proceedings of the 11th Central European Workshop on Services and Their Composition, edited by Stefan Kolb, and Christian Sturm. 2339, 62–65. CEUR Workshop Proceedings, Bayreuth, Germany.
- Goodhue, Dale L., and Ronald L. Thompson. 1995. “Task-Technology Fit and Individual Performance.” MIS Quarterly 19 (2): 213–236.
- Gough, David, Sandy Oliver, and James Thomas, eds. 2017. An Introduction to Systematic Reviews. Second Edition. Los Angeles: SAGE.
- Grosse, Eric H., Fabio Sgarbossa, Cecilia Berlin, and W. P. Neumann. 2023. “Human-centric Production and Logistics System Design and Management: Transitioning from Industry 4.0 to Industry 5.0.” International Journal of Production Research 61 (22): 7749–7759. https://doi.org/10.1080/00207543.2023.2246783.
- Hoy, Matthew B. 2018. “Alexa, Siri, Cortana, and More: An Introduction to Voice Assistants.” Medical Reference Services Quarterly 37 (1): 81–88. https://doi.org/10.1080/02763869.2018.1404391.
- Hsiao, Wei-Hung, and Tsung-Sheng Chang. 2019. “Exploring the Opportunity of Digital Voice Assistants in the Logistics and Transportation Industry.” Journal of Enterprise Information Management 32 (6): 1034–1050. https://doi.org/10.1108/JEIM-12-2018-0271.
- Hüsson, Daniel, Alexander Holland, and Rocío A. Sánchez. 2020. “Intelligent Personal Assistant in Business-Context: Key-Feature Evaluation for User Acceptance.” Business Systems Research Journal 11 (3): 147–166. https://doi.org/10.2478/bsrj-2020-0032 .
- IBM. 2022. “Watson Assistant: Build Better Virtual Agents, Powered by AI.” Accessed November 2, 2022. https://www.ibm.com/products/watson-assistant.
- Ivanov, Dmitry. 2023. “The Industry 5.0 Framework: Viability-Based Integration of the Resilience, Sustainability, and Human-Centricity Perspectives.” International Journal of Production Research 61 (5): 1683–1695. https://doi.org/10.1080/00207543.2022.2118892 .
- Jiang, Kan, Meilian Qin, and Shurong Li. 2022. “Chatbots in Retail: How do They Affect the Continued Use and Purchase Intentions of Chinese Consumers?” Journal of Consumer Behaviour 21 (4): 756–772. https://doi.org/10.1002/cb.2034.
- Juniper Research. 2023. “Chatbots: Vector Analysis, Competitor Leaderboard & Market Forecasts 2023-2028.”
- Jwo, Jung-Sing, Ching-Sheng Lin, and Cheng-Hsiung Lee. 2021. “An Interactive Dashboard Using a Virtual Assistant for Visualizing Smart Manufacturing.” Mobile Information Systems 2021: 1–9. https://doi.org/10.1155/2021/5578239 .
- Kagermann, Henning, Wolfgang Wahlster, and Johannes Helbig. 2013. “Securing the Future of German Manufacturing Industry: Recommendations for Implementing the Strategic Initiative INDUSTRIE 4.0.”
- Kassner, Laura, Pascal Hirmer, Matthias Wieland, Frank Steimle, Jan Königsberger, and Bernhard Mitschang. 2017. “The Social Factory: Connecting People, Machines and Data in Manufacturing for Context-Aware Exception Escalation.” Proceedings of the 50th Hawaii International Conference on System Sciences (2017). Proceedings of the Annual Hawaii International Conference on System Sciences: Hawaii International Conference on System Sciences.
