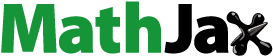
Abstract
Drones and Unmanned Aerial Vehicles (UAVs) have evolved into advanced instruments with diverse applications within the Logistics and Supply Chain (LSC) sector. Our study uncovers a significant disparity highlighted through an examination of recent review papers, indicating a deficiency in a thorough examination of the applications of drones/UAVs across the entirety of supply chains. Due to the abundance of publications in this field, a fresh analytical approach is required. Employing a novel text mining approach, qualitative assessments, and temporal trend analysis, we examine a dataset encompassing 5364 papers from Scopus spanning the years 1978 to 2023. By employing the Latent Dirichlet Allocation (LDA) model, we identify ten distinct research topics within the domain. Our findings suggest four promising future research directions: (1) fostering sustainable SCs through innovative drone-based solutions, (2) harnessing emerging technologies to achieve global scalability within drone-based SCs, (3) exploring the applications of drone-based supply chains in pandemic control efforts, and (4) integrating drone-based logistics with multimodal transportation for efficient traffic management. These directions provide valuable insights on the integration of drone-based transportation within existing and future supply chains, suggesting potential pathways for advancing production and logistics systems research.
Sustainable Development Goals:
1. Introduction
In the current landscape of logistics and supply chain management (SCM), profound technological advancements are notably marked by the distinguished use of drones and unmanned aerial vehicles (UAVs). The concept of drones, however, has a historical lineage dating back to the invention of the first powered aircraft by the Wright brothers. The first military-style drone was deployed during the Vietnam War (Hildmann and Kovacs Citation2019). In the past decade, pioneering companies such as Amazon and Domino's have emerged as trailblazers in the practical application of drones for product delivery. Subsequently, we have witnessed the rapid proliferation of these advanced technologies in various sectors in a relatively short timeframe. Recent research conducted in Europe, as reported by the UK's National Aeronautical Centre, indicates that 42% of carriers are actively considering the integration of drones into their operations. Consequently, it is projected that the near future will witness the creation of approximately 100,000 jobs directly related to the drone industry (Indeed Citation2023).
Despite the expansive growth in drone technology applications within SCM, the challenge of efficiently integrating the ever-expanding volume of research remains. The traditional review methods categorise articles through manual coding or bibliometric analysis without full-text evaluation, failing to comprehensively capture the nuanced advancements of applications of drones. This limitation highlights the necessity for an innovative supervised approach. Therefore, our study introduces a machine learning-based topic modelling method to systematically analyse and synthesise the extensive body of literature. This innovative approach facilitates uncovering hidden topics and emerging trends in drone application within SCM, derived from large-scale textual data. Thus, this study reveals some new research themes and future directions that complement and extend the insights of existing reviews.
Due to the specialised focus of this research, it is essential to define the main terms used in this paper clearly. These definitions, outlined in Appendix 1, will serve as the foundational basis for our discourse. The development of drones is closely tied to various human needs spanning across multiple domains, including military, medical, artistic, delivery services, warehousing, mining, agriculture, and more. Drones have the potential to bring about a transformative shift in transportation by enhancing the speed of deliveries and simultaneously reducing associated costs (Bhatt, Pourmand, and Sikka Citation2018; Lagkas et al. Citation2018). In addition to the advantageous applications of drones, it is imperative to acknowledge that there exist noteworthy obstacles and challenges, particularly in the context of logistics and supply chain (LSC). In this study, our primary focus revolves around the exploration of these applications, the modelling approaches employed, with a particular emphasis on operational research methodologies, and the substantial impediments and challenges encountered when utilising drones within the LSC domain.
UAVs are commonly categorised based on their aerodynamic design and flying principles. Recent advances have highlighted that UAVs with a heavier mass primarily depend on generating propulsive thrust to attain and maintain flight in the air. Moreover, there exist various types of UAVs or drones, each tailored to specific purposes and applications. In particular, two main types of UAVs that are widely recognised are rotor-based and wing-based UAVs (Ahmed et al. Citation2022; Singhal, Bansod, and Mathew Citation2018).
Rotor-based UAVs, also known as multirotor UAVs, employ multiple rotors and propellers attached to the aircraft to generate the necessary thrust for vertical lift-off. These rotors create upward forces that enable the UAV to ascend and maintain flight. Multirotor unmanned aerial vehicles possess the capability of vertical takeoff and landing (VTOL) and can hover in place, a capability not shared by fixed-wing aircraft (Floreano and Wood Citation2015). Their ability to hover in place and maintain speed makes them highly suitable for surveillance purposes and monitoring applications (Singhal, Bansod, and Mathew Citation2018). However, a drawback of multirotor UAVs is their limited endurance and speed, as they require more power consumption to counteract gravity and remain airborne. Multirotor UAVs are categorised based on their size into different classifications, including micro or mini-UAVs, tactical UAVs, strategic UAVs, and special-task UAVs (Israr et al. Citation2022).
Wing-based drones rely on the aerodynamic properties of their wings to generate lift and enable upward flight. The wings of these UAVs play a vital role in generating the necessary aerodynamic forces that lift the aircraft into the air (Ahmed et al. Citation2022). These specific types of UAVs provide exceptional capabilities for long-range missions, allowing them to cover greater distances, conduct extensive mapping over larger areas, and maintain extended periods of monitoring, especially for military surveillance. However, one disadvantage of fixed-wing drones is their inability to hover in a stationary position and maintain low speeds (Singhal, Bansod, and Mathew Citation2018). The Wing-based UAVs can be further classified into three sub-categories based on their wing configurations, including fixed-wing, flapping-wing, and flying-wing UAVs (Ahmed et al. Citation2022).
When examining the key applications of drones/UAVs, their widespread integration into the LSC domain is apparent. Historically, drones were predominantly utilised for military operations until 2001 (Drones&SC Citation2023), but their versatility has since propelled them into a myriad of applications. Drones stand as cutting-edge instruments facilitating access to otherwise inaccessible or perilous locations (Hildmann and Kovacs Citation2019). Their utilisation extends to various domains, including mitigating risks in the medical field through the transport of medicines and blood supplies (Drones&Logistics Citation2015). Presently, drones/UAVs are also indispensable in areas such as light displays, photography, and video recording (Javier Citation2012). Certainly, within SCs, one compelling application of these tools is in the management of deliveries and packages (Drone-Delivery Citation2023). For instance, the research by Zou et al. (Citation2024) propose a novel locker-drone delivery system, optimising network design for cost-effective operations in community and intra-facility logistics. In another study, Shen et al. (Citation2021) investigate operational policies in a multi-warehouse drone delivery system, focusing on the innovative application of drones for home deliveries instead of military or disaster management. Additionally, the integration of mobile additive manufacturing with truck-drone delivery systems enhances supply chain resilience by optimising delivery schedules and improving cost performance. (Abdolazimi and Ma Citation2024). SCs have embraced collaboration with drone manufacturers to enhance their delivery networks (Shen et al. Citation2021). For instance, Domino, a food supply chain, partnered with Flirtey, a drone company, to offer food delivery services via drones. Furthermore, Walmart utilises drones for inventory management (Drones&SC Citation2023) and the control of RFID tags (Zhang and Furusho Citation2017). Moreover, the agility and cost-effectiveness of contemporary drone/UAV services have positioned them as alternatives to traditional forklifts, particularly in warehouse and distribution centre operations (Drones&SC Citation2023). Similarly, they have found a niche in logistics by replacing conventional shipping methods, showcasing their prowess in this domain (Zhang and Furusho Citation2017). Drones/UAVs are also used in other sectors. They are instrumental in monitoring planetary health, assessing crop yields, and conducting soil testing for land optimisation in agricultural SCs (Telmo Citation2017). Furthermore, the mining industry relies on drones/UAVs for activities such as inspections, automated surveying, measurements, and mapping (Drones&Mining Citation2019). Hildmann and Kovacs (Citation2019) provides a succinct summary of relevant domains where drones/UAVs make substantial contributions, encompassing public safety in smart cities, remote sensing, overcrowding and traffic management, emergency monitoring and control, security in urban or public areas, 3D environment surveying and monitoring, coordination among heterogeneous systems, communications, and big data generation in IoT networks. This study delves into the applications of drones/UAVs specifically designed for LSC purposes.
The remainder of this paper is organised as follows: Section 2 provides a comprehensive review of related literature. In Section 3, we explain the methodology we used for our study, including how we selected and excluded research materials. Section 4 presents our findings, with explanations of various insights we gained from data visualisations and the output of the topic modelling process. Section 5 includes detailed discussions, particularly focusing on the rearrangement of the identified topics and outlining potential future research directions in the field of drone-based LSC. We summarise our conclusions in Section 6.
2. Literature review
2.1. Challenges in utilisation of drones/UAVs
Within scholarly literature, many studies have delved into the array of opportunities arising from the use of drones/UAVs across various domains. Nonetheless, despite these potential benefits, several obstacles impede their effective implementation in practice. A paramount challenge lies in the underlying technology of these systems, which necessitates consideration of cultural, legal, environmental, and technical facets (Bhatt, Pourmand, and Sikka Citation2018). For instance, legislative bodies in many regions are in the process of formulating regulations governing the use of these aerial tools (Sah, Gupta, and Bani-Hani Citation2021). Some governments, such as the United States, have imposed strict rules governing drones. In the U.S., regulations mandate that industrial/commercial drones/UAVs operate only during daylight hours and below an altitude of 400 feet. Similar restrictions can be encountered in various contexts. Moreover, the operation of these vehicles poses numerous challenges in adverse weather conditions, including storms, strong winds, snowfall, or fog (Drones&SC Citation2023; Liu Citation2023). Furthermore, the performance of drone batteries, particularly their capacity, is one of the main factors affecting the delivery range and has emerged as a critical concern in the effective deployment of drones/UAVs (Drones&SC Citation2023; Liu Citation2023; Pei et al. Citation2024). On the flip side, this technology is not immune to computational and technical errors and is susceptible to cyberattacks (Bhatt, Pourmand, and Sikka Citation2018). There's also the risk of not reaching the intended destination due to potential obstacles, bird interference, or buildings along the flight path (Hildmann and Kovacs Citation2019; Mourtzis, Angelopoulos, and Panopoulos Citation2024).
The challenges associated with the application of drones/UAVs are often categorised into these domains: security, privacy, reliability, energy conservation, infrastructure management, limited payload capacity, restricted flight range, and deployment logistics for drones/UAVs. Among these challenges, path planning emerges as one of the most critical considerations, especially in the context of drone delivery (Mourtzis, Angelopoulos, and Panopoulos Citation2024; Pinto and Lagorio Citation2022; Zou et al. Citation2024). Effective path planning enables drones to navigate their environment efficiently, optimising routes by considering factors such as travel distance, collision avoidance, and mission execution time (Mourtzis, Angelopoulos, and Panopoulos Citation2024). While many articles have acknowledged the cost-effectiveness of this technology, only a limited number have delved into the nuanced aspects of cost parameters. In this study, we undertake an in-depth investigation into the obstacles associated with the utilisation of these aerial vehicles within the LSC framework.
