Abstract
The Internet of Behaviours (IoB) is a network designed and used for replicating, understanding, predicting, and influencing human behaviour through data analytics. This study aims to advance our understanding of how the IoB will impact supply chain and operations management (SCOM). Based on a combination of the existing IoB frameworks with industrial Metaverse and digital supply chain twins, we propose a framework for IoB in SCOM encompassing management, organisation, and technology dimensions. We conclude that the IoB will impact a multitude of SCOM areas such as supply chain design, demand forecasting, sales and operations planning, distribution and transportation, inventory and production management, sourcing strategy and purchasing, vehicle routeing, supply chain resilience and sustainability. We offer a structured future research agenda pointing to new research questions and topics stemming from IoB-driven real-time visibility, data analytics, and remote control. Methodology of SCOM can also be enriched by the IoB through a combination of artificial intelligence and digital technology with operations research and behavioural operations management.
Sustainable Development Goals:
1. Introduction
Internet of Behaviours (IoB) is a new area that can influence supply chain and operations management (SCOM). Initial studies on the IoB suggest that it could have a wide range of potential implications for SCOM (Zhao et al. Citation2023). For example, companies can use IoB to understand and influence customer behaviours and gather data on supplier behaviour, both under normal conditions and during disruptions. However, to the best of our knowledge, the existing literature on the IoB has not specifically analyzed its impact on SCOM, and no published research has yet conceptualised these potential future impacts.
This position paper aims to close this research gap and offer some insights into how IoB can influence SCOM both from practical decision-making and methodological, academic perspectives. Our main objective is to highlight the potential and significance of the IoB for SCOM research and practice. Since the topic of the IoB is relatively new, we do not delve into empirical case studies or technological implementations, as these are currently lacking. Instead, we consider the IoB through the lens of existing frameworks such as the industrial Metaverse and digital supply chain twins. This allows us to explore the potential topics and implications that the IoB might bring to SCOM.
Let us start with the IoB definition. The literature on the IoB commonly refers to professor Gote Nyman (Nyman Citation2012), who coined the term Internet of Behaviours (IoB) in 2012 ‘to describe a network in which behavioural patterns would have an IoB address in the same way that each device has an IP address in the Internet of Things (IoT)’ (Lareo Citation2023). Zhao et al. (Citation2023) underline that the key ideas of the IoB are that ‘people’s behaviors are very good predictors of their needs, and hence technology companies can network human behaviors and construct behavior-based systems to deliver more timely, responsive, and intelligent services with privacy protection.’ Sun et al. (Citation2023) define IoB as ‘a network of interconnected physical objects that collect and exchange information over the Internet, linking this data to specific human behaviors.’
The obvious idea behind the IoB is that the new methods and technology for data analytics can allow analysing, understanding, and influencing behaviours of people (or their digital twins) based on their actions on the Internet. Gartner estimated in 2020 that ‘by the end of 2025, more than half of the World’s population will have been exposed to at least one IoB program, whether commercial or government-sponsored’ (Sun et al. Citation2023). For example, in healthcare, patients can be equipped with sensors sending information about their health. This can help understand their physical conditions and develop specific measures to influence patients’ behaviours for health improvement. Smartwatch is one of the common technologies used in this area.
When summarising the views of the IoB, we can define the IoB as a network designed and used for replicating, understanding, predicting, and influencing human behaviour through data analytics. For the SCOM, the IoB could create a new digital world where every physical supply chain entity (e.g. customers, products, suppliers, and trucks) has a digital replica characterising its behaviour. When taking an extra step forward, these entities can be equipped with artificial intelligence (AI) capabilities possessing and changing their own properties and behaviours and even influencing the behaviours and processes of physical entities.
In order to analyse the potential impacts of the IoB on SCOM, we refer to the concepts of industrial Metaverse and digital supply chain twins (Berti and Finco Citation2022; Dolgui and Ivanov Citation2023), which share common elements with the IoB. Through a symbiosis of these concepts with the Sun et al. (Citation2023) and Zhao et al. (Citation2023) frameworks of the IoB, we propose a framework for IoB in SCOM encompassing management, organisation and technology dimensions.
