Abstract
This paper studies the sampled-data state-estimation problem of delayed complex-valued neural networks (CVNNs). By using Lyapunov–Krasovskii functional (LKF), standard integral inequality together with the reciprocal convex approach, a delay-dependent condition is established in terms of the solution to linear matrix inequalities (LMIs) such that the consider CVNNs is asymptotically stable. As a result, an estimator gain matrix can be obtained through sampling instant. Finally, a simulation example is given to illustrate the theoretical analysis.
Disclosure statement
No potential conflict of interest was reported by the authors.
Additional information
Notes on contributors
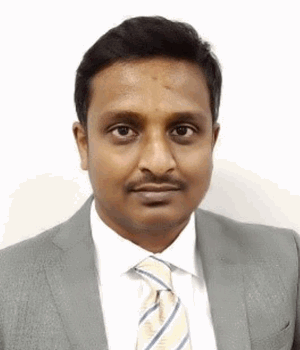
Nallappan Gunasekaran
Dr. Nallappan Gunasekaran was born in 1987. He received the bachelor degree from the Mahendra Arts and Science College, Namakkal affiliated to Periyar University, Salem, Tamil Nadu, India, in 2009. He received his post-graduation in mathematics from Jamal Mohamed College affiliated to Bharathidasan University, Trichy, Tamil Nadu, India, in 2012. He was awarded Master of Philosophy in 2013 in the field of mathematics with a specialized area of cryptography from Bharathidasan University, Trichy, India, and the Ph.D. degree from Thiruvalluvar University, Vellore, India, in 2017, in mathematics. He was a Junior Research Fellow with the Department of Science and Technology- Science and Engineering Research Board (DST-SERB), Government of India, New Delhi, India. He was a Post-Doctoral Research Fellow in the Research Center for Wind Energy Systems, Kunsan National University, Gunsan, South Korea, from 2017 to 2018. Currently, he is working as a Post-Doctoral Research Fellow in Department of Mathematical Sciences, Shibaura Institute of Technology, Saitama, Japan. His research interests are complex-valued neural networks, complex dynamical networks, control theory, sampled-data control, multi-agent systems, T-S fuzzy theory, and cryptography, etc. Dr Gunasekaran serves as a reviewer for various SCI journals. He has authored and co-authored of more than 25 research articles in various SCI journals.
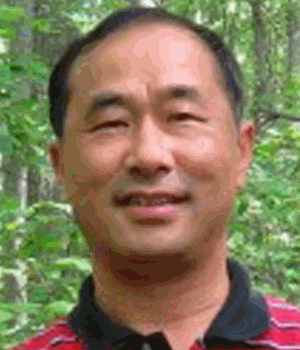
Guisheng Zhai
Dr. Guisheng Zhai received his B.S. degree from Fudan University, China, in 1988, and he received his M.E. and Ph.D. degrees, both in system science, from Kobe University, Japan, in 1993 and 1996, respectively. After two years of industrial experience, Dr. Zhai moved to Wakayama University, Japan, in 1998, and then to Osaka Prefecture University, Japan, in 2004. He held visiting professor positions at University of Notre Dame from August 2001 to July 2002, and at Purdue University from March 2016 through February 2017. In April 2010, he joined the faculty board of Shibaura Institute of Technology, Japan, where he currently is a full Professor of Mathematical Sciences. His research interests include large scale and decentralized control systems, robust control, switched systems and switching control, networked control and multi-agent systems, neural networks and signal processing, etc. Dr Zhai is on the editorial board of several academic journals including IET Control Theory & Applications, International Journal of Applied Mathematics and Computer Science, Journal of Control and Decision, and Frontiers of Mechanical Engineering. He is a Senior Member of IEEE, a member of ISCIE, SICE, JSST and JSME.