2.2. Exploring recent relevant literature reviews
Based on previous research in the field of drones/UAVs, it is evident that there is a limited number of survey papers that explore the applications of drones/UAVs in LSC. Our investigation primarily focused on reviewing articles that offered diverse perspectives on the utilisation of drones and UAVs. Table provides a concise summary of these pertinent articles. Among the studies examined, the earliest comprehensive survey on drones and UAVs was conducted by Bravo and Leiras (Citation2015). This systematic review delved into the various applications of drones and UAVs within the context of humanitarian relief efforts. The study scrutinised the use of these aerial technologies in domains such as 3D mapping, imaging, sensor networks, and medical surgery, taking into consideration the specific type and occurrence of disasters. The authors concluded that the integration of UAVs with other vehicles holds the potential to enhance the efficiency and expediency of humanitarian relief operations.
Table 1. Review studies on the applications of drones/UAVs.
Natu and Kulkarni (Citation2016) provide a comprehensive review of the utilisation of precision agriculture through aerial-based systems, including drones or UAVs, highlighting their role in enhancing sustainability in agriculture. Similarly, Lagorio, Pinto, and Golini (Citation2016) aggregate a list of topicsrelated to urban urban logistics from the existing literature, with a specific focus on transportation management, logistics, and operational management. They acknowledge that the literature on urban logistics is highly fragmented, encompassing a diverse array of topics, which has consequently hindered the emergence of a comprehensive study addressing the various issues within urban logistics. Conversely, in the study conducted by Altawy and Youssef (Citation2016), the primary emphasis is placed on the challenges associated with the adoption of drone technologies. The authors acknowledge the paramount importance of security, privacy, and safety concerns within the domain of civilian drones.
The study by Büyüközkan and Göçer (Citation2018) reviews new ways in the digital supply chain, including smart solutions and new technologies. The paper highlights UAVs as an innovative solution in the digital supply chain. However, it does not delve into the applications and challenges of using UAVs in detail (see also Ardolino et al. Citation2024). Bhatt, Pourmand, and Sikka (Citation2018) review the delivery of automated external defibrillators, medicine, vaccines, and medical specimens by drones/UAVs. They categorise selected articles by model type (geographic or mathematical), findings, and results. However, they do not provide in-depth details about each model; they only list them in the survey. Allison et al. (Citation2016) review the use of UAVs and sensor platforms in monitoring, detecting, and mapping wildland fires. Rosser et al. (Citation2018) review the applications of drones/UAVs and categorise them into three categories based on medical articles: (1) disaster relief or public health; (2) telemedicine; (3) medical transport.
Otto et al. (Citation2018) examined optimisation modelling in the operation planning of drones/UAVs, with a specific focus on civil applications. The authors reviewed the various types and features of drones while emphasising their utility in civil applications, particularly in areas characterised by poor physical infrastructure. It was shown that drones/UAVs are deployed for cost-effective and environmentally friendly delivery, resulting in reduced emissions. The authors also underscored the significance of drone landing as a pivotal stage within the delivery process. Otto et al. (Citation2018) comprehensively introduced and described the parameters and constraints governing drone planning, encompassing strategic, operational, and tactical considerations. The study also delved into the intricacies of setting up drone operations and scheduling human operators, as well as the synchronisation of drones with other vehicles. Consequently, this research offers valuable guidelines for the modelling and optimisation of drone/UAV-related issues.
The reviews by Hildmann and Kovacs (Citation2019) and Chung, Sah, and Lee (Citation2020) cover various aspects of utilising UAVs in different sectors. Hildmann and Kovacs (Citation2019) primarily focus on UAVs as data acquisition platforms within mobile sensing and actuation systems, summarising applications in civilian affairs, agriculture, urban services, transportation, and healthcare. They categorise these applications into areas such as remote sensing, search and rescue, surveillance, delivery, imaging, and more. Meanwhile, Chung, Sah, and Lee (Citation2020) delve into mathematical models and solution strategies for integrating drones and trucks in operational contexts. They explore combining drones and trucks to enhance delivery capabilities, addressing challenges like battery recharging. Their study categories literature based on modelling approaches, optimisation methodologies, practical applications, and the distinct features of drone-vehicle operations.
Similar to the work of Otto et al. (Citation2018), this research conducted a comprehensive investigation into mathematical modelling. However, it primarily concentrates on the application of drones in conjunction with trucks, while somewhat overlooking the parameters and constraints that pertain to alternative drone/UAV applications.
2.3. Research gaps
The significance of using drones/UAVs to enhance efficiency by reducing costs and service times has been recognised over time. However, many researchers and practitioners still remain uncertain regarding the optimal application of these technologies across various sectors. Our research identifies a crucial gap highlighted by Table , which points to the lack of a comprehensive analysis of drones/UAVs applications throughout the end to end supply chains. To address this gap, our research conducts a text mining-based literature review with a specific focus on the utilisation of drones/UAVs within the LSC. We employ a novel text mining approach to extract and synthesise knowledge from a substantial number of studies classified as unstructured data. This approach not only seeks to provide a better understanding of current drone/UAV applications but also to identify requirements and best practices across various industries, offering valuable insights into their applications in supply chains.
Considering the identified gap in the litreture, this survey aims to address key research questions that directly contribute to bridging this divide. These questions are designed to uncover the breadth and depth of drone/UAV applications across various industries and to highlight the need for such technologies within the supply chain. Through this approach, We aim to provide comprehensive insights into the integration and impact of supply chain dynamics. Specifically, we seek to respond the following research questions:
What are the current industry-specific applications and requirements concerning drones and UAVs in the LSC domain?
In which phases of the supply chain is the demand for drones and UAVs most pronounced, and in what domains do their applications exhibit the most substantial growth?
What research gaps and unexplored areas remain within the academic literature concerning industry demands and drone/UAV requirements?
The primary goal of this study is to present a comprehensive review of the literature pertaining to LSC with regard to the utilisation of drones/UAVs. Specifically, the study is guided by the following objectives:
To identify and categorise the diverse industry requirements for drones/UAVs within the LSC domain.
To identify the scope of drone/UAV applications appealing to academia.
To identify the disparities between the proposed models and the industry demands for drones/UAVs.
The outcome of this study will deliver valuable insights not only for scholars and academic researchers but also for practitioners and industry professionals.
3. Methodology
This study presents a targeted systematic text mining literature review, employing an integrated methodology for the identification, assessment, and interpretation of pertinent studies in accordance with the research questions and objectives. The research process encompasses five primary steps, as illustrated in Figure . The first step involves the collection of materials from pertinent sources, a procedure expounded upon in the subsequent section. Raw data is used for visualisation and the derivation of insights. In the second step, we deploy a novel topic modelling approach to initially discern extant themes within the literature. The third step entails the analysis and classification of the identified topics. Subsequently, the significant trends are delineated, taking into account the prevailing gaps in the literature. Finally, in the concluding step, we summarise several key insights drawn from the literature.
Figure 1. Overview of the research process.
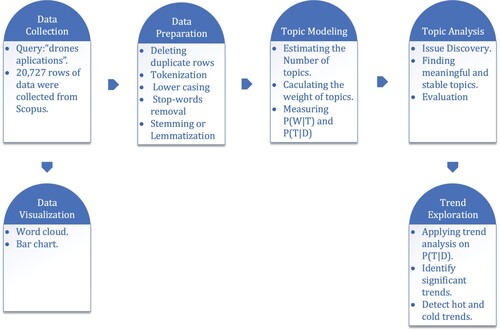
3.1. Data collection
Since we need to get data from pertinent research databases offering an Application Programming Interface (API) or large data extraction, we focused on Google Scholar (GS) and Scopus data sets. GS does not support any API, thus, we could not employ it in our data collection process. We selected Scopus database as it provides downloading 2000 records at a time. To detect research sources, we searched articles written in the English language and published before 2023. We gathered available journal and conference papers' data from Scopus containing drones or UAVs in their title, abstract, or keywords. We also collected year, source title (name of journal or conference), and number of citations for each paper. We decide to analyse only title, abstracts, and keywords, instead of full-text papers for two reasons: (1) Title, abstract, and keywords are available for all papers, however, the full-text format is limited for download. (2) title, abstract, and keywords include wealthy information of the methodology and main findings. Westergaard et al. (Citation2018) confirm this basis by investigating 15 million full-text papers. Since the data for the year 2023 was not completed, we dropped them from some processes. Based on the keywords used in the recent survey papers and our research objectives (see Section 1), initially, we selected a wide range of keywords to select articles (Table ). Then we excluded unrelated articles and limited the search results based on exclusion keywords (listed in Table ). Since this study is aimed to demonstrate the applications of drones/UAVs in LSC, in order to examine the literature more closely and limit the related articles, the articles on special engineering topics like ‘Antennas’, ‘Vehicular Ad Hoc Networks’, ‘Mobile Telecommunication Systems’, ‘Telecommunication Networks’, and ‘Robotics’ were also identified as irrelevant and excluded from the list. After this step and consensus among the authors, our last search in May 2023 included 3983 articles for the research process. We downloaded the data by every 2000 batch extracted from Scopus and combined them as a corpus CSV file. We checked the number of missing values as shown in Table . Since there was no missing value in the title, abstract, and year columns, we decided to consider other missing values as Null values.
Table 2. Initial search keywords.
Table 3. Secondary exclusion keywords.
Table 4. Missing data in every column of data.
3.2. Data preparation
After deleting few duplicate rows from our collected data, we integrated title, abstract and keywords as a new ‘content’ attribute for our topic modelling input data. We removed ‘drone, drones, UAV, UAVs’ words from the content since they are repetitive and pointless words in all records. After this step we reached to 5364 unique papers (published between 1978 and March 2023) with their pre-processed contents.
3.3. Topic modelling
In responding to research questions 1 and 2, we employed topic modelling, specifically using Latent Dirichlet Allocation (LDA), to reveal the latent semantic structures within the literature. Among various topic modelling methods, LDA stands out for its robustness and efficiency in extracting topics from extensive collections of text, making it an ideal choice for analysing the diverse and complex field of drone applications in LSC. The LDA's ability to systematically uncover themes in large datasets has proven invaluable in fields ranging from health and politics to social media analysis and opinion mining (Jelodar et al. Citation2019; Xue et al. Citation2020). The choice of LDA was motivated by its capacity to manage and interpret extensive unstructured text, enabling the discovery of hidden topics that are not immediately apparent. This approach is particularly beneficial for our study, allowing us to navigate through and categorise the breadth of research on drones and UAVs in LSC. However, we recognise the constraints of LDA, including its susceptibility to parameter adjustments (such as the number of topics), which can impact both the results and the ease of interpretation of the model. Despite these challenges, LDA's strengths in text analysis and topic identification uniquely position us to advance understanding in the LSC domain, filling a notable gap by highlighting emerging themes and areas lacking in drone application research. Other new applications of LDA includes Trend analysis (Hyojung, Inho, and Minjung Citation2022), Quality Assessment (Bodrunova, Blekanov, and Kukarkin Citation2019), Sentiment Analysis (Bagherzadeh et al. Citation2021), Health care analysis (Evansa, Copenhaver, and Nguyen Citation2019), Review mining (Cao, Lu, and Xu Citation2018). Our critical review on survey studies using LDA demonstrated that although several studies employ LDA for topic modelling of supply chain papers such as (Akhtar et al. Citation2017; Nezafati and Sheikhattar Citation2022; Tavana et al. Citation2022), in general, there is no study focusing on drones and their applications in LSC.