The remainder of this paper is organised as follows. Section 2 reviews the extant literature and describes the existing frameworks of the IoB, industrial Metaverse, digital supply chain twins, and Industry 5.0 frameworks. Section 3 merges frameworks described in Section 2 into an IoB for SCOM framework. Section 4 discusses the impacts of IoB on SCOM practice and theory. We conclude in Section 5 by summarising major outcomes of this study and outlining some future research directions.
2. Literature analysis
2.1 . IoB frameworks
Literature on the IoB is at its infancy. It can be roughly classified into technological and conceptual studies. On the technology side, Stary (Citation2020) examined the recognisable behaviour and user behavioural interaction patterns to carry out personalised intelligent development. Moghaddam et al. developed a system for crowd monitoring and queue management. With the help of a data-driven approach, the system obtains real-time data, builds user behaviour models, adapts to changing behaviours in real time, and constantly learns from repeated behaviours. Naturally AI and data analytics play a vital role in the IoB research. Embarak (Citation2022) integrated explainable AI technology achieving power consumption reduction by influencing user behaviour. An application is shown using student behaviour to assess the usefulness of education systems. Yan et al. (Citation2020) used data mining techniques to build a customer-centered intelligent decision-making model to improve customer experience. Li, Lin, and Chou (Citation2022) report development of an analysis and prediction method for dangerous driving behaviour using a fuzzy inference system.
On the conceptual side, the primary focus of the literature is to depart from IoT (Internet-of-Things) to explain the IoB. The IoB is understood as an extension of the IoT . Kidd (Citation2019) proposed a four-stage framework consisting of data, information, knowledge, and wisdom. Data and information are related to the IoT while knowledge and wisdom are connected to the IoB. While the IoT connects data from different machines and devices for intelligent identification and management, the IoB serves as a human-machine interface for capturing behavioural dynamics. IoB integrates digital technology, data analytics and behavioural science connecting physical, digital, and society spaces.
According to Sun et al. (Citation2023), IoB is ‘a research theme that aims to analyze human behavior data on the Internet from the perspective of behavioral psychology, obtain insights about human behavior, and better understand the intention behind the behaviour.’ Their IoB framework defines five major steps, i.e. track behaviour through IoT devices, collect data, analyse data to obtain logical and structured information, understand information to obtain accurate knowledge through behavioural science, and predict and influence user behaviour (related to wisdom dimension). In addition, they define three major IoB dimensions, which are real-time, automony and reliability. Finally, their survey points to five major technologies intersecting and enabling the IoB, namely Blockchain, digital twin, AI, 5G, and big data. We take this insight into the development of our IoB framework for SCOM.
Zhao et al. (Citation2023) propose a five-layer IoB architecture consisting of behaviour perception, behaviour networking, behaviour computing, service provision and security/privacy. Furthermore, they identify four important implications of behaviour computing, i.e. intention inference, behaviour derivation, behaviour programming, and behaviour-chain optimisation.
2.2. Relevant frameworks in SCOM
IoB is about cyber-physical systems, digital twins, metaverse, and Industry 5.0. Dolgui and Ivanov (Citation2023) developed a metaverse SCOM framework. They show that ‘physical and metaverse worlds are connected through digital technology such as augmented/virtual reality, blockchain, artificial intelligence, the Internet of things, 5G/Edge computing, ERP (enterprise resource planning), big data analytics, and simulation’ (Brintrup et al. Citation2020; Cai, Choi, and Zhang Citation2021; Cui, Li, and Zhang Citation2022; Dolgui and Ivanov Citation2022; Holzwarth, Staib, and Ivanov Citation2022). Their framework for metaverse SCOM encompasses seven socio-technological dimensions, i.e. organisation, management, people, technology, scope, task, and modelling. They show that
new research areas can appear which are specifically dedicated to the metaverse and novel SCOM processes and decision-making areas (e.g. joint demand forecasting for metaverse and physical products, digital inventory allocation in the metaverse, integrated production planning for the metaverse and physical worlds, and pricing and contracting for digital products), as well as new performance measures (e.g. virtual customer experience level, availability of digital products, and digital resilience and sustainability).
