Abstract
In this paper, an event-triggered neural critic learning algorithm is investigated to address constrained nonzero-sum game problems with discrete-time nonaffine dynamics. First, in order to ensure the saturation independence of two controllers in the nonzero-sum game problem, we adopt two different boundaries to constrain them respectively. Then, a novel triggering condition is designed to reduce the update times of the controllers, which achieves the purpose of less calculation. It is emphasised that the triggering condition is established based on the iteration of the time-triggered mechanism. Meanwhile, we prove that the real cost function possesses a predetermined upper bound, which realises the cost guarantee of the controlled system. In addition, we prove that the closed-loop system using the developed algorithm is asymptotically stable and that the system state and the sampling state are uniformly ultimately bounded during the process of training neural networks. Finally, two simulation examples are conducted to demonstrate the effectiveness of the proposed algorithm.
Disclosure statement
No potential conflict of interest was reported by the author(s).
Data availability statement
Data sharing is not applicable to this article as no new data were created or analysed in this study.
Additional information
Funding
Notes on contributors
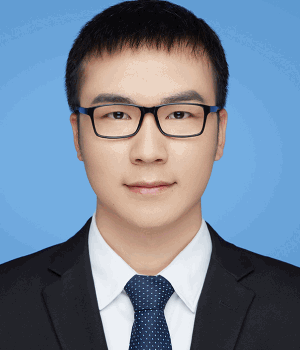
Lingzhi Hu
Lingzhi Hu received B.E. in automation from Heilongjiang University of Science and Technology, Harbin, China, in 2019. He is pursuing the M.E. degree in control science and engineering with the Beijing University of Technology, Beijing, China. His research interests include adaptive dynamic programming, reinforcement learning with industrial applications, and intelligent systems.
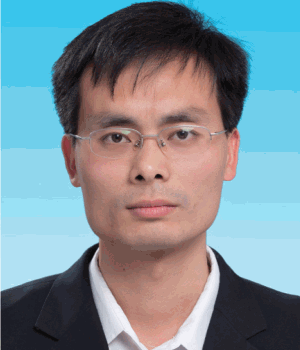
Ding Wang
Ding Wang received the B.S. degree in mathematics from Zhengzhou University of Light Industry, Zhengzhou, China, the M.S. degree in operations research and cybernetics from Northeastern University, Shenyang, China, and the Ph.D. degree in control theory and control engineering from Institute of Automation, Chinese Academy of Sciences, Beijing, China, in 2007, 2009, and 2012, respectively. He was an Associate Professor with The State Key Laboratory of Management and Control for Complex Systems, Institute of Automation, Chinese Academy of Sciences. He is currently a Professor with the Faculty of Information Technology, Beijing University of Technology. His research interests include adaptive and learning systems, computational intelligence, and intelligent control. He has published over 120 journal and conference papers, and coauthored four monographs. He currently or formerly serves as an Associate Editor of IEEE Transactions on Neural Networks and Learning Systems, IEEE Transactions on Systems, Man, and Cybernetics: Systems, Neural Networks, International Journal of Robust and Nonlinear Control, Neurocomputing, and Acta Automatica Sinica.
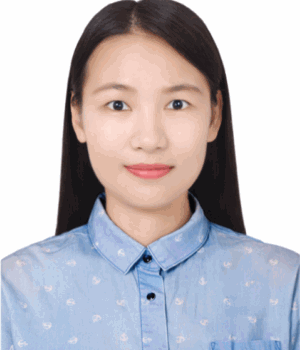
Jin Ren
Jin Ren received B.E. in automation from Henan Polytechnic University, Jiaozuo, China, in 2020. She is pursuing the M.E. degree in control engineering from Beijing University of Technology, Beijing, China. Her research interests include adaptive dynamic programming, reinforcement learning with industrial applications, and intelligent systems.
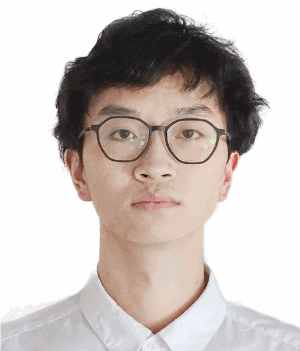
Jiangyu Wang
Jiangyu Wang received B.E. in automation from Tianjin University of Technology, Tianjin, China, in 2021. He is pursuing the M.E. degree in control science and engineering with the Beijing University of Technology, Beijing, China. His research interests include adaptive dynamic programming, reinforcement learning with industrial applications, and intelligent systems.
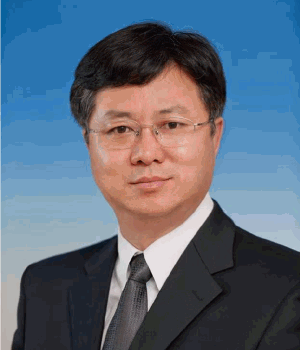
Junfei Qiao
Junfei Qiao received the B.E. and M.E. degrees in control engineering from Liaoning Technical University, Fuxin, China, in 1992 and 1995, respectively, and the Ph.D. degree from Northeastern University, Shenyang, China, in 1998. He is currently a Professor with the Faculty of Information Technology, Beijing University of Technology, Beijing, China, where he is also the Director of Beijing Laboratory of Smart Environmental Protection. His current research interests include neural networks, intelligent systems, self-adaptive systems, and process control.