LDA Offers two matrices: and
. The first matrix diagnoses semantically relevant words characterising a theme. The second matrix diagnoses distribution of topics inside a document (here we use the combination of title, abstract, and keywords for each paper as a document. The latter matrix helps in detecting documents related to every topic. For t topics, m words, and n papers (documents), the probability of each word existing in each topic or
and the probability of each topic existing in each document or
is formulated as:
(1)
(1)
The top topics for all documents are found by and the top words are listed by
for each topic. To find the importance of each topic,
is also calculated. A normalisation approach is applied on STs employing the summation of weights as follows:
(2)
(2)
Once , it means that researchers have been discussed topic a more than topic b. The normalisation process has been applied on all documents/papers for all years/journals to find the weight of topics accordingly.
3.4. Topic analysis
We employ a qualitative methodology to analyse the topics in four steps to reveal the meaning of every topic and its theme: (1) recognise a theme for each topic, (2) identify a meaningful and sustainable list of topics, (3) recognise comprehensive categories, and (4) examine the reliability of the coding process. The steps are explained in more detail as follows.
Step 1. Identify a suitable theme for each topic: The authors initially identified and coded ten (10) topics. In this context, ‘coding’ refers to one author's review of the most frequently used words (as indicated in Table ) and a more extensive examination of the relevant abstracts and keywords of papers associated with each topic generated by the LDA model. To prioritise and pinpoint the most relevant papers for each topic, the authors organised the probabilities in descending order. As stated by Pejić Bach et al. (Citation2019), coding agreement is a prerequisite for all themes discovered within the topics. To establish coding agreement, one author independently formulates a theme for each topic, followed by a collaborative discussion between the authors to reconcile the themes they have generated. This iterative process continues until a consensus is reached on the final themes. In this study, coding agreement is used to specify the name/tag and description of the identified topics. For instance, one of the identified topics included these words: ‘risk’, ‘factor’, ‘find’, ‘signific’, ‘product’, ‘result’, ‘medic’, ‘indic’, ‘remot’ and ‘assess’. After analysing the top relevant papers' abstracts and keywords (‘covid-19’, ‘drone food delivery services’, ‘hiv’), the authors agreed to introduce ‘medical services’ as a theme for this topic (see T0 in Table ).
Table 5. Annual top 10 cited papers (Not survey studies).
Table 6. Annual top 10 cited papers per year (Not survey studies).
Table 7. Dominant topics and their top keywords (the numbers are the percentages contribution in each topic) using whole data set.
Step 2. Identify meaningful and relevant list of topics: This step is focused on determining topics that are meaningful and relevant to drone applications in LSC. To achieve this objective, we eliminated three types of topics: (1) Topics containing general words that do not exhibit a coherent theme. (2) Unstable topics that do not consistently appear in all tests, even after running the LDA process multiple times (in our study, three times). (3) Topics associated with FANet (Feedback Attention Network).Footnote1 We employed the coding agreement process and LDA test results to refine the topics, ultimately agreeing upon ten meaningful and relevant topics pertaining to drone applications.
Step 3. Categorisation: In this step, One author consolidates topics into fewer categories, while another author reviews these categories. In our study, we examined our initial ten topics and opted to merge some with others. Subsequently, another author advocated for the remaining topics, leading to consensus on eight topics with their corresponding category labels.
Step 4. Examine the reliability of the coding process: After completing the final coding, we employed an additional, distinct coding process to assess the agreement in our coding. This allowed us to determine whether another individual, working with the same dataset, would arrive at the same results.
3.5. Trend exploration
As shown in Figure , we applied statistical trend analysis of topics that helps in identifying hidden patterns to travel beyond superficial observations of research trends. To identify trends across drone application topics, the linear trend model has been utilised, tracing the weight of the annual topics using . Table presents a detailed linear temporal trend analysis of these significant topics. Table categorises topics (T0 through T9) based on their slope (R) and statistical significance (P value), along with their frequency of occurrence. Topics with a positive slope (R>0) indicate an increasing trend over time, while a negative slope (R<0) denotes a decreasing trend. The significance of these trends is determined through P values, with ‘ns’ indicating no significant change (
), ‘
’ for significant (P<0.05), ‘
’ for highly significant (P<0.01), and ‘
’ for extremely significant trends (
). The frequency column reflects the occurrence of these topics in the literature, providing a quantitative measure of attention each topic receives. Based on Table , topics T3, T5, T6, and T7 all show a positive slope, with T7 exhibiting the most substantial growth, thus highlighting its significant rise in scholarly discussions. Conversely, topic T1, with a negative slope (
) and ‘
’ significance level, suggests a declining interest. Topics labelled ‘ns’ for their P value indicate no significant change over time, suggesting stable interest levels without clear trends of increase or decrease. This methodology allows us to systematically identify areas of growing scholarly interest, guiding future research directions in drone technology applications.
Table 8. Linear temporal trend of meaningful and stable topics related to drones applications (ns: : Positive Slope; R<0: Negative Slope).
4. Results
4.1. Data visualisation
In this section, the graphical representations and insights obtained from the analysis of the collected data are presented. Due to the incomplete data for 2023, this year has been excluded from our analysis of the annual distribution of papers, as depicted in Figure . The trend observed in the article distribution indicates an exponential increase, with approximately 85% of the articles being published within the timeframe spanning from 2015 to 2022.
Figure 2. Distribution of papers during years (1978 to 2022).
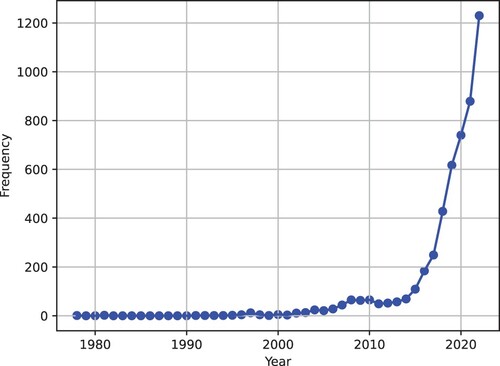
Figure shows the top-10 high-frequency publication outlets. The main journals publishing research on drone applications in LSC include IEEE Access, Sensors (Switzerland), IEEE Transactions on Vehicular Technology, Drones, Journal of Intelligent and Robotic Systems: Theory and Applications, and Applied Sciences (Switzerland). These journals primarily focus on modelling, designing, and applications of drones, along with advancements in emerging technologies related to vehicular electronics and transportation systems, as well as potential applications for robotics and automation across diverse fields. Among these journals, IEEE Access stands out with the highest frequency, including 84 papers, while Applied Sciences (Switzerland) has the lowest frequency with 19 papers.
Figure 3. Top-10 high-frequency venues.
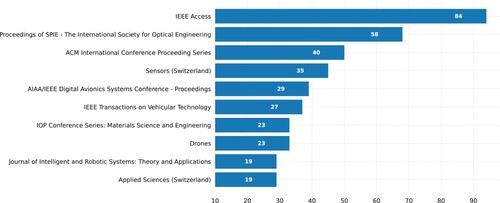
Notably, Drones is a specific journal found among the top-10 journals in the list. Drones is a monthly peer-reviewed, open-access journal published by MDPI,Footnote2 with a global reach. The journal is dedicated to exploring the design and diverse applications of drones, encompassing UAVs, Remotely Piloted Aircraft Systems (RPAS)UAS, Unmanned Aircraft Systems (UAS), and similar technologies.
Several conferences also express interest in publishing research on drone applications, including Proceedings of SPIE – The International Society for Optical Engineering, ACM International Conference Proceeding Series, and IOP Conference Series: Materials Science and Engineering. The various venues demonstrate the growing popularity of drones in various fields, highlighting the necessity to continually advance the state of the art in drone/UAV technology and its wide-ranging applications.
We present the word frequencies in Appendix 2, offering detailed insights into the frequency of words and terms found in the content analysed for this study. We further analyse the keywords of the papers in Figure . We decided to remove our searched words (reported in Table ), including drone, UAV, and their acronyms. The figures illustrates that vehicle routing problems are extensively employed as main keywords in papers and appears in sole ‘routing’ word and other combinations like ‘routing protocol(s)’ (see review study of Drone-based routing problems done by Macrina et al. Citation2020). Figure depicts the wordcloud of the top-50 high-frequency keywords in which the searched words and top words are highlighted as expected.
Figure 4. Number of papers used any of the top-10 keywords.
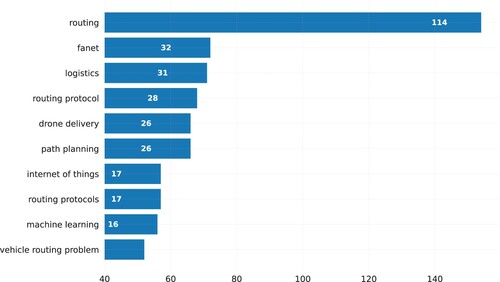
Figure 5. Wordcloud of the top-50 high-frequency keywords.
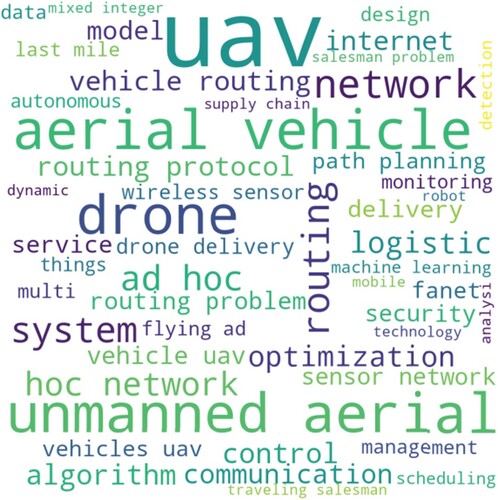
Figure demonstrates the number of citations given to articles published during a year. In the figure, we have utilised log scale transformation. One main insight from this figure is that the recent growth of the literature has been occurred in the last seven years. We go through more details of the top cited papers in Tables and . While Table provides the total number of citations for each paper, Table represents the citations per year, indicating the ongoing impact of these papers. Comparing the two tables reveals that some papers, such as ‘A Tutorial on UAVs for Wireless Networks: Applications, Challenges, and Open Problems’ (Mozaffari et al. Citation2019) and ‘Vehicle Routing Problems for Drone Delivery’ (Dorling et al. Citation2016) consistently rank high in both categories. However, other papers, like ‘The flying sidekick travelling salesman problem: Optimisation of drone-assisted parcel delivery’ (Murray and Chu Citation2015) and ‘Cloud-based design and manufacturing: A new paradigm in digital manufacturing and design innovation’ (Wu et al. Citation2015) demonstrate a higher impact when considering the number of citations per year. This comparison underscores the long-term influence of certain papers and highlights the emerging relevance of others over time.
Figure 6. Number of citations given to each paper and the year each paper was published.