Mourtzis et al. (Citation2022) considers the metaverse as ‘a new era in Internet connectivity, characterized by interactivity, simulation, a decentralized environment, and persistent reality facilitated by the next evolution of the Internet (also known as Web 3.0) to combine the digital and physical worlds’ (Dolgui and Ivanov Citation2023). Lee and Kundu (Citation2022) elaborate on conceptual similarities of the metaverse and cyber-physical systems, in line with Panetto et al. (Citation2019).
Another relevant concept is digital twins. Digital twin in SCOM is understood as ‘a solution for better visualization and understanding of supply chains and an opportunity for further analysis, simulation, and optimization’ (van der Valk et al. Citation2022), ‘a combination of multiple enabling technologies, such as sensors, cloud computing, AI and advanced analytics, simulation, visualization, and augmented and virtual reality’ (Tozanli and Saénz Citation2022), and ‘computerized models that represent the network state for any given moment in time’ (Ivanov and Dolgui Citation2021).
According to Ivanov (Citation2024), a digital twin of a supply chain is
a virtual system comprised of (i) a digital visualization of a physical supply chain and its elements (e.g. firms, flows, and products) in a computer model, (ii) digital technologies providing data about the physical object (e.g. sensors, blockchain, clouds), and (iii) descriptive, predictive and prescriptive analytics for decision-making support.
through continuous data exchange, the digital twin always behaves synchronously with its real counterpart, and they influence each other. It mirrors both the state and the performance of the real system and can also send control signals back to the real system. Digital twins can be used for descriptive (e.g. performance visualisation), predictive (e.g. demand forecasting), and prescriptive (e.g. supply chain recovery simulation and optimisation) decision-making support. (Badakhshan and Ball Citation2023; Boyes and Watson Citation2022; Burgos and Ivanov Citation2021; Rolf et al. Citation2023)
3. IoB SCOM framework
Based on the analysis of the IoB and metaverse/digital twin SCOM frameworks, we can now propose an IoB SCOM framework (Figure ).
Figure 1. IoB SCOM framework.
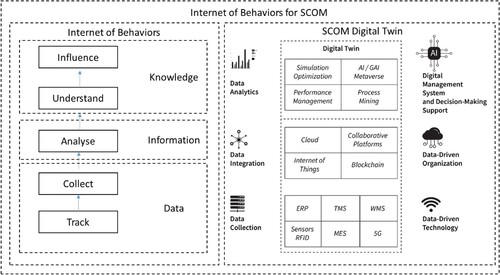
The IoB SCOM framework is based on the Sun et al. (Citation2023) IoB framework and metaverse/digital twin SCOM frameworks (Dolgui and Ivanov Citation2023; Ivanov Citation2023). An IoB part of the framework contains five major elements in line with Sun et al. (Citation2023), i.e. track and collect data, analyse information, and understand and influence information obtaining knowledge about behaviours. The SCOM digital twin part is based on a three-level architecture integrating insights from MacCarthy and Ivanov (Citation2022) and Ivanov (Citation2023; Citation2024). The first level is data collection, which is linked to the track&collect data layer of the IoB framework. The second level is data integration, which is essentially the analysis layer of the IoB framework. The third layer is data analytics and knowledge management.
The IoB SCOM framework encompasses three major dimensions, i.e. technology, organisation, and management. Data-driven technology is the first dimension. ERP, TMS (transportation management systems), WMS (warehouse management system), MES (manufacturing execution system), sensors, RFID, and 5G are the technologies for data tracking and collection in the supply chain part (Dolgui and Ivanov Citation2022). The IoB part technologies are related to customer and supplier data (i.e. e-commerce platforms for customer purchasing behaviour analysis).
Data-driven organisation is the second dimension. It takes the collected data to the level of analysis and producing information. Through data integration in the cloud, collaborative platforms, IoT, and Blockchain, the IoB applications analyse information that is used for understanding, predicting, and influencing behaviours of customers, supply chain entities, and suppliers.
At the third level of the digital management system and decision support, data analytics, simulation and optimisation, AI and generative AI, process mining, and performance visual analytics enable the transformation of information into knowledge. In summary, all these three levels constitute a digital twin that provides data and information for analysis of behaviours in the supply chain.
