Abstract
In this paper, a data-based neural policy learning method is established to solve the robust tracking control problem of a class of continuous-time systems which have two kinds of uncertainties at the same time. First, the robust trajectory tracking is achieved by controlling the tracking error to zero. The specific implementation strategy is to construct an augmented system including the tracking error and then transform the robust tracking control problem into an optimal control problem by selecting a suitable cost function. Then, a neural network identifier is built to reconstruct the unknown dynamics and a policy iteration algorithm is adopted by using a critic neural network. In this way, the Hamilton–Jacobi–Bellman equation can be solved. Through this learning algorithm, the approximate optimal control policy is obtained and the solution of the robust tracking control problem can be derived. Finally, two simulation examples are proposed to verify the effectiveness of the developed method.
Disclosure statement
No potential conflict of interest was reported by the author(s).
Additional information
Funding
Notes on contributors
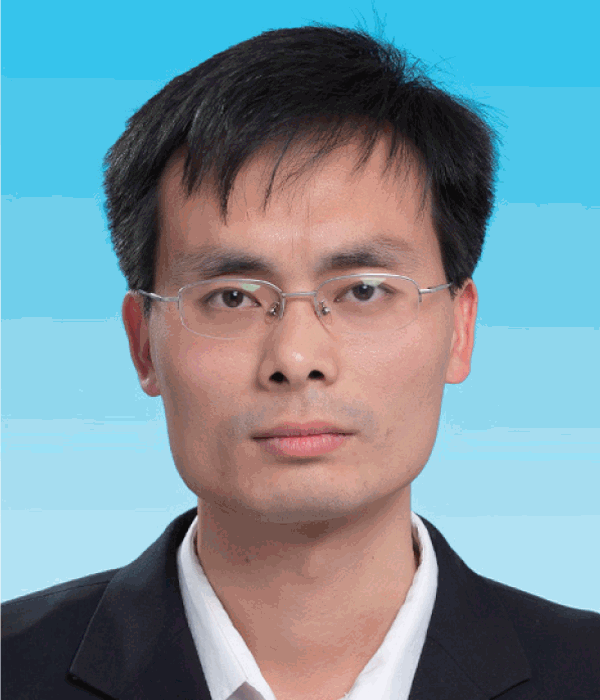
Ding Wang
Ding Wang received the B.S. degree in mathematics from Zhengzhou University of Light Industry, Zhengzhou, China, the M.S. degree in operations research and cybernetics from Northeastern University, Shenyang, China, and the Ph.D. degree in control theory and control engineering from Institute of Automation, Chinese Academy of Sciences, Beijing, China, in 2007, 2009, and 2012, respectively. He was a Visiting Scholar in the Department of Electrical, Computer, and Biomedical Engineering, University of Rhode Island, Kingston, RI, USA, from December 2015 to January 2017. He was an Associate Professor in The State Key Laboratory of Management and Control for Complex Systems, Institute of Automation, Chinese Academy of Sciences. He is currently a Professor in the Faculty of Information Technology, Beijing University of Technology. His research interests include adaptive and learning systems, computational intelligence, and intelligent control. He has published over 120 journal and conference papers, and coauthored 3 monographs. He currently or formerly serves as an Associate Editor of IEEE Transactions on Neural Networks and Learning Systems, IEEE Transactions on Systems, Man, and Cybernetics: Systems, Neural Networks, International Journal of Robust and Nonlinear Control, Neurocomputing, and Acta Automatica Sinica.
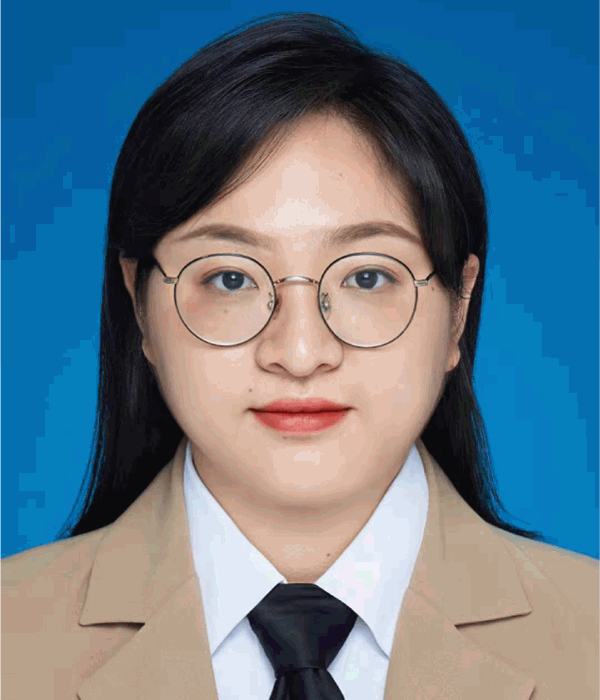
Xin Xu
Xin Xu received the B.S. degree in automation from Beijing University of Technology, Beijing, China, in 2019, where she is currently working toward the M.S. degree in control science and engineering. Her research interests include adaptive dynamic programming, neural networks, optimal control, and reinforcement learning.