- Kern, S., T. Dettborn, R. Eckhaus, Yang Ji, C. Erfurth, W. Rossak, and P. Braun. 2006. “Assistant-based Mobile Supply Chain Management.” In 13th Annual IEEE International Symposium and Workshop on Engineering of Computer-Based Systems (ECBS’06), 9–31. Potsdam: IEEE. https://doi.org/10.1109/ECBS.2006.25
- Kernan Freire, Samuel, Mina Foosherian, Chaofan Wang, and Evangelos Niforatos. 2023. “Harnessing Large Language Models for Cognitive Assistants in Factories.” In Proceedings of the 5th International Conference on Conversational User Interfaces, edited by Minha Lee, Cosmin Munteanu, Martin Porcheron, Johanne Trippas, and Sarah T. Völkel, 1–6. New York, NY, USA: ACM. https://doi.org/10.1145/3581641.3584042.
- Kernan Freire, Samuel, Evangelos Niforatos, Zoltan Rusak, Doris Aschenbrenner, and Alessandro Bozzon. 2022. “A Conversational User Interface for Instructional Maintenance Reports.” In 4th Conference on Conversational User Interfaces, edited by Martin Halvey, Mary E. Foster, Jeff Dalton, Cosmin Munteanu, and Johanne Trippas, 1–6. New York, NY, USA: ACM.
- Kernan Freire, Samuel, Evangelos Niforatos, Chaofan Wang, Santiago Ruiz-Arenas, Mina Foosherian, Stefan Wellsandt, and Alessandro Bozzon. 2023a. “Lessons Learned from Designing and Evaluating CLAICA: A Continuously Learning AI Cognitive Assistant.” In Proceedings of the 28th International Conference on Intelligent User Interfaces, 553–568. New York, NY: ACM. https://doi.org/10.1145/3581641.3584042
- Kernan Freire, Samuel, Chaofan Wang, Santiago Ruiz-Arenas, and Evangelos Niforatos. 2023b. “Tacit Knowledge Elicitation for Shop-Floor Workers with an Intelligent Assistant.” In Extended Abstracts of the 2023 CHI Conference on Human Factors in Computing Systems, edited by Albrecht Schmidt, Kaisa Väänänen, Tesh Goyal, Per O. Kristensson, and Anicia Peters, 1–7. New York, NY, USA: ACM.
- Knote, Robin, Andreas Janson, Matthias Söllner, and Jan M. Leimeister. 2019. “Classifying Smart Personal Assistants: An Empirical Cluster Analysis.” In Proceedings of the 52nd Hawaii International Conference on System Sciences, edited by Tung Bui, 2024–2033. Proceedings of the Annual Hawaii International Conference on System Sciences: Hawaii International Conference on System Sciences. Honolulu, Hawaii: University of Hawaii at Manoa, Hamilton Library, ScholarSpace. https://doi.org/10.24251/HICSS.2019.245.
- Lampen, Eva, Maximilian Liersch, and Jannes Lehwald. 2020. “Towards Motor Learning in Augmented Reality: Imitating an Avatar.” In HCI International 2020 – Late Breaking Posters. Vol. 1294, edited by Constantine Stephanidis, Margherita Antona, and Stavroula Ntoa, 181–188. Communications in Computer and Information Science. Cham: Springer International Publishing.
- Li, Chen, Dimitris Chrysostomou, and Hongji Yang. 2023. “A Speech-Enabled Virtual Assistant for Efficient Human–Robot Interaction in Industrial Environments.” Journal of Systems and Software 205: 111818. https://doi.org/10.1016/j.jss.2023.111818.
- Li, Chen, Dimitrios Chrysostomou, Xiaochun Zhang, and Hongji Yang. 2023. “IRWoZ: Constructing an Industrial Robot Wizard-of-OZ Dialoguing Dataset.” IEEE Access 11: 28236–28251. https://doi.org/10.1109/ACCESS.2023.3259325.