Figure also depicts that – in line with Dolgui and Ivanov (Citation2023), ‘physical and metaverse worlds are connected through digital technology such as [ … ] blockchain, artificial intelligence, the Internet of things, 5G/Edge computing, ERP (enterprise resource planning), big data analytics, and simulation’ (Brintrup et al. Citation2020; Cai, Choi, and Zhang Citation2021; Cui, Li, and Zhang Citation2022; Dolgui and Ivanov Citation2022). Smart devices and sensors in physical products along with different internet sources showing customer and supplier behaviours represent other data sources for the IoB SCOM framework. Using digital collaboration spaces for sourcing, production, and logistics coordination, behaviours of supply chain actors can be identified, analysed, and understood. Also, ‘digital stores, factories, and warehouses represent the supply chain in the metaverse, which can be digital replicas of physical stores, factories, and warehouses, or represent new, additional entities which do not exist in the physical supply chain’ (Dolgui and Ivanov Citation2023). These metaverse objects can be an additional component of the IoB in SCOM.
4. Implications of IoB on SCOM
In this section, we outline the implications of the IoB on SCOM. The scope of the IoB SCOM covers digital products, digital factories and warehouses, the digital supply chain, and digital ecosystems. The IoB for SCOM is not just a digital replica of a physical supply chain behaviours: the digital and physical supply chains evolve co-jointly and behaviours in the digital space are likely to be translated to those in the physical one. As such, the IoB enhances decision-making support and analytics applications for SCOM.
Assuming that people will have more and more activities in the digital world, we can expect new SCOM activities specifically dedicated to the IoB and leading to the appearance of novel SCOM processes and decision-making areas (Figure ).
Consider some examples. First, analysis of customer search and purchasing behaviours at e-commerce platforms can help firms to improve their demand forecasting and sales & operations planning (Jackson and Ivanov Citation2023). In addition, behavioural information can help in negotiations with both customers and suppliers. Combining technology and behavioural science, the IoB can help firms understand, analyze, and even influence customer’s (or potential customers) behaviours and attitudes toward their products and services. Second, in the area of transportation, IoB applications can help analyse driving behaviours, identify dangerous drivers, analyse eco-friendly driving behaviours, and even be used to driving behaviour-based insurance. Third, increased popularity of a product on an e-commerce product can be identified through customer purchasing behaviour resulting in increased demand and adjustment of inventory and production planning in the supply chain with increased record accuracy.
A timely recognition of the behavioural trends through data analytics can help supply chain managers to adapt and meet the changing demands in a timely manner. The behavioural data analysis can also be used for the introduction of new products into the market. They are also important for resilience and risk management. For example, some anomalies in supplier behaviour can be early signals of upcoming product shortages (Aldrighetti et al. Citation2024). Using data analytics, testing and forecasting customer and supplier behaviours can be analysed and used for demand, production, inventory, and purchasing planning. Furthermore, IoB applications can be of high relevance for human–robot collaboration (Sheu and Choi Citation2023). At the same time, ethical implications of IoB, particularly concerning data privacy and potential behavioural manipulations should be critically reviewed. An in-depth examination of these issues is crucial, considering the sensitive nature of behavioural data.
The methodology of SCOM can be enriched by the IoB through a combination of AI and digital technology with operations research and behavioural operations management (Jahani, Jain, and Ivanov Citation2023). The IoB can be used for the development of advanced decision-making support models through enhanced management capabilities such as visibility, computational power for data analytics, digital collaboration, and connectivity (Dolgui and Ivanov Citation2022). Empirical studies can help examine behavioural and ethical issues in the IoB along with technology adoption and technological barriers.
IoB can enhance supply chain mapping through more accurate, real-time data on customer and supplier behaviours (MacCarthy, Ahmed, and Demirel Citation2022). For example, collaborative e-commerce platforms and supplier portals can provide valuable information for supply chain managers ‘to review expected sales forecasts, projected production plans and possible supplier limitations that could affect manufacturing volume’ (Accenture Citation2022).
