Abstract
Digital twins in Industry 4.0 enhance lean supply chains by optimizing efficiency, reducing costs, and ensuring responsiveness to consumers. However, their relationship with lean supply chains remains understudied. We address this gap through a systematic literature review, analyzing 33 selected papers from 759 articles. Utilizing the supply-chain operations reference (SCOR) framework, we assess digital twins’ impact on supply chain processes and performances. Our findings indicate that digital twins are primarily used in plan, make, and delivery processes, with limited exploration in source and return processes. They enhance lean practices by improving information flow, eliminating waste, optimizing logistics, and enabling just-in-time production. However, top management commitment, supplier relationship management, and customer relationship management are understudied areas. We also recognize two additional areas where digital twins contribute: enhancing supply chain coordination, and bolstering supply chain resilience, particularly against disruptions such as COVID-19 and geopolitical events. Additionally, we propose a framework for digital twin-driven smart lean supply chain management and highlight the importance of future research in areas such as supply chain digital twins (SCDT) mapping, convergence, interaction, cognition and service. This study pioneers exploring the motivations, applications, and contributions of digital twins in lean supply chain management.
SUSTAINABLE DEVELOPMENT GOALS:
1. Introduction
Efficiently managing the supply chains is crucial for running modern business operations, and the adoption of lean principles plays a key role in driving operational improvement (Jasti and Kodali Citation2015a). Lean philosophies have been instrumental in increasing efficiency in the manufacturing industry over the past few decades, and the literature suggests that many companies have greatly benefitted from lean manufacturing practices. The lean concepts, initially introduced in Japan by Toyota in the 1950s (Womack, Jones, and Roos Citation1990) have evolved from manufacturing to cover the entire supply chain. The concept of lean supply chain management became popular from the 1990s when academic studies researched the application of lean principles to the entire supply chain beyond the factory floor. David Levy (Levy Citation1997) proposed that lean production principles can stabilise supply chains by reducing defects and engineering change orders, helping to mitigate the problems associated with global supply chains, such as longer lead times, expensive transportation costs, higher inventory levels, and delays in forecasting demands and resolving technical problems.
Lean supply chain management (SCM) aims to minimise waste and maximise supply chain efficiency, and reduce costs of manufacturing and supply by fostering collaboration among companies in the supply chain (Vitasek, Manrodt, and Abbott Citation2005). It is defined as a set of companies directly linked by upstream and downstream flows of products, services, information, and funds that collaborate to reduce cost and waste by efficiently meeting the needs of the customer (Vitasek, Manrodt, and Abbott Citation2005). Jasti and Kodali (Citation2015b) critically analyse the existing frameworks in lean supply chain management and provide a conceptual framework with 8 elements (e.g. elimination of waste, continuous improvement) that are integral part of an organisation. Present-day global supply chains are complex and face many challenges such as high inventory costs, poor collaboration between supply chain partners, and complex logistics networks. These challenges motivate companies to seek innovative solutions that leverage digital advancements in Industry 4.0 to enhance their supply chain operations. One such concept that holds promise for improving supply chain visibility and performance is the digital twin.
Digital twin, defined as a virtual representation of a physical object, process or system, enables real-time monitoring, analysis, and optimisation of supply chain operations (Tao, Cheng et al., Citation2018). The digital twin model was first presented by Grieves at a Society of Manufacturing Engineering conference in Michigan in October of 2002 (Grieves Citation2023). This concept deals with the creation of a virtual counterpart that faithfully represents its physical counterpart in real-time, enabling seamless convergence between the two realms. Over the years, the advancement of various technologies such as data acquisition, management and processing, information and communications, as well as simulation, has transformed the digital twin into a comprehensive concept encompassing digital representations of objects, processes, and systems, which are dynamically updated as their physical counterparts evolve (Jones et al. Citation2020; Liu, Fang, et al. Citation2021; Lu et al. Citation2020). By creating the digital replica of a physical asset or process, organisations can gain intelligence that enables responsiveness of the supply chains (Ivanov and Dolgui Citation2020). With the Covid-19 pandemic exposing the vulnerabilities of companies with complex supply chains, such as supply and demand fluctuations, unpredictable productivity levels and inventory placement problems, prominent scholars (Burgos and Ivanov Citation2021; Ivanov Citation2023; Tozanli and Saénz Citation2022) argue for the need for digital twins to map supply networks and ensuring visibility to handle such shocks. Seven major elements of a digital twin in the supply chain and operations management with scope, technology, people, organisation, modelling management and task have been identified in a recent study (Ivanov Citation2024). Moreover, a four-layered digital twin reference architecture has been introduced to structure digital twin perception, interaction, cognition, and application capabilities, which has been successfully adopted in the real-world manufacturing industry to support cost-efficient and scalable digital transformation (Guo et al. Citation2023).
We use the supply-chain operations reference model (SCOR) framework to assess the application of digital twins within the supply chain processes. The SCOR framework has emerged as a widely used tool to analyse and optimise supply chain processes and performance. SCOR, released by the Supply Chain Council (SCC) in 1996, is the first cross-industry framework that provides a standardised, cross-functional approach to analyse and optimise supply chain processes and performance (Huan, Sheoran, and Wang Citation2004). SCOR defines a set of major processes (plan, source, make, deliver, return and enable) aimed at managing materials, information, and financial resources to enhance the performance (reliability, responsiveness, agility, costs, and asset management efficiency) of the supply chain (Lockamy and McCormack Citation2004). The framework is further decomposed into lower levels (e.g. process categories level, process elements level and improvement tools/activities level) to describe, measure, and evaluate supply chains in support of strategic planning and continuous improvement (Lima-Junior and Carpinetti Citation2019). Due to its ability to provide a standardised approach to communication, analysis, and optimisation of supply chain processes and performance, SCOR has gained widespread adoption across various industries and organisations of all sizes, including manufacturing, retail, healthcare, and logistics (Zhou et al. Citation2011). Through the utilisation of SCOR, companies can effectively map out the current state of their supply chain processes, identify areas of inefficiency or waste, and leverage the framework for continuous improvement.
Although lean supply chain management and the SCOR framework are two different approaches to improve supply chain efficiency, in this paper we use them as complementary approaches to explain the applications of digital twins. By using SCOR, we present the definition and standardisation of supply chain processes to make it easier to identify where lean thinking can be applied. For example, in identifying and eliminating non-value-added activities in the processes.
1.1. Theoretical background
This paper draws upon three core concepts derived from existing studies: the SCOR framework, lean supply chain management (Jasti and Kodali Citation2015b) and the digital twin framework in supply chain management (Guo et al. Citation2023; Ivanov Citation2024) as presented in Figure . These concepts provide a strong theoretical foundation for understanding the integration and impact of digital twins in lean supply chain management. The intersection of the Venn diagram in Figure indicates our core research area.
Figure 1. Theoretical background and research scope. Notes: The illustration of theoretical background and research scope of this work.
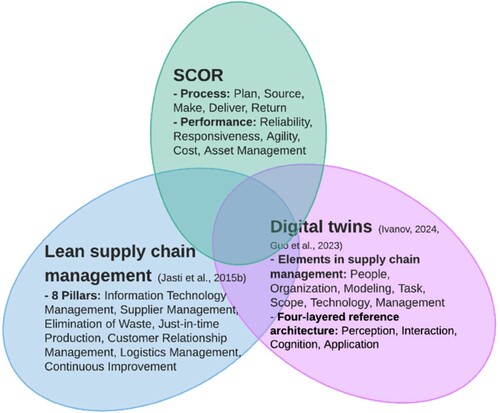
While the principles of lean supply chain management have been well-established and the potential benefits of emerging Industry 4.0 technologies such as digital twins in the context of lean SCM are evident, the literature on the specific roles and applications of digital twins is still scarce. To explore this gap, our paper aims to review the existing literature to provide insights into their motivations and applications. To better understand the context and challenges of digital twins for lean SCM, it is crucial to first study the applications of digital twins in the supply chains. While there are no recent literature reviews on digital twins for lean supply chain management, the table below summarises key studies in the overall supply chain context.
The recent literature on the role of digital twins in supply chains highlights several issues that motivate the need for a comprehensive literature review on lean supply chain management and the exploration of research directions. While many studies explore exciting concepts of smart supply chain management enabled by digital twins (Bhandal et al. Citation2022; Ivanov Citation2024; Wang et al. Citation2022), their specific roles in the context of lean are underexplored. Other literature streams explore potentials of digital twins in improving the performance and efficiency of supply chains (Guo et al. Citation2023; van der Valk et al. Citation2022), but focus on specific use cases without lean practices. Moreover, most of these studies do not explore the digital twin application in SCOR-based supply chain processes and performances. In light of these issues, there is a strong need for a comprehensive study on digital twins within lean supply chain practices to offer guidance for both academics and practitioners in the field to assess the readiness and detailed working of digital twin applications to provide the way forward.
