Abstract
This paper investigates the parameter estimation of errors-in-variables Wiener (EIV-W) nonlinear systems. In such nonlinear systems, both input and output contain interference noises, and some intermediate processes are also interfered by noises. The hierarchical technology is applied to decompose the whole system into two subsystems firstly. For the linear subsystem, in order to obtain unbiased estimates of model parameters, a bias compensation method is introduced. Then, the bias-compensated least squares (BLS) algorithm is proposed. For the nonlinear subsystem, on the basis of particle swarm optimisation (PSO), the fuzzy control technology is added to improve the ability of jumping out of the local optimum. Thus, a bias-compensated least squares and fuzzy PSO based hierarchical (BLS-FPSO-H) method is derived at last. In simulation, a numerical example and a case study about the carbon fibre stretching process are implemented. Results indicate that the BLS-FPSO-H algorithm can effectively identify EIV-W nonlinear systems, the convergence speed and identification accuracy are greatly improved than the basic PSO method and some other PSO variants.
Disclosure statement
No potential conflict of interest was reported by the author(s).
Data availability statement
This manuscript has no associated data.
Additional information
Funding
Notes on contributors
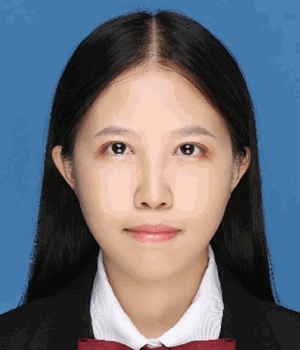
Tiancheng Zong
Tiancheng Zong was born in Nantong, China, in 1994. She received the B.Eng. degree from the School of Electrical Information Engineering, Jiangsu University, Zhenjiang, China, in 2017. She is currently pursuing the Ph.D. degree from the School of Electrical Engineering, Nantong University, Nantong, China, since 2020. Her current research interests include system identification and parameter estimation.

Junhong Li
Junhong Li received her Ph.D. degree in Control Theory and Control Engineering from Jiangnan University, Wuxi, China, in 2014. She is presently working as a Professor in the School of Electrical Engineering, Nantong University, Nantong, China. Her current research interests include system identification, parameter estimation, and system modeling.
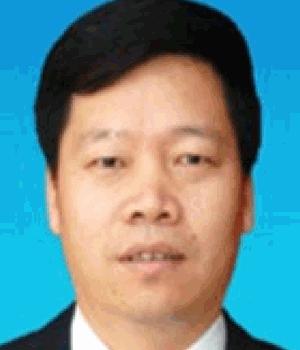
Guoping Lu
Guoping Lu received the B.S. degree from the Department of Applied Mathematics, Chengdu University of Science and Technology, Chengdu, China, in 1984, and the M.S. and Ph.D. degrees in applied mathematics from the Department of Mathematics, East China Normal University, Shanghai, China, in 1989 and 1998, respectively. He is currently a Professor with the School of Electrical Engineering, Nantong University, Jiangsu, China. His research interests include singular systems, multi-agent systems, networked control, and nonlinear signal processing.