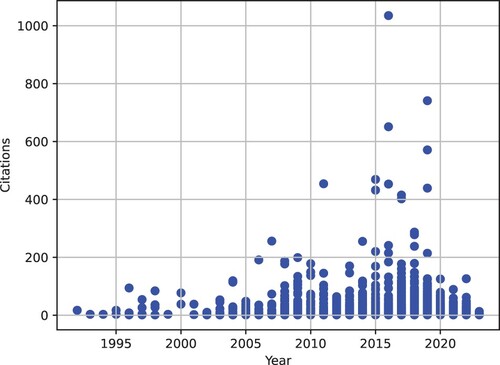
4.2. Topic analysis
In addition to the basic frequency analysis, we used LDA to reveal the hidden semantic structure of papers. LDA's pre-processing step includes defining the ideal number of topics, denoting as K in Equation (Equation1(1)
(1) ), by using the coherence metric to estimate the optimum point. Selecting an optimal K is crucial. Higher values of K result in more specific and focused topics, while excessively large K choices may lead to topics that lack meaningful distinctions and appear strikingly similar. Conversely, opting for a smaller K may amalgamate distinct data aspects into broader topics, potentially compromising their individuality.
Following Alizadeh et al. (Citation2023), a selection of four distinct evaluation metrics was employed to ascertain the optimal K. These metrics encompassed both minimisation approaches introduced by Cao et al. (Citation2009) and Arun et al. (Citation2010) as well as maximisation metrics developed by Griffiths and Steyvers (Citation2004) and Deveaud, SanJuan, and Bellot (Citation2014). In Figure , we present the four metrics for our dataset by selecting the number of topics from 1 to 50. Subsequently, considering both maximisation and minimisation approaches, the optimal K is found as 10.
Figure 7. Coherence scores for finding the optimal number of topics using both maximisation and minimisation approaches.
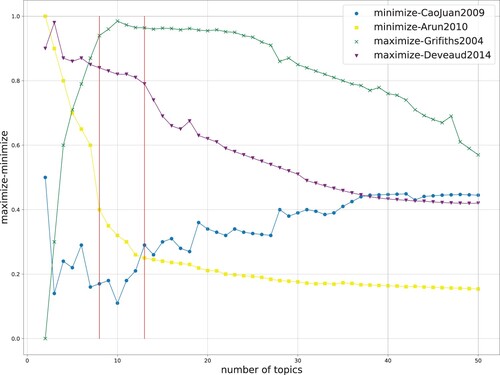
Table and Figure show the detected 10 topics. The arrangement of topics in Figure is based on the values of sorted from the highest to the lowest value (the highest weight: FANET, the lowest weight: humanitarian drone). The drone network is known as FANET (Flying Ad-Hoc Network), and it is a subset of the MANET (Mobile Ad-Hoc Network). From the above results and LDAvis package in Python (a method for visualising and interpreting topic models), we decided to merge 8 topics into 4 topics (T0 and T3), (T6 and T7), (T4 and T8), (T1 and T5).
Figure 8. Weights of topics gained by the LDA topic modelling approach.
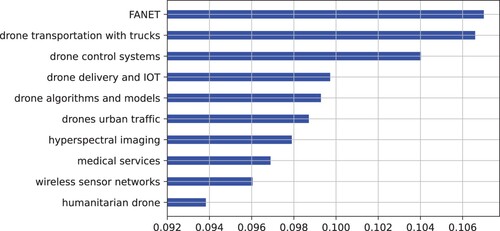
As listed in Table , the prominent keywords identified in the T0 and T3 topics included COVID-19, drone food delivery, HIV, IoT, routing, and energy efficiency. Based on the subject and abstract analysis of the top papers in this domain and their corresponding three main keywords, we determined the label ‘Medical Services using drones’ for the merged topic. This approach was also applied to select labels for other merged topics, ensuring consistency in the categorisation process. We changed the labels of these topics to new labels as shown in Figure .
Among the eight relevant and interconnected topics analysed, it was observed that 50% of them did not show a significant trend over time. However, 40% of the topics displayed an increasing trend (referred to as ‘hot’), while 10% experienced a decreasing trend (referred to as ‘cold’). It is worth mentioning that the topic labelled as FANET consistently held the highest weight each year from 2017 to 2022, as depicted in Figure . Furthermore, in the years 2020 and 2021, researchers showed particular interest in drone delivery and IoT, FANET, and drone transportation with trucks, indicating their popularity within the research community.
Figure 9. Weights of topics gained by the LDA topic modelling approach with respect to years of publications.
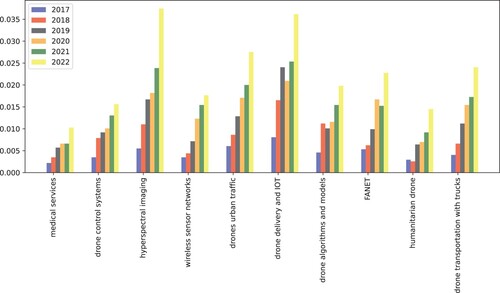
In our analysis spanning from 1978 to 2023, we investigated the temporal patterns of 10 topics, shown in Figure . Our findings revealed that 5 of these topics did not exhibit statistically significant trends , while the remaining 5 topics demonstrated significant trends
. Among 5 topics with significant trends, one topic displayed a decreasing trend (referred to as ‘cold’), while the remaining 4 topics showed increasing trends (referred to as ‘hot’), as illustrated in Figure . Notably, the majority of the hot topics focused on the communication aspects between drones.
4.3. Logistics and supply chain
Our primary objective in addressing research questions 1 and 2 is to thoroughly investigate the applications of UAVs specifically in the context of LSC. Thus, we decided to separate these articles from the basic ones focused exclusively on unmanned aircraft applications and redo the text mining process specifically to this subset. For this data set, we selected only papers that include ‘logistics’ or ‘supply chain’ only in their topics or keywords. As a result, we found 882 articles to discover the applications of drones in LSC. By implementing the steps listed in Section 3.4, the obtained topics are gained and reported in Table . In general, 7 topics have been obtained after doing topic modelling for the data. After checking and talking with the team, two of the topics were removed and five topics were labelled as final. Table reports the final topics along with the number of related articles and the top three keywords. These topics primarily focus on the utilisation of drones in LSC, emphasising their importance and the increasing integration of drone applications in this domain.
Table 9. Dominant topics and their most frequent keywords using only logistics and supply chain domain data.
Table 10. Final dominant topics of logistics and supply chain domain.
Figure illustrates the top-ten keywords of these articles and reflects that machine learning is the most frequent keyword (method) employed in the domain. Figure also introduces the 10 most frequent words in the articles and reflects ‘system’ word as the most frequent one.
Figure 12. Frequency of top-10 keywords in logistics and supply chain papers.
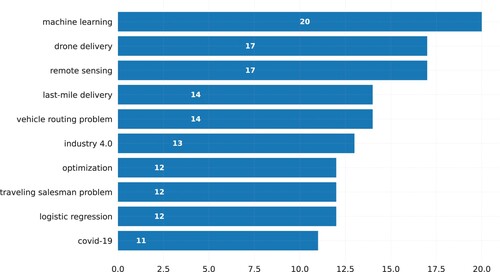
Figure 13. Frequency of the top-ten high-frequency words in logistics and supply chain papers.
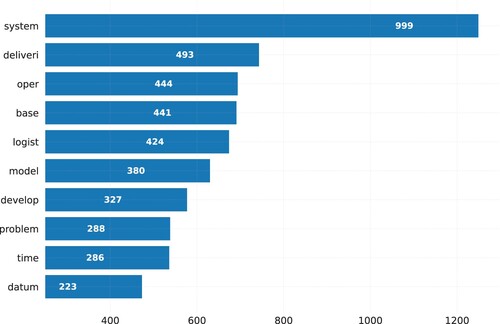
We labelled the Topic 0 as ‘Artificial intelligence and technology’ due to the predominant focus of the articles in this area on topics such as artificial intelligence, object recognition, and image processing using artificial intelligence algorithms. The top three keywords in this category are blockchain, artificial intelligence, and industry 4.0. Regarding Topic 1, we assigned the label ‘Sustainable and e-commerce SCM’ based on the predominant content of the articles. Notably, the main keywords associated with this category include sustainability, e-commerce, and supply chain management. As evidenced, the majority of these articles primarily discuss strategies and techniques for effectively handling supply chains that incorporate sustainability and online sales in the SC. In terms of Topic 2, the papers predominantly focus on exploring the application of drones in wildlife/animals monitoring studies. Specifically, these papers delve into areas such as animal identification and monitoring, as well as the assessment of unnatural factors in their living environment. The top three keywords associated with this category are risk assessment, paraglider, and parachute. These keywords highlight the key aspects addressed in the articles, including evaluating potential risks to wildlife, utilising drones in aerial observations, and employing parachutes or paragliders for specific research purposes.
Regarding Topic 3, on the other hand, emerged as one of the most popular research topics among scholars, centring on the utilisation of drones for the delivery of goods. The main keywords are drone delivery, vehicle routing problem, and travelling salesman problem (Bogyrbayeva et al. Citation2023; Luo et al. Citation2021). Furthermore, the articles also explore the combination of using trucks for long-distance transportation and employing drones for the final delivery in the last mile (Wen and Wu Citation2022). The research in this domain aims to enhance the overall efficiency and effectiveness of goods delivery systems by integrating drone technology into supply chain logistics.
The papers categorised under Topic 4, labelled as ‘Machine Learning Sensors’, primarily discuss the utilisation of novel sensors in unmanned drones and their practical applications in various aspects of human life. The related studies focus on harnessing the potential of advanced sensors to enhance and improve different domains. The top three keywords in this category are machine learning, remote sensing, and precision agriculture. These keywords highlight the main areas explored in the articles, including the application of machine learning techniques to analyse sensor data, utilising remote sensing technology for data collection and analysis, and leveraging precision agriculture methods with the help of sensor-equipped drones.
5. Discussion and future directions
Our research highlights the rapidly growing demand for UAVs in the LSC industry, marking a pivotal transition towards more agile, responsive, and efficient supply chain operations. The applications of UAVs have witnessed notable growth, highlighting the increasing need and expanding the utilisation of UAVs to improve supply chain operations. Our results demonstrate that drone applications have been explored across various fields, such as medical, IoT, Flying FANET, humanitarian, hyperspectral, and drone transportation. Among these applications, FANET emerged as a particularly popular area of investigation among scholars. This review provides valuable insights for researchers, publishers, and policymakers, enabling them to gain a comprehensive understanding of the broader landscape of issues, research topics, and their evolving trends in the field of drone applications.
Summarising the extensive research on drones and UAVs applications for LSC over the past few years would be an enormous undertaking using traditional review methods. Our methodology extends beyond traditional review methods by efficiently analysing research papers, revealing the transformative impact of UAV technologies on supply chain dynamics. Our approach enables the examination of a large sample of papers, contrasting with the time-consuming and resource-intensive nature of traditional methods. Furthermore, this efficient approach enables the identification and prediction of both hot and cold research themes, providing insights into emerging trends as well as unexplored areas. Lastly, the flexible research framework used in this study can be easily adapted and applied to other research issues. This efficiency and adaptability in exploring UAV applications provide a strategic advantage for decision-makers in the LSC, equipping them with actionable insights to leverage UAV technology effectively.