1.2. Problem statement
Despite the transformative potential of digital twins in the context of Industry 4.0 and supply chain management, there are significant gaps in understanding their role and impact on the lean supply chain as mentioned above in Table . There are also significant knowledge gaps on how digital twins affect the performance of supply chains based on the SCOR framework and the future research directions in this area are not clear. Considering the limited literature specifically exploring the role of digital twins in lean SCM, this paper aims to answer the following questions:
RQ 1: What is the current state of the digital twin application in supply chain management?
RQ 2: What is the role of the digital twin in enhancing lean management and improving the SCOR-based supply chain performance?
RQ 3: What are the research directions and future research agenda for leveraging the digital twin in lean supply chain management?
Table 1. Summary of recent literature reviews on digital twins for supply chain management.
Section 2 describes the methodology used for the literature review and Section 3 presents the descriptive analysis of the literature review followed by a thematic analysis in Section 4. Section 5 discusses the findings to answer the research questions of the paper and presents the future research directions while proposing a novel framework for digital twin-enabled lean supply chain management. Finally, conclusions are drawn in Section 6.
2. Methodology
We use a systematic literature review to identify the current state of literature on the role of digital twins for lean supply chain management in the manufacturing industry. In this section, we describe the approach we took to identify the relevant studies and the protocols used and the data extraction process and screening for their eligibility. Our research design followed the widely accepted Preferred Reporting Items for Systematic Reviews and Meta-Analysis (PRISMA) (Moher et al. Citation2009) framework to manage various stages of the literature starting from identification of research question, searching, screening, and charting the data and synthesising for findings. The review process was designed to cover studies from both Industry 4.0 as well as operations management field.
2.1. Search strategy
We conducted the search using multiple electronic databases, including Scopus, Web of Science and ABI Inform. We also ran searches on Google Scholar to capture prominent studies in the selected field, while acknowledging the overlaps with the main databases. The search strategy includes titles and abstracts appropriate to the selected databases. We used search keywords such as ‘digital’, ‘supply chain’, ‘logistics’, ‘value chain’, and ‘twin’ to identify the articles.
2.2. Inclusion & exclusion criteria
Our inclusion criteria selected articles that are peer-reviewed and written in English language. Our Exclusion criteria omitted articles in languages other than English and those less than 8 pages in length. The decision of including papers longer than 8 pages was to ensure quality and depth of the selected studies and to review more substantial contributions on the digital twin applications and evaluations in lean. We noted from previous literature that restricting to only English was a common practice in systematic reviews and the majority of the journals indexed in the databases we considered are in English language, we did not include non-English language studies to avoid any potential translation errors and misinterpretations. We have also excluded articles with digital twin applications other than those in the manufacturing industry. For example, many studies we came across studied the digital twin for construction and mining problems, and they did not meet our inclusion criteria. Our study covers articles from the year 2019–2022 and Table below provides further information on the protocol and the search string.
Table 2. Summary of the search strategy.
2.3.Data Extraction and synthesis
Following the PRISMA framework, we created a systematic method to perform our data screening. Figure presents different steps involved in the process.
Figure 2. The PRISMA flow diagram. Notes: The illustration of PRISMA flow diagram for the literature review.
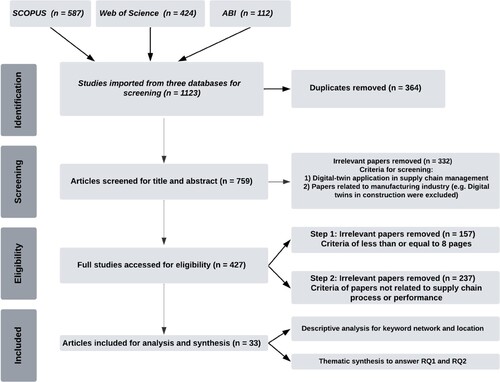
The different phases of the review process is as follows:
Identification phase: We imported a total of 1123 studies from three databases for screening and removed duplicates (n = 364), leaving us with 759 studies for further screening.
Screening phase: We screened articles on the basis of title and abstract and excluded 322 irrelevant papers that did not meet the criteria of digital twin’s application for operations management problems. This phase left us with 427 studies to be screened in the eligibility phase.
Eligibility phase: This phase involved two steps and only selected articles with full text access were deemed eligible. In Step 1, articles that were less than or equal to 8 pages in length (n = 157) were excluded. In Step 2, articles not related to the supply chain process or supply chain performance (n = 237) were removed. This left a total of 33 studies that met the eligibility criteria and we included them for final analysis.
We followed a four-eye screening process as all the co-authors independently evaluated the eligibility of selected articles to guarantee rigour and consistency. The data extraction and synthesis process aimed to analyse the findings from the included articles based on two approaches:
Descriptive analysis: A keyword analysis was performed to descriptively analyse the literature. The occurrence of keywords related to the digital twin in supply chain management was examined, providing insights into their frequency and distribution over time. Through this quantitative analysis of systematically counting and tracking the occurrence of keywords in the literature, we could visually present how prevalent and influential certain topics are within the field including the geographies that are active in these areas of research. This approach enables a data-driven understanding of trends and patterns contributing to a comprehensive overview of the field of investigation.
Thematic analysis: Through a qualitative approach to uncover and explore underlying themes, patterns, or categories within a dataset, we have analysed manually each of selected 33 papers and categorised them into distinct groups based on shared characteristics or themes. As an example, all the selected papers were grouped according to their application industry. We used Excel spreadsheets to extract relevant information and presented the findings using tables. Thematic analysis served to derive insights and answering research questions through in-depth exploration of the data.
We used Mendeley to manage the references and synthesised the information from individual articles. PRISMA protocol allowed the systematic reporting of the review process, ensuring that all essential elements were adequately addressed.
3. Descriptive analyses
Figure shows the number of published documents and their citations over years from the Web of Science database. It can be seen that the topic of the digital twin in the supply chain is relatively new with the first document published in 2019. The number of published documents and associated citations has experienced a rapid increase in recent years, indicating a growing attention to this topic.
Figure 3. The number of published documents and their citations over years. Notes: The statistical results of published documents and their citations from the year 2019–2022 collected from the Web of Science database.
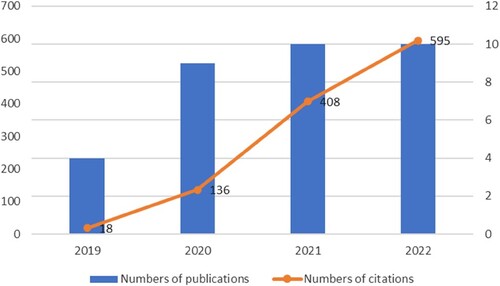
Figure shows the top fields of the published documents from the Web of Science database. The studies span across a range of research fields, with the top five fields, as per Web of Science categorisation, being engineering manufacturing, engineering industrial, operations research, management science, computer science interdisciplinary applications, and automation control systems, with 15, 12, 12, 7 and 4 publications, respectively.
Figure 4. The top fields of the published documents. Notes: The statistical results of the top fields of the published documents from the Web of Science database.
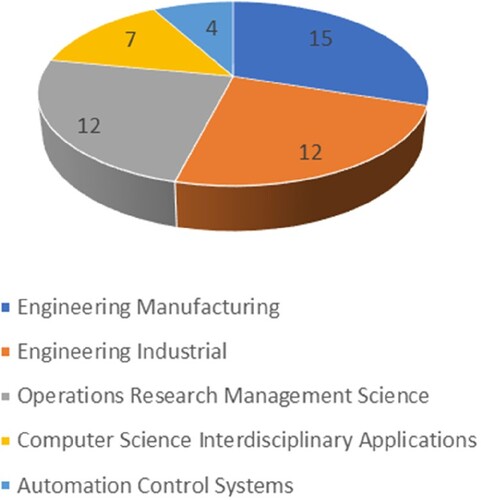
The keywords of the included articles have been collected and are presented as a co-occurrence network as depicted in Figure using VOSviewer software. In the visualisation of the keyword co-occurrence network, a link between two nodes denotes the co-occurrence of the associated keywords, with the thickness of a link is proportional to the number of articles in which the linked keywords co-occur. Additionally, the size of a node corresponds to the number of articles in which the associated keyword occurs. As shown in Figure , ‘digital twin’, ‘simulation’ and ‘design’, are the top three frequent keywords, occurring in 22, 12 and 11 articles, respectively.
Figure 5. Keyword co-occurrence network of the selected studies. Notes: The visualisation of the keyword co-occurrence network using VOSviewer software.
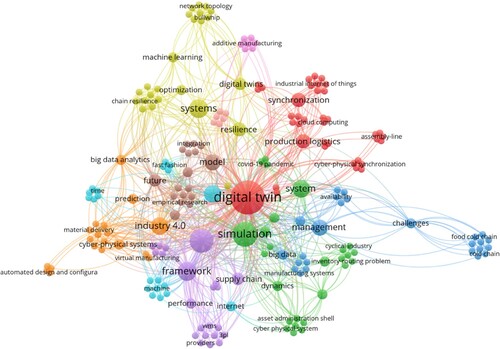
The descriptive analysis reveals that the topic of digital twins in supply chain management is attracting increasing attention from scholars, with both the number of research studies and associated citations experiencing a rapid increase from 2019. The topic draws interest from cross-disciplinary fields, including engineering, management science, operations research, economics and computer science. The bibliometric analysis indicates that current studies on digital twins in the supply chain are primarily associated with simulation and design.
