ABSTRACT
This paper addresses a practical finite-time leader-following formation controller design for the second-order stochastic Lipchitz nonlinear systems under uncertain communication environments and external disturbances. It is desired that the orientation of the formation change according to the variations in the leader’s orientation. The time-varying weighted topology matrix is modelled as a linear combination of a finite number of constant Laplacian matrices with time-varying coefficients. The proposed back-stepping sliding-mode controller guarantees that all the signals in the closed-loop system remain bounded in probability and the norm of sliding trajectories converge almost surely in finite-time to an arbitrary small neighbourhood of origin, which can be called almost-surely practical finite-time formation. The results are then modified for solving the consensus problem as well. In the simulation section, a stochastic multi-aircraft model, as well as a numerical example, are used to illustrate the effectiveness of the proposed design methods.
Disclosure statement
No potential conflict of interest was reported by the authors.
Additional information
Notes on contributors
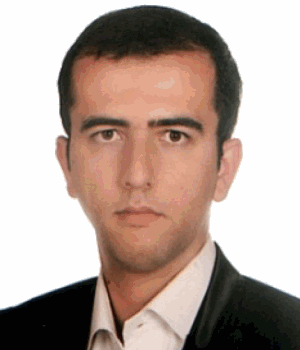
Mahdi Siavash
Mahdi Siavash received his B.Sc. in control engineering from K. N. Toosi University of Technology, Tehran, Iran in 2012. Then he received his M.Sc. degree in control engineering from Isfahan University of Technology, Isfahan, Iran in 2014. He is currently pursuing the Ph.D. degree in control engineering at Tarbiat Modares University, Tehran, Iran. He is also a research assistant at intelligent control systems laboratory in Tarbiat Modares University. His research interests include multi-agent systems, stochastic systems and switching systems control.
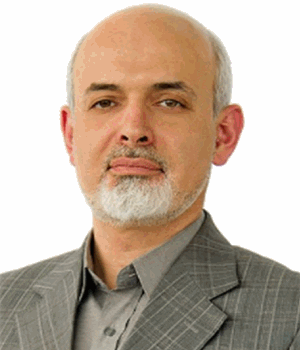
Vahid Johari Majd
Vahid Johari Majd received his B.Sc. degree in 1989 from the electrical engineering department of the University of Tehran, Iran. He then received his M.Sc. and Ph.D. degrees in the area of Control Theory from the electrical engineering department of the University of Pittsburgh, PA, U.S.A. in 1991 and 1995, respectively. He is currently an associate professor in the control system department of Tarbiat Modares University, Tehran, Iran, and is the director of intelligent control systems laboratory. His areas of interest include: Intelligent identification and control, multi-agent learning, fuzzy control, cooperative control, formation control, robust nonlinear control, and fractional order control.
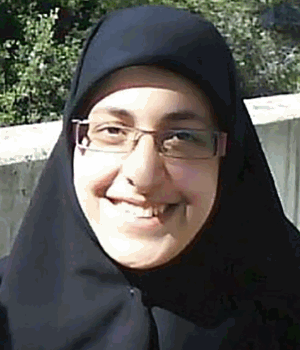
Mahdie Tahmasebi
Mahdieh Tahmasebi received the B.Sc. degree in applied mathematics from University of Shahid Beheshti, Tehran, Iran in 2002 and the M.Sc. and Ph.D. degrees in mathematics from Sharif University of Technology in 2005, 2010, respectively. From 2009 to 2010, she was a Research Assistant with INRIA Institute, Sophia Antipolis, France. Her research interests include Malliavin calculus, stochastic control, financial mathematics, numerical analysis of SDEs, stochastic analysis, and ordinary and partial stochastic differential equations. Dr Tahmasebi is currently an assistant professor at the department of applied mathematics, school of mathematical sciences, Tarbiat Modares University, Tehran, Iran.