- Li, Chen, Andreas K. Hansen, Dimitrios Chrysostomou, Simon Bogh, and Ole Madsen. 2022. “Bringing a Natural Language-Enabled Virtual Assistant to Industrial Mobile Robots for Learning, Training and Assistance of Manufacturing Tasks.” In 2022 IEEE/SICE International Symposium on System Integration (SII), 238–243. Narvik: IEEE. https://doi.org/10.1109/SII52469.2022.9708757
- Li, Chen, Jinha Park, Hahyeon Kim, and Dimitrios Chrysostomou. 2021. “How Can I Help you? An Intelligent Virtual Assistant for Industrial Robots.” In Companion of the 2021 ACM/IEEE International Conference on Human-Robot Interaction, edited by Cindy Bethel, Ana Paiva, Elizabeth Broadbent, David Feil-Seifer, and Daniel Szafir, 220–224. New York, NY, USA: ACM.
- Li, Rui, and Weitian Wang. 2021. “Augmenting the Communication Naturalness via A 3D Audio-Visual Virtual Agent for Collaborative Robots.” In 2021 IEEE International Conference on Big Data (Big Data), 5944–5946. Orlando, FL: IEEE. https://doi.org/10.1109/BigData52589.2021.9671664
- Li, Chen, and Hong J. Yang. 2021. “Bot-X: An AI-Based Virtual Assistant for Intelligent Manufacturing.” Multiagent and Grid Systems 17 (1): 1–14. https://doi.org/10.3233/MGS-210340.
- Li, Chen, Xiaochun Zhang, Dimitrios Chrysostomou, and Hongji Yang. 2022. “ToD4IR: A Humanised Task-Oriented Dialogue System for Industrial Robots.” IEEE Access 10: 91631–91649. https://doi.org/10.1109/ACCESS.2022.3202554.
- Loh, Siu H., Vincent J. Jeuk, Jia J. Sim, and Kim H. Yeap. 2023. “Implementation of Artificial Intelligence Chatbot in Semiconductor Manufacturing to Optimize Overall Equipment Effectiveness.” In 2023 IEEE 13th International Conference on Control System, Computing and Engineering (ICCSCE), 220–225. Penang: IEEE. https://doi.org/10.1109/ICCSCE58721.2023.10237164
- Longo, Francesco, Letizia Nicoletti, and Antonio Padovano. 2017. “Smart Operators in Industry 4.0: A Human-Centered Approach to Enhance Operators’ Capabilities and Competencies Within the new Smart Factory Context.” Computers & Industrial Engineering 113: 144–159. https://doi.org/10.1016/j.cie.2017.09.016 .
- Longo, Francesco, Letizia Nicoletti, and Antonio Padovano. 2022. “New Perspectives and Results for Smart Operators in Industry 4.0: A Human-Centered Approach.” Computers & Industrial Engineering 163: 107824. https://doi.org/10.1016/j.cie.2021.107824.
- Maedche, Alexander, Christine Legner, Alexander Benlian, Benedikt Berger, Henner Gimpel, Thomas Hess, Oliver Hinz, Stefan Morana, and Matthias Söllner. 2019. “AI-Based Digital Assistants.” Business & Information Systems Engineering 61 (4): 535–544. https://doi.org/10.1007/s12599-019-00600-8.
- Makokha, Joseph. 2022. “Enhancing Human-AI (H-AI) Collaboration On Design Tasks Using An Interactive Text/Voice Artificial Intelligence (AI) Agent.” In Proceedings of the 2022 International Conference on Advanced Visual Interfaces, edited by Paolo Bottoni, and Emanuele Panizzi, 1–4. New York, NY, USA: ACM.
- Mantravadi, Soujanya, Andreas D. Jansson, and Charles Møller. 2020. “User-Friendly MES Interfaces – Recommendations for an AI-Based Chatbot Assistance in Industry 4.0 Shop Floors.” In Intelligent Information and Database Systems. ACIIDS 2020. Lecture Notes in Computer Science. Vol. 12034: 189–201. https://doi.org/10.1007/978-3-030-42058-1_16 .
