ABSTRACT
This paper presents an approximation-based nonlinear disturbance observer (NDO) methodology for adaptive tracking of uncertain pure-feedback nonlinear systems with unmatched external disturbances. Compared with existing control results using NDO for nonlinear systems in lower-triangular form, the major contribution of this study is to develop an NDO-based control framework in the presence of non-affine nonlinearities and disturbances unmatched in the control input. An approximation-based NDO scheme is designed to attenuate the effect of compounded disturbance terms consisting of external disturbances, approximation errors and control coefficient nonlinearities. The function approximation technique using neural networks is employed to estimate the unknown nonlinearities derived from the recursive design procedure. Based on the designed NDO scheme, an adaptive dynamic surface control system is constructed to ensure that all signals of the closed-loop system are semi-globally uniformly ultimately bounded and the tracking error converges to a neighbourhood of the origin. Simulation examples including a mechanical system are provided to show the effectiveness of the proposed theoretical result.
Acknowledgments
This research was supported by the MSIP (Ministry of Science, ICT and Future Planning), Korea, under the ITRC (Information Technology Research Center) support program (IITP-2016-H8501-16-1018) supervised by the IITP (Institute for Information & communications Technology Promotion), by the Human Resources Development (No.20154030200860) of the Korea Institute of Energy Technology Evaluation and Planning (KETEP) grant funded by the Korea government Ministry of Trade, Industry and Energy, and by Basic Science Research Program through the National Research Foundation of Korea (NRF) funded by the Ministry of Education (NRF-2016R1D1A1B03931312).
Disclosure statement
No potential conflict of interest was reported by the authors.
Additional information
Notes on contributors
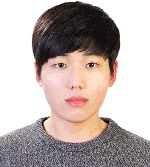
Hyoung Oh Kim
Hyoung Oh Kim received his B.S. degree from the School of Electrical and Electronics Engineering, Chung-Ang University, Seoul, Korea, in 2016, where he is currently pursuing the Master degree with the Department of Electrical and Electronic Engineering. His current research interests include nonlinear control, nonlinear disturbance observer, adaptive control using fuzzy logic systems and neural networks.
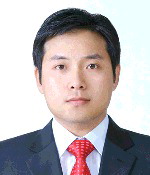
Sung Jin Yoo
Sung Jin Yoo received his B.S., M.S., and Ph.D. degrees in Electrical and Electronic Engineering from Yonsei University, Seoul, South Korea, in 2003, 2005, and 2009 respectively. He has been a Post-doctoral researcher in the Department of Mechanical Science and Engineering, University of Illinois at Urbana-Champaign, Illinois, from 2009 to 2010. Since 2011, he has been with the School of Electrical and Electronics Engineering, Chung-Ang University, Seoul, South Korea, where he is currently an Associate Professor. His research interests include nonlinear adaptive control, decentralized control, distributed control, fault tolerant control, and neural networks theories, and their applications to robotic, flight, nonlinear time-delay systems, large-scale systems, and multi-agent systems.