Abstract
This paper studies the mean square consensus of discrete-time linear time-invariant multi-agent systems with communication noises. A distributed consensus protocol, which is composed of the agent's own state feedback and the relative states between the agent and its neighbours, is proposed. A time-varying consensus gain a[k] is applied to attenuate the effect of noises which inherits in the inaccurate measurement of relative states with neighbours. A polynomial, namely ‘parameter polynomial’, is constructed. And its coefficients are the parameters in the feedback gain vector of the proposed protocol. It turns out that the parameter polynomial plays an important role in guaranteeing the consensus of linear multi-agent systems. By the proposed protocol, necessary and sufficient conditions for mean square consensus are presented under different topology conditions: (1) if the communication topology graph has a spanning tree and every node in the graph has at least one parent node, then the mean square consensus can be achieved if and only if ∑∞k = 0a[k] = ∞, ∑∞k = 0a2[k] < ∞ and all roots of the parameter polynomial are in the unit circle; (2) if the communication topology graph has a spanning tree and there exits one node without any parent node (the leader–follower case), then the mean square consensus can be achieved if and only if ∑∞k = 0a[k] = ∞, limk → ∞a[k] = 0 and all roots of the parameter polynomial are in the unit circle; (3) if the communication topology graph does not have a spanning tree, then the mean square consensus can never be achieved. Finally, one simulation example on the multiple aircrafts system is provided to validate the theoretical analysis.
Additional information
Funding
Notes on contributors
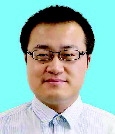
Yunpeng Wang
Yunpeng Wang received the BS degree in information and computing science from Shandong University, China, in July 2010. He is currently working toward the PhD degree in control theory and control engineering at the State Key Laboratory of Management and Control for Complex Systems, Institute of Automation, Chinese Academy of Sciences. He is also with University of Chinese Academy of Sciences, Beijing. His current research interests include MASs, human–robot interaction and brain–computer interface.
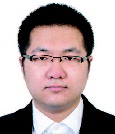
Long Cheng
Long Cheng received the BS degree (with honours) in control engineering from Nankai University, Tianjin, China, in July 2004 and the PhD degree (with honours) in control theory and control engineering from the Institute of Automation, Chinese Academy of Sciences, Beijing, China, in July 2009. Currently, he is an Associate Professor at the State Key Laboratory of Management and Control for Complex Systems, Institute of Automation, Chinese Academy of Sciences. His current research interests include coordination of MASs, neural networks, optimisation and their applications to robotics.
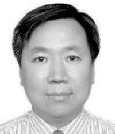
Zeng-Guang Hou
Zeng-Guang Hou received the BE and ME degrees in electrical engineering from Yanshan University (formerly North-East Heavy Machinery Institute), Qinhuangdao, China, in 1991 and 1993, respectively, and the PhD degree in electrical engineering from the Beijing Institute of Technology, Beijing, China, in 1997. He is now a Professor in the State Key Laboratory of Management and Control for Complex Systems, Institute of Automation, Chinese Academy of Sciences. His current research interests include neural networks, optimisation algorithms, robotics and intelligent control systems.
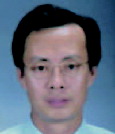
Min Tan
Min Tan received the BS degree in control engineering from Tsinghua University, Beijing, China, in 1986 and the PhD degree in control theory and control engineering from the Institute of Automation, Chinese Academy of Sciences, Beijing, in 1990. He is a Professor in the State Key Laboratory of Management and Control for Complex Systems, Institute of Automation, Chinese Academy of Sciences. His research interests include advanced robot control, multi-robot systems, biomimetic robots and manufacturing systems.
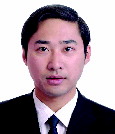
Chao Zhou
Chao Zhou received the BS degree (Hons.) in Automation from Southeast University, Nanjing, China, in July 2003, and the PhD degree in control theory and control engineering from the Institute of Automation, Chinese Academy of Sciences, Beijing, China, in 2008. Since July 2008, he has been an Assistant Professor in the Key Laboratory of Complex Systems and Intelligent Science, Institute of Automation, Chinese Academy of Sciences, where he has been an Associate Professor since October 2011. His current research interests include the motion control of robot, the bio-inspired robotic fish and embedded system of robot.
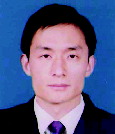
Ming Wang
Ming Wang received the BE degree in automation from Shandong Institute of Mining and Technology, Taishan, China, in 1998, and the ME and PhD degrees in control theory and control engineering from Shandong University, Jinan, China, and the Institute of Automation, Chinese Academy of Sciences, Beijing, China, in 2004 and 2010, respectively. He is currently an Associate Professor with the School of Information and Electrical Engineering, Shandong Jianzhu University, Jinan, China. His research interests include intelligent mechatronics, robotics and embedded system.