- Mark, Benedikt G., Sarah Hofmayer, Erwin Rauch, and Dominik T. Matt. 2019. “Inclusion of Workers with Disabilities in Production 4.0: Legal Foundations in Europe and Potentials Through Worker Assistance Systems.” Sustainability 11 (21): 5978. https://doi.org/10.3390/su11215978.
- Mark, Benedikt G., Erwin Rauch, and Dominik T. Matt. 2022. “Systematic Selection Methodology for Worker Assistance Systems in Manufacturing.” Computers & Industrial Engineering 166: 107982. https://doi.org/10.1016/j.cie.2022.107982.
- Murciego, Álvaro L., Diego M. Jiménez-Bravo, Denis Pato Martínez, Adrián Valera Román, and Gabino Luis Lazo. 2020. “Voice Assistant and Route Optimization System for Logistics Companies in Depopulated Rural Areas.” Sustainability 12 (13): 5377. https://doi.org/10.3390/su12135377.
- Myers, Karen, Pauline Berry, Jim Blythe, Ken Conley, Melinda Gervasio, Deborah McGuinness, David Morley, Avi Pfeffer, Martha Pollack, and Milind Tambe. 2007. “An Intelligent Personal Assistant for Task and Time Management.”
- Neumann, W. P., Sven Winkelhaus, Eric H. Grosse, and Christoph H. Glock. 2021. “Industry 4.0 and the Human Factor – A Systems Framework and Analysis Methodology for Successful Development.” International Journal of Production Economics 233: 107992. https://doi.org/10.1016/j.ijpe.2020.107992.
- Nyrkko, Seppo, Lauri Carlson, Matti Keijola, Helena Ahonen-Myka, Jyrki Niemi, Jussi Piitulainen, Sirke Viitanen, et al. 2007. “Ontology-based Knowledge in Interactive Maintenance Guide.” In 2007 40th Annual Hawaii International Conference on System Sciences (HICSS'07), 47. Waikoloa, HI: IEEE. https://doi.org/10.1109/HICSS.2007.411
- OpenAI. 2023a. “ChatGPT FAQ.” Accessed January 31, 2023. https://help.openai.com/en/articles/6783457-chatgpt-general-faq.
- OpenAI. 2023b. “Be My Eyes: Be My Eyes uses GPT-4 to transform visual accessibility.” Accessed August 30, 2023. https://openai.com/customer-stories/be-my-eyes.
- Oracle. 2021. “Fast-Evolving Digital Assistants are Getting More Attention from Manufacturers.”
- Oracle. 2022. “What is a Digital Assistant?” https://www.oracle.com/chatbots/what-is-a-digital-assistant/.
- Pace, Francesco de, Federico Manuri, Andrea Sanna, and Davide Zappia. 2019. “A Comparison Between Two Different Approaches for a Collaborative Mixed-Virtual Environment in Industrial Maintenance.” Frontiers in Robotics and AI 6: 18. https://doi.org/10.3389/frobt.2019.00018.
- Penica, Mihai, Mangolika Bhattacharya, William O’Brien, Sean McGrath, Martin Hayes, and Eoin O’Connell. 2023. “Adaptable Decision Making Chatbot System: Unlocking Interoperability in Smart Manufacturing.” In 2023 International Conference on Computing, Electronics & Communications Engineering (iCCECE), 23–29. Swansea: IEEE. https://doi.org/10.1109/iCCECE59400.2023.10238531
- Pereira, Rodrigo, Claudio Lima, Tiago Pinto, and Arsénio Reis. 2023. “Virtual Assistants in Industry 4.0: A Systematic Literature Review.” Electronics 12 (19): 4096. https://doi.org/10.3390/electronics12194096.
- Peruzzini, Margherita, Fabio Grandi, and Marcello Pellicciari. 2020. “Exploring the Potential of Operator 4.0 Interface and Monitoring.” Computers & Industrial Engineering 139: 105600. https://doi.org/10.1016/j.cie.2018.12.047.
