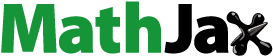
Abstract
Tracking control of general dynamical systems in high-order fully actuated (HOFA) system representation is solved. For the case of tracking a constant or slow time-varying signal in the presence of constant or slow time-varying disturbances, a generalised PID control scheme is proposed, which realises asymptotical tracking to a prescribed signal and also guarantees that the state derivatives of certain orders converge to the origin. For the case that the signal to be tracked is generated by a reference model, a model reference tracking (MRT) controller is presented, which relies on the solution to a type of generalised Sylvester matrix equations and guarantees the desired asymptotical tracking requirement. Due to the full-actuation property of the HOFA models, closed-loop systems under both control schemes are constant and linear. Furthermore, based on a general parametric solution to the type of Sylvester matrix equations and a general parametric eigenstructure assignment result, simple and complete parameterisation of the two types of control designs are provided, and feasibility conditions in terms of the system initial values for sub-fully actuated systems are also derived. An illustrative example is presented to demonstrate the application of the proposed approaches and their effects.
1. Introduction
1.1. About PID control
PID controller exhibits a lasting vitality in the domain of automatic control due to its simple structure and ease of use. It dates back to 1910 with the first PID controller developed by Elmer Sperry for the U.S. Navy (Ang et al., Citation2005; Borase et al., Citation2020), and the Ziegler–Nichols rule (Ziegler & Nichols, Citation1942, Citation1943) is one of the most prominent method to choose controller parameters which is seen as the most challenging work for PID controller design.
Most of existing works of PID control focus on linear systems (e.g. Åström & Hägglund, Citation1995; Åström et al., Citation2006). Furthermore, Ho and Lin (Citation2003) synthesises a PID controller for a class of SISO systems subject to uncertainty with robust performance, and Silva et al. (Citation2002) considers stabilisation of a first-order time-delay system using a PID controller with a complete set of PID parameters determined.
Although almost all the systems in fact are nonlinear with uncertainties, there exist only a few basic theories of PID control for nonlinear systems (Zhang & Guo, Citation2019). Among those, Chang et al. (Citation2002) studies a class of nonlinear PID control systems based on the Lyapunov approach and proposes a direct adaptive tuning method; Zhao and Guo (Citation2017) proposes a theory on PID controllers for nonlinear uncertain systems, which gives a simple and analytic design method for the PID parameters based on the knowledge of some upper bound, and guarantees the global stability and asymptotic regulation of the closed-loop system. Very recently, an extended PID control is considered by Zhao and Guo (Citation2020) for high-order affine nonlinear uncertain systems, with the semi-global stabilisation of systems and exponential convergence of regulation errors guaranteed under some suitable conditions.
1.2. About model reference tracking 
MRT 

Model reference control, also termed as model following control, appeared in the late 1970s (Young, Citation1978). It regulates the system output to follow a reference model output and has attracted much attention. Duan et al. (Citation2001) and Duan and Zhang (Citation2007) investigate the robust model reference control for multivariable linear systems and descriptor linear systems, respectively, with structural parameter uncertainties. A robust model following control for a class of second-order dynamical systems subject to parameter uncertainties is considered in Duan and Huang (Citation2008). For a class of SISO systems with parametric uncertainties, the model reference control is considered by incorporating a linear ‘modeling error compensation’ in Sun et al. (Citation1994). Cunha et al. (Citation2003) and Yan et al. (Citation2008) investigate the variable structure model reference robust control (VS-MRRC) for linear time invariant systems, where the strong stability, disturbance rejection and nice tracking performance properties are guaranteed. Furthermore, an linear parameter varying (LPV) model reference control scheme is designed in Abdullah and Zribi (Citation2009) for LPV systems. Based on a multi-objective optimisation problem, Gonçalves et al. (Citation2011) proposes robust HH
reference model dynamic output-feedback controllers for uncertain continuous- or discrete-time linear time-invariant systems with polytopic uncertainty.
In addition, some of the recent literature focuses on model reference adaptive control (MRAC). It is used for the systems of which the parameters are unknown and/or change with time, and designs controllers with controller parameter adjustment mechanism by comparing the output of the systems and the reference models (Pathak & Adhyaru, Citation2012). However, this method has the shortcomings of effectiveness for nonlinear systems, and the theories on sensitivity, controllability, observability, stability and robustness need further investigation (Shekhar & Sharma, Citation2018).
1.3. Fully actuated system approaches
As outlined above, the problem of tracking and regulation control in the state-space framework can be only effectively solved for linear systems and certain very special nonlinear ones with strict conditions. While the results for the nonlinear cases are also often limited to certain local senses.
High-order fully actuated (HOFA) models proposed in Duan (Citation2020a, Citation2020b, Citation2021c, Citation2021e), although subject to a full-actuation condition in appearance, are general models for dynamical control systems. A huge advantage of such models is that their full-actuation feature allows one to cancel the known nonlinearities in the system and hence to convert, to a great extent, a nonlinear problem into a linear one. Consequently, the HOFA approaches for control systems design have been demonstrated to be extremely convenient and effective in dealing with nonlinear control problems (Duan, Citation2021a, Citation2021b, Citation2021d, Citation2021g, Citation2021h).
This paper further contributes to the HOFA approaches by treating the problem of tracking and regulation in general nonlinear systems. The contributions are of the following several aspects.
Firstly, the well-known PID scheme is generalised to be applicable to HOFA systems. It is shown that generalised PID scheme guarantees asymptotical tracking of the system output to an arbitrarily prescribed constant or slow time-varying signal in subjection to constant or slow time-varying disturbances. Moreover, the derivatives, of orders up to the system orders, of the system states all converge to zero in spite of the existence of constant disturbances. The last feature eventually provides for the system very smooth and steady state responses.
Secondly, the MRT approach for linear systems is also extended to the HOFA system case. The controller is composed of a feedback term and a feed-forward term, with the latter determined by a generalised Sylvester matrix equation. Asymptotical tracking of the system output to that of the reference model is achieved. When such a design is combined with the proposed generalised PID control design, additionally the difference between the outputs of the system and the reference model can be made to track asymptotically an arbitrary given constant signal, also in the presence of constant system disturbances.
