Abstract
This paper investigates the sensor fault estimation problem in finite-frequency domain for nonlinear time-delayed systems via T–S fuzzy approach with local nonlinear models. First, to estimate the fault, an augmented system is constructed, where the auxiliary state vector consists of the states of the system and the auxiliary filter. Second, an unknown input observer with finite-frequency specifications is designed to achieve fault estimation. Then, by utilising the Parseval's theorem, the sufficient conditions of the presented observer are established. Different from some existing methods, the state estimation error dynamics are decoupled from the local nonlinear dynamics via the designed observer such that the observer synthesis is simplified and the conservatism can be reduced. Meanwhile, compared with some conventional methods in the entire-frequency domain, a less restrictive analysis condition could be derived by the developed observer scheme. Finally, simulation results are given to illustrate the merit of the proposed approach.
Disclosure statement
No potential conflict of interest was reported by the authors.
Additional information
Notes on contributors
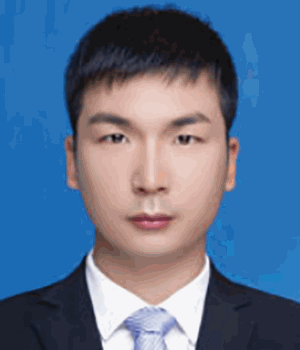
Yue Wu
Yue Wu received the M.S. degree in control science and engineering from Dalian Maritime University, Dalian, China, in 2015, respectively. He is currently pursuing the Ph.D. degree in navigation guidance and control from Northeastern University, Shenyang, China. His current research interests include fault tolerant control, fault detection and isolation, adaptive control and cyber-physical system security.
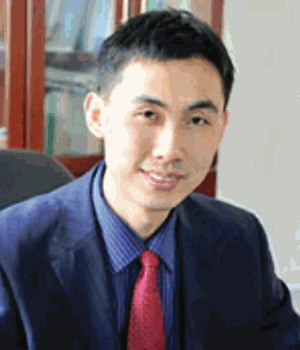
Jiuxiang Dong
Jiuxiang Dong received the B.S. degree in mathematics and applied mathematics, the M.S. degree in applied mathematics from Liaoning Normal University, China, in 2001 and 2004, respectively. He received the Ph.D. degree in navigation guidance and control from Northeastern University, China in 2009. He is currently a Professor at the College of Information Science and Engineering, Northeastern University. His research interests include fuzzy control, robust control and reliable control. Dr. Dong is an Associate Editor for the International Journal of Control, Automation, and Systems (IJCAS).
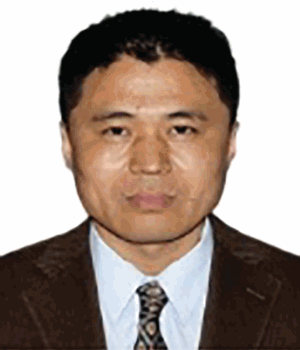
Tieshan Li
Tieshan Li received the B.S. degree in ocean fisheries engineering from the Ocean University of China, Qingdao, China, in 1992 and the Ph.D. degree in vehicle operation engineering from Dalian Maritime University (DMU), Dalian, China, in 2005. He was a Lecturer with DMU from 2005 to 2006, where he was an Associate Professor from 2006 to 2011, and has been a Ph.D. Supervisor since 2009 and a Full Professor since 2011. From 2007 to 2010, he was a Post-Doctoral Scholar with the School of Naval Architecture, Ocean and Civil Engineering, Shanghai Jiao Tong University, Shanghai, China. From 2008 to 2009, and from 2014 to 2015, he visited the City University of Hong Kong, Hong Kong, as a Senior Research Associate. Since 2013, he has been visiting the University of Macau, Macau, China, as a Visiting Scholar for many times. His current research interests include intelligent learning and control for nonlinear systems, and multiagent systems and their applications to marine vehicle control.