4. Thematic analyses
4.1. The application of the digital twin in supply chain management: RQ1
With a total of 33 studies that met the eligibility criteria and we included them for the thematic analysis in this section. This section gives a summary of the application of the digital twin in the supply chain regarding the industry sector using SCOR-based major supply chain processes, digital twin application and development degree with 33 selected studies, please refer to Appendix Table A for details. Below, we provide the major summary of Appendix Table A:
Industry sector: According to the Global Industry Classification Standard (GICS), the concept of digital twin related to supply chain management is being exploited in various industries, such as 351020-Health Care Providers & Services (Baruffaldi, Accorsi, and Manzini Citation2019), 201010-Aerospace & Defense (Mandolla et al. Citation2019), 201060-Machinery (Guo, Li, Ling et al. Citation2021; Guo, Zhong et al. Citation2021; Liu, Cao et al. Citation2021; Toro, Seif, and Akhtar Citation2020; Wang et al. Citation2021; Wang and Wu Citation2020; Xia et al. Citation2022; Zhang et al. Citation2022), 201040-Electrical Equipment (Ciano et al. Citation2021), 352020-Pharmaceuticals (Marmolejo-Saucedo Citation2020), 251020-Automobiles (Park, Son, and Noh Citation2020; Park, Woo, and Choi Citation2020), 251010-Automobile Components (Wang, Zhang, and Zhong Citation2020), 151010-Chemicals (Pan et al. Citation2021; Zhang et al. Citation2020), 302020-Food Products (Burgos and Ivanov Citation2021; Shoji et al. Citation2021), 202010-Commercial Services & Supplies (Kombaya Touckia, Hamani, and Kermad Citation2022), 252030-Textiles, Apparel & Luxury Goods (dos Santos et al. Citation2021; Rahmanzadeh, Pishvaee, and Govindan Citation2023; Tang et al. Citation2022), 301010-Consumer Staples Distribution & Retail (Wang et al. Citation2022); 452020-Technology Hardware, Storage & Peripherals (Wu et al. Citation2022), 201020-Building Products (Zhao et al. Citation2022).
SCOR -based major supply chain processes: The selected studies were analysed for application of digital twins in the plan, source, make, deliver and return processes across the supply chain. Plan, make and delivery are the three major processes that the digital twin is being used and exploited in the supply chain, which has been reported in 27, 26 and 18 studies. While the application of digital twins in source and return processes is relatively less explored, only one published research has been reported on the implementation of digital twins in return processes.
Digital twin application and development degree: With digital mapping of the physical entity in a digital twin supply chain, the digital twin enhanced supply chain connectivity, optimisation, and collaboration across multi-echelons and multiple stakeholders by combining simulation and optimisation algorithms. In terms of development degree, nearly half have reported successful implementation in an authentic industry setting or have been utilised to address real-world problems through qualitative and quantitative studies. The remaining studies have reported their work as proof-of-concept demonstrations, or have been implemented solely in simulation or laboratory environments.
4.2. The application of the digital twin in enhancing lean management and improving supply chain performance: RQ2
Table provides a comprehensive overview of the application of the digital twin in enhancing lean management and improving supply chain performance from the aspects of major pillars of lean supply chain management (Jasti and Kodali Citation2015b), SCOR-based supply chain performance metrics (Lockamy and McCormack Citation2004) and method for 33 selected studies. Below, we provide the details of each column:
Major pillars of lean supply chain management: The analysis of the selected studies presents the application of the digital twin to enhance major pillars of lean supply chain management. Eight pillars include top management commitment, supplier relationship management, customer relationship management, information technology, JIT production, elimination of waste, continuous improvement and logistics management, identified from 30 lean supply chain management frameworks in the literature (Jasti and Kodali Citation2015b). The utilisation of digital twins has been identified as an emerging information technology with significant potential in the realm of supply chain management, as indicated by all 33 papers reviewed. Among these papers, 21 specifically highlight how the integration of digital twin-enabled visibility has contributed to the enhancement of waste elimination within supply chain management practices. Furthermore, the improvement of logistics management through the implementation of digital twins is mentioned in 16 papers, emphasising its efficacy in optimising logistical operations. The pillar of just-in-time (JIT) production is discussed in 7 papers with a particular focus on employing digital twins for facilitating JIT production within supply chains. Additionally, 6 papers emphasise the potential of digital twins in enabling continuous improvement initiatives, thereby promoting ongoing enhancements in supply chain performance. In examining the application of digital twins in supply chain management, attention was also given to three key pillars: top management commitment, supplier relationship management, and customer relationship management. Although relatively limited in number (2, 1, and 2 papers respectively), these studies shed light on the importance of these pillars and their interactions within the context of digital twin applications.
SCOR-based supply chain performance improvement: The analysis of the examined studies reveals significant improvements in SCOR-based supply chain performance metrics, including reliability, responsiveness, agility, cost, and asset management efficiency (Lockamy and McCormack Citation2004). 20 of the studies highlighted a clear enhancement in asset management efficiency, including improved utilisation of materials, machines, and manpower, which can be attributed to the implementation of digital twins. The second most frequently reported improvement was observed in the domain of reliability, as seen in 12 studies. These studies emphasised improvements in order fulfillment, on-time delivery, and maintaining products in perfect condition. Furthermore, the studies consistently demonstrated that the application of digital twins yielded considerable improvements in responsiveness, as presented in 11 studies. This enhancement included reduced lead time and cycle time, contributing to a more agile and responsive supply chain. Additionally, the positive impact of digital twins on agility, including factors such as adaptability, resilience, and risk management, was underscored by 8 studies. The potential for cost optimisation through the utilisation of digital twins was acknowledged in 6 studies, highlighting the benefits of enhanced cost management practices associated with lean. Therefore, organisations can identify cost-saving opportunities and optimise their supply chain costs effectively by leveraging the digital twin capabilities.
Method: After careful examination of the 33 studies, we observe a pattern that all of them exhibit a combination of the digital twin and simulation methodologies. However, it is crucial to note that only 13 studies out of this pool explicitly presented the utilisation of both the digital twin and simulation techniques in conjunction with optimisation strategies, with a focus on the application of digital twins for supply chain management. This is not surprising, as the concept of the digital twin was initially treated as an extension of simulation with a high-fidelity digital counterpart in manufacturing and logistics studies (Agalianos et al. Citation2020; Boschert and Rosen Citation2016; Glaessgen and Stargel Citation2012; Schluse and Rossmann Citation2016). With a higher level of data integration between the physical and digital counterparts, interaction and optimisation can be achieved by combining digital twins with big data, operations research and artificial intelligence techniques (Kritzinger et al. Citation2018; Qi et al. Citation2021; Rathore et al. Citation2021).
Table 3. The overview of the application of the digital twin in enhancing lean practices and improving supply chain performance.
After careful analysis of the role of the digital twin in lean supply chain management, in addition to boosting the eight pillars in lean supply chain management, the studies also indicate the roles of digital twins in enhancing (1) supply chain integration, coordination, and collaboration (Guo, Zhong et al. Citation2021; Leung, Lee, and Ouyang Citation2022; Marmolejo-Saucedo Citation2020; Pan et al. Citation2020; Pan et al. Citation2021; Park, Woo, and Choi Citation2020; Rahmanzadeh, Pishvaee, and Govindan Citation2023; Tang et al. Citation2022; Wang et al. Citation2022; Xia et al. Citation2022; Zhang et al. Citation2020; Zhang et al. Citation2022), and (2) supply chain flexibility, resilience, and adaptability (Badakhshan and Ball Citation2022; Burgos and Ivanov Citation2021; Cavalcante et al. Citation2019; Guo, Zhong et al. Citation2021; Kombaya Touckia, Hamani, and Kermad Citation2022; Marmolejo-Saucedo Citation2020; Wang et al. Citation2022). Despite these two pillars not being explicitly explored in the eight pillars framework (Jasti and Kodali Citation2015b), these topics have received increasing attention in the lean supply chain field in the context of industry 4.0 (Manavalan and Jayakrishna Citation2019; Núñez-Merino et al. Citation2020; Qader et al. Citation2022; Reyes, Mula, and Díaz-Madroñero Citation2023). Particularly, the recent COVID-19 pandemic and geopolitical influences (e.g. US–China tensions and Russia–Ukraine war) threatened supply chains domestically and globally with demand volatility, supply shortages, sourcing limitations, transportation and logistics constraints, and shift to e-commerce, reshaping global supply chain management practices and emphasising the importance of coordination, collaboration, resilience, and adaptability in preparing for future disruptions (Handfield, Graham, and Burns Citation2020; Ivanov Citation2021, Citation2022; Praharsi et al. Citation2021; Pujawan and Bah Citation2022; Remko Citation2020).
5. Discussion
In this section, we address the research questions posed in this paper and provide a summary of our findings.