Thirdly, as a huge advantage of the HOFA approaches, the closed-loop systems under both the above mentioned generalised PID controller and the MRT controller are constant linear. This not only assures the desired exponential stability of the systems but also allows us to establish a complete parameterisation of both the two designs. As a consequence, complete and analytical closed-form parametric expressions for all the controller gains are obtained, and the design degrees of freedom provided in the parametric designs can be further utilised to achieve additional system performance.
Lastly, the sub-fully actuated system case is also dealt with. Although many systems in practical applications can be represented by global fully actuated models, there still exist some systems which can be only represented by sub-fully actuated models (Duan, Citation2020a, Citation2021c). Such systems are relatively more difficult to handle due to a problem of feasibility. For both the above mentioned generalised PID control system and the MRT system, feasibility conditions are established for the case of sub-fully actuated systems. It turns out that these feasibility conditions are in fact restrictions on the system initial values.
In the sequential sections, denotes the identity matrix,
denotes the null set, and
represents the complement of the set Θ in set Ω. For a square matrix P,
and
denote its inverse and determinant, respectively, while for a nonsingular matrix P, its condition number is denoted by
. Furthermore, for
and
as in the former papers in the series, the following symbols are used in the paper:
The paper is organised into eight sections. The next section formulates the problems of generalised PID control and MRT control, and the solutions to these two problems are presented in Sections 3 and 4. Section 5 gives parameterisation of the two types of control system designs, while Section 6 further treats the case of sub-fully actuated systems. An illustrative example is fully studied in Section 7, followed by a brief concluding remark in Section 8. The appendix gives the proof of a technical lemma.
2. Problems formulation
2.1. The HOFA model
Consider the following general HOFA system proposed in Duan (Citation2021c):
(1)
(1) which, by our notations, can be also written more compactly as
(2)
(2) where
is the control vector,
is the disturbance vector,
is a constant matrix,
may represent a parameter vector, an external variable vector, a time-delayed state vector, an unmodelled dynamic state vector, etc.;
are a set of integers,
are a set of vectors, with
being a set of integers satisfying
(3)
(3) Further,
with
being a set of nonlinear vector functions, and
is a matrix function satisfying the following full-actuation condition:
Assumption A1
ζ, and
.
The system (Equation1(1)
(1) ) satisfying the above Assumption A1 is called a (global) fully actuated system (Duan, Citation2021c).
Since
we have
Denote
(4)
(4) then it is easy to see
For the above system (Equation2
(2)
(2) ), the following output equation is imposed:
(5)
(5) where
is a known constant full-row rank matrix.
About the considered HOFA model (Equation1(1)
(1) ), or equivalently, (Equation2
(2)
(2) ), the following remarks are made (see also, Duan, Citation2021d).
Remark 2.1
The above HOFA model (Equation2(2)
(2) ) might be easily mistaken to represent a very small portion of systems due to the full-actuation Assumption A1. While as discussed in Duan (Citation2020a, Citation2021c, Citation2021e), it serves as a general model for dynamical control systems. Many systems which are in state-space forms can be converted into HOFA systems (see Duan, Citation2020a, Citation2020b, Citation2021e, Citation2021f), and practical systems can also be modelled as HOFA systems (see the Remark 3 in Duan, Citation2021d).
Remark 2.2
A special case of the above general HOFA model (Equation1(1)
(1) ) is clearly the following:
(6)
(6) which forms the basic part of the system models involved in the problems of robust control, adaptive control and disturbance rejection treated in Duan (Citation2021a, Citation2021b, Citation2021g, Citation2021h).
2.2. Formulation of problems
Basically, in this paper the tracking control in the HOFA system (Equation2(2)
(2) ) is considered. Two circumstances are dealt with.
Firstly, when the design objective is to let the output y track a properly given constant vector we intend to solve the following generalised PID control problem.
Problem 2.1
Under Assumption A1, find for the HOFA system (Equation2(2)
(2) ) a controller in the form of
(7)
(7) such that the closed-loop system is stable and
(8)
(8)
Obviously, represents a term of proportional plus derivative feedback, while
is an integral feedback. Therefore, the above controller (Equation7
(7)
(7) ) is in a generalised PID form.
Secondly, let us consider the case that the design objective is to let the output y track a properly given signal vector generated by the following reference model:
(9)
(9) where
and
are known constant matrices of appropriate dimensions.
Problem 2.2
Under Assumption A1, find for the HOFA system (Equation2(2)
(2) ) a controller in the form of
(10)
(10) such that the closed-loop system is stable and
(11)
(11) where
is generated by the reference model (Equation9
(9)
(9) ),
is any desired constant vector.
Obviously, when does not exist, or equivalently, when
Problem 2.2 reduces to Problem 2.1.
3. Generalised PID control
In this section, the solution to Problem 2.1 is considered.
3.1. Deriving the linear system
Denote
and
then, under the following control input transformation
(12)
(12) the system (Equation2
(2)
(2) ) is turned into the following series of linear systems:
(13)
(13) which can be equivalently written in the state-space form
(14)
(14) where, by our notations,
(15)
(15) Define
then the set of systems in (Equation14
(14)
(14) ) can be more compactly written as
(16)
(16)
3.2. Solution to Problem 2.1
Define the vector
(17)
(17) then we have
(18)
(18)
Combining the above (Equation18(18)
(18) ) with (Equation16
(16)
(16) ), gives the following extended system
(19)
(19) where
(20)
(20) The following lemma gives a necessary and sufficient condition for the controllability of the linear system (Equation19
(19)
(19) ).
Lemma 3.1
The matrix pair is controllable if and only if
(21)
(21) Furthermore, it is not stabilisable if
(22)
(22)
Proof.
Since , are all controllable, it is easy to show that
is also controllable. Therefore, the first conclusion follows immediately from the Theorem 7.3.2 in Duan (Citation2016).
Now let us show the second conclusion. When condition (Equation22(22)
(22) ) is met, partition C as
Then
Clearly, the existence of
adds m−r number of transmission zeros
It follows from the first conclusion that these added transmission zeros are not the controllable modes of the matrix pair
Since they are not (asymptotically) stable ones, the system is then not stabilisable.
The first conclusion in the above Lemma 3.1 implies while the second conclusion further indicates that the system is even not stabilisable when
Therefore, the case of m>r is after all of no necessity of consideration. This clearly gives a very important insight, that is, the maximum number of signals (counting by scalar dimension) that the system can track is not greater than the number of the system inputs.
