Abstract
In this paper, the decentralised tracking control (DTC) problem is investigated for a class of continuous-time large-scale systems with external disturbance by utilising adaptive dynamic programming (ADP). Firstly, the DTC problem is solved by designing corresponding optimal controllers of the isolated subsystems, which are formulated with N augmented subsystems consisting of the tracking error and the reference trajectory. Then, considering the external disturbance, we can effectively construct the DTC scheme by means of adding suitable feedback gains to the optimal control strategies associated with each augmented tracking isolated subsystems (ATISs). Due to the approximate nature, a series of critic neural networks are constructed to solve the Hamilton–Jacobi–Isaacs equation, so as to derive the estimation of the Nash equilibrium solution containing the optimal control strategy and the worst disturbance law. Herein, a modified weight updating criterion is developed by employing a stabilising term. Consequently, we remove the requirement of initial admissible control in the proposed algorithm. After that, stability analysis of the ATIS is performed through the Lyapunov theory, in the sense that tracking states and weight approximation errors are uniformly ultimately bounded. Finally, an experimental simulation is demonstrated to ensure the validity of the proposed DTC scheme.
Data availability statement
Data sharing is not applicable to this article as no new data were created or analysed in this study.
Disclosure statement
No potential conflict of interest was reported by the author(s).
Additional information
Funding
Notes on contributors
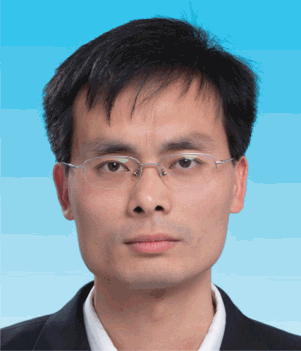
Ding Wang
Ding Wang received the B.S. degree in Mathematics from Zhengzhou University of Light Industry, Zhengzhou, China, the M.S. degree in Operations Research and Cybernetics from Northeastern University, Shenyang, China, and the Ph.D. degree in Control Theory and Control Engineering from Institute of Automation, Chinese Academy of Sciences, Beijing, China, in 2007, 2009, and 2012, respectively. He was a Visiting Scholar with the Department of Electrical, Computer, and Biomedical Engineering, University of Rhode Island, Kingston, RI, USA, from December 2015 to January 2017. He was an Associate Professor with The State Key Laboratory of Management and Control for Complex Systems, Institute of Automation, Chinese Academy of Sciences. He is currently a Professor with the Faculty of Information Technology, Beijing University of Technology. His research interests include adaptive and learning systems, computational intelligence, and intelligent control. He has published over 120 journal and conference papers, and coauthored three monographs. He currently or formerly serves as an Associate Editor of IEEE Transactions on Neural Networks and Learning Systems, IEEE Transactions on Systems, Man, and Cybernetics: Systems, Neural Networks, International Journal of Robust and Nonlinear Control, Neurocomputing, and Acta Automatica Sinica.
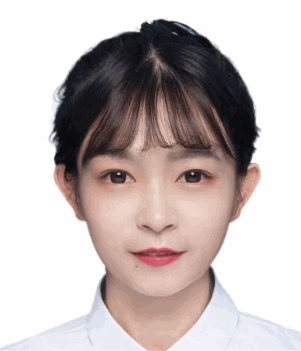
Wenqian Fan
Wenqian Fan received the B.E. degree in Automation from Shanxi University, Taiyuan, China, in 2021. She is currently pursuing the M.E. degree in Control Engineering at the Beijing University of Technology, Beijing, China. Her research interests include adaptive dynamic programming, intelligent control, decentralised control, and related applications.
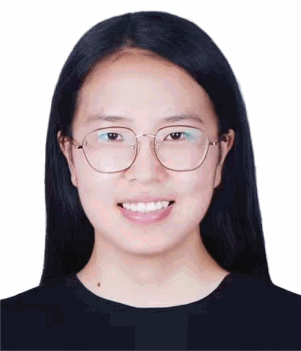
Menghua Li
Menghua Li received the B.E. degree in Automation from Henan Polytechnic University, Jiaozuo, China, in 2020. She is currently pursuing the Ph.D. degree in Control Science and Engineering with the Beijing University of Technology, Beijing, China. Her research interests include adaptive dynamic programming, intelligent control, differential games, and related applications.
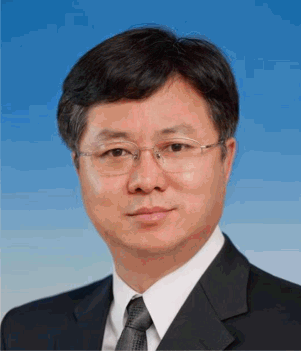
Junfei Qiao
Junfei Qiao received the B.E. and M.E. degrees in Control Engineering from Liaoning Technical University, Fuxin,China, in 1992 and 1995, respectively, and the Ph.D. degree from Northeastern University, Shenyang, China, in 1998. He is currently a Professor with the Faculty of Information Technology, Beijing University of Technology, Beijing, China, where he is also the Director of the Beijing Key Laboratory of Computational Intelligence and Intelligent Systems and Beijing Laboratory of Smart Environmental Protection. His current research interests include neural networks, intelligent systems, self-adaptive systems, and process control.