- Pfohl, Hans-Christian. 2022. Logistics Systems: Business Fundamentals. Heidelberg: Springer Nature; Springer.
- Porter, Michael E. 2011. Competitive Advantage of Nations: Creating and Sustaining Superior Performance: Creating and Sustaining Superior Performance. Simon and Schuster; Free Press.
- Quarteroni, Silvia. 2018. “Natural Language Processing for Industry.” Informatik-Spektrum 41 (2): 105–112. https://doi.org/10.1007/s00287-018-1094-1.
- Rabelo, Ricardo J., David Romero, and Saulo P. Zambiasi. 2018. “Softbots Supporting the Operator 4.0 at Smart Factory Environments.” In Advances in Production Management Systems. Smart Manufacturing for Industry 4.0. Vol. 536, edited by Ilkyeong Moon, Gyu M. Lee, Jinwoo Park, Dimitris Kiritsis, and Gregor von Cieminski, 456–464. IFIP Advances in Information and Communication Technology. Cham: Springer International Publishing.
- Rabelo, Ricardo J., David Romero, Saulo P. Zambiasi, and Luciano C. Magalhães. 2021. “When Softbots Meet Digital Twins: Towards Supporting the Cognitive Operator 4.0.” In Advances in Production Management Systems. Artificial Intelligence for Sustainable and Resilient Production Systems. Vol. 634, edited by Alexandre Dolgui, Alain Bernard, David Lemoine, Gregor von Cieminski, and David Romero, 37–47. IFIP Advances in Information and Communication Technology. Cham: Springer International Publishing.
- Rabelo, Ricardo J., Saulo P. Zambiasi, and David Romero. 2019. “Collaborative Softbots: Enhancing Operational Excellence in Systems of Cyber-Physical Systems.” In Collaborative Networks and Digital Transformation. Vol. 568, edited by Luis M. Camarinha-Matos, Hamideh Afsarmanesh, and Dario Antonelli, 55–68. IFIP Advances in Information and Communication Technology. Cham: Springer International Publishing.
- Rabelo, Ricardo J., Saulo P. Zambiasi, and David Romero. 2023. “Softbots 4.0: Supporting Cyber-Physical Social Systems in Smart Production Management.” International Journal of Industrial Engineering and Management 14 (1): 63–94. https://doi.org/10.24867/IJIEM-2023-1-325.
- Reis, Arsénio, João Barroso, Arlindo Santos, Paulo Rodrigues, and Rodrigo Pereira. 2022. “Virtual Assistance in the Context of the Industry 4.0: A Case Study at Continental Advanced Antenna.” In Information Systems and Technologies. Vol. 468, edited by Alvaro Rocha, Hojjat Adeli, Gintautas Dzemyda, and Fernando Moreira, 651–662. Lecture Notes in Networks and Systems. Cham: Springer International Publishing.
- Romero, David, Johan Stahre, Thorsten Wuest, Ovidiu Noran, Peter Bernus, Åsa Fast-Berglund, and Dominic Gorecky, eds. 2016. Towards an Operator 4.0 Typology: A Human-Centric Perspective on the Fourth Industrial Revolution Technologies.
- Rooein, Donya, Devis Bianchini, Francesco Leotta, Massimo Mecella, Paolo Paolini, and Barbara Pernici. 2020. “Chatting About Processes in Digital Factories: A Model-Based Approach.” In Enterprise, Business-Process and Information Systems Modeling. Vol. 387, edited by Selmin Nurcan, Iris Reinhartz-Berger, Pnina Soffer, and Jelena Zdravkovic, 70–84. Lecture Notes in Business Information Processing. Cham: Springer International Publishing.