Under the condition (Equation21(21)
(21) ), a feedback controller can be designed for the above compound system (Equation19
(19)
(19) ) as follows:
(23)
(23) Further denote
(24)
(24) and
(25)
(25) Then, the following lemma can be proven, which gives the property of the above controller (Equation23
(23)
(23) ).
Lemma 3.2
Consider the system (Equation16(16)
(16) ), with the output equation (Equation5
(5)
(5) ), satisfying condition (Equation21
(21)
(21) ). If d is also constant, and
and
are matrices making
Hurwitz, then the controller (Equation23
(23)
(23) ) guarantees
(26)
(26)
(27)
(27) and
(28)
(28)
Proof.
When the controller (Equation23(23)
(23) ) is applied to the system (Equation16
(16)
(16) ), the closed-loop system is obtained as
(29)
(29) where
is given by (Equation24
(24)
(24) ), which can be made Hurwitz by selecting the gain matrices
and
due to Lemma 3.1
Recall that is constant. If the disturbance d is also constant, then, taking the first-order derivative on both sides of (Equation29
(29)
(29) ), yields
(30)
(30) Therefore, when
is Hurwitz, there holds
which immediately gives
(31)
(31) and
(32)
(32) These are, respectively, the conditions (Equation27
(27)
(27) ) and (Equation26
(26)
(26) ).
Using the notations in (Equation25(25)
(25) ), we have
Further, using again the closed-loop system (Equation29
(29)
(29) ), we have, in the frequency domain,
where
Therefore, by the well-known Final Value Theorem,
This is the condition (Equation28
(28)
(28) ). By now the proof is complete.
Combining the above lemma with the results in Subsection 3.1, produces the solution to the generalised PID control problem.
Theorem 3.3
Consider the system (Equation2(2)
(2) ) with the output equation (Equation5
(5)
(5) ). If
Assumption A1 and condition (Equation21
(21)
(21) ) are satisfied;
the disturbance vector d is constant; and
the two gain matrices
and
make the matrix
in (Equation24
(24)
(24) ) Hurwitz,
It is well-known that PID is a very popular control technique, and has been widely and successfully used in various practical processes. The above Lemma 3.2 and Theorem 3.3 reveal theoretically the reason behind such a fact, as given in the following remark.
Remark 3.1
The generalised PID controller (Equation7(7)
(7) ) possesses two advantages:
it guarantees asymptotical tracking to a constant vector under an arbitrary constant disturbance, and often works practically well enough with slow time-varying tracked vectors and disturbances; and
it provides very smooth and steady transient response due to the relations in (Equation27
(27)
(27) ).
As a matter of fact, the relation (Equation27
(27)
(27) ) is really an extra and seldom by-product. For instance, when such a generalised PID controller is applied to a second-order system, besides the velocity signals, the acceleration signals also converge to zero.
3.3. Decoupled design
The above design of the intermediate control vector v based on the overall model (Equation16(16)
(16) ) is a coupled design (see, Duan, Citation2021c). In certain cases, e.g. when
is dependent on only some, but not all,
's and their derivatives, a decoupled design can be proposed, which is simpler in the sense that it deals with certain decoupled linear systems with lower dimensions.
For convenience, let us simply assume that
In this case, instead of converting (Equation14
(14)
(14) ) into a whole system (Equation16
(16)
(16) ), the following subsystem can be particularly considered:
(33)
(33) According to our approach, a PID controller for the system can be designed as
where
and
are two matrices making
Hurwitz. As a result, the asymptotical tracking condition (Equation26
(26)
(26) ) is met.
Regarding the design of the other we can again adopt either the decoupled design or the coupled one. When the coupled design is adopted, a control vector
can be designed by controlling the following system
(34)
(34) where
4. Model reference tracking
In this section, let us consider the problem of MRT control.
4.1. The error system
Let us introduce the following assumption.
Assumption A2
There exist matrices and
satisfying the following two equations
(35)
(35)
In (Equation35(35)
(35) ), there are clearly
equations, and
unknowns. Recall that
and note
we generally have more unknowns than the number of the equations. Therefore, this set of equations generally have more than one solution.
Define the generalised error variables
(36)
(36) then, by taking derivatives of the above variables, and using (Equation5
(5)
(5) ) and (Equation16
(16)
(16) ), we can easily obtain the following lemma.
Lemma 4.1
Under Assumption A2, the system (Equation16(16)
(16) ) with the output equation (Equation5
(5)
(5) ) is equivalent to the following error system
(37)
(37) where
and
are defined as in (Equation36
(36)
(36) ).
4.2. Solutions
In this subsection, two cases are treated separately.
4.2.1. Solution for the case of d = 0
In this case the error system (Equation37(37)
(37) ) becomes
(38)
(38) Choosing the following state feedback controller for this system:
(39)
(39) results in the closed-loop system
(40)
(40) where
(41)
(41) If
makes the above matrix
Hurwitz, then
which implies
Finally, note that combination of (Equation39
(39)
(39) ) and (Equation36
(36)
(36) ) yields
we now obtain the following result about the solution to Problem 2.2.
Theorem 4.2
Consider the system (Equation2(2)
(2) ) with the output equation (Equation5
(5)
(5) ). Let
Assumptions A1 and A2 be met, and d = 0;
be a matrix making
in (Equation41
(41)
(41) ) Hurwitz, and
be given by
(42)
(42)
Then the controller
(43)
(43) guarantees
(44)
(44) and
(45)
(45)
In the above controller (Equation43(43)
(43) ), clearly,
is a state-plus-derivative feedback term, while
is a feed-forward term.
4.2.2. Solution for the case of d being a constant vector
In this case, let us apply Lemma 3.2 to the error system (Equation37(37)
(37) ), and a controller is designed as
(46)
(46) where the feedback gains
and
make the matrix
defined in (Equation24
(24)
(24) ) Hurwitz. In this case, there hold
(47)
(47)
(48)
(48) and
(49)
(49) Substituting the expressions in (Equation36
(36)
(36) ) into (Equation46
(46)
(46) ), gives
(50)
(50) The above process proves the following solution to Problem 2.2.
Theorem 4.3
Consider the system (Equation2(2)
(2) ) with the output equation (Equation5
(5)
(5) ). Let
Assumptions A1 and A2 be met, and d and
be constant vectors;
and
be matrices making
in (Equation24
(24)
(24) ) Hurwitz, and
be given by
(51)
(51)
Then the controller
(52)
(52) guarantees
(53)
(53)
(54)
(54) and
(55)
(55)
Obviously, the three relations (Equation53(53)
(53) )–(Equation55
(55)
(55) ) are, respectively, the interpretations of the relations (Equation47
(47)
(47) )–(Equation49
(49)
(49) ) in terms of the original system variables.