Following an extensive analysis in response to research questions 1 and 2, we have identified six distinct UAV application areas in LSC, each offering unique contributions to operational efficiency and cost reduction: (1) hyperspectral imaging, (2) drone transportation, (3) IoT, (4) humanitarian drones, (5) FANET, and (6) medical services (as shown in Table , Figures and ). The hyperspectral imaging class specifically examines the capabilities of RGB and/or NIR sensors with hyperspectral sensors. It explores the application of these sensors in IoT and UAVs for image processing, object detection, and face recognition. It is worth noting that this class had the lowest frequency compared to the other classes, indicating that it received relatively less attention or discussion in the exciting literature. The drone transportation class, represented by the topic with the ID T9, primarily focused on proposing models and algorithms for the efficient delivery of goods using drones in conjunction with trucks. The main objective of this class was to address the challenge of reducing costs and time while increasing the speed of delivery. By integrating drones with traditional transportation methods like trucks, the aim was to optimise the delivery process and enhance overall efficiency in the transportation of goods. The proposed models and algorithms likely aim to streamline logistics, improve route planning, and enhance coordination between drones and trucks to achieve these desired outcomes.
The third class, known as IoT, consisted of topics T1 and T5. Among all the classes, this class had the highest frequency, indicating its significance and the extensive research conducted within it. The primary focus of this class was to explore the challenges that arise when integrating IoT systems with drones and propose potential solutions to overcome these challenges. The research conducted within this class involves investigations into various aspects such as data synchronisation, communication protocols, security considerations, and interoperability between IoT devices and drones. By advancing the understanding and knowledge in this area, the research conducted within this class contributes to the broader field of IoT and drone integration, paving the way for enhanced collaboration and unlocking new possibilities in numerous domains and applications.
The humanitarian drone class consisted of topics T4 and T8, both focusing on the use of drones for humanitarian purposes. The primary focus of this class is to investigate and understand how drones can be effectively employed to facilitate humanitarian efforts and address challenges encountered by humans in various contexts. This class represents research on identifying specific use cases where drones can play a pivotal role, such as in disaster response, search and rescue missions, delivering essential supplies to remote and inaccessible areas, and monitoring critical infrastructure. By harnessing the capabilities of drones, this class aimed to find innovative solutions that can enhance efficiency, speed, and accessibility in humanitarian endeavours. Ultimately, the goal was to leverage drone technology to alleviate human suffering, enhance the effectiveness of relief efforts, and improve overall outcomes in humanitarian operations.
The FANET class consisted of topics T6 and T7, focusing on the establishment of a network among multiple drones and the transmission of information between them to enable collaborative operations. This class primarily aims to explore the methods and techniques involved in developing a network infrastructure that facilitates communication and coordination among drones. The research conducted in this class delves into developing practical solutions for establishing seamless connectivity between drones, allowing them to share data, coordinate movements, and perform joint operations effectively.
The last class, known as medical services, included topics T0 and T3. This class revolved around the utilisation of drones in the context of medical and therapeutic services. This class includes research on exploring how drones can be employed to provide various services in healthcare. The highest keyword frequencies associated with this class included terms such as machine learning, path planning, logistics, deep learning, routing, and COVID-19. These keywords indicate the key areas of interest and emphasis within the medical services class. The research aimed to enhance the capabilities of drones in medical settings, optimise route planning, improve logistics, and address healthcare challenges, including the impact of COVID-19. The class sought to develop innovative solutions to improve healthcare services, enhance access to medical resources, and support efficient and effective healthcare delivery.
Exploring the most prevalent topics during each year provides a comprehensive overview of UAV application areas in LSC management, responding to our investigation into research questions 1 and 2. Figure shows the average weight of selected topics between 2017 to 2022. From 2017 to 2022, FANET held the top spot as the most popular research area among scholars and researchers. However, during 2020 and 2021, IoT class also gained significant popularity, emerging as another major area for research besides FANET. In 2021, the outbreak of the coronavirus had a profound impact on the medical services class, leading to increased attention and interest from researchers. This topic garnered increased interest among researchers as they aimed to address the challenges posed by the pandemic. Furthermore, the COVID-19 pandemic also sparked a significant rise in interest and research activity in IoT and drone transportation classes. As a result, these three classes experienced a surge in research and exploration throughout 2021, compared to the previous year. In response to the pandemic challenges, researchers were motivated to explore effective strategies aiming to mitigate the disastrous effects of the pandemic and to leverage the capabilities of drones/UAVs to efficiently address healthcare and logistical needs.
5.1. Production research
As this study is targeted at the International Journal of Production Research (IJPR), we explore IJPR papers on the application of drones and UAVs in production–a crucial supply chain component. Key themes include optimisation models, delivery systems, healthcare logistics, and technological and operational innovations.
Optimisation models enhance UAV-based delivery systems within production environments. For example, a branch-and-price-and-cut algorithm optimises flight routes and battery swapping through a mixed-integer linear programming (MILP) model (Pei et al. Citation2024). Heuristic approaches address uncertainties in drone-enabled supply chains using stochastic mixed-integer programming and genetic algorithms (Tolooie, Sinha, and Raftar Nouri Citation2024). These models are essential for improving production logistics efficiency, especially in just-in-time (JIT) manufacturing.
Delivery systems integrate last-mile and collaborative delivery with traditional production logistics. Urban logistics benefit from flexible drone delivery strategies that optimise routes and energy consumption using MILP models (Pachayappan and Sundarakani Citation2023). Collaborative systems combine UAVs and ground vehicles, enhancing warehouse allocation and resource sharing (Cha et al. Citation2023; Shen et al. Citation2021). These strategies significantly improve material handling and distribution in production facilities.
Healthcare logistics have advanced with drones, especially during the pandemic. Drones delivered vaccines to remote areas, maintaining cold chains and improving availability (Kolter et al. Citation2022). During COVID-19, drones optimised specimen transportation, reducing contact and speeding delivery (Ozkan and Atli Citation2021). Drones in blood supply chains reduced waste and improved efficiency by following lean principles (Mora and Araujo Citation2022). These innovations offer insights for enhancing production systems’ resilience and flexibility.
Technological and operational innovations push UAV applications in production research. Augmented reality (AR) enhances indoor UAV operations with advanced path planning (Mourtzis, Angelopoulos, and Panopoulos Citation2024). Integrating UAVs with traditional logistics optimises locker locations and complex routing by combining UAVs with trucks (Hamdi Citation2024; Zou et al. Citation2024). These innovations are crucial for advancing production research, improving efficiency, reducing lead times, and enhancing supply chain performance.
Future research should focus on integrating UAVs into advanced production systems, supporting smart manufacturing and Industry 4.0. Drones can facilitate real-time monitoring and data collection for predictive maintenance and inventory management. Hybrid UAV systems combining aerial and ground capabilities could further enhance production logistics, providing flexible solutions to meet modern production demands.
5.2. Drone-based sustainable SCs
Given the increasing significance of sustainability on a global scale, it is crucial for future research to explore the integration of drones into various phases of the supply chain while taking sustainability into account. By examining the sustainability aspects of drone-based supply chains, researchers can analyse the environmental and social consequences of employing drones or Unmanned Aerial Vehicles (UAVs) for delivery and transportation. This investigation would encompass factors such as energy consumption, emissions, and noise pollution. For instance, replacing conventional trucks with drones for specific delivery routes, particularly in the last-mile delivery process, has the potential to significantly reduce carbon emissions in supply chain operations.
Furthermore, drones equipped with advanced route optimisation algorithms can assist in optimising delivery routes, thereby reducing the distance travelled and minimising fuel consumption. Hence, future research should aspire to investigate and leverage the advantages of deploying drones and UAVs in SC operations to elevate sustainability practices. Additionally, it is worthwhile for future research to take into account the economic and social facets of sustainability, encompassing factors such as cost-effectiveness, social equity, and community acceptance of drone-based supply chains.
5.3. Utilising emerging technologies to scale global drone-based SCs
While existing studies have primarily focused on drone application in local and regional SCs, a significant research gap exists in comprehensively investigating the potential benefits and challenges of integrating drones within complex and global SC networks. Investigating the capabilities of emerging techniques such as Machine Learning (ML), cloud computing, and blockchain in drone-based SCs can greatly enhance system scalability and decision-making processes. Future research can focus on developing advanced ML techniques to analyse large volumes sensor data collected by drones in LSC operations. This might include exploring methods to process, interpret, and extract valuable insights from sensor data, enabling proactive decision-making and optimisation in LSC operations.
Further exploration is warranted in harnessing remote sensing technology for the integration of drones into LSC systems. Research endeavours should concentrate on the utilisation of remote sensing techniques, such as Light Detection and Ranging (LiDAR) and hyperspectral imaging, to collect data pertaining to terrain, infrastructure, and environmental conditions. This data can be invaluable for purposes including route planning, asset monitoring, and risk assessment, ultimately contributing to the augmentation of logistics operations in terms of efficiency and effectiveness.
Furthermore, it is worthwhile to delve into the potential of cloud computing in reinforcing global scalability within drone-based SCs. Investigating cloud-based platforms for functions such as data storage, real-time collaboration, and scalable computing can enhance the flexibility and responsiveness of SC operations, particularly when spanning multiple regions.
5.4. Integrating drone-based SC with multimodal transportation
Current research has largely concentrated on deploying drones within transportation systems; however, the integration of drones into multimodal transportation and traffic management has received considerably less attention.
Exploring the capabilities of drones and UAVs within traffic systems offers a compelling opportunity to transform how urban traffic operations are conducted, aiming to significantly boost both efficiency and safety. The adoption of drone technology in traffic management can lead to significant improvements, making these systems more agile, adaptable, and effective. Consequently, this could result in more fluid traffic flow, decreased congestion, and improved road safety.
For instance, drones equipped with cameras and sensors can collect data on traffic volumes at various times of the day, providing insights into peak hours and congestion patterns. Moreover, drones can analyse driver behaviour, including instances of speeding, improper lane changes, or other unsafe practices, thereby offering valuable input for the implementation of traffic safety measures. Drones can also serve to identify and categorise various types of vehicles (e.g. cars, trucks, buses) on the road, facilitating lane allocations and enhancing our understanding of traffic composition on specific routes. Consequently, future research endeavours should centre on the integration of drone-based logistics into traffic control and management systems, aimed at mitigating traffic congestion, elevating safety levels, and streamlining transportation systems.
Furthermore, the integration of drones with trucks and rails can lead to the development of hybrid systems where trucks and rails cover long distances, and drones handle short, final-mile deliveries. This approach can result in significant fuel savings and reduced emissions. Future studies can explore the concept of truck-drone or rail-drone integration to create more intelligent transportation systems.
Drones have the capability to provide real-time traffic updates, helping to identify congestion, emergencies, accidents, and other incidents more quickly than traditional methods. Based on the real-time data collected by drones, trucks and rails can be dynamically rerouted to avoid congested areas, enabling more efficient goods transportation within urban areas and thereby reducing traffic congestion and emissions. Future research directions should rigorously explore the prospects and implications of integrating drones with trucks and rails, aiming to foster a more integrated, efficient, and sustainable transportation system. Additionally, this integration has the potential to deliver substantial benefits to industry stakeholders and society as a whole.
5.5. Application of drones in efficient pandemic response and healthcare SCs
Although existing literature predominantly explores the application of drones in SC, the advantages of utilising drones for pandemic control have been largely overlooked in the LSC literature. Thus, we highlight the need for further studies to develop efficient and reliable drone-based systems for vaccine distribution during pandemics. This involves addressing key factors such as identifying optimal routes to maximise coverage and minimising delivery times, determining suitable payload capacities to accommodate vaccine quantities, and implementing effective temperature control mechanisms to ensure the timely and efficient distribution of vaccines to remote areas. Researchers also can look at crowd monitoring or supply delivery to minimise crowded contexts within a pandemic situation.