5.1. Key findings from the review
5.1.1. RQ1: what is the current state of the digital twin application in supply chain management?
Digital twin applications in supply chain management have been explored across various industry sectors, including pharmaceuticals, health care providers & services, chemicals, machinery, electrical equipment, automobiles, automobile components, food products, commercial services & supplies, textiles, apparel & luxury goods, consumer staples distribution & retail, technology hardware, storage & peripherals, and building products. The concept of the digital twin is being utilised in different supply chain processes based on SCOR framework, with a focus on plan, make, and delivery processes, as reported in numerous studies. However, the application of digital twins in source and return processes has received relatively less exploration, with only a limited number of studies reporting on it. By digitally mapping physical entities, digital twins enhance supply chain connectivity, optimisation, and collaboration among multiple stakeholders and echelons. This enables them to address real-world problems and achieve successful implementation in authentic industry settings in many cases. However, some studies remain at the proof-of-concept stage or have been implemented solely in simulation or laboratory environments.
5.1.2. RQ2: what is the role of the digital twin in enhancing lean management and improving supply chain performance?
The reviewed papers collectively affirm the significance of the digital twin in enhancing lean management and improving supply chain performance. We analysed them through eight major pillars of lean supply chain management, and the digital twin has been found to contribute to all of these pillars. It enables the streamlined flow of information, waste elimination, optimises logistics management, facilitates JIT production, and promotes continuous improvement. Limited but significant studies emphasise the importance of digital twins in enhancing top management commitment, supplier relationship management, and customer relationship management for lean supply chain management. Besides, the digital twin has been found to go beyond enhancing the recognised eight pillars of lean supply chain management. It also contributes to two additional areas: supply chain integration, coordination, and collaboration, as well as supply chain flexibility, resilience, and adaptability. These aspects have gained attention in the context of Industry 4.0, especially due to disruptions like the COVID-19 pandemic and geopolitical influences (Handfield, Graham, and Burns Citation2020; Ivanov Citation2021, Citation2022; Manavalan and Jayakrishna Citation2019; Núñez-Merino et al. Citation2020; Praharsi et al. Citation2021; Pujawan and Bah Citation2022; Qader et al. Citation2022; Remko Citation2020; Reyes, Mula, and Díaz-Madroñero Citation2023). The digital twin enables seamless information flow and interaction among supply chain partners while empowering organisations to effectively respond to changes and disruptions in the business environment. Furthermore, the digital twin has been instrumental in improving SCOR-based supply chain performance metrics. It enhances asset management efficiency, reliability, responsiveness, agility, and cost optimisation. By utilising digital twins, organisations can improve their utilisation of resources, fulfil orders on time, reduce lead time, and manage risks more effectively.
5.2. Future research directions
5.2.1. RQ3: what are the research directions and future research agenda for leveraging the digital twin in lean supply chain management?
The findings of this review have important implications for future research. Despite the extensive discussion on digital twin applications in supply chain management across various industries, there is still no unified understanding of the composition and subsequent usage of digital twins for lean supply chain management. Recently, prominent scholars have identified seven major elements of a digital twin in the supply chain and operations management: scope, technology, people, organisation, modelling, management, and task, which provides a conceptual framework for further structuring the knowledge on the utilisation of digital twins in supply chain management (Ivanov Citation2024). Moreover, a four-layered digital twin reference architecture has been introduced to structure digital twin perception, interaction, cognition, and application capabilities, which has been successfully adopted in the real-world manufacturing industry to support cost-efficient and scalable digital transformation (Guo et al. Citation2023). These works lay the groundwork by providing both a theoretical foundation and practical guidance for leveraging the potential of digital twins within the context of lean supply chain management in the future. Building upon the seven-element supply chain and operations management (SCOM) digital twin framework and the four-layered digital twin reference architecture outlined in recent literature (Guo et al. Citation2023; Ivanov Citation2024), as illustrated in Figure , this section introduces an open and innovative framework designed for digital twin-driven smart lean supply chain management, by integrating digital twin capabilities into lean supply chain management.
Figure 6. A framework of digital twin-driven smart lean supply chain management (developed on the basis of the four-layered digital twin reference architecture (Guo et al. Citation2023) and the seven-element digital twin framework (Ivanov Citation2024)). Notes: A framework of digital twin-driven smart lean supply chain management that incorporates seven major elements of scope, technology, organisation, people, modelling management and task into four layers including the supply chain digital twins (SCDT) mapping & convergence layer, SCDT interaction layer, SCDT cognition layer and SCDT service layer.
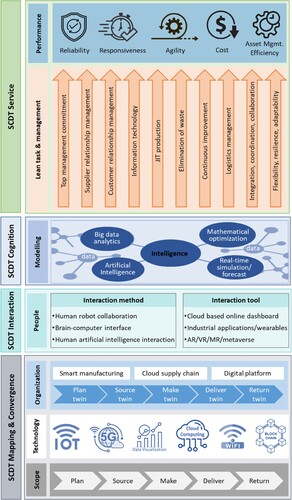
The proposed framework incorporates seven major elements of scope, technology, organisation, people, modelling, management, and task into four layers: the supply chain digital twins (SCDT) mapping & convergence layer, SCDT interaction layer, SCDT cognition layer, and SCDT service layer. Based on the four-layered digital twin reference architecture, the SCDT mapping & convergence layer acts as the technical infrastructure of the framework, focusing on real-time perception and mapping of a physical supply chain into a digital twin while maintaining convergence between them. The SCDT interaction layer supports real-time interaction between the SCDT and its physical counterpart. The SCDT cognition layer acts as the brain of the framework by offering digital tiwn-driven insights from prescriptive and predictive analytics. The SCDT service layer augments the capabilities of lean task & management for enhancing supply chain performance metrics such as reliability, responsiveness, agility, cost, and asset management efficiency.
Below are the main challenges and opportunities from the aspects of SCDT mapping & convergence, SCDT interaction, SCDT cognition, and SCDT service emerging from this framework:
SCDT mapping & convergence: This layer encompasses major elements of scope, technology, organisation, focusing on real-time mapping and convergence between the SCDT and its equivalent physical supply chain, as well as how it innovates business and organisational models. The scope of the supply chain processes covers plan, source, make, deliver, and return from the aspects of the SCOR framework. With the rapid application and integration of emerging technologies, such as the Internet of Things (IoT), data visualisation, big data analytics, cloud computing, 5G, WIFI, blockchain, and many other related technologies, it is possible to build an SCDT that includes a physical supply chain in the real space, a virtual supply chain with appropriate sets of information in the virtual space, and connections of data and information that connect and synchronise them at an appropriate frequency. With static data that maps the structure and properties of the physical supply chain and real-time dynamic data that synchronise the behaviours, states and processes between physical and digital spaces, real-time mapping and convergence between the SCDT and its equivalent physical supply chain can be achieved (Barykin et al. Citation2020; Ivanov Citation2023; Ivanov and Dolgui Citation2020; Wang et al. Citation2022). This facilitates the realisation of various conceptual constructs, namely, the plan digital twin, source digital twin, make digital twin, delivery digital twin, and return digital twin, thereby engendering new business and organisational paradigms, such as smart manufacturing (Tao, Qi et al., Citation2018), cloud supply chain (Ivanov, Dolgui, and Sokolov Citation2022), and digital platforms (De Reuver, Sørensen, and Basole Citation2018). However, digital twins in supply chains lag behind their counterparts in production. Important issues related to SCDT mapping & convergence are for example: How to efficiently map a physical supply chain into an appropriate SCDT while maintaining high fidelity? How to achieve interoperability among individual digital twins (e.g. plan, source, make, deliver, and return digital twins) to ensure seamless communication and data exchange? How to ensure convergence and synchronisation between the SCDT and its equivalent physical supply chain at an appropriate frequency? How to effectively handle the legal considerations, security concerns, and privacy issues that arise when multiple stakeholders collaboratively create and use an SCDT?
SCDT interaction: This layer pertains to the interaction between an SCDT within the virtual realm and its corresponding physical supply chain in the real-world domain. SCDTs are developed and used by people, and concurrently, they transform the manner in which supply chain managers and on-site operators engage with related resources and activities with enhanced visibility. This facilitates interaction and collaboration among people, machines, and materials by providing a real-time digital navigation environment. For example, production operations could be organised in an agile, resilient, and cost-efficient manner with spatiotemporal synchronisation of men, machines, and materials by utilising cyber-physical visibility and traceability (Guo et al. Citation2021). However, this requires innovative interaction methods like human-robot collaboration (Hjorth and Chrysostomou Citation2022), brain-computer interface (Liu, Wang, and Gao Citation2024), and human-artificial intelligence interface (Adobor, Awudu, and Norbis Citation2023). In addition, new interaction tools such as cloud-based dashboards, AR/VR/MR/Metaverse, industrial applications and industrial wearables are needed to enhance real-time communication, interaction and collaboration (Dolgui and Ivanov Citation2023; Ivanov, Dolgui, and Sokolov Citation2022; Queiroz et al. Citation2023).