It can be easily recognised that the above MRT result is a generalisation of the generalised PID control result given in Theorem 3.3. The above result clearly reduces to that in Theorem 3.3 when does not exist, or, equivalently,
5. Parameterisation
In this section, parametric solutions to the problems of generalised PID control and MRT control are investigated. Before presenting the parametric expressions of the corresponding controllers, we first give some preliminaries.
5.1. Preliminary lemma
Consider the linear system
(56)
(56) where
and
are the state vector and the control input vector, respectively,
is an external vector; A, B and Γ are real matrices of appropriate dimensions. When a controller for the system is chosen as
(57)
(57) the closed-loop system is obtained as
(58)
(58) where
(59)
(59) If the matrix pair
is controllable, according to Duan (Citation2015, Chapter 3), there exist a pair of right coprime polynomial matrices
and
satisfying the following right coprime factorisation (RCF):
(60)
(60) If we denote
and
then
and
can be written in the form of
(61)
(61) The following lemma performs an important role in this section (see, Duan, Citation2015).
Lemma 5.1
Let be controllable, and
and
be a pair of right coprime polynomial matrices given by (Equation61
(61)
(61) ) and satisfy the RCF (Equation60
(60)
(60) ). Then,
for an arbitrarily chosen
, all the matrices
and
satisfying the following Sylvester matrix equation
are given by
(62)
(62) where
is an arbitrary parameter matrix; and
for an arbitrarily chosen
, all the matrices
and
satisfying
and
(63)
(63) are given by (Equation62
(62)
(62) ), with
and
(64)
(64) with
being an arbitrary parameter matrix satisfying the following constraint:
(65)
(65)
The meaning of Equation (Equation63(63)
(63) ) is very clear. If F is chosen to be a stable matrix, then K turns to be a stabilising gain matrix for the state feedback controller (Equation57
(57)
(57) ). Therefore, the second conclusion in the above Lemma 5.1 has given all the stabilising state feedback controllers for the system (Equation56
(56)
(56) ). The parameter matrix Z in the above lemma represents the design degrees of freedom in the design, and can be properly utilised to achieve additional system performance.
5.2. Right coprime factorisations
It is seen from the above lemma that the pair of right coprime polynomial matrices and
satisfying the RCF (Equation60
(60)
(60) ) is essential. The following two lemmas summarise the several pairs of such polynomial matrices associated with the solution to the Sylvester matrix equation in (Equation35
(35)
(35) ) and the feedback gains in the controllers (Equation7
(7)
(7) ), (Equation43
(43)
(43) ) and (Equation52
(52)
(52) ).
The conclusions of the following lemma can be easily verified (see, also Duan, Citation2021c).
Lemma 5.2
Consider the matrix pairs
and
For
the pair of right coprime polynomial matrices
and
satisfying the RCF
(66)
(66) are given by
(67)
(67)
The pair of right coprime polynomial matrices
and
satisfying the RCF
(68)
(68) are given by
(69)
(69)
The following lemma further gives the RCF associated with the matrix pair .
Lemma 5.3
Let and
be a pair of right coprime polynomial matrices given by (Equation61
(61)
(61) ) and satisfy the RCF (Equation68
(68)
(68) ). Then the matrix pair
given by (Equation20
(20)
(20) ) is controllable if and only if
(70)
(70) In this case, the pair of right coprime polynomial matrices
and
satisfying the RCF
(71)
(71) are given by
(72)
(72) where
and
and
, with
and
are nonsingular matrices satisfying
(73)
(73)
For a proof of the result, refer to the Appendix.
It is obvious that, in the case of (Equation72
(72)
(72) ) becomes
(74)
(74)
5.3. Parametric solutions
Let us firstly consider the Sylvester matrix equation in (Equation35(35)
(35) ).
5.3.1. Solution of G and H
Let and
given by (Equation69
(69)
(69) ) obey the expressions in (Equation61
(61)
(61) ), then, according to the first conclusion in Lemma 5.1, the general expressions of the matrices G and H satisfying the Sylvester matrix equation in (Equation35
(35)
(35) ) are given by
(75)
(75) where
is an arbitrary parameter matrix.
With the matrix G given by (Equation75(75)
(75) ), the second equation in (Equation35
(35)
(35) ) becomes
(76)
(76) which is a linear equation with respect to
With the above understanding, Assumption A2 is clearly equivalent to
Proof
Assumption A2

There exists a parameter matrix satisfying constraint (Equation76
(76)
(76) ).
5.3.2. Solution of feedback gains
Firstly, it can be easily observed that the decoupled design proposed in Section 3.3 is basically a problem as follows.
Problem 5.1
For some find a matrix
such that the following matrix
is Hurwitz.
Secondly, according to Theorem 4.2, finding the feedback gain in the generalised PID controller (Equation43
(43)
(43) ) is mainly to solve the following problem:
Problem 5.2
Find a matrix such that the following matrix
(77)
(77) is Hurwitz.
Thirdly, according to Theorems 3.3 and 4.3, finding the gain matrices and
in the generalised PID controllers (Equation7
(7)
(7) ) and (Equation52
(52)
(52) ) is to solve the following problem.
Problem 5.3
Find a matrix such that the following matrix
is Hurwitz.
All the above three problems can be solved in a parametric manner by using Lemma 5.1 and Lemma 5.2 or 5.3. In the following, let us briefly illustrate the basic ideas in solving Problems 5.2 and 5.3.
To solve Problem 5.2, let and
given by (Equation69
(69)
(69) ) obey the expressions in (Equation61
(61)
(61) ), then according to the second conclusion in Lemma 5.1, the general expression of the gain matrix
is given by
(78)
(78) where
is a stable matrix, and
is an arbitrary parameter matrix satisfying the constraint (Equation65
(65)
(65) ). As a consequence, we have
To solve Problem 5.3, let
and
be a pair of right coprime polynomial matrices satisfying the RCF (Equation71
(71)
(71) ) and possess the following expressions:
(79)
(79) Then according to the second conclusion in Lemma 5.1, the general expression of the gain matrix
is given by
(80)
(80) where
is a stable matrix, and
is an arbitrary parameter matrix satisfying the constraint:
(81)
(81) As a consequence, the following relation holds:
(82)
(82) It is important to emphasise again that the design degrees of freedom represented by
and
can be properly utilised to achieve additional closed-loop system performance (see, e.g. Duan, Citation1992, Citation1993).