Real-time monitoring and tracking play a pivotal role in ensuring the efficient and secure transportation of critical medical supplies, particularly during unprecedented pandemics. The integration of real-time monitoring and tracking technologies with drones enables us to seamlessly track and monitor the location, condition, and movement of essential items such as personal protective equipment (PPE), test kits, and vaccines. Future research can explore the utilisation of IoT sensors and blockchain technology to enhance SC visibility, traceability, reduce losses, and improve overall inventory management.
Advancing the autonomy of drones in healthcare SCs holds the potential to significantly enhance response times during health crises, reduce human intervention, and improve operational efficiency. Autonomous drones can expedite the delivery of medical supplies to remote areas quickly and reliably, thereby supporting healthcare providers in their efforts to effectively combat pandemics like COVID-19. Future research in this domain can delve into the development of advanced algorithms and machine learning techniques to enable drones to operate autonomously, navigate complex urban environments, and detect obstacles, thereby facilitating the timely and precise transportation of critical medical supplies.
6. Conclusion
The integration of drones and UAVs into logistics and supply chain (LSC) management has sparked a surge in recent studies, consequently influencing literature reviews in this domain. However, these reviews are frequently hindered by biases and the time-consuming nature of manual coding or bibliographic analysis. This study, by leveraging a systematic research methodology and examining a vast array of academic articles from 1978 to 2023, has highlighted the necessity for innovative review strategies. Employing text mining, temporal trend analysis methods, and an LDA model, the research efficiently navigated through a comprehensive database of journal articles, aiming to extract latent topics and offer a broad perspective on drone applications in LSC.
Our findings reveal five main topics within the LSC literature: artificial intelligence and technology, management, wildlife, delivery, and sensors. This diverse identification underscores the significant potential of drones and UAVs in enhancing LSC practices. By developing an efficient data-driven reviewing approach, the study addresses the critical need for unbiased and expedited analysis in LSC research, moving beyond the constraints of traditional review methods that rely heavily on manual efforts and are limited by their scope and speed.
Although this review offers a comprehensive overview of drone and UAV applications in LSC research, it has some limitations. Firstly, our data collection was limited to articles found in Scopus and Google Scholar, which may exclude relevant studies available in other databases. Secondly, our focus was solely on English-language papers, potentially excluding topics published in other languages. While this review primarily covered research studies, valuable insights may exist in other document formats, such as book chapters, opinion editorials, and news pieces. Fourthly, our analysis was based on abstracts due to restricted access to full-text papers and the time limitations involved in reading a large number of papers. This approach may overlook diverse topics and insights from other databases, languages, and document formats. Therefore, future research endeavors could encompass data collection from additional databases, examine manuscripts in languages other than English, incorporate various document formats and full-text papers, and analyse topics separately to facilitate deeper investigations. Using research databases can provide researchers with platforms to access papers in a suitable format for text mining. Another potential future research direction involves expanding the scope of LSC. This includes conducting separate analyses for each supply chain process (e.g. production planning and inventory management) to provide a more comprehensive and in-depth analysis.
Disclosure statement
No potential conflict of interest was reported by the author(s).
Data availability statement
The authors confirm that the data supporting the findings of this study are available within the article [and/or] its supplementary materials.
Correction Statement
This article has been corrected with minor changes. These changes do not impact the academic content of the article.
Additional information
Notes on contributors
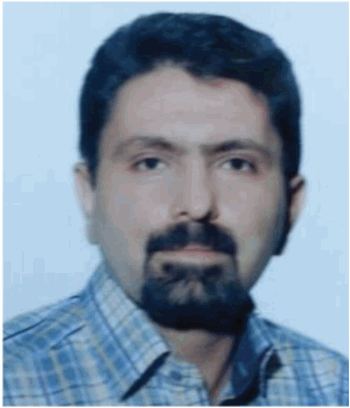
Hamed Jahani
Dr. Hamed Jahani is a lecturer at RMIT University, School of AISSC, Department of Information Systems and Business Analytics (ISBA). His research focuses on applying advanced operations research, machine learning, and text-mining methods in the supply chain and logistics domain. He is particularly interested in understanding quantitative, analytical, and mathematical models applicable to real business problems. Hamed has several papers published in top-ranked A* and A-ranked journals (in ABDC ranking) such as Decision Sciences, European Journal of Operational Research, Journal of the Association for Information Science and Technology, and Transportation Research Part E.
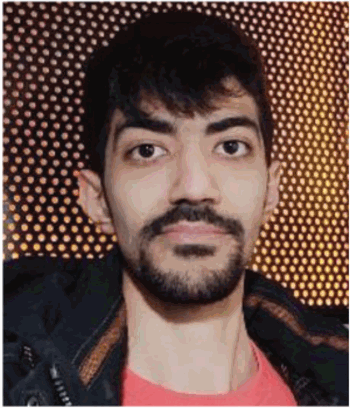
Yunes Khosravi
Younes Khosravi is a master's student in computer architecture at University of Kharazmi, Tehran, Iran. He completed his bachelor's in computer engineering at University of Bojnord in Iran. His research interest is in-memory computing area and data analytics. He also has experience of working in several IT projects using programming skills.
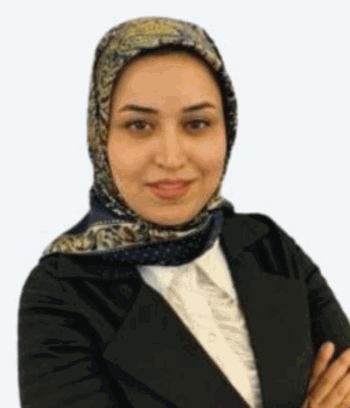
Bahareh Kargar
Bahareh Kargar is a doctoral candidate in Industrial Engineering at the New Jersey Institute of Technology (NJIT), USA. She will join as an Adjunct Professor at the Martin Tuchman School of Management at NJIT for the new academic year. Her research focuses on data-driven decision-making for sustainable planning and operations of large-scale networks. Bahareh's work addresses critical issues in healthcare and sustainable supply chain management, employing methodologies such as stochastic programming, machine learning, and reinforcement learning. She has developed innovative models for disease control planning, dynamic pricing for demand response, and resilient medical waste supply chains.
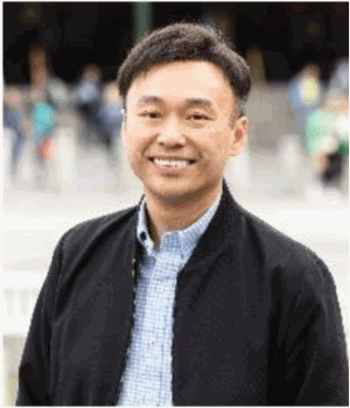
Kok-Leong Ong
Kok-Leong Ong is currently Professor of Business Analytics at RMIT University. His research focuses on analytics and machine learning translation into practice within different business verticals, and the development of new techniques as required to meet individual business needs. As a result, Kok-Leong's research has had a strong focus on impact, with many of his works ranked by Altmetric to be in the top 25% and 5% of research in their respective domains. Since joining RMIT University in 2021, he has founded the Enterprise AI and Data Analytics Hub which quickly went on to secure over $1.4 m in research grants on various projects in e-Commerce, aged care, cybersecurity and other sectors. In 2021, Kok-Leong was one of the four Australia-based academics to be named Leading Data Academics by CDO Magazine, an online publication that grew out of MIT's annual CDO and Information Quality Symposium.
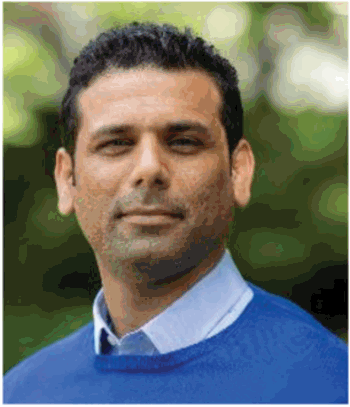
Sobhan Arisian
Dr. Sean Arisian is the discipline lead for Sustainable Operations Management and the founding coordinator of the Logistics and Supply Chain Management programme at La Trobe University, Australia. As an applied operations researcher, Sean has a special interest in modelling and solving practical and interdisciplinary problems. His research addresses issues related to sustainability, security, and resiliency at the global supply chain/international trade interface. His current project aims to enhance the sustainability and resilience of global agricultural supply chains, addressing critical food security challenges posed by disruptions such as trade wars and economic shocks. Sean also conducts research on the development of transformative digital supply chain strategies that promote indigenous innovation at regional and national levels.
Notes
1 FANET: Flying Ad-Hoc Network
2 MDPI: Multidisciplinary Digital Publishing Institute
References
- Abdolazimi, O., and J. Ma. 2024. “An Integrative Production-Delivery System with Mobile Additive Manufacturing and Truck-Drone Delivery Considering Optimal Printing Sequence and Preferred Delivery Time Window.” International Journal of Production Research 1–26.
- Ahmed, F., J. Mohanta, A. Keshari, and P. S. Yadav. 2022. “Recent Advances in Unmanned Aerial Vehicles: A Review.” Arabian Journal for Science and Engineering 47 (7): 7963–7984. https://doi.org/10.1007/s13369-022-06738-0.
- Akhtar, M., V. K. Manupati, M. L. R. Varela, G. D. Putnik, A. M. Madureira, and A. Abraham. 2017. “Manufacturing Services Classification in a Decentralized Supply Chain using Text Mining.” In International Conference on Hybrid Intelligent Systems, 186–193. Springer.
- Alizadeh, M., A. Kashef, Y. Wang, J. Wang, G. E. O. Kremer, and J. Ma. 2023. “Circular Economy Conceptualization Using Text Mining Analysis.” Sustainable Production and Consumption 35: 643–654. https://doi.org/10.1016/j.spc.2022.12.016.
- Allison, R. S., J. M. Johnston, G. Craig, and S. Jennings. 2016. “Airborne Optical and Thermal Remote Sensing for Wildfire Detection and Monitoring.” Sensors 16 (8): 1310. https://doi.org/10.3390/s16081310.
- Altawy, R., and A. M. Youssef. 2016. “Security, Privacy, and Safety Aspects of Civilian Drones: A Survey.” ACM Transactions on Cyber-Physical Systems 1 (2): 1–25. https://doi.org/10.1145/3001836.
- Ardolino, M., A. Bacchetti, A. Dolgui, G. Franchini, D. Ivanov, and A. Nair. 2024. “The Impacts of Digital Technologies on Coping with the Covid-19 Pandemic in the Manufacturing Industry: A Systematic Literature Review.” International Journal of Production Research 62 (5): 1–24.
- Arun, R., V. Suresh, C. Veni Madhavan, and M. Narasimha Murthy. 2010. “On Finding the Natural Number of Topics with Latent Dirichlet Allocation: Some Observations.” In Advances in Knowledge Discovery and Data Mining: 14th Pacific-Asia Conference, PAKDD 2010, Hyderabad, India, June 21–24, 2010. Proceedings. Part I 14, 391–402. Springer.