SCDT cognition: This layer acts as the brain of the framework by offering digital twin-driven insights from prescriptive and predictive analytics. Real-time mapping and interaction between the SCDT and its physical counterpart provide a transparent and real-time view of supply chain operations, such as order progress, supplier performance, inventory levels, production and logistics monitoring, quality control, and workforce management. By feeding the digital twin-driven historical and real-time data to sophisticated modelling technologies such as big data analytics, artificial intelligence (AI), mathematical optimisation, and real-time simulation and forecasting, stakeholders actively engaged in the supply chain can access and scrutinise contemporaneous information pertaining to their assets, operational processes, and performance metrics (Bhandal et al. Citation2022; van der Valk et al. Citation2022; Wang et al. Citation2022). This empowers stakeholders within the supply chain to make informed decisions, react promptly to dynamic shifts, and enhance processes through the invaluable support of intelligent decision-making. Nonetheless, relying solely on data-driven modelling approaches may inadvertently neglect the qualitative and contextual insights crucial for comprehending intricate supply chain dynamics (Schniederjans, Curado, and Khalajhedayati Citation2020). In this context, the integration of domain expertise and data-driven insights stands as a pivotal focus for future research in the realm of digital supply chain management (Jahani, Jain, and Ivanov Citation2023). Additionally, the integration of Generative AI and supply chain management is a promising area that deserves further investigation (Castañé et al. Citation2023; Jackson, Jesus Saenz, and Ivanov Citation2024; Wamba et al. Citation2023).
SCDT service: This layer pertains to the SCDT service, which augments the capabilities of lean task & management for enhancing supply chain performance metrics such as reliability, responsiveness, agility, cost, and asset management efficiency. The introduction of SCDT effectively expands the operational scope from the physical realm into the digital domain with the digital twin-driven insights, which extends two additional pillars: supply chain integration, coordination, and collaboration, as well as supply chain flexibility, resilience, and adaptability identified from reviewing the application of digital twins in supply chain management. Together with the other eight pillars identified in the literature (Jasti and Kodali Citation2015b), this expansion results in ten pillars of lean supply chain task and management. Lean supply chain initiatives are essential in the digital era to leverage the capabilities of digital technologies, drive efficiency, enhance agility, and deliver customer value. This has been evidenced even in turbulent times with the Covid-19 pandemic and other recent disruptions (Choi et al. Citation2023). However, in practice, supply chain-focused lean initiatives are often undertaken without a clear conceptualisation and a systematic thinking process, which results in the variety of lean supply chain management definitions, multiple conceptual frameworks, and an absence of standardised constructs found in the current literature (Cusumano et al. Citation2021; Jasti and Kodali Citation2015b). It becomes crucial to have a clear understanding of the concepts underlying digital twins’ disruptions and lean supply chain management if we want to offer efficient lean task and management services in the digital era (Cifone et al. Citation2021; Naranjo, Menor, and Johnson Citation2023). Future research in lean digital supply chain management should focus on a generic conceptual framework, standardised constructs and methods that integrate systematic lean thinking and emerging technologies to address the evolving needs and complexities of future supply chains.
6. Conclusions
In this paper, we investigated the role of digital twins in lean supply chain management to provide insights into their applications and potential contributions. We performed a systematic literature review and selected 33 papers for final inclusion in descriptive and thematic analysis. Our descriptive analysis shows that the studies of digital twins in supply chain are mainly associated with simulation and design at the current stage, and the topic is attracting cross-disciplinary studies from engineering, management science, operations research, economics and computer science, with the number of research studies and associated citations experiencing a rapid increase since 2019. We explored digital twin applications in supply chain management across various industry sectors. By digitally mapping physical entities, the digital twin enhance supply chain connectivity, optimisation, and collaboration among multiple stakeholders. By utilising digital twins, organisations can improve their utilisation of resources, fulfil orders on time, reduce lead time, and manage risks more effectively. Our analysis revealed that the digital twin has the potential to enhance lean management and improve supply chain performance. Below, we summarise our key findings and contributions:
Classification of digital twin applications: Based on the SCOR framework, we classified the digital twin applications into various supply chain processes, including plan, make, delivery, and return. Digital twins are being utilised across all supply chain processes, with a focus on plan, make, and delivery processes, as reported in numerous studies. However, the application of digital twins in source and return processes has received relatively less exploration, with only a limited number of studies reported. We sorted the studies by their application sectors and observed that the machinery industry had the highest prevalence of digital twin applications. Additionally, half of the studies claimed their digital twin applications in real-world settings, while the rest were either proof-of-concept demonstrations or limited to simulations or laboratories.
Digital twins improve supply chain performance: Our analysis reveals that the implementation of digital twins has resulted in significant advancements in SCOR-based supply chain performance. The studies highlighted improved asset management efficiency, including improved utilisation of materials, machines, and manpower. Reliability improvements were also observed, particularly in areas such as order fulfillment, on-time delivery, and product preservation. The positive impact of digital twins on responsiveness was evident through reduced lead time and cycle time, contributing to a more agile and responsive supply chain. Additionally, digital twins were found to enhance agility, adaptability, resilience, and risk management. The potential for cost optimisation was acknowledged, with organisations able to identify cost-saving opportunities and optimise their supply chain costs. By leveraging the capabilities of digital twins, organisations can enhance their operational performance and improve customer satisfaction.
Digital twins enhance lean supply chain management: Following the widely recognised lean supply chain management framework, the digital twin enables efficient information flow, waste elimination, optimised logistics management, JIT production, and continuous improvement. Notably, limited but significant research emphasises its impact on top management commitment, supplier relationship management, and customer relationship management. Furthermore, the digital twin extends its influence to two additional areas: enhancing supply chain integration, coordination, and collaboration, and bolstering supply chain flexibility and resilience, particularly in response to recent disruptions such as the COVID-19 pandemic and geopolitical influences. These findings underscore the transformative potential of the digital twin in advancing lean supply chain practices, promoting operational efficiency, and fostering strategic collaboration in a rapidly changing business landscape.
Framework and research directions: We also proposed a digital twin-driven framework to achieve smart lean supply chain management (Figure ). This framework is built upon the recent seven-element digital twin framework and the four-layered digital twin reference architecture, which serves as a guide to improve supply chain performances of reliability, responsiveness, agility, cost, and asset management efficiency by integrating lean supply chain management and SCDT. Additionally, we outlined future research directions from four research clusters including the SCDT mapping & convergence, SCDT interaction, SCDT cognition and SCDT service.
While we ensured the reliability and inclusivity of our research, our study is constrained by its exclusive reliance on peer-reviewed material sourced from Scopus, Web of Science, and ABI Inform databases. Although these repositories offer extensive and up-to-date scholarly works, it is possible that our review might have overlooked studies published in alternative outlets and industry reports. To address this limitation, future research should consider incorporating non-academic publications and a wider range of academic articles, thereby broadening the scope and depth of the literature review. Additionally, upcoming research will delve into an examination of how various technologies contributing to the digital twin concept can offer advantages to the different stages of the supply chain. Furthermore, the exploration on using the proposed open framework of digital twin-driven smart supply chain management to enhance supply chain viability in the context of disruptions such as the COVID-19 pandemic and geopolitical influences deserves further investigation (Ivanov et al. Citation2023).
Disclosure statement
No potential conflict of interest was reported by the author(s).
Data availability statement
The authors confirm that the data supporting the findings of this study are available within the article [and/or] its supplementary materials.
Additional information
Funding
Notes on contributors
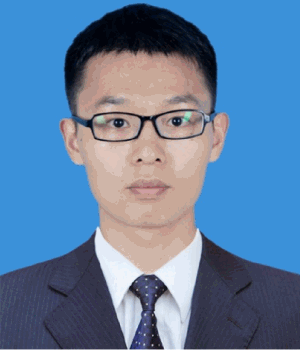
Daqiang Guo
Dr Daqiang Guo is currently an Assistant Professor with the Smart Manufacturing Thrust, Systems Hub, The Hong Kong University of Science and Technology (Guangzhou). Before this, he worked as a Postdoctoral Research Associate at the University of Cambridge. He holds a Ph.D. degree in Industrial and Manufacturing Systems Engineering, and B.Eng. and M.Eng. degrees in Mechanical Engineering. His research focuses on design and optimisation of manufacturing systems for economic, social, and environmental performance improvement through industrial digital technologies interventions. Dr Guo has published 30 research articles in journals/conferences including one ESI Highly Cited Paper and one 2023 IJPR Top Trending Article. He is a recipient of the Best Paper Award of the 32nd International Conference on Flexible Automation and Intelligent Manufacturing (FAIM2023), and the UKRI Made Smarter Network+ (‘InterAct’) Early Career Researcher Fellowships.
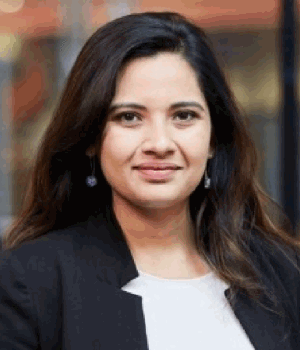
Soujanya Mantravadi
Dr Soujanya Mantravadi is a researcher at Cambridge University's Institute for Manufacturing. She has a bachelor's degree in mechanical engineering and a PhD in industrial engineering and information systems. Before PhD, she worked in industry and consulting and has work experience in six countries, such as Denmark and Switzerland. She is interested in supply chain research, designing industrial systems that leverage modern artificial intelligence solutions and the Internet of Things, and drawing from stakeholder inputs. Currently, her work focuses on food supply chains and digital interventions in the Epidemiology field as part of a transdisciplinary project. She is also involved in teaching activities at Cambridge Judge Business School, and IFM Engage industry training programsme.