- Roth, Elisa, Mirco Moencks, and Thomas Bohné. 2023. “The Augmented Workforce: A Systematic Review of Operator Assistance Systems.” In Handbook of Human-Machine Systems, 267–279. https://doi.org/10.1002/9781119863663.ch23.
- Santos, Joao, Joel J. P. C. Rodrigues, Joao Casal, Kashif Saleem, and Victor Denisov. 2018. “Intelligent Personal Assistants Based on Internet of Things Approaches.” IEEE Systems Journal 12 (2): 1793–1802. https://doi.org/10.1109/JSYST.2016.2555292.
- Schmidt, Benedikt, Reuben Borrison, Andrew Cohen, Marcel Dix, Marco Gärtler, Martin Hollender, Benjamin Klöpper, Sylvia Maczey, and Shunmuga Siddharthan. 2018. “Industrial Virtual Assistants – Challenges and Opportunities.” In Proceedings of the 2018 ACM International Joint Conference and 2018 International Symposium on Pervasive and Ubiquitous Computing and Wearable Computers, 794–801. New York, NY: ACM. https://doi.org/10.1145/3267305.3274131
- Schwartz, Tim, Ingo Zinnikus, Hans-Ulrich Krieger, Christian Bürckert, Joachim Folz, Bernd Kiefer, Peter Hevesi, et al. 2016. “Hybrid Teams: Flexible Collaboration Between Humans, Robots and Virtual Agents.” In Multiagent System Technologies. Vol. 9872, edited by Matthias Klusch, Rainer Unland, Onn Shehory, Alexander Pokahr, and Sebastian Ahrndt, 131–146. Lecture Notes in Computer Science. Cham: Springer International Publishing.
- Serras, Manex, Laura García-Sardiña, Bruno Simões, Hugo Álvarez, and Jon Arambarri. 2020a. ��AREVA – Augmented Reality Voice Assistant for Industrial Maintenance.” 135–138. https://doi.org/10.26342/2020-65-21.
- Serras, Manex, Laura García-Sardiña, Bruno Simões, Hugo Álvarez, and Jon Arambarri. 2020b. “Dialogue Enhanced Extended Reality: Interactive System for the Operator 4.0.” Applied Sciences 10 (11): 3960. https://doi.org/10.3390/app10113960.
- Silva, Allan d. B., Marcio M. Gomes, Cristiano A. Da Costa, Rodrigo Da Rosa Righi, Jorge L. V. Barbosa, Gustavo Pessin, Geert de Doncker, and Gustavo Federizzi. 2020. “Intelligent Personal Assistants: A Systematic Literature Review.” Expert Systems with Applications 147: 113193. https://doi.org/10.1016/j.eswa.2020.113193.
- Talacio, Matheus, Gustavo Funchal, Victória Melo, Luis Piardi, Marcos Vallim, and Paulo Leitao. 2021. “Machine Vision to Empower an Intelligent Personal Assistant for Assembly Tasks.” In International Conference on Optimization, Learning Algorithms and Applications // Optimization, Learning Algorithms and Applications. Vol. 1488, edited by Ana I. Pereira, Florbela P. Fernandes, João P. Coelho, João P. Teixeira, Maria F. Pacheco, Paulo Alves, and Rui P. Lopes, 447–462. Cham: Springer International Publishing.
- Tanaka, Kazuaki, Tomoaki Ozaki, Norihiro Abe, and Horikazu Taki. 2003. “Verbal/Nonverbal Communication Between Man and Avatar in Virtual Mechanical Assembly Training System.” In Third International Conference on Virtual Reality and Its Application in Industry, edited by Zhigeng Pan, and Jiaoying Shi, 142–151. Hangzhou: SPIE Proceedings: SPIE. https://doi.org/10.1117/12.497762.
- Trappey, Amy J., Charles V. Trappey, Min-Hua Chao, and Chun-Ting Wu. 2022. “VR-enabled Engineering Consultation Chatbot for Integrated and Intelligent Manufacturing Services.” Journal of Industrial Information Integration 26: 100331. https://doi.org/10.1016/j.jii.2022.100331.