- Bagherzadeh, S., S. Shokouhyar, H. Jahani, and M. Sigala. 2021. “A Generalizable Sentiment Analysis Method for Creating a Hotel Dictionary: Using Big Data on Tripadvisor Hotel Reviews.” Journal of Hospitality and Tourism Technology 12 (2): 210–238. https://doi.org/10.1108/JHTT-02-2020-0034.
- Bhatt, K., A. Pourmand, and N. Sikka. 2018. “Targeted Applications of Unmanned Aerial Vehicles (Drones) in Telemedicine.” Telemedicine and E-Health 24 (11): 833–838. https://doi.org/10.1089/tmj.2017.0289.
- Bodrunova, S. S., I. S. Blekanov, and M. Kukarkin. 2019. “Topic Modeling for Twitter Discussions: Model Selection and Quality Assessment.” In 6th SWS International Scientific Conferences on Social Sciences 2019, 207–214.
- Bogyrbayeva, A., T. Yoon, H. Ko, S. Lim, H. Yun, and C. Kwon. 2023. “A Deep Reinforcement Learning Approach for Solving the Traveling Salesman Problem with Drone.” Transportation Research Part C: Emerging Technologies 148: 103981. https://doi.org/10.1016/j.trc.2022.103981.
- Bravo, R., and A. Leiras. 2015. “Literature Review of the Application of UAVs in Humanitarian Relief.” In Proceedings of the XXXV Encontro Nacional de Engenharia de Producao, Fortaleza, CE, 13–16.
- Büyüközkan, G., and F. Göçer. 2018. “Digital Supply Chain: Literature Review and a Proposed Framework for Future Research.” Computers in Industry 97: 157–177. https://doi.org/10.1016/j.compind.2018.02.010.
- Cao, J., Y. Lu, and J. Xu. 2018. “Topic Modeling for Customer Review Mining: A Comparative Study.” In IEEE International Conference on Big Data (Big Data), 3796–3804. The Westin Seattle, Seattle: IEEE.
- Cao, J., T. Xia, J. Li, Y. Zhang, and S. Tang. 2009. “A Density-Based Method for Adaptive LDA Model Selection.” Neurocomputing 72 (7-9): 1775–1781. https://doi.org/10.1016/j.neucom.2008.06.011.
- Cha, H., D. Kim, J. Eun, and T. Cheong. 2023. “Collaborative Traveling Salesman Problem with Ground Vehicle as a Charger for Unmanned Aerial Vehicle.” Transportation Letters 15 (7): 707–721. https://doi.org/10.1080/19427867.2022.2082006.
- Chung, S. H., B. Sah, and J. Lee. 2020. “Optimization for Drone and Drone-Truck Combined Operations: A Review of the State of the Art and Future Directions.” Computers & Operations Research 123: 105004.
- Deveaud, R., E. SanJuan, and P. Bellot. 2014. “Accurate and Effective Latent Concept Modeling for Ad Hoc Information Retrieval.” Document Numérique 17 (1): 61–84. https://doi.org/10.3166/dn.17.1.61-84.
- Dorling, K., J. Heinrichs, G. G. Messier, and S. Magierowski. 2016. “Vehicle Routing Problems for Drone Delivery.” IEEE Transactions on Systems, Man, and Cybernetics: Systems 47 (1): 70–85. https://doi.org/10.1109/TSMC.2016.2582745.
- Drone-Delivery. 2023. “Drone Delivery is About to Revolutionize the Supply Chain Industry.” Visited on June 28, 2023. https://redstagfulfillment.com/drone-delivery-is-about-to-revolutionize-the-supply-chain-industry/.
- Drones&Logistics. 2015. “Drones – A View into the Future for the Logistics Sector?” http://www.oliverwyman.com/content/dam/marsh/Documents/PDF/UK-en/Drones A View into the Future for the Logistics Sector-10-2015.pdf.
- Drones&Mining. 2019. “Five Trends Influencing Drone Development in the Mining Industry.” https://www.nsenergybusiness.com/features/drones-in-mining-trends/.
- Drones&SC. 2023. “Drones – A “Buzz” Kill to Supply Chain Optimization?” Visited on June 25, 2023. https://www.fronetics.com/drones-buzz-kill-supply-chain-optimization/.
- Evansa, J. R., B. J. H. Copenhaver, and H. A. T. Nguyen. 2019. “Discovering Topics in Healthcare Claims Data using Latent Dirichlet Allocation.” In IEEE International Conference on Big Data (Big Data), 1909–1918. Los Angeles, CA: IEEE.
- Floreano, D., and R. J. Wood. 2015. “Science, Technology and the Future of Small Autonomous Drones.” Nature 521: 460–466. https://doi.org/10.1038/nature14542.
- Ghelichi, Z., M. Gentili, and P. B. Mirchandani. 2022. “Drone Logistics for Uncertain Demand of Disaster-Impacted Populations.” Transportation Research Part C: Emerging Technologies 141: 103735. https://doi.org/10.1016/j.trc.2022.103735.
- Griffiths, T. L., and M. Steyvers. 2004. “Finding Scientific Topics.” Proceedings of the National Academy of Sciences 101 (suppl_1): 5228–5235. https://doi.org/10.1073/pnas.0307752101.
- Gupta, L., R. Jain, and G. Vaszkun. 2016. “Survey of Important Issues in UAV Communication Networks.” IEEE Communications Surveys and Tutorials 18 (2): 1123–1152. https://doi.org/10.1109/COMST.2015.2495297.
- Hamdi, I. 2024. “Solving the Cumulative Capacitated Vehicle Routing Problem with Drones.” Journal of Industrial and Production Engineering 1–18.
- Hayat, S., E. Yanmaz, and R. Muzaffar. 2016. “Survey on Unmanned Aerial Vehicle Networks for Civil Applications: A Communications Viewpoint.” IEEE Communications Surveys and Tutorials 18 (4): 2624–2661. https://doi.org/10.1109/COMST.2016.2560343.
- Hildmann, H., and E. Kovacs. 2019. “Using Unmanned Aerial Vehicles (UAVs) As Mobile Sensing Platforms (MSPs) for Disaster Response, Civil Security and Public Safety.” Drones 3 (3): 59. https://doi.org/10.3390/drones3030059.
- Hyojung, K., C. Inho, and P. Minjung. 2022. “Analyzing Genderless Fashion Trends of Consumers' Perceptions on Social Media: Using Unstructured Big Data Analysis Through Latent Dirichlet Allocation-Based Topic Modeling.” Fashion and Textiles 9 (1): 21. https://doi.org/10.1186/s40691-022-00290-z.
- Indeed. 2023. “9 Jobs in the Drone Industry (With Salaries and Primary Duties).” Visited on June 28, 2023. https://www.indeed.com/career-advice/finding-a-job/jobs-in-the-drone-industry.
- Israr, A., Z. A. Ali, E. H. Alkhammash, and J. J. Jussila. 2022. “Optimization Methods Applied to Motion Planning of Unmanned Aerial Vehicles: A Review.” Drones 6 (5): 126. https://doi.org/10.3390/drones6050126.
- Jahani, H., B. Abbasi, J. B. Sheu, and W. Klibi. 2023. “Supply Chain Network Design with Financial Considerations: A Comprehensive Review.” European Journal of Operational Research 312 (3): 799–839.
- Jahani, H., R. Jain, and D. Ivanov. 2023. “Data Science and Big Data Analytics: A Systematic Review of Methodologies Used in the Supply Chain and Logistics Research.” Annals of Operations Research 1–58.
- Javier, I. 2012. “Usability Assessment of Drone Technology as Safety Inspection Tools.” Information Technology in Construction 14: 194.
- Jelodar, H., Y. Wang, C. Yuan, X. Feng, X. Jiang, Y. Li, and L. Zhao. 2019. “Latent Dirichlet Allocation (LDA) and Topic Modeling: Models, Applications, a Survey.” Multimedia Tools and Applications 78 (11): 15169–15211. https://doi.org/10.1007/s11042-018-6894-4.
- Kolter, M., S. D. Eksioglu, S. N. Pinkley, and R. A. Proano. 2022. “Designing Drone Delivery Networks for Vaccine Supply Chain: A Case Study of Niger.” Preprint. arXiv:2208.04357.
- Lagkas, T., V. Argyriou, S. Bibi, and P. Sarigiannidis. 2018. “UAV IoT Framework Views and Challenges: Towards Protecting Drones As “things”.” Sensors 18 (11): 4015. https://doi.org/10.3390/s18114015.
- Lagorio, A., R. Pinto, and R. Golini. 2016. “Research in Urban Logistics: A Systematic Literature Review.” International Journal of Physical Distribution & Logistics Management 46 (10): 908–931.
- Lee, S., and Y. Choi. 2016. “Reviews of Unmanned Aerial Vehicle (Drone) Technology Trends and Its Applications in the Mining Industry.” Geosystem Engineering 19 (4): 197–204. https://doi.org/10.1080/12269328.2016.1162115.
- Liu, Y. 2023. “Routing Battery-Constrained Delivery Drones in a Depot Network: A Business Model and Its Optimization–Simulation Assessment.” Transportation Research Part C: Emerging Technologies 152: 104147. https://doi.org/10.1016/j.trc.2023.104147.
- Luo, Z., M. Poon, Z. Zhang, Z. Liu, and A. Lim. 2021. “The Multi-Visit Traveling Salesman Problem with Multi-Drones.” Transportation Research Part C: Emerging Technologies 128: 103172.
- Macrina, G., L. D. P. Pugliese, F. Guerriero, and G. Laporte. 2020. “Drone-Aided Routing: A Literature Review.” Transportation Research Part C: Emerging Technologies 120: 102762. https://doi.org/10.1016/j.trc.2020.102762.
- Mohsan, S. A. H., N. Q. H. Othman, Y. Li, M. H. Alsharif, and M. A. Khan. 2023. “Unmanned Aerial Vehicles (UAVs): Practical Aspects, Applications, Open Challenges, Security Issues, and Future Trends.” Intelligent Service Robotics 16 (1): 109–137.
- Mora, P., and C. A. S. Araujo. 2022. “Delivering Blood Components through Drones: A Lean Approach to the Blood Supply Chain.” Supply Chain Forum: An International Journal 23 (2): 113–123.
- Mourtzis, D., J. Angelopoulos, and N. Panopoulos. 2024. “Unmanned Aerial Vehicle (UAV) Path Planning and Control Assisted by Augmented Reality (AR): The Case of Indoor Drones.” International Journal of Production Research 62 (9): 3361–3382. https://doi.org/10.1080/00207543.2023.2232470.
- Mozaffari, M., W. Saad, M. Bennis, Y. H. Nam, and M. Debbah. 2019. “A Tutorial on UAVs for Wireless Networks: Applications, Challenges, and Open Problems.” IEEE Communications Surveys & Tutorials 21 (3): 2334–2360. https://doi.org/10.1109/COMST.2019.2902862.
- Murray, C. C., and A. G. Chu. 2015. “The Flying Sidekick Traveling Salesman Problem: Optimization of Drone-Assisted Parcel Delivery.” Transportation Research Part C: Emerging Technologies 54: 86–109. https://doi.org/10.1016/j.trc.2015.03.005.
- Natu, A. S., and S. Kulkarni. 2016. “Adoption and Utilization of Drones for Advanced Precision Farming: A Review.” International Journal on Recent and Innovation Trends in Computing and Communication 4: 563–565.
