Abstract
Hand tremors may cause some blemishes in precision and stability of a minimally invasive surgery (MIS). To track the tremor signals accurately, there are two main problems left to be settled. First, it is not practical to collect the sample data of tremor in large scale in practical applications. To deal with the hand tremors, a learning method based on small samples sizes and high dimensional input space is needed. Second, the hand tremors have time-varying characteristics. This fact is neglected by traditional learning methods, which could lead to imprecision and instability of a MIS. In this work, a time-sequence-based fuzzy support vector machine adaptive filter (TSF-SVMAF) for tremor cancelling is proposed. The proposed method is based on support vector machine and time series. It is suitable for solving the problem that the inputs are time-varying and the samples are small-scale. To cancel the time-varying hand tremors, different learning-weight-functions are designed for tremor signals with different frequencies. From the simulation results, compared with the existing methods such as back propagation (BP), weighted-frequency Fourier combiner (WFLC) and bandlimited multiple Fourier linear combiner (BMFLC), the proposed method has better performance when learning the time-varying hand tremors with small sample sizes.
Acknowledgements
The authors would like to appreciate the associated editors and the reviewers for the constructive comments and suggestions. This work was supported by the National Natural Science Foundation of China under Project 60974047, U1134004 and 61275001, Natural Science Foundation of Guangdong Province S2012010008967 and Science Fund for Distinguished Young Scholars (S20120011437), 2011Zhujiang New Star, FOK Ying Tung Education Foundation of China 121061, the Ministry of Education of New Century Excellent Talent and the 973 Program of China 2011CB013104, and by the Doctoral Fund of Ministry of Education of China under Grant No. 20124420130001.
Additional information
Notes on contributors
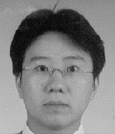
Zhi Liu
Zhi Liu was born in Hunan, China, in 1977. He received BS degree from the Huazhong University of Science and Technology, Wuhan, China; MS degree in Automatic Engineering from Hunan University, Changsha, China and PhD degree in Control Theory and Control Engineering from Tsinghua University, Beijing, China, in 1997, 2000 and 2004, respectively. Currently, he is a Prof. in the Department of Automation, Guangdong University of Technology, Guangzhou, China. His research interests include fuzzy control, robotics, neural networks, robust control and network systems.
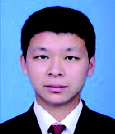
Jing Luo
Jing Luo was born in Hunan, China, in 1988. He received BS degree in Automation from Xiangtan University, Xiangtan, China, in 2011. He is currently pursuing a MS degree in Control Theory and Control Engineering from Guangdong University of Technology, Guangzhou, China. His research interests include robotics and learning control.
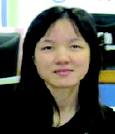
Liyang Wang
Liyang Wang received BS degree in Communication Engineering from Tongji University, Shanghai, China, in 2002, MS degree in Electrical and Communication Engineering from Guangdong University of Technology, Guangzhou, China, in 2007. During 2010–2011, she was a Research Associate in Intelligent Systems Research Institute, Sungkyunkwan University, Korea. She is currently pursuing a PhD degree in Control Theory and Control Engineering from Guangdong University of Technology, Guangzhou, China. Her research interests include robotics and learning control.
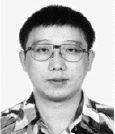
Yun Zhang
Yun Zhang was born in Hunan, China, in 1963. He received BS and MS degrees in Automatic Engineering from Hunan University, Changsha, China, in 1982 and 1986, respectively, and PhD degree in Automatic Engineering from the South China University of Science and Technology, Guangzhou, China, in 1998. He is currently a Prof. with the Department of Automation, Guangdong University of Technology, Guangzhou, China. His research interests include intelligent control systems, network systems and signal processing.
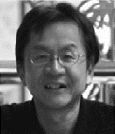
C. L. Philip Chen
C. L. Philip Chen (S’87–M’88–SM’93–F’07) received MS degree from the University of Michigan, Ann Arbor, MI, USA, in 1985, and PhD degree from Purdue University, West Lafayette, IN, USA, in 1988. He is currently a Dean and Chair Prof. with the Faculty of Science and Technology, University of Macau, Macau, China. He is also a Prof. and the Chair of the Department of Electrical and Computer Engineering and an Associate Dean for Research and Graduate Studies of the College of Engineering with the University of Texas at San Antonio, San Antonio, TX, USA.
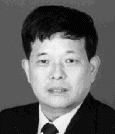
Xin Chen
Xin Chen received BS degree in Mechanical Engineering from Changsha Railway Institute, Hunan, China, in 1982, MS degree in Mechanical Engineering from the Harbin Institute of Technology, Heilongjiang, China, in 1988, and PhD degree in Mechanical Engineering from the Huazhong University of Science and Technology, Wuhan, China, in 1995. He is currently a Prof. with the Department of Mechatronics Engineering, Guangdong University of Technology, Guangzhou, China. His research interests include mechatronics and robotics.