- Trist, Eric. 1981. The Evolution of Socio Technical-Systems: A Conceptual Framework and an Action Research Program. Toronto: Ontario Quality of Working Life Centre.
- Turner, Christopher J., Ruidong Ma, Jingyu Chen, and John Oyekan. 2021. “Human in the Loop: Industry 4.0 Technologies and Scenarios for Worker Mediation of Automated Manufacturing.” IEEE Access 9: 103950–66. https://doi.org/10.1109/ACCESS.2021.3099311.
- van Pinxteren, Michelle M., Mark Pluymaekers, and Jos G. Lemmink. 2020. “Human-like Communication in Conversational Agents: A Literature Review and Research Agenda.” Journal of Service Management 31 (2): 203–225. https://doi.org/10.1108/JOSM-06-2019-0175.
- von Wolff, Raphael M, Sebastian Hobert, Matthias Schumann, and Raphael Meyer von Wolff. 2019. “How May I Help You? – State of the Art and Open Research Questions for Chatbots at the Digital Workplace.” In Proceedings of the 52nd Hawaii International Conference on System Sciences, edited by Tung Bui, 95–104.. Proceedings of the Annual Hawaii International Conference on System Sciences: Hawaii International Conference on System Sciences. Hawaii: University of Hawaii at M?noa, Hamilton Library. https://doi.org/10.24251/HICSS.2019.013.
- Wallace, Richard S., ed. 2009. The Anatomy of A.L.I.C.E. With the assistance of G. R. Epstein and Beber G. Dordrecht: Springer Netherlands.
- Wang, Tzu-Yang, Yuji Sato, Mai Otsuki, Hideaki Kuzuoka, and Yusuke Suzuki. 2020. “Effect of Body Representation Level of an Avatar on Quality of AR-Based Remote Instruction.” Multimodal Technologies and Interaction 4 (1): 3. https://doi.org/10.3390/mti4010003.
- Weizenbaum, Joseph. 1966. “ELIZA—a Computer Program for the Study of Natural Language Communication Between man and Machine.” Communications of the ACM 9 (1): 36–45. https://doi.org/10.1145/365153.365168.
- Wellsandt, Stefan, Mina Foosherian, Katerina Lepenioti, Mattheos Fikardos, Gregoris Mentzas, and Klaus-Dieter Thoben. 2022. “Supporting Data Analytics in Manufacturing with a Digital Assistant.” In Advances in Production Management Systems. Smart Manufacturing and Logistics Systems: Turning Ideas Into Action. Vol. 664, edited by Duck Y. Kim, Gregor von Cieminski, and David Romero, 511–518. IFIP Advances in Information and Communication Technology. Cham: Springer Nature Switzerland.
- Wellsandt, Stefan, Mina Foosherian, and Klaus-Dieter Thoben. 2020. “Interacting with a Digital Twin Using Amazon Alexa.” Procedia Manufacturing 52: 4–8. https://doi.org/10.1016/j.promfg.2020.11.002.
- Wellsandt, Stefan, Karl Hribernik, and Klaus-Dieter Thoben. 2021. “Anatomy of a Digital Assistant.” In Advances in Production Management Systems. Artificial Intelligence for Sustainable and Resilient Production Systems. Vol. 633, edited by Alexandre Dolgui, Alain Bernard, David Lemoine, Gregor von Cieminski, and David Romero, 321–330. IFIP Advances in Information and Communication Technology. Cham: Springer International Publishing.
- Wellsandt, Stefan, Konstantin Klein, Karl Hribernik, Marco Lewandowski, Alexandros Bousdekis, Gregoris Mentzas, and Klaus-Dieter Thoben. 2021. “Towards Using Digital Intelligent Assistants to Put Humans in the Loop of Predictive Maintenance Systems.” IFAC-PapersOnLine 54 (1): 49–54. https://doi.org/10.1016/j.ifacol.2021.08.005.
