Abstract
In the automotive industry, some vehicles, failed vehicles, cannot be produced according to the planned schedule due to some reasons such as material shortage, paint failure, etc. These vehicles are pulled out of the sequence, potentially resulting in an increased work overload. On the other hand, the reinsertion of failed vehicles is executed dynamically as suitable positions occur. In case such positions do not occur enough, either the vehicles waiting for reinsertion accumulate or reinsertions are made to worse positions by sacrificing production efficiency. This study proposes a bi-objective two-stage stochastic program and formulation improvements for a mixed-model sequencing problem with stochastic product failures and integrated reinsertion process. Moreover, an evolutionary optimisation algorithm, a two-stage local search algorithm, and a hybrid approach are developed. Numerical experiments over a case study show that while the hybrid algorithm better explores the Pareto front representation, the local search algorithm provides more reliable solutions regarding work overload objective. Finally, the results of the dynamic reinsertion simulations show that we can decrease the work overload by 20% while significantly decreasing the waiting time of the failed vehicles by considering vehicle failures and integrating the reinsertion process into the mixed-model sequencing problem.
Acknowledgments
The authors acknowledge Clemson University for generous allotment of compute time on Palmetto cluster.
Data availability statement
Data available on request from the authors.
Disclosure statement
No potential conflict of interest was reported by the author(s).
Additional information
Notes on contributors
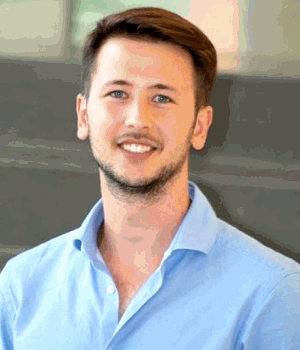
I. Ozan Yilmazlar
Ibrahim Ozan Yilmazlar, PhD, received his doctoral degree from the Clemson University in Industrial Engineering in 2023, focusing on mixed-model sequencing problems. Dr. Yilmazlar is interested in improving the production efficiency of assembly lines by focusing on operational decisions such as sequencing. He is also interested in last-mile delivery problems. He develops mathematical models, heuristics and metaheuristics to address optimisation problems. He is currently working as a Operations Research Scientist at JustEatTakeaway.
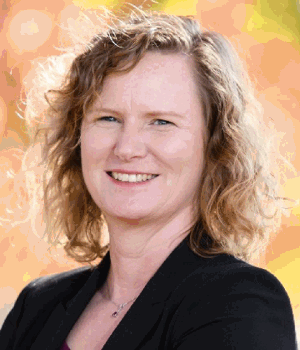
Mary E. Kurz
Mary E. Kurz, PhD, is an Associate Professor in Industrial Engineering at Clemson University. She is a member of INFORMS and a Senior member of the Institute of Industrial Engineers. Dr. Kurz received her doctoral degree from the University of Arizona in Systems and Industrial Engineering in 2001, focusing on scheduling flexible flowlines. Dr. Kurz is interested in improving the efficiency of large assembly systems through better integration of various functions, such as sequencing, line balancing, and material handling. She develops mathematical models, heuristics and metaheuristics to evaluate the potential for system improvements.
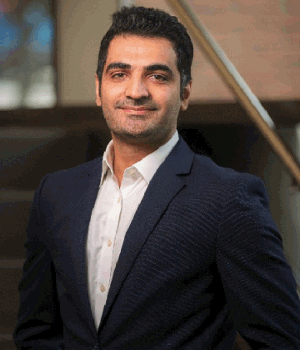
Hamed Rahimian
Hamed Rahimian, PhD, is an Assistant Professor in the Department of Industrial Engineering at Clemson University. His research focuses on the theory and applications of stochastic and robust optimisation.