ABSTRACT
In this research, we propose a preference-guided optimisation algorithm for multi-criteria decision-making (MCDM) problems with interval-valued fuzzy preferences. The interval-valued fuzzy preferences are decomposed into a series of precise and evenly distributed preference-vectors (reference directions) regarding the objectives to be optimised on the basis of uniform design strategy firstly. Then the preference information is further incorporated into the preference-vectors based on the boundary intersection approach, meanwhile, the MCDM problem with interval-valued fuzzy preferences is reformulated into a series of single-objective optimisation sub-problems (each sub-problem corresponds to a decomposed preference-vector). Finally, a preference-guided optimisation algorithm based on MOEA/D (multi-objective evolutionary algorithm based on decomposition) is proposed to solve the sub-problems in a single run. The proposed algorithm incorporates the preference-vectors within the optimisation process for guiding the search procedure towards a more promising subset of the efficient solutions matching the interval-valued fuzzy preferences. In particular, lots of test instances and an engineering application are employed to validate the performance of the proposed algorithm, and the results demonstrate the effectiveness and feasibility of the algorithm.
Disclosure statement
No potential conflict of interest was reported by the authors.
Additional information
Funding
Notes on contributors
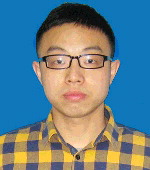
Bin Luo
Bin Luo received the M.E. degrees in the School of Mechatronics Engineering from Harbin Institute of Technology, Harbin, China, in 2014. He is currently working towards the PhD degree in the School of Mechatronics Enegineering in Harbin Institute of Technology. His research interests include Multi-objective optimization and maintenance decision-making.
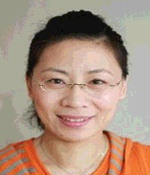
Lin Lin
Lin Lin received the PhD degree in Mechanical Design from Harbin Institute of Technology, Harbin, China, in 2013. She is currently a professor in the School of Mechatronics Engineering in Harbin Institute of Technology. Her research interests include time series prediction, neural networks, Multi-objective optimization and maintenance decision-making.
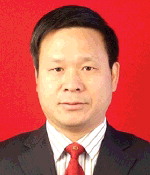
ShiSheng Zhong
Shisheng Zhong is a Professor of Mechanical Engineering at Harbin Institute of Technology, China. He received the PhD degree in Mechanical Engineering in 1995 from Huazhong University of Science and Technology, China. His research interests include neural networks, prognostics and health management (PHM).