6. The sub-fully actuated case
Firstly, let us recall the concept of singular points of sub-fully actuated systems, introduced in Duan (Citation2021c). For simplicity, in this paper let us consider a simpler case and impose the following assumption.
Assumption A3
The matrix in system (Equation1
(1)
(1) ) depends on only the state, that is,
For the general case, please refer to Duan (Citation2021c, Citation2021d).
6.1. Singularity and feasibility
For a sub-fully actuated system in the form of (Equation1(1)
(1) ) satisfying the above Assumption A3, the following concept is essential.
Definition 6.1
If satisfies
(83)
(83) then it is called a singular point of system (Equation1
(1)
(1) ).
Let be the set of all singular points of system (Equation1
(1)
(1) ), that is,
Then the following set
is called the set of feasible points of system (Equation1
(1)
(1) ). In general, the system (Equation1
(1)
(1) ) is called a sub-fully actuated system if
is different from
and is a set with dimension not less than 1 (Duan, Citation2021c).
To implement the controllers (Equation7(7)
(7) ), (Equation43
(43)
(43) ) and (Equation52
(52)
(52) ), the following feasibility condition
(84)
(84) or, equivalently,
(85)
(85) needs to be met. Such a problem can be generally turned into a state constrained control problem and solved via an eigenstructure-based approach.
6.2. State constrained control
It is easily observed that, with our designed PID and MRT controllers, the closed-loop systems are linear constant ones, and generally obey the form of (Equation58(58)
(58) ).
The state constrained control problem can be simply stated as follows:
Problem 6.1
Let be stabilisable, and
be a manifold. For the linear system (Equation56
(56)
(56) ) with
being a constant external input, find a state feedback controller (Equation57
(57)
(57) ) such that the following requirements are met:
the matrix
is Hurwitz; and
the state constraint
is met.
In Duan (Citation2021d), a solution to such a problem based on Lyapunov matrix equations is presented. In this section, let us present an eigenstructure-based solution to the problem.
Clearly, the equilibrium point of the system (Equation58(58)
(58) ) is
(86)
(86) Let
then the system (Equation58
(58)
(58) ) is transformed into
(87)
(87) Applying Lemma 5.1 to system (Equation56
(56)
(56) ), gives the feedback gain
(88)
(88) and eventually, there holds
(89)
(89) For only demonstration, let us consider the case of
(90)
(90) where
are a series of negative real scalars. While the result can be easily generalised into the case that F contains a diagonal real block of the following form:
With F given by (Equation90
(90)
(90) ), the solution to the closed-loop system (Equation87
(87)
(87) ) is given by
which gives
(91)
(91) Therefore, for some
implies
The above process clearly proves the following important lemma about state constrained control.
Lemma 6.2
Let be stabilisable,
be constant,
be the maximum number such that
(92)
(92) and K be the state feedback gain, of the state feedback controller (Equation57
(57)
(57) ), given by (Equation88
(88)
(88) ), with F being a stable diagonal matrix given by (Equation90
(90)
(90) ). Further, if the initial values are chosen to satisfy
(93)
(93) then
(94)
(94)
Recalling (Equation86(86)
(86) ) and (Equation89
(89)
(89) ), we have
(95)
(95) which is dependent on V. Furthermore, the condition (Equation93
(93)
(93) ) is also dependent on
In applications, it is desirable to select the free parameter matrix Z to let
be as big as possible, and
be as small as possible.
6.3. Feasibility conditions
In this subsection, let us apply the above Lemma 6.2 to our generalised PID control and MRT control.
6.3.1. Case of PID control
The purpose here is to provide a solution to Problem 2.1 with the feasibility requirement (Equation85(85)
(85) ) satisfied. The general idea is as follows.
Applying Lemma 5.3, we can obtain a pair of polynomial matrices and
in the form of (Equation79
(79)
(79) ) satisfying the RCF (Equation71
(71)
(71) ). Then according to the second conclusion in Lemma 5.1, the general expression of the gain matrix
can be obtained as in (Equation80
(80)
(80) ). Finally, with the help of Lemma 6.2, the result about generalised PID control of sub-fully actuated systems can be obtained.
The equilibrium point of the closed-loop system is easily obtained as
(96)
(96) where the disturbance d may be substituted by an estimate.
Theorem 6.3
Consider the system (Equation2(2)
(2) ) with the output equation (Equation5
(5)
(5) ). Let Assumptions A2 and A3 and condition (Equation21
(21)
(21) ) be met, and d be absent,
be given by (Equation80
(80)
(80) ), with
being chosen to be a stable diagonal matrix, and
be an arbitrary parameter matrix satisfying the constraint (Equation81
(81)
(81) ). Further, if the initial values are chosen to satisfy
(97)
(97) where
is the maximum number such that
(98)
(98) then the controller (Equation7
(7)
(7) ) for the system (Equation2
(2)
(2) ) guarantees (Equation26
(26)
(26) )–(Equation28
(28)
(28) ) and the feasibility requirement (Equation85
(85)
(85) ).
Proof.
It follows from Lemma 6.2 that, under condition (Equation97(97)
(97) ), there also holds
(99)
(99) Thus we have
(100)
(100) This shows
The proof is done.
6.3.2. Case of MRT control
For simplicity, let us only consider the MRT control scheme without an integration, that is, the case of Problem 5.2, but with Assumption A1 removed, and the additional feasibility requirement (Equation85(85)
(85) ) added. Again, the idea of solving this problem is to adopt the following outline:
Applying Lemma 5.2, gives a pair of polynomial matrices and
in the form of (Equation61
(61)
(61) ) satisfying the RCF (Equation69
(69)
(69) ). Then according to the second conclusion in Lemma 5.1, the general expression of the gain matrix
can be obtained. Finally, with the help of Lemma 6.2, the feasibility condition for MRT in sub-fully actuated systems can be obtained.
Before implementing this idea, let us first impose some assumption on the reference model (Equation9(9)
(9) ). Let
be a set of admissible initial values of system (Equation9
(9)
(9) ), then the following objective set can be defined:
(101)
(101) Theoretically, the reference model (Equation9
(9)
(9) ) is allowed to be unstable. However, such a case is very rare. Thus in this subsection the reference model (Equation9
(9)
(9) ) is restricted to be stable or critically stable. As a consequence, the above set Ω defined in (Equation101
(101)
(101) ) is bounded. Furthermore, since the reference model is often ideally chosen, it is reasonable to require that Ω is far away enough from
.