References
- Adobor, H., I. Awudu, and M. Norbis. 2023. “Integrating Artificial Intelligence into Supply Chain Management: Promise, Challenges and Guidelines.” International Journal of Logistics Systems and Management 44 (4): 458–488. https://doi.org/10.1504/IJLSM.2023.130782
- Agalianos, K., S. T. Ponis, E. Aretoulaki, G. Plakas, and O. Efthymiou. 2020. “Discrete Event Simulation and Digital Twins: Review and Challenges for Logistics.” Procedia Manufacturing 51: 1636–1641. https://doi.org/10.1016/j.promfg.2020.10.228
- Badakhshan, E., and P. Ball. 2022. “Applying Digital Twins for Inventory and Cash Management in Supply Chains Under Physical and Financial Disruptions.” International Journal of Production Research 61 (15): 5094–5116. https://doi.org/10.1080/00207543.2022.2093682
- Bai, Y., J.-B. You, and I.-K. Lee. 2021. “Design and Optimization of Smart Factory Control System Based on Digital Twin System Model.” Mathematical Problems in Engineering 2021: 1–16.
- Baruffaldi, G., R. Accorsi, and R. Manzini. 2019. “Warehouse Management System Customization and Information Availability in 3PL Companies: A Decision-Support Tool.” Industrial Management & Data Systems 119 (2): 251–273. https://doi.org/10.1108/IMDS-01-2018-0033
- Barykin, S. Y., A. A. Bochkarev, O. V. Kalinina, and V. K. Yadykin. 2020. “Concept for a Supply Chain Digital Twin.” International Journal of Mathematical, Engineering and Management Sciences 5 (6): 1498. https://doi.org/10.33889/IJMEMS.2020.5.6.111
- Bhandal, R., R. Meriton, R. E. Kavanagh, and A. Brown. 2022. “The Application of Digital Twin Technology in Operations and Supply Chain Management: A Bibliometric Review.” Supply Chain Management: An International Journal 27 (2): 182–206. https://doi.org/10.1108/SCM-01-2021-0053
- Boschert, S., and R. Rosen. 2016. “Digital Twin—the Simulation Aspect.” In Mechatronic Futures: Challenges and Solutions for Mechatronic Systems and Their Designers. Edited by Peter Hehenberg, 59–74. Springer.
- Burgos, D., and D. Ivanov. 2021. “Food Retail Supply Chain Resilience and the COVID-19 Pandemic: A Digital Twin-Based Impact Analysis and Improvement Directions.” Transportation Research Part E: Logistics and Transportation Review 152: 102412. https://doi.org/10.1016/j.tre.2021.102412
- Castañé, G., A. Dolgui, N. Kousi, B. Meyers, S. Thevenin, E. Vyhmeister, and P. O. Östberg. 2023. “The ASSISTANT Project: Ai for High Level Decisions in Manufacturing.” International Journal of Production Research 61 (7): 2288–2306.
- Cavalcante, I. M., E. M. Frazzon, F. A. Forcellini, and D. Ivanov. 2019. “A Supervised Machine Learning Approach to Data-Driven Simulation of Resilient Supplier Selection in Digital Manufacturing.” International Journal of Information Management 49: 86–97. https://doi.org/10.1016/j.ijinfomgt.2019.03.004
- Choi, T. Y., T. H. Netland, N. Sanders, M. S. Sodhi, and S. Wagner. 2023. “Just-in-Time for Supply Chains in Turbulent Times.” Production and Operations Management 32 (7): 2331–2340. https://doi.org/10.1111/poms.13979
- Ciano, M. P., P. Dallasega, G. Orzes, and T. Rossi. 2021. “One-to-one Relationships Between Industry 4.0 Technologies and Lean Production Techniques: A Multiple Case Study.” International Journal of Production Research 59 (5): 1386–1410. https://doi.org/10.1080/00207543.2020.1821119
- Cifone, F. D., K. Hoberg, M. Holweg, and A. P. Staudacher. 2021. “‘Lean 4.0’: How Can Digital Technologies Support Lean Practices?” International Journal of Production Economics 241: 108258. https://doi.org/10.1016/j.ijpe.2021.108258
- Cusumano, M. A., M. Holweg, J. Howell, T. Netland, R. Shah, J. Shook, P. Ward, et al. 2021. “Commentaries on ‘The Lenses of Lean’.” Journal of Operations Management 67 (5): 627–639. https://doi.org/10.1002/joom.1138
- De Reuver, M., C. Sørensen, and R. C. Basole. 2018. “The Digital Platform: A Research Agenda.” Journal of Information Technology 33 (2): 124–135. https://doi.org/10.1057/s41265-016-0033-3
- Dolgui, A., and D. Ivanov. 2023. “Metaverse Supply Chain and Operations Management.” International Journal of Production Research 61 (23): 8179–8191. https://doi.org/10.1080/00207543.2023.2240900
- dos Santos, C. H., G. T. Gabriel, J. V. S. do Amaral, J. A. B. Montevechi, and J. A. de Queiroz. 2021. “Decision-making in a Fast Fashion Company in the Industry 4.0 Era: A Digital Twin Proposal to Support Operational Planning.” The International Journal of Advanced Manufacturing Technology 116 (5-6): 1653–1666. https://doi.org/10.1007/s00170-021-07543-z
- Glaessgen, E., and D. Stargel. 2012. “The Digital Twin Paradigm for Future NASA and US Air Force Vehicles.” 53rd AIAA/ASME/ASCE/AHS/ASC Structures, Structural Dynamics and Materials Conference 20th AIAA/ASME/AHS Adaptive Structures Conference 14th AIAA, 1818.
- Grieves, M. W. 2023. “Digital Twins: Past, Present, and Future.” In The Digital Twin. Edited by Noel Crespi, Adam T. Drobot, and Roberto Minerva, 97–121. Cham: Springer International Publishing.
- Guo, D., M. Li, S. Ling, R. Y. Zhong, Y. Rong, and G. Q. Huang. 2021. “Synchronization-oriented Reconfiguration of FPAI Under Graduation Intelligent Manufacturing System in the COVID-19 Pandemic and Beyond.” Journal of Manufacturing Systems 60: 893–902.
- Guo, D., M. Li, Z. Lyu, K. Kang, W. Wu, R. Y. Zhong, and G. Q. Huang. 2021. “Synchroperation in Industry 4.0 Manufacturing.” International Journal of Production Economics 238: 108171. https://doi.org/10.1016/j.ijpe.2021.108171
- Guo, D., T. Zhang, Y. Rong, G. Q. Huang, and J. S. Srai. 2023. “Lightweight Digital Twin as a Service (LDTaaS): A Cost-Efficient Digital Transformation Approach for Manufacturing SMEs.” In Low-Cost Digital Solutions for Industrial Automation (LoDiSA 2023) (Vol. 2023, 28–33), September. IET.
- Guo, D., R. Y. Zhong, Y. Rong, and G. G. Q. Huang. 2021. “Synchronization of Shop-Floor Logistics and Manufacturing Under IIoT and Digital Twin-Enabled Graduation Intelligent Manufacturing System.” IEEE Transactions on Cybernetics 53 (3): 2005–2016. https://doi.org/10.1109/TCYB.2021.3108546
- Handfield, R. B., G. Graham, and L. Burns. 2020. “Corona Virus, Tariffs, Trade Wars and Supply Chain Evolutionary Design.” International Journal of Operations & Production Management 40 (10): 1649–1660. https://doi.org/10.1108/IJOPM-03-2020-0171
- Hauge, J. B., M. Zafarzadeh, Y. Jeong, Y. Li, W. A. Khilji, C. Larsen, and M. Wiktorsson. 2021. “Digital Twin Testbed and Practical Applications in Production Logistics with Real-Time Location Data.” International Journal of Industrial Engineering and Management 12 (2): 129. https://doi.org/10.24867/IJIEM-2021-2-282
- Hjorth, S., and D. Chrysostomou. 2022. “Human–Robot Collaboration in Industrial Environments: A Literature Review on non-Destructive Disassembly.” Robotics and Computer-Integrated Manufacturing 73: 102208. https://doi.org/10.1016/j.rcim.2021.102208
- Huan, S. H., S. K. Sheoran, and G. Wang. 2004. “A Review and Analysis of Supply Chain Operations Reference (SCOR) Model.” Supply Chain Management: An International Journal 9 (1): 23–29. https://doi.org/10.1108/13598540410517557
- Ivanov, D. 2021. “Lean Resilience: AURA (Active Usage of Resilience Assets) Framework for Post-COVID-19 Supply Chain Management.” The International Journal of Logistics Management 33 (4): 1196–1217. https://doi.org/10.1108/IJLM-11-2020-0448
- Ivanov, D. 2022. “Viable Supply Chain Model: Integrating Agility, Resilience and Sustainability Perspectives—Lessons from and Thinking Beyond the COVID-19 Pandemic.” Annals of Operations Research 319 (1): 1411–1431. https://doi.org/10.1007/s10479-020-03640-6
- Ivanov, D. 2023. “Intelligent Digital Twin (iDT) for Supply Chain Stress-Testing, Resilience, and Viability.” International Journal of Production Economics 263: 108938. https://doi.org/10.1016/j.ijpe.2023.108938.