Resilience management can be enhanced by the IoB and especially behavioural anomaly detection for timely disruption recognition, impact prediction, and recovery actions (Ivanov Citation2021; Ivanov and Dolgui Citation2021; Ralston and Blackhurst Citation2020). As shown in (Badakhshan and Ball Citation2023; Ivanov Citation2023), digital twin-based simulation environments can help analyse different scenarios in the virtual world using the digital supply chain models. It can allow recognising bottlenecks and enforcing supply chains for stress situations (e.g. demand disruptions) through higher knowledge awareness. Sustainability management can also be improved through transparency about carbon emissions, visibility about the entire product life cycle, and associated environmental footprints.
A very important part of IoB in SCOM is related to human–AI collaboration. As shown in Ivanov (Citation2023), intelligent digital twins are based on the principles close to the IoB applications. Their mutual combinations can help develop decision-support systems that do not only replicate the logical rules of humans but also allow understanding the supply chains better through self-learning. In this setting, behavioural data analysis is an important element of building intelligent digital twins. Generative AI helps in prediction, optimisation, and anomaly/failure detection in SCOM (Jackson et al. Citation2024; Jackson, Saenz, and Ivanov Citation2024). As such, generative AI is an important contributor to the IoB in SCOM. Finally, privacy and cybersecurity issues are of utmost importance for the IoB in SCOM.
5. Conclusion
More and more behavioural data are available on the Internet and people, and behaviour becomes increasingly connected through the extension of the Internet of Things – the Internet of Behaviour (IoB). IoB is a network designed and used for replicating, understanding, predicting, and influencing human behaviour through data analytics. Despite the IoB concept resembles some ideas being developed in SCOM theory such as digital twins and metaverse SCOM, literature is silent about IoB in SCOM. This study aims to close this research gap and advance our understanding of how the IoB will impact the SCOM.
Based on a combination of the existing IoB frameworks with industrial Metaverse and digital supply chain twins, we proposed a framework for IoB in SCOM encompassing management, organisation, and technology dimensions. We conclude that IoB will impact a multitude of SCOM areas such as supply chain design, demand forecasting, sales and operations planning, distribution and transportation, inventory and production management, sourcing strategy and purchasing, vehicle routeing, supply chain resilience, and sustainability.
We offer a structured future research agenda pointing to new research questions and topics stemming from IoB-driven real-time visibility, data analytics, and remote control. The methodology of SCOM can also be enriched by the IoB through a combination of AI and digital technology with operations research and behavioural operations management.
IoB can help in decision-making support in SCOM enhancing the expertise of managers, their knowledge and skills through real-time data and information about customers, the firm’s supply chain, and supplier behaviours. IoB can help managers providing accurate real-time data, detecting behavioural anomalies and trends, and simulating potential outcomes of certain behavioural observations. In combination with digital twins, IoB in SCOM contributes to real-time, data-driven decision-making support and digitally driven management systems.
Further research can examine the impacts of IoB on SCOM theory and practices in particular areas identified in this paper. Increasing access to real-time behavioural data can have a profound effect on managerial decision-making. Behavioural and ethical aspects of data-driven decision-making and cognitive biases in human–AI interactions belong to the novel topics that will emerge when digital twins and IoB are integrated in SCOM. Further examination of technological challenges and barriers to IoB implementation in SCOM should be addressed in future. Obviously, multi-disciplinary research of computer science, software engineers, industrial engineers, and SCOM will be mandatory for the exploration and exploitation of the IoB in SCOM.
Acknowledgement
We cordially thank five anonymous reviewers whose comments helped us to improve the manuscript immensely.
Disclosure statement
No potential conflict of interest was reported by the author(s).
Data availability statement
Data related with this paper are available with authors and will be available upon reasonable request.