If represents the closed-cover of Ω, and
represents the distance between the two sets
and
, which is defined by
then the above discussion motivates us to propose the following assumption.
Assumption A4
There exists a and a constant
such that
(102)
(102) and
(103)
(103)
Clearly, (Equation102(102)
(102) ) assumes the boundedness of set Ω, while (Equation103
(103)
(103) ) assumes that Ω is far away enough from
.
With the above preparation, the following result about the MRT control of the sub-fully actuated system (Equation2(2)
(2) ) can be proven.
Theorem 6.4
Consider the system (Equation2(2)
(2) ) with the output equation (Equation5
(5)
(5) ). Let Assumptions A2
, A3 and A4 be met, and d = 0;
be given by (Equation78
(78)
(78) ), with
being chosen to be a stable diagonal matrix, and
be an arbitrary parameter matrix satisfying the constraint (Equation65
(65)
(65) ). Further, if the initial values are chosen to satisfy
and
(104)
(104) where V is given by (Equation78
(78)
(78) ), then the controller (Equation43
(43)
(43) ) for the system guarantees (Equation44
(44)
(44) )–(Equation45
(45)
(45) ) and the feasibility requirement (Equation85
(85)
(85) ).
Proof.
Recall that, under given conditions, the controller (Equation43(43)
(43) ) results in the closed-loop system given by (Equation40
(40)
(40) ), with
Applying Lemma 6.2, we have, due to (Equation104
(104)
(104) ), the following relation:
(105)
(105) On the other hand, since
there holds
. Further, recalling
, we obviously have
(106)
(106) Therefore, using (Equation105
(105)
(105) ) and (Equation106
(106)
(106) ), gives
This clearly implies (Equation85
(85)
(85) ) (see Figure ).
Remark 6.1
It is easily recognised that the feasibility conditions given in Theorems 6.3 and 6.4 may be conservative. In certain cases, e.g. when is dependent on only some, but not all,
's and their derivatives, less conservative initial value ranges can be provided by applying decoupled design approaches (see Section 3.3, and also Duan (Citation2021d)). The next section gives a demonstration of this idea with an illustrative example.
Remark 6.2
The results presented in this subsection are conservative but are strictly correct. While in many cases, the set of singular points is only a low-dimensional hyperplane in the space , in this case, as long as the steady response of the closed-loop system does not intersect with
, the probability that the system response in the transient process intersects with
is very small. This is why some practical applications on control of sub-fully actuated systems simply overlook such a feasibility analysis problem. However, for applications of high-grade security or high cost, a trial and test approach is certainly not acceptable.
7. Illustrative example
7.1. The system model
The Example 6.2 in Duan (Citation2021c) treats a classical example system proposed in Brocket (Citation1983). In the treatment of Duan (Citation2021c), the problem is reduced to the control of a HOFA system. When a disturbance d is added to the system, it appears as follows:
(107)
(107) where
and
is the control vector. Clearly, the set of singular points of the system is
In the Example 6.2 in Duan (Citation2021c), a decoupled design is proposed, which gives a nonconservative feasibility condition. However, with decoupled designs, the design degrees of freedom is dramatically reduced. Now in this section, coupled designs using the PID and MRT control are presented.
By the following control transformation,
(108)
(108) where v is a two-dimensional intermediate control vector, the system is turned into
(109)
(109) which can be converted into the following state-space form:
(110)
(110) where
(111)
(111) The output equation of the system is taken to be
(112)
(112) where
7.2. Generalised PID design
In this section, our aim of the design is to let the system output y regulate at a constant value As a result of Theorem 3.3, the controller finally designed for the system is
(113)
(113) It follows from Theorem 3.3 that
is determined by
(114)
(114) where
(115)
(115)
(116)
(116) and
is a stable real matrix, and
a nonsingular real matrix.
7.2.1. Solution of RCFs
It follows from Lemmas 5.2 and 5.3,
(117)
(117)
(118)
(118) and
(119)
(119) Thus
(120)
(120) Note that
we have
(121)
(121) Thus it follows from Lemma 5.3 that
(122)
(122) which gives
7.2.2. Solution of 
and 

Choose
we obtain
(123)
(123) By minimising the condition number
the parameter matrix
is obtained as
(124)
(124) which corresponds to
Hence
Thus
(125)
(125)
(126)
(126) It can be obtained that
Further choose
(127)
(127) we have
(128)
(128) Note that the distance of
from
is
by (Equation97
(97)
(97) ) the set of feasible initial values is obtained as
(129)
(129)
7.3. Model reference tracking design
Let the coefficient matrices of the reference model (Equation9(9)
(9) ) be taken as
It can be easily observed that this corresponds to
(130)
(130) where ρ and ϕ are two real numbers determined by the initial values of the reference model.
In view of (Equation119(119)
(119) ), we have
(131)
(131)
(132)
(132) thus Assumption A2
, that is,
(133)
(133) gives
(134)
(134) where α and β are two arbitrary real scalars. Therefore, it follows from (Equation75
(75)
(75) ) and (Equation134
(134)
(134) ) that
(135)
(135)
(136)
(136) For simplicity, let us just take
and
as in (Equation125
(125)
(125) ) and (Equation126
(126)
(126) ), respectively, then
is given by (Equation42
(42)
(42) ). Through minimising
the optimal parameters are derived as
Hence
7.4. Simulation results
7.4.1. PID control
When the initial values are chosen as
(137)
(137) it can be easily verified that the condition (Equation129
(129)
(129) ) is met. Corresponding to the cases of d = 0 and
the corresponding simulation results are shown in Figures and , respectively. It is clearly seen from these figures that in both cases the signal
remains the same and tracks
the results clearly support the theoretical conclusions.
7.4.2. Model reference tracking
Again, take and the system initial values as in (Equation137
(137)
(137) ). Furthermore, take the initial value of the reference model as
Then, for the case of d = 0 and
the simulation is also carried out, and the results are shown in Figures and , respectively. It is clearly seen from these two figures that in both cases
and
remain unaltered and
gets closed to
Again, the results coincide with the theories.