- Nezafati, Navid, and Mohammad Reza Sheikhattar. 2022. “Modeling the Impact of the Covid-19 Risks on Global Supply Chains using Text Mining Methods: A Case Study of the Food Supply Chain.” Journal of Industrial Management Perspective 12 (3): 141–172.
- NicolePontius. 2020. “What is Supply Chain Logistics? A Definition of and Analysis of the Challenges and Benefits of Supply Chain Logistics.” https://www.camcode.com/asset-tags/what-is-supply-chain-logistics/.
- Otto, A., N. Agatz, J. Campbell, B. Golden, and E. Pesch. 2018. “Optimization Approaches for Civil Applications of Unmanned Aerial Vehicles (UAVs) Or Aerial Drones: A Survey.” Networks 72 (4): 411–458. https://doi.org/10.1002/net.v72.4.
- Ozkan, O., and O. Atli. 2021. “Transporting Covid-19 Testing Specimens by Routing Unmanned Aerial Vehicles with Range and Payload Constraints: The Case of Istanbul.” Transportation Letters 13 (5-6): 482–491. https://doi.org/10.1080/19427867.2021.1896063.
- Pachayappan, M., and B. Sundarakani. 2023. “Drone Delivery Logistics Model for on-Demand Hyperlocal Market.” International Journal of Logistics Research and Applications 26 (12): 1728–1760. https://doi.org/10.1080/13675567.2022.2107189.
- Pei, Z., W. Pan, K. Weng, and T. Fang. 2024. “A Branch-And-Price-And-Cut Algorithm for the Unmanned Aerial Vehicle Delivery with Battery Swapping.” International Journal of Production Research 1–26. https://doi.org/10.1080/00207543.2024.2318017.
- Pejić Bach, M., Ž. Krstić, S. Seljan, and L. Turulja. 2019. “Text Mining for Big Data Analysis in Financial Sector: A Literature Review.” Sustainability 11 (5): 1277.
- Pinto, R., and A. Lagorio. 2022. “Point-To-Point Drone-Based Delivery Network Design with Intermediate Charging Stations.” Transportation Research Part C: Emerging Technologies 135: 103506. https://doi.org/10.1016/j.trc.2021.103506.
- Rejeb, A., K. Rejeb, S. J. Simske, and H. Treiblmaier. 2023. “Drones for Supply Chain Management and Logistics: A Review and Research Agenda.” International Journal of Logistics Research and Applications 26 (6): 708–731. https://doi.org/10.1080/13675567.2021.1981273.
- Rosser, J. C., Jr., V. Vignesh, B. A. Terwilliger, and B. C. Parker. 2018. “Surgical and Medical Applications of Drones: A Comprehensive Review.” JSLS: Journal of the Society of Laparoendoscopic Surgeons 22 (3): e2018.00018. https://doi.org/10.4293/JSLS.2018.00018.
- Rouse, M. 2019. “Drone,(UAV).” https://internetofthingsagenda.techtarget.com/definition/drone.
- Sah, B., R. Gupta, and D. Bani-Hani. 2021. “Analysis of Barriers to Implement Drone Logistics.” International Journal of Logistics Research and Applications 24 (6): 531–550. https://doi.org/10.1080/13675567.2020.1782862.
- Shakhatreh, H., A. H. Sawalmeh, A. Al-Fuqaha, Z. Dou, E. Almaita, I. Khalil, N. S. Othman, A. Khreishah, and M. Guizani. 2019. “Unmanned Aerial Vehicles (UAVs): A Survey on Civil Applications and Key Research Challenges.” IEEE Access 7: 48572–48634. https://doi.org/10.1109/Access.6287639.
- Shen, Y., X. Xu, B. Zou, and H. Wang. 2021. “Operating Policies in Multi-Warehouse Drone Delivery Systems.” International Journal of Production Research 59 (7): 2140–2156. https://doi.org/10.1080/00207543.2020.1756509.
- Singhal, G., B. Bansod, and L. Mathew. 2018. “Unmanned Aerial Vehicle Classification, Applications and Challenges: A Review.” Preprints.
- Tavana, M., A. Shaabani, I. Raeesi Vanani, and R. Kumar Gangadhari. 2022. “A Review of Digital Transformation on Supply Chain Process Management Using Text Mining.” Processes 10 (5): 842. https://doi.org/10.3390/pr10050842.
- Telmo, A. 2017. “Hyperspectral Imaging: A Review on UAV-Based Sensors, Data Processing and Applications for Agriculture and Forestry.” Remote Sensing 9: 9.
- Tolooie, A., A. Sinha, and A. K. Raftar Nouri. 2024. “Heuristic Approach for Optimising Reliable Supply Chain Network Using Drones in Last-Mile Delivery Under Uncertainty.” International Journal of Systems Science: Operations & Logistics 11 (1): 2301610. https://doi.org/10.1080/23302674.2023.2301610.
- Wen, X., and G. Wu. 2022. “Heterogeneous Multi-Drone Routing Problem for Parcel Delivery.” Transportation Research Part C: Emerging Technologies 141: 103763. https://doi.org/10.1016/j.trc.2022.103763.
- Westergaard, D., H. H. Stærfeldt, C. Tønsberg, L. J. Jensen, and S. Brunak. 2018. “A Comprehensive and Quantitative Comparison of Text-Mining in 15 Million Full-Text Articles Versus their Corresponding Abstracts.” PLoS Computational Biology 14 (2): e1005962. https://doi.org/10.1371/journal.pcbi.1005962.
- Wikipedia. 2022. “Zipf's Law.” Visited on September 25, 2020. https://en.wikipedia.org/wiki/Zipf's_law.
- Wu, D., D. W. Rosen, L. Wang, and D. Schaefer. 2015. “Cloud-Based Design and Manufacturing: A New Paradigm in Digital Manufacturing and Design Innovation.” Computer-Aided Design 59: 1–14. https://doi.org/10.1016/j.cad.2014.07.006.
- Xue, J., J. Chen, C. Chen, C. Zheng, S. Li, and T. Zhu. 2020. “Public Discourse and Sentiment During the Covid 19 Pandemic: Using Latent Dirichlet Allocation for Topic Modeling on Twitter.” PloS One 15 (9): e0239441. https://doi.org/10.1371/journal.pone.0239441.
- Zhang, R., and M. Furusho. 2017. “Conversion Timing of Seafarer's Decision-Making for Unmanned Ship Navigation.” TransNav, International Journal on Marine Navigation and Safety od Sea Transportation 11 (3): 463–468.
- Zou, B., S. Wu, Y. Gong, Z. Yuan, and Y. Shi. 2024. “Delivery Network Design of a Locker-Drone Delivery System.” International Journal of Production Research 62 (11): 4097–4121. https://doi.org/10.1080/00207543.2023.2254402.
Appendices
Appendix 1.
Basic terminology
Drones/UAVs: A drone, in technological terms, is an autonomously or semi-autonomously operating unmanned aircraft. Drones are more formally known in academic literature as unmanned aerial vehicles (UAVs), remotely piloted aircraft (RPAs), or unmanned aircraft systems (UASs). Essentially, a drone can be remotely controlled by a human pilot or be fully automated, equipped with onboard sensors and GPS (Rouse Citation2019). Different types of UAVs exhibit varying features based on altitude, speed, and energy consumption, making them suitable for various applications. Drone designs can be broadly categorised into rotary-wing and fixed-wing types. Rotary-wing drones consist of configurations with one rotor (helicopter), three rotors (tricopter), four rotors (quadcopter), six rotors (hexacopter), or eight rotors (octocopter) and are capable of maintaining a stable position. Drones with fixed-wing designs are better suited for high-speed, long-distance travel. Throughout this paper, we will refer to all types of drones and UAVs interchangeably.
Supply Chain: Supply chain refers to a collaborative network of various facilities, encompassing producers, vendors, manufacturers, warehouses, distribution centres, transportation systems, and retailers, working together to meet customer demand for a specific product or service. This chain comprises multiple critical processes, such as the transportation of raw materials to manufacturing facilities for the production of finished goods, as well as the subsequent transportation and distribution of these products to the end customer (Jahani et al. Citation2023; Jahani, Jain, and Ivanov Citation2023).
Logistics: Any attempt involved in transmitting products and services within the supply chain's facilities to reach the consumer (Ghelichi, Gentili, and Mirchandani Citation2022; NicolePontius Citation2020).
Appendix 2.
Word frequencies in content
We investigated the frequency of the words in the content attribute and found that the frequency of unique words varied between 1 and 5326 repetitions with a median of 3 and a mean of 33. Figure illustrates that only 10% of the unique words had a frequency greater than 100. The figure also shows the alignment between word frequency and Zipf's rule (inverse relationship between frequency of words and heir frequency rating) (Wikipedia Citation2022). This figure also shows the position of the top 50 words out of over 10,000 words, using the vertical line. The top 50 words are illustrated as a Wordcloud in Figure . Note that the words are counted after doing the stemming process in data preparation. The figure demonstrates that the words ‘network’, ‘system’, ‘rout’, and ‘delivery’ are the highly frequent words used by authors in the literature. Figure reports the frequency of the words in more details for the top ten words. As can be seen, the first four words (network, system, rout, and deliveri) are being used more than others.
Figure A1. Frequency of words in title, abstract, and keywords.
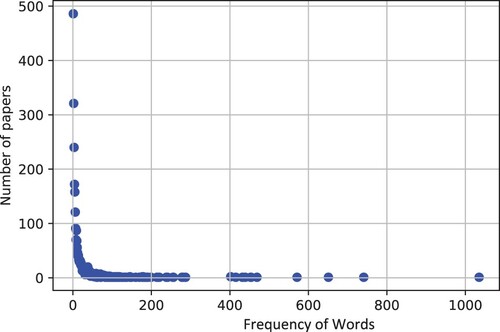
Figure A2. Wordcloud of the top-50 high-frequency words in title, abstract, and keywords.
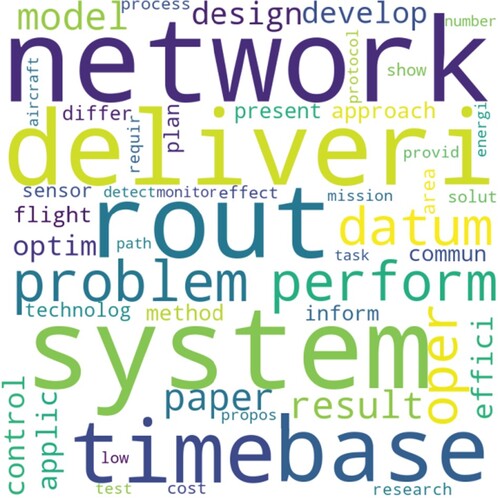
Figure A3. Number of papers that used any of the top-10 high-frequency words in title, abstract, and keywords.
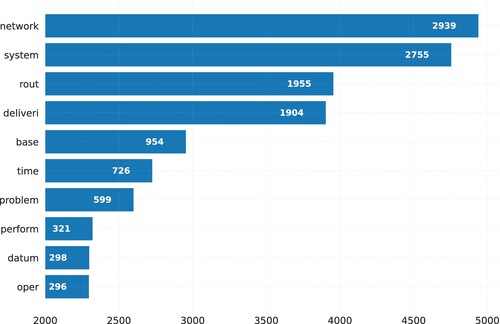