Additional information
Notes on contributors
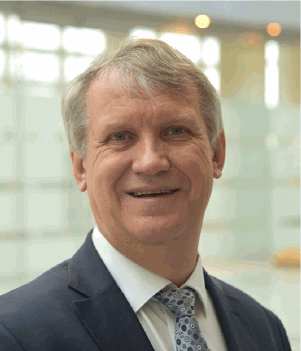
Alexandre Dolgui
Alexandre Dolgui is an IISE Fellow, a Distinguished Professor, and the Head of Automation, Production and Computer Sciences Department at the IMT Atlantique, France. His research focuses on manufacturing line design, production planning and supply chain optimisation. His main results are based on the exact mathematical programming methods and their intelligent coupling with heuristics and metaheuristics algorithms. He is the co-author of 5 books, the co-editor of 32 books or conference proceedings, the author of over 315 refereed journal papers, as well as over 450 papers in conference proceedings. He is an Area Editor of Computers & Industrial Engineering, and the past Associate Editor of the International Journal of Systems Science, IEEE Transactions on Industrial Informatics, and Omega. He is a Member of the Editorial Boards for 27 other journals, including the International Journal of Production Economics. He is an Active Fellow of the European Academy for Industrial Management, a Member of the Board of the International Foundation for Production Research, a former Chair of IFAC TC 5.2 Manufacturing Modelling for Management and Control (2011–2017, currently a vice-chair) and a Member of IFIP WG 5.7 Advances in Production Management Systems, IEEE System Council Analytics and Risk Technical Committee. He has received many scientific awards and recognitions for his outstanding achievements. He is the Editor-in-Chief of the International Journal of Production Research (IJPR).

Dmitry Ivanov
Dmitry Ivanov is a professor of supply chain and operations management at the Berlin School of Economics and Law. He serves at the school as an Academic Director of M.A. Global Supply Chain and Operations Management and is the director of the Digital-AI Supply Chain Lab. His publication list includes around 430 publications, including over 150 papers in international academic journals and leading textbooks Global Supply Chain and Operations Management, Introduction to Supply Chain Resilience, and Introduction to Supply Chain Analytics. His main research interests and results span resilience, viability and ripple effects in supply chains, risk analytics and digital twins. He is the author of the Viable Supply Chain Model and a founder of the ripple effect research in supply chains. He is the Principal investigator in several projects about supply chain digital twins and resilience, e.g. EU Horizon and DFG. He is a recipient of the IISE Transactions Best Paper Award 2021, Best Paper and Most Cited Paper Awards of IJPR (2018–2023), OMEGA Best Paper Award 2022, and Clarivate Highly Cited Researcher Award (2021, 2022). He is the Editor-in-Chief of the IJISM and is an associate editor of the IJPR, OMEGA and ANOR. He is Chairman of IFAC CC 5 ‘Cyber-Physical Manufacturing Enterprise.’
References
- Accenture. 2022. Accessed January 12, 2023. https://www.accenture.com/us-en/blogs/business-functions-blog/metaverse-supply-chain-networks.
- Aldrighetti, R., M. Calzavara, M. Martignago, I. Zennaro, D. Battini, and D. Ivanov. 2024. “A Methodological Framework for the Design of Efficient Resilience in Supply Networks.” International Journal of Production Research 62 (1-2): 271–290. https://doi.org/10.1080/00207543.2023.2285424.
- Badakhshan, E., and P. Ball. 2023. “Applying Digital Twins for Inventory and Cash Management in Supply Chains under Physical and Financial Disruptions.” International Journal of Production Research 61 (15): 5094–5116. https://doi.org/10.1080/00207543.2022.2093682.
- Berti, N., and S. Finco. 2022. “Digital Twin and Human Factors in Manufacturing and Logistics Systems: State of the Art and Future Research Directions.” IFAC-PapersOnLine 55 (10): 1893–1898. https://doi.org/10.1016/j.ifacol.2022.09.675.
- Boyes, H., and T. Watson. 2022. “Digital Twins: An Analysis Framework and Open Issues.” Computers in Industry 143:103763. https://doi.org/10.1016/j.compind.2022.103763.
- Brintrup, A., J. Pak, D. Ratiney, T. Pearce, P. Wichmann, P. Woodall, and D. McFarlane. 2020. “Supply Chain Data Analytics for Predicting Supplier Disruptions: A Case Study in Complex Asset Manufacturing.” International Journal of Production Research 58 (11): 3330–3341. https://doi.org/10.1080/00207543.2019.1685705.
- Burgos, D., and D. Ivanov. 2021. “Food Retail Supply Chain Resilience and the COVID-19 Pandemic: A Digital Twin-Based Impact Analysis and Improvement Directions.” Transportation Research Part E Logistics and Transportation Review 152:102412. https://doi.org/10.1016/j.tre.2021.102412.