7.4.3. Case of slow time-varying d and 

In this subsection, let us further check the effect of the designed controllers when applied to systems with slow time-varying disturbance d and a slow time-varying commend signal . For paper length limitation, here only the results of the generalised PID controller are presented.
Case A: is time-varying only
Consider the case where only the disturbance d is replaced with the following time-varying signal
(138)
(138) while keeping all the other parameters unchanged. The simulation results are shown in Figure . Comparison of Figure with Figure gives the following observation: the time-varying disturbance does affect the variables z and
but it almost has no affection on the steady response of the variable y, as desired.
Case B: Both d and are time-varying
In the case that d is replaced with that in (Equation138(138)
(138) ), and, simultaneously,
is replaced with
while keeping the initial values still the same as in (Equation137
(137)
(137) ), the simulation results are shown in Figure . It is observed that in this case the variable
still follows the signal
closely, as desired.
8. Conclusions
Tracking and regulation is one of the most important design objectives in control systems design. For tracking and regulation in linear systems, the problem has been well solved. While for nonlinear systems, with the widely used state-space approaches the problem is only solved for some very special systems and the results are also often limited to certain local senses.
Parallel to the state-space approaches, HOFA approaches have been recently proposed and demonstrated to be much more effective in dealing with system control problems (Duan, Citation2020a, Citation2020b, Citation2020c, Citation2021a, Citation2021b, Citation2021c, Citation2021e, Citation2021f, Citation2021g, Citation2021h). It is further shown in this paper that, once a general nonlinear dynamical system is represented by a HOFA model, the problem of tracking control can be solved perfectly in the sense that the closed-loop system becomes constant linear, besides realisation of the required asymptotical tracking property. Concretely, the paper has shown the following:
the well-known PID scheme can be easily generalised to suit a HOFA system subject to a constant or slow time-varying disturbance, and to achieve asymptotical tracking of a constant or slow time-varying signal;
an MRT controller also exists for an HOFA system, which guarantees asymptotical tracking of the signal generated by the reference model, and the tracking error remains unchanged when the system is subject to a constant disturbance;
the closed-loop systems resulted in by both designs are constant linear, and the controllers as well as the closed-loop systems can be completely parameterised, with the degrees of freedom properly utilised to further improve the system performance; and
both designs can be generalised to the case of sub-fully actuated systems, and effective feasibility conditions in terms of the system initial values are provided by using the parameterised closed-loop eigenstructure.
Acknowledgements
The author is grateful to his Ph.D. students Qin Zhao, Guangtai Tian, Xiubo Wang, Weizhen Liu, Kaixin Cui, etc., for helping him with reference selection and proofreading. His particular thanks go to his student Tianyi Zhao for help with the simulations.
Disclosure statement
No potential conflict of interest was reported by the author(s).
Additional information
Funding
Notes on contributors
Guangren Duan
Guangren Duan received his Ph.D. degree in Control Systems Sciences from Harbin Institute of Technology, Harbin, P. R. China, in 1989. After a two-year post-doctoral experience at the same university, he became professor of control systems theory at that university in 1991. He is the founder and now the Honourary Director of the Center for Control Theory and Guidance Technology at Harbin Institute of Technology, and recently he is also in charge of the Center for Control Science and Technology at the Sounthen University of Science and Technology. He visited the University of Hull, the University of Sheffield, and also the Queen's University of Belfast, UK, from December 1996 to October 2002, and has served as Member of the Science and Technology Committee of the Chinese Ministry of Education, Vice President of the Control Theory and Applications Committee, Chinese Association of Automation (CAA), and Associate Editors of a few international journals. He is currently an Academician of the Chinese Academy of Sciences, and Fellow of CAA, IEEE and IET. His main research interests include parametric control systems design, nonlinear systems, descriptor systems, spacecraft control and magnetic bearing control. He is the author and co-author of 5 books and over 340 SCI indexed publications.
References
- Abdullah, A., & Zribi, M. (2009). Model reference control of LPV systems. Journal of the Franklin Institute, 346(9), 854–871. https://doi.org/10.1016/j.jfranklin.2009.04.006
- Ang, K. H., Chong, G., & Li, Y. (2005). PID control system analysis, design, and technology. IEEE Transactions on Control Systems Technology, 13(4), 559–576. https://doi.org/10.1109/TCST.2005.847331
- Åström, K. J., & Hägglund, T. (1995). PID controllers: Theory, design, and tuning. Instrument society of America.
- Åström, K. J., Hägglund, T., & Astrom, K. J. (2006). Advanced PID control. ISA-The Instrumentation, Systems, and Automation Society.
- Borase, R. P., Maghade, D. K., Sondkar, S. Y., & Pawar, S. N. (2020). A review of PID control, tuning methods and applications. International Journal of Dynamics and Control, 9, 818–827. https://doi.org/10.1007/s40435-020-00665-4
- Brocket, R. W. (1983). Asymptotic stability and feedback stabilisation. In Differential geometric control theory (pp. 112–121). Birkhauser.
- Chang, W. D., Hwang, R. C., & Hsieh, J. G. (2002). A self-tuning PID control for a class of nonlinear systems based on the Lyapunov approach. Journal of Process Control, 12(2), 233–242. https://doi.org/10.1016/S0959-1524(01)00041-5
- Cunha, J. P. V. S., Hsu, L., Costa, R. R., & Lizarralde, F. (2003). Output-feedback model-reference sliding mode control of uncertain multivariable systems. IEEE Transactions on Automatic Control, 48(12), 2245–2250. https://doi.org/10.1109/TAC.2003.820156
- Duan, G. R. (1992). Simple algorithm for robust pole assignment in linear output feedback. IEE Proceedings-Control Theory and Applications, 139(5), 465–470. https://doi.org/10.1049/ip-d.1992.0058
- Duan, G. R. (1993). Robust eigenstructure assignment via dynamical compensators. Automatica, 29(2), 469–474. https://doi.org/10.1016/0005-1098(93)90140-O
- Duan, G. R. (2015). Generalized Sylvester equations: Unified parametric solutions. CRC Press.
- Duan, G. R. (2016). Linear system theory (Vol. II, 3rd ed.). The Science Press.