- Ivanov, D. 2024. “Conceptualisation of a 7-Element Digital Twin Framework in Supply Chain and Operations Management.” International Journal of Production Research 62 (6): 2220–2232. https://doi.org/10.1080/00207543.2023.2217291
- Ivanov, D., and A. Dolgui. 2020. “A Digital Supply Chain Twin for Managing the Disruption Risks and Resilience in the era of Industry 4.0.” Production Planning & Control 32 (9): 775–788. https://doi.org/10.1080/09537287.2020.1768450
- Ivanov, D., A. Dolgui, J. V. Blackhurst, and T. M. Choi. 2023. “Toward Supply Chain Viability Theory: From Lessons Learned Through COVID-19 Pandemic to Viable Ecosystems.” International Journal of Production Research 61 (8): 2402–2415. https://doi.org/10.1080/00207543.2023.2177049
- Ivanov, D., A. Dolgui, and B. Sokolov. 2022. “Cloud Supply Chain: Integrating Industry 4.0 and Digital Platforms in the “Supply Chain-as-a-Service”.” Transportation Research Part E: Logistics and Transportation Review 160: 102676. https://doi.org/10.1016/j.tre.2022.102676
- Jackson, I., M. Jesus Saenz, and D. Ivanov. 2024. “From Natural Language to Simulations: Applying AI to Automate Simulation Modelling of Logistics Systems.” International Journal of Production Research 62 (4): 1434–1457. https://doi.org/10.1080/00207543.2023.2276811
- Jahani, H., R. Jain, and D. Ivanov. 2023. “Data Science and big Data Analytics: A Systematic Review of Methodologies Used in the Supply Chain and Logistics Research.” Annals of Operations Research 1–58. https://doi.org/10.1007/s10479-023-05390-7
- Jasti, N. V. K., and R. Kodali. 2015a. “Lean Production: Literature Review and Trends.” International Journal of Production Research 53 (3): 867–885. https://doi.org/10.1080/00207543.2014.937508
- Jasti, N. V. K., and R. Kodali. 2015b. “A Critical Review of Lean Supply Chain Management Frameworks: Proposed Framework.” Production Planning & Control 26 (13): 1051–1068. https://doi.org/10.1080/09537287.2015.1004563
- Jones, D., C. Snider, A. Nassehi, J. Yon, and B. Hicks. 2020. “Characterising the Digital Twin: A Systematic Literature Review.” CIRP Journal of Manufacturing Science and Technology 29: 36–52. https://doi.org/10.1016/j.cirpj.2020.02.002
- Kombaya Touckia, J., N. Hamani, and L. Kermad. 2022. “Digital Twin Framework for Reconfigurable Manufacturing Systems (RMSs): Design and Simulation.” The International Journal of Advanced Manufacturing Technology 120 (7-8): 5431–5450. https://doi.org/10.1007/s00170-022-09118-y
- Kritzinger, W., M. Karner, G. Traar, J. Henjes, and W. Sihn. 2018. “Digital Twin in Manufacturing: A Categorical Literature Review and Classification.” IFAC-PapersOnline 51 (11): 1016–1022. https://doi.org/10.1016/j.ifacol.2018.08.474
- Leung, E. K. H., C. K. H. Lee, and Z. Ouyang. 2022. “From Traditional Warehouses to Physical Internet Hubs: A Digital Twin-Based Inbound Synchronization Framework for PI-Order Management.” International Journal of Production Economics 244: 108353. https://doi.org/10.1016/j.ijpe.2021.108353
- Levy, D. L. 1997. Lean Production in an International Supply Chain, 94–102. Boston: CiteSeerX.
- Lima-Junior, F. R., and L. C. R. Carpinetti. 2019. “Predicting Supply Chain Performance Based on SCOR® Metrics and Multilayer Perceptron Neural Networks.” International Journal of Production Economics 212: 19–38. https://doi.org/10.1016/j.ijpe.2019.02.001
- Liu, J., X. Cao, H. Zhou, L. Li, X. Liu, P. Zhao, and J. Dong. 2021. “A Digital Twin-Driven Approach Towards Traceability and Dynamic Control for Processing Quality.” Advanced Engineering Informatics 50: 101395. https://doi.org/10.1016/j.aei.2021.101395
- Liu, M., S. Fang, H. Dong, and C. Xu. 2021. “Review of Digital Twin About Concepts, Technologies, and Industrial Applications.” Journal of Manufacturing Systems 58: 346–361. https://doi.org/10.1016/j.jmsy.2020.06.017
- Liu, S., L. Wang, and R. X. Gao. 2024. “Cognitive Neuroscience and Robotics: Advancements and Future Research Directions.” Robotics and Computer-Integrated Manufacturing 85: 102610. https://doi.org/10.1016/j.rcim.2023.102610
- Lockamy, A., and K. McCormack. 2004. “Linking SCOR Planning Practices to Supply Chain Performance: An Exploratory Study.” International Journal of Operations & Production Management 24 (12): 1192–1218. https://doi.org/10.1108/01443570410569010
- Lu, Y., C. Liu, I. Kevin, K. Wang, H. Huang, and X. Xu. 2020. “Digital Twin-Driven Smart Manufacturing: Connotation, Reference Model, Applications and Research Issues.” Robotics and Computer-Integrated Manufacturing 61: 101837. https://doi.org/10.1016/j.rcim.2019.101837
- Manavalan, E., and K. Jayakrishna. 2019. “A Review of Internet of Things (IoT) Embedded Sustainable Supply Chain for Industry 4.0 Requirements.” Computers & Industrial Engineering 127: 925–953. https://doi.org/10.1016/j.cie.2018.11.030
- Mandolla, C., A. M. Petruzzelli, G. Percoco, and A. Urbinati. 2019. “Building a Digital Twin for Additive Manufacturing Through the Exploitation of Blockchain: A Case Analysis of the Aircraft Industry.” Computers in Industry 109: 134–152. https://doi.org/10.1016/j.compind.2019.04.011
- Mantravadi, S., J. S. Srai, and C. Møller. 2023. “Application of MES/MOM for Industry 4.0 Supply Chains: A Cross-Case Analysis.” Computers in Industry 148: 103907. https://doi.org/10.1016/j.compind.2023.103907
- Marmolejo-Saucedo, J. A. 2020. “Design and Development of Digital Twins: A Case Study in Supply Chains.” Mobile Networks and Applications 25 (6): 2141–2160. https://doi.org/10.1007/s11036-020-01557-9
- Moher, D., A. Liberati, J. Tetzlaff, D. G. Altman, and The PRISMA Group*. 2009. “Preferred Reporting Items for Systematic Reviews and Meta-Analyses: The PRISMA Statement.” Annals of Internal Medicine, American College of Physicians 151 (4): 264–269. https://doi.org/10.7326/0003-4819-151-4-200908180-00135
- Naranjo, F., L. J. Menor, and P. F. Johnson. 2023. “Lean Supply Chain Management: A Contextual Contingent Reconceptualization and Delphi Method Study.” International Journal of Operations & Production Management 43 (9): 1456–1480. https://doi.org/10.1108/IJOPM-07-2022-0436
- Núñez-Merino, M., J. M. Maqueira-Marín, J. Moyano-Fuentes, and P. J. Martínez-Jurado. 2020. “Information and Digital Technologies of Industry 4.0 and Lean Supply Chain Management: A Systematic Literature Review.” International Journal of Production Research 58 (16): 5034–5061. https://doi.org/10.1080/00207543.2020.1743896
- Pan, Y. H., T. Qu, N. Q. Wu, M. Khalgui, and G. Q. Huang. 2021. “Digital Twin Based Real-Time Production Logistics Synchronization System in a Multi-Level Computing Architecture.” Journal of Manufacturing Systems 58: 246–260. https://doi.org/10.1016/j.jmsy.2020.10.015
- Pan, Y. H., N. Q. Wu, T. Qu, P. Z. Li, K. Zhang, and H. F. Guo. 2020. “Digital-twin-driven Production Logistics Synchronization System for Vehicle Routing Problems with Pick-up and Delivery in Industrial Park.” International Journal of Computer Integrated Manufacturing 34 (7–8): 814–828.