- Wellsandt, Stefan, Konstantin Klein, Karl Hribernik, Marco Lewandowski, Alexandros Bousdekis, Gregoris Mentzas, and Klaus-Dieter Thoben. 2022. “Hybrid-Augmented Intelligence in Predictive Maintenance with Digital Intelligent Assistants.” Annual Reviews in Control 53: 382–390. https://doi.org/10.1016/j.arcontrol.2022.04.001.
- Wellsandta, Stefan, Zoltan Rusak, Santiago Ruiz Arenas, Doris Aschenbrenner, Karl A. Hribernik, and Klaus-Dieter Thoben. 2020. “Concept of a Voice-Enabled Digital Assistant for Predictive Maintenance in Manufacturing.” SSRN Electronic Journal. https://doi.org/10.2139/ssrn.3718008.
- Wohlin, Claes. 2014. “Guidelines for Snowballing in Systematic Literature Studies and a Replication in Software Engineering.” In Proceedings of the 18th International Conference on Evaluation and Assessment in Software Engineering – EASE ‘14, edited by Martin Shepperd, Tracy Hall, and Ingunn Myrtveit, 1–10. New York, NY: ACM Press.
- Ye, Yang, Hengxu You, and Jing Du. 2023. “Improved Trust in Human-Robot Collaboration with ChatGPT.” IEEE Access 11: 55748–55754. https://doi.org/10.1109/ACCESS.2023.3282111.
- Zambiasi, Lara P., Ricardo J. Rabelo, Saulo P. Zambiasi, and Rafael Lizot. 2022. “Supporting Resilient Operator 5.0: An Augmented Softbot Approach.” In Advances in Production Management Systems. Smart Manufacturing and Logistics Systems: Turning Ideas Into Action. Vol. 664, edited by Duck Y. Kim, Gregor von Cieminski, and David Romero, 494–502. IFIP Advances in Information and Communication Technology. Cham: Springer Nature Switzerland.
- Zambiasi, Lara P., Ricardo J. Rabelo, Saulo P. Zambiasi, and David Romero. 2023. “Metaverse-Based Softbot Tutors for Inclusive Industrial Workplaces: Supporting Impaired Operators 5.0.” In Advances in Production Management Systems. Production Management Systems for Responsible Manufacturing, Service, and Logistics Futures. Vol. 689, edited by Erlend Alfnes, Anita Romsdal, Jan O. Strandhagen, Gregor von Cieminski, and David Romero, 662–677. IFIP Advances in Information and Communication Technology. Cham: Springer Nature Switzerland.
- Zhu, Zhiwei, Vlad Branzoi, Michael Wolverton, Glen Murray, Nicholas Vitovitch, Louise Yarnall, Girish Acharya, Supun Samarasekera, and Rakesh Kumar. 2014. “AR-mentor: Augmented Reality Based Mentoring System.” In 2014 IEEE International Symposium on Mixed and Augmented Reality (ISMAR), 17–22. Munich: IEEE. https://doi.org/10.1109/ISMAR.2014.6948404
- Ziegeler, Dirk, and Detlef Zuehlke. 2005. “Emotional User Interfaces and Humanoid Avatars in Industrial Environments.” IFAC Proceedings Volumes 38 (1): 106–111. https://doi.org/10.3182/20050703-6-CZ-1902.01420.
- Zimmer, Melanie, Ali Al-Yacoub, Pedro Ferreira, and Niels Lohse. 2020. “Towards Human-Chatbot Interaction: A Virtual Assistant for the Ramp-up Process.” In UKRAS20 Conference: “Robots into the Real World” Proceedings, 108–110. UK-RAS Conference for PhD and Early Career Researchers Proceedings: EPSRC UK-RAS Network. https://doi.org/10.31256/Qx5Dt5V