- Duan, G. R. (2020a). High-order system approaches: I. Full-actuation and parametric design. Acta Automatica Sinica, 46(7), 1333–1345. (in Chinese). https://doi.org/10.16383/j.aas.c200234
- Duan, G. R. (2020b). High-order system approaches: II. Controllability and fully-actuation. Acta Automatica Sinica, 46(8), 1571–1581. (in Chinese). https://doi.org/10.1080/00207721.2020.1829168
- Duan, G. R. (2020c). High-order system approaches: III. Observability and observer design. Acta Automatica Sinica, 46(9), 1885–1895. (in Chinese). https://doi.org/10.16383/j.aas.c200370
- Duan, G. R. (2021a). High-order fully actuated system approaches: Part V. Robust adaptive control. International Journal of System Sciences, 52(10), 2129–2143. https://doi.org/10.1080/00207721.2021.1879964
- Duan, G. R. (2021b). High-order fully actuated system approaches: Part VI. Disturbance attenuation and decoupling. International Journal of System Sciences, 52(10), 2161–2181. https://doi.org/10.1080/00207721.2021.1879966
- Duan, G. R. (2021c). High-order fully actuated system approaches: Part VII. Controllability, stabilizability and parametric designs. International Journal of System Sciences. https://doi.org/10.1080/00207721.2021.1921307
- Duan, G. R. (2021d). High-order fully actuated system approaches: Part VIII. Optimal control with application in spacecraft attitude stabilization. International Journal of System Sciences. https://doi.org/10.1080/00207721.2021.1937750
- Duan, G. R. (2021e). High-order fully actuated system approaches: Part I. Models and basic procedure. International Journal of System Sciences, 52(2), 422–435. https://doi.org/10.16383/j.aas.c200234
- Duan, G. R. (2021f). High-order fully actuated system approaches: Part II. Generalized strict-feedback systems. International Journal of System Sciences, 52(3), 437–454. https://doi.org/10.1080/00207721.2020.1829168
- Duan, G. R. (2021g). High-order fully actuated system approaches: Part III. Robust control and high-order backstepping. International Journal of System Sciences, 52(5), 952–971. https://doi.org/10.1080/00207721.2020.1849863
- Duan, G. R. (2021h). High-order fully actuated system approaches: Part IV. Adaptive control and high-order backstepping. International Journal of System Sciences, 52(5), 972–989. https://doi.org/10.1080/00207721.2020.1849864
- Duan, G. R., & Huang, L. (2008). Robust model following control for a class of second-order dynamical systems subject to parameter uncertainties. Transactions of the Institute of Measurement and Control, 30(2), 115–142. https://doi.org/10.1177/0142331207078510
- Duan, G. R., Liu, W. Q., & Liu, G. P. (2001). Robust model reference control for multivariable linear systems subject to parameter uncertainties. Proceedings of the Institution of Mechanical Engineers, Part I: Journal of Systems and Control Engineering, 215(6), 599–610. https://doi.org/10.1243/0959651011541337
- Duan, G. R., & Zhang, B. (2007). Robust model-reference control for descriptor linear systems subject to parameter uncertainties. Journal of Control Theory and Applications, 5(3), 213–220. https://doi.org/10.1007/s11768-006-6025-z
- Gonçalves, E. N., Bachur, W. E. G., Palhares, R. M., & Takahashi, R. H. C. (2011). Robust H2/ H∞ reference model dynamic output-feedback control synthesis. International Journal of Control, 84(12), 2067–2080. https://doi.org/10.1080/00207179.2011.633232
- Ho, M. T., & Lin, C. Y. (2003). PID controller design for robust performance. IEEE Transactions on Automatic Control, 48(8), 1404–1409. https://doi.org/10.1109/TAC.2003.815028
- Pathak, K. B., & Adhyaru, D. M. (2012). Survey of model reference adaptive control. In 2012 Nirma university international conference on engineering (pp. 1–6).
- Shekhar, A., & Sharma, A. (2018). Review of model reference adaptive control. In 2018 International conference on information, communication, engineering and technology (pp. 29–31).
- Silva, G. J., Datta, A., & Bhattacharyya, S. P. (2002). New results on the synthesis of PID controllers. IEEE Transactions on Automatic Control, 47(2), 241–252. https://doi.org/10.1109/9.983352
- Sun, J., Olbrot, A. W., & Polis, M. P. (1994). Robust stabilization and robust performance using model reference control and modeling error compensation. IEEE Transactions on Automatic Control, 39(3), 630–635. https://doi.org/10.1109/9.280776
- Yan, L., Hsu, L., Costa, R. R., & Lizarralde, F. (2008). A variable structure model reference robust control without a prior knowledge of high frequency gain sign. Automatica, 44(4), 1036–1044. https://doi.org/10.1016/j.automatica.2007.08.011
- Young, K. K. (1978). Design of variable structure model-following control systems. IEEE Transactions on Automatic Control, 23(6), 1079–1085. https://doi.org/10.1109/TAC.1978.1101913
- Zhang, J., & Guo, L. (2019). Theory and design of PID controller for nonlinear uncertain systems. IEEE Control Systems Letters, 3(3), 643–648. https://doi.org/10.1109/LCSYS.7782633
- Zhao, C., & Guo, L. (2017). PID controller design for second order nonlinear uncertain systems. Science China Information Sciences, 60(2), 1–13. https://doi.org/10.1007/s11432-016-0879-3
- Zhao, C., & Guo, L. (2020). Control of nonlinear uncertain systems by extended PID. IEEE Transactions on Automatic Control, 66(8), 3840–3847. https://doi.org/10.1109/TAC.2020.3030876
- Ziegler, J. G., & Nichols, N. B. (1942). Optimum settings for automatic controllers. Transactions of the A.S.M.E, 64(11), 759–765. https://doi.org/10.1115/1.2899060
- Ziegler, J. G., & Nichols, N. B. (1943). Process lags in automatic control circuits. Transactions of the A.S.M.E, 65(5), 433–443.
Appendix. Proof of Lemma 5.3
Since is controllable, there exists a unimodular matrix
which satisfies
Using the above relation, yields
Therefore,
if and only if
Thus the first conclusion of the lemma follows from the well-known PBH criterion.
From (Equation73(73)
(73) ), it can be obtained that
(A1)
(A1)
(A2)
(A2) While the former is easily seen to be equivalent to
(A3)
(A3) Using the above equations and the given conditions in the lemma, produces
and
Combining the above two aspects, proves that and
satisfy the right factorisation (Equation71
(71)
(71) ).
Next, using a series of row transformations, finally gives
thus the right coprimeness of
and
is also preserved. The whole proof is then completed.