- Cai, Y., T. M. Choi, and J. Zhang. 2021. “Platform Supported Supply Chain Operations in the Blockchain Era: Supply Contracting and Moral Hazards.” Decision Sciences 52 (4): 866–892. https://doi.org/10.1111/deci.12475.
- Cui, R., M. Li, and S. Zhang. 2022. “AI and Procurement.” Manufacturing & Service Operations Management 24 (2): 691–706. https://doi.org/10.1287/msom.2021.0989.
- Dolgui, A., and D. Ivanov. 2022. “5G in Digital Supply Chain and Operations Management: Fostering Flexibility, End-to-End Connectivity and Real-Time Visibility through Internet-of-Everything.” International Journal of Production Research 60 (2): 442–451. https://doi.org/10.1080/00207543.2021.2002969.
- Dolgui, A., and D. Ivanov. 2023. “Metaverse Supply Chain and Operations Management.” International Journal of Production Research 61 (23): 8179–8191. https://doi.org/10.1080/00207543.2023.2240900.
- Embarak, O. H. 2022. “Internet of Behaviour (IoB)-Based AI Models for Personalized Smart Education Systems.” Procedia Computer Science 203:103–110. https://doi.org/10.1016/j.procs.2022.07.015.
- Holzwarth, A., C. Staib, and D. Ivanov. 2022. “Building Viable Digital Business Ecosystems with Collaborative Supply Chain Platform SupplyOn.” In Supply Network Dynamics and Control, edited by A. Dolgui, D. Ivanov, and B. Sokolov, 187–210. Cham: Springer.
- Ivanov, D. 2021. “Digital Supply Chain Management and Technology to Enhance Resilience by Building and Using End-to-End Visibility during the COVID-19 Pandemic.” IEEE Transactions on Engineering Management. https://doi.org/10.1109/TEM.2021.3095193.
- Ivanov, D. 2023. “Intelligent Digital Twin (iDT) for Supply Chain Stress-Testing, Resilience, and Viability.” International Journal of Production Economics 263:108938. https://doi.org/10.1016/j.ijpe.2023.108938.
- Ivanov, D. 2024. “Conceptualisation of a 7-Element Digital Twin Framework in Supply Chain and Operations Management.” International Journal of Production Research 62 (6): 2220–2232. https://doi.org/10.1080/00207543.2023.2217291.
- Ivanov, D., and A. Dolgui. 2021. “A Digital Supply Chain Twin for Managing the Disruption Risks and Resilience in the Era of Industry 4.0.” Production Planning & Control 32 (9): 775–788. https://doi.org/10.1080/09537287.2020.1768450.
- Jackson, I., and D. Ivanov. 2023. “A Beautiful Shock? Exploring the Impact of Pandemic Shocks on the Accuracy of AI Forecasting in the Beauty Care Industry. ” Transportation Research Part E Logistics and Transportation Review 180 (3): 103360. https://doi.org/10.1016/j.tre.2023.103360.
- Jackson, I., D. Ivanov, A. Dolgui, and J. Namdar. 2024. “Generative Artificial Intelligence in Supply Chain and Operations Management: A Capability-Based Framework for Analysis and Implementation.” International Journal of Production Research, 1–26. https://doi.org/10.1080/00207543.2024.2309309.
- Jackson, I., M. Saenz, and D. Ivanov. 2024. “From Natural Language to Simulations: Applying AI to Automate Simulation Modelling of Logistics Systems.” International Journal of Production Research 62 (4): 1434–1457. https://doi.org/10.1080/00207543.2023.2276811.
- Jahani, H., R. Jain, and D. Ivanov. 2023. “Data Science and Big Data Analytics: A Systematic Review of Methodologies Used in the Supply Chain and Logistics Research.” Annals of Operations Research. https://doi.org/10.1007/s10479-023-05390-7.
- Kidd, C. 2019. What is the Internet of Behaviors? IoB Explained. Accessed January 7, 2024. https://www.bmc.com/blogs/iob-internet-of-behavior/.
- Lareo, X. 2023. Internet of Behaviours. Accessed January 7, 2024. https://edps.europa.eu/data-protection/technology-monitoring/techsonar/internet-behaviours_en.