- Park, K. T., Y. H. Son, and S. D. Noh. 2020. “The Architectural Framework of a Cyber Physical Logistics System for Digital-Twin-Based Supply Chain Control.” International Journal of Production Research 59 (19): 5721–5742. https://doi.org/10.1080/00207543.2020.1788738
- Park, Y., J. Woo, and S. Choi. 2020. “A Cloud-Based Digital Twin Manufacturing System Based on an Interoperable Data Schema for Smart Manufacturing.” International Journal of Computer Integrated Manufacturing 33 (12): 1259–1276. https://doi.org/10.1080/0951192X.2020.1815850
- Praharsi, Y., M. A. Jami’in, G. Suhardjito, and H. M. Wee. 2021. “The Application of Lean Six Sigma and Supply Chain Resilience in Maritime Industry During the era of COVID-19.” International Journal of Lean Six Sigma 12 (4): 800–834. https://doi.org/10.1108/IJLSS-11-2020-0196
- Pujawan, I. N., and A. U. Bah. 2022. “Supply Chains Under COVID-19 Disruptions: Literature Review and Research Agenda.” Supply Chain Forum: An International Journal 23: 81–95.
- Qader, G., M. Junaid, Q. Abbas, and M. S. Mubarik. 2022. “Industry 4.0 Enables Supply Chain Resilience and Supply Chain Performance.” Technological Forecasting and Social Change 185: 122026. https://doi.org/10.1016/j.techfore.2022.122026
- Qi, Q., F. Tao, T. Hu, N. Anwer, A. Liu, Y. Wei, L. Wang, et al. 2021. “Enabling Technologies and Tools for Digital Twin.” Journal of Manufacturing Systems 58: 3–21. https://doi.org/10.1016/j.jmsy.2019.10.001
- Queiroz, M. M., S. Fosso Wamba, S. C. F. Pereira, and C. J. Chiappetta Jabbour. 2023. “The Metaverse as a Breakthrough for Operations and Supply Chain Management: Implications and Call for Action.” International Journal of Operations & Production Management 43 (10): 1539–1553. https://doi.org/10.1108/IJOPM-01-2023-0006
- Rahmanzadeh, S., M. S. Pishvaee, and K. Govindan. 2023. “Emergence of Open Supply Chain Management: The Role of Open Innovation in the Future Smart Industry Using Digital Twin Network.” Annals of Operations Research 329 (1): 979–1007.
- Rathore, M. M., S. A. Shah, D. Shukla, E. Bentafat, and S. Bakiras. 2021. “The Role of ai, Machine Learning, and big Data in Digital Twinning: A Systematic Literature Review, Challenges, and Opportunities.” IEEE Access 9: 32030–32052. https://doi.org/10.1109/ACCESS.2021.3060863
- Remko, V. H. 2020. “Research Opportunities for a More Resilient Post-COVID-19 Supply Chain–Closing the gap Between Research Findings and Industry Practice.” International Journal of Operations & Production Management 40 (4): 341–355. https://doi.org/10.1108/IJOPM-03-2020-0165
- Reyes, J., J. Mula, and M. Díaz-Madroñero. 2023. “Development of a Conceptual Model for Lean Supply Chain Planning in Industry 4.0: Multidimensional Analysis for Operations Management.” Production Planning & Control 34 (12): 1209–1224. https://doi.org/10.1080/09537287.2021.1993373
- Schluse, M., and J. Rossmann. 2016. “From Simulation to Experimentable Digital Twins: Simulation-based Development and Operation of Complex Technical Systems.” 2016 IEEE International Symposium on Systems Engineering (ISSE), 1–6.
- Schniederjans, D. G., C. Curado, and M. Khalajhedayati. 2020. “Supply Chain Digitisation Trends: An Integration of Knowledge Management.” International Journal of Production Economics 220: 107439. https://doi.org/10.1016/j.ijpe.2019.07.012
- Shoji, K., S. Schudel, D. Onwude, C. Shrivastava, and T. Defraeye. 2021. ““Mapping the Postharvest Life of Imported Fruits from Packhouse to Retail Stores Using Physics-Based Digital Twins.” Resources, Conservation and Recycling 176: 105914. https://doi.org/10.1016/j.resconrec.2021.105914
- Tang, Y.-M., G. T. S. Ho, Y.-Y. Lau, and S.-Y. Tsui. 2022. “Integrated Smart Warehouse and Manufacturing Management with Demand Forecasting in Small-Scale Cyclical Industries.” Machines, MDPI 10 (6): 472. https://doi.org/10.3390/machines10060472
- Tao, F., J. Cheng, Q. Qi, M. Zhang, H. Zhang, and F. Sui. 2018. “Digital Twin-Driven Product Design, Manufacturing and Service with Big Data.” The International Journal of Advanced Manufacturing Technology 94 (9-12): 3563–3576. https://doi.org/10.1007/s00170-017-0233-1
- Tao, F., Q. Qi, A. Liu, and A. Kusiak. 2018. “Data-driven Smart Manufacturing.” Journal of Manufacturing Systems 48: 157–169. https://doi.org/10.1016/j.jmsy.2018.01.006
- Toro, C., A. Seif, and H. Akhtar. 2020. “Modeling and Connecting Asset Administrative Shells for Mini Factories.” Cybernetics and Systems 51 (2): 232–245. https://doi.org/10.1080/01969722.2019.1705554
- Tozanli, Ö, and M. J. Saénz. 2022. “Unlocking the Potential of Digital Twins in Supply Chains.” MIT Sloan Management Review 63 (4): 1–4.
- van der Valk, H., G. Strobel, S. Winkelmann, J. Hunker, and M. Tomczyk. 2022. “Supply Chains in the Era of Digital Twins–A Review.” Procedia Computer Science 204: 156–163. https://doi.org/10.1016/j.procs.2022.08.019
- Vitasek, K. L., K. B. Manrodt, and J. Abbott. 2005. “What Makes a Lean Supply Chain?” Supply Chain Management Review 9 (7): 39–45.
- Wamba, S. F., M. M. Queiroz, C. J. C. Jabbour, and C. V. Shi. 2023. “Are Both Generative AI and ChatGPT Game Changers for 21st-Century Operations and Supply Chain Excellence?” International Journal of Production Economics 265: 109015. https://doi.org/10.1016/j.ijpe.2023.109015
- Wang, L., T. Deng, Z.-J. M. Shen, H. Hu, and Y. Qi. 2022. “Digital Twin-Driven Smart Supply Chain.” Frontiers of Engineering Management 9 (1): 56–70. https://doi.org/10.1007/s42524-021-0186-9
- Wang, X., Y. Wang, F. Tao, and A. Liu. 2021. “New Paradigm of Data-Driven Smart Customisation Through Digital Twin.” Journal of Manufacturing Systems 58: 270–280. https://doi.org/10.1016/j.jmsy.2020.07.023
- Wang, Y., and Z. Wu. 2020. ““Digital Twin-Based Production Scheduling System for Heavy Truck Frame Shop”, Proceedings of the Institution of Mechanical Engineers.” Part C: Journal of Mechanical Engineering Science 236 (4): 1931–1942. https://doi.org/10.1177/0954406220913306
- Wang, W., Y. Zhang, and R. Y. Zhong. 2020. “A Proactive Material Handling Method for CPS Enabled Shop-Floor.” Robotics and Computer-Integrated Manufacturing 61: 101849. https://doi.org/10.1016/j.rcim.2019.101849
- Womack, J. P., D. T. Jones, and D. Roos. 1990. The Machine That Changed the World (Vol. 323 No. 1, pp. 273–287. New York: Rawson Associates.
- Wu, W., Z. Zhao, L. Shen, X. T. R. Kong, D. Guo, R. Y. Zhong, and G. Q. Huang. 2022. “Just Trolley: Implementation of Industrial IoT and Digital Twin-Enabled Spatial-Temporal Traceability and Visibility for Finished Goods Logistics.” Advanced Engineering Informatics 52: 101571. https://doi.org/10.1016/j.aei.2022.101571
- Xia, L., J. Lu, H. Zhang, M. Xu, and Z. Li. 2022. “Construction and Application of Smart Factory Digital Twin System Based on DTME.” The International Journal of Advanced Manufacturing Technology 120 (5-6): 4159–4178. https://doi.org/10.1007/s00170-022-08971-1
- Zhang, L., L. Feng, J. Wang, and K.-Y. Lin. 2022. “Integration of Design, Manufacturing, and Service Based on Digital Twin to Realize Intelligent Manufacturing.” Machines, MDPI 10 (4): 275. https://doi.org/10.3390/machines10040275
- Zhang, K., T. Qu, D. Zhou, H. Jiang, Y. Lin, P. Li, H. Guo, et al. 2020. “Digital Twin-Based Opti-State Control Method for a Synchronized Production Operation System.” Robotics and Computer-Integrated Manufacturing 63: 101892. https://doi.org/10.1016/j.rcim.2019.101892
- Zhao, Z., M. Zhang, J. Chen, T. Qu, and G. Q. Huang. 2022. “Digital Twin-Enabled Dynamic Spatial-Temporal Knowledge Graph for Production Logistics Resource Allocation.” Computers & Industrial Engineering 171: 108454.
- Zhou, H., W. C. Benton Jr, D. A. Schilling, and G. W. Milligan. 2011. “Supply Chain Integration and the SCOR Model.” Journal of Business Logistics 32 (4): 332–344. https://doi.org/10.1111/j.0000-0000.2011.01029.x
Appendix
Table A1. The summary of the application of the digital twin in supply chain management.