- Lee, J., and P. Kundu. 2022. “Integrated Cyber-Physical Systems and Industrial Metaverse for Remote Manufacturing.” Manufacturing Letters 34:12–15. https://doi.org/10.1016/j.mfglet.2022.08.012.
- Li, D. C., M. Y.-C. Lin, and L.-D. Chou. 2022. “Macroscopic Big Data Analysis and Prediction of Driving Behavior with an Adaptive Fuzzy Recurrent Neural Network on the Internet of Vehicles.” IEEE Access 10 (47): 881–895.
- MacCarthy, B., W. Ahmed, and G. Demirel. 2022. “Mapping the Supply Chain: Why, What and How?” International Journal of Production Economics 250:108688. https://doi.org/10.1016/j.ijpe.2022.108688.
- MacCarthy, B., and D. Ivanov. 2022. “The Digital Supply Chain—Emergence, Concepts, Definitions, and Technologies.” In The Digital Supply Chain, edited by B. MacCarthy and D. Ivanov, 3–14. Amsterdam: Elsevier.
- Mourtzis, D., N. Panopoulos, J. Angelopoulos, B. Wang, and L. Wang. 2022. “Human Centric Platforms for Personalized Value Creation in Metaverse.” Journal of Manufacturing Systems 65:653–659. https://doi.org/10.1016/j.jmsy.2022.11.004.
- Nyman, G. 2012. Internet of Behaviors (IoB). Accessed January 7, 2024. https://gotepoem.wordpress.com/2012/03/.
- Panetto, H., B. Iung, D. Ivanov, G. Weichhart, and X. Wang. 2019. “Challenges for the Cyber-Physical Manufacturing Enterprises of the Future.” Annual Reviews in Control 47:200–213. https://doi.org/10.1016/j.arcontrol.2019.02.002.
- Ralston, P., and J. Blackhurst. 2020. “Industry 4.0 and Resilience in the Supply Chain: A Driver of Capability Enhancement or Capability Loss?” International Journal of Production Research 58 (16): 5006–5019. https://doi.org/10.1080/00207543.2020.1736724.
- Rolf, B., I. Jackson, M. Müller, S. Lang, T. Reggelin, and D. Ivanov. 2023. “A Review on Reinforcement Learning Algorithms and Applications in Supply Chain Management.” International Journal of Production Research 61 (20): 7151–7179. https://doi.org/10.1080/00207543.2022.2140221.
- Sheu, J. B., and T.-M. Choi. 2023. “Can We Work More Safely and Healthily with Robot Partners? A Human-Friendly Robot-Human Coordinated Order Fulfillment Scheme.” Production and Operations Management 32 (3): 794–812. https://doi.org/10.1111/poms.13899.
- Stary, C. 2020. “The Internet-of-Behavior as Organizational Transformation Space with Choreographic Intelligence.” In International Conference on Subject-Oriented Business Process Management, 113–132. Springer.
- Sun, J., W. Gan, H.-C. Chao, P. S. Yu, and W. Ding. 2023. “Internet of Behaviors: A Survey.” IEEE Internet of Things Journal 10 (13): 11117–11134. https://doi.org/10.1109/JIOT.2023.3247594.
- Tozanli, Ö., and M. E. Saénz. 2022. “Unlocking the Potential of Digital Twins in Supply Chains.” MIT Sloan Management Review, August 2022.
- van der Valk, H., G. Strobel, S. Winkelmann, J. Hunker, and M. Tomczyka. 2022. “Supply Chains in the Era of Digital Twins – A Review.” Procedia Computer Science 204:156–163. https://doi.org/10.1016/j.procs.2022.08.019.
- Yan, Y., C. Huang, Q. Wang, and B. Hu. 2020. “Data Mining of Customer Choice Behavior in Internet of Things within Relationship Network.” International Journal of Information Management 50:566–574. https://doi.org/10.1016/j.ijinfomgt.2018.11.013.
- Zhao, Q., G. Li, J. Cai, M. C. Zhou, and L. Feng. 2023. “A Tutorial on Internet of Behaviors: Concept, Architecture, Technology, Applications, and Challenges.” IEEE Communications Surveys & Tutorials 25 (2): 1227. https://doi.org/10.